What Business Stakeholders Want to See from the ML Teams
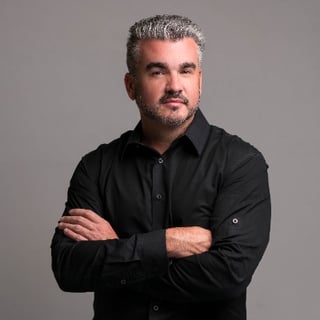
Peter Guagenti is the President and Chief Marketing Officer at Tabnine. Guagenti is an accomplished business leader and entrepreneur with expertise in strategy, product development, marketing, sales, and operations. He most recently served as chief marketing officer at Cockroach Labs, and he previously held leadership positions at SingleStore, NGINX (acquired by F5 Networks), and Acquia (acquired by Vista Equity Partners). Guagenti also serves as an advisor to a number of visionary AI and data companies including DragonflyDB, Memgraph, and Treeverse.
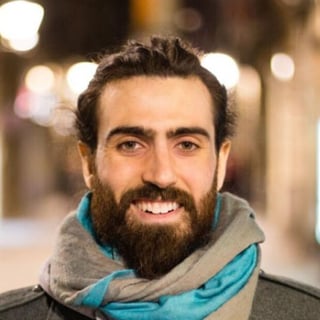
At the moment Demetrios is immersing himself in Machine Learning by interviewing experts from around the world in the weekly MLOps.community meetups. Demetrios is constantly learning and engaging in new activities to get uncomfortable and learn from his mistakes. He tries to bring creativity into every aspect of his life, whether that be analyzing the best paths forward, overcoming obstacles, or building lego houses with his daughter.
Peter Guagenti shares his expertise in the tech industry, discussing topics from managing large-scale tech legacy applications and data experimentation to the evolution of the Internet. He returns to his history of building and transforming businesses, such as his work in the early 90s for People magazine's website and his current involvement in AI development for software companies. Guagenti discusses the use of predictive modeling in customer management and emphasizes the importance of re-architecting solutions to fit customer needs.
He also delves deeper into the AI tools' effectiveness in software development and the value of maintaining privacy. Guagenti sees a bright future in AI democratization and shares his company's development of AI coding assistants. Discussing successful entrepreneurship, Guagenti highlights balancing technology and go-to-market strategies and the value of failing fast.
Join us at our first in-person conference on June 25 all about AI Quality: https://www.aiqualityconference.com/
Peter Guagenti [00:00:00]: I'm Peter Guagenti. I'm president and chief marketing officer of tab nine. Tab nine is the AI coding assistant that you control. I am a coffee addict, and I am usually, if you find me at a coffee shop, it'll be a dry cappuccino. At home, it's just black coffee. Sympathy.
Demetrios [00:00:16]: Welcome back to another mlops community podcast. I am your host, Demetrios. Today we're talking with Peter G. Wow. I loved it. Okay, I'm gonna be straight with you all. I usually say no to people that reach out to me. And if they are not engineers or they do not have a technical background, I usually will say no.
Demetrios [00:00:39]: Not right now, maybe later. And later never comes. Let's be honest, this is the first time that I had someone from a marketing department on the podcast. But I do not look at Peter as a marketer. He talked about his whole life and how he started in tech and how he went through so many different companies, building companies, selling companies, joining companies. And the reason I wanted to have him on here was because of his diverse range of experience. Right now, he's a marketer, but he's been in production. He's led teams that dealt with ML and AI.
Demetrios [00:01:22]: He's also been the CEO of a company that scales from 20 to 300 people. So I felt like he had a lot to offer. This was not sponsored in any way. I'm going to come right out and say it. I was not held with a gun to my head to have him on. It was my choice. I really wanted to see what we could get into in this conversation, and I am very glad that we did, because he gave me some antidotes that I was needing to hear. I really appreciated the conversation.
Demetrios [00:01:54]: I would also love to hear what you all thought about it. I know there's been a few people that have reached out to me that have asked about bridging the barriers between the technical teams and the business teams and how to make sure that all stakeholders are happy. When you are dealing with data, data products, machine learning products, this feels like it is a conversation for all of those people that have reached out to me asking for that. If you just want to hear the nitty gritty on the tech and on what the newest, latest and greatest tools and methods, architectures, system designs are, then we've got a whole lot of other material for you. But if you're interested in getting some, some insights around how to build a more effective team when it comes to technical and business stakeholders, this is what we got into today. There were so many nuggets in here, I can't even begin to list them off because it will take me at least 20 minutes. And I think it's better that we just get into the conversation. I will drop this bomb on you.
Demetrios [00:03:06]: Peter G. Is hiring. The company is hiring for engineers, machine learning engineers, like crazy right now. They're growing. They're doing so well. And you may be using them. You may have heard of them. They are a coding solution.
Demetrios [00:03:23]: It seems like the coding space, the AI generated code space, is one that is blowing up right now. Just on this podcast. In the last eight months, we've had four people that are doing something in the code generation space, and none of those people worked at Copilot. The big name in this space. What I love about this space is that all of these different companies are having to differentiate themselves in one way or another. I know there's a lot of people out there that are using coding tools and coding assistants to help them get more done. So if you're that person and you've been using it, what I really would like to hear from you is how much of your code stays in the code base four weeks after you merge that pr. That's what I really want to know because we got into a bit of a survey that just came out recently, today, actually, on how much of the code that is accepted from GitHub copilot ends up having to go and be completely rewritten four weeks after it is accepted.
Demetrios [00:04:42]: So if you're using some copilot or some coding assistant, let us know. I want to know, is it worth the hassle? Does it really power up all of your abilities? Or is it something that you have to go back and refactor once or twice a week? Let's get into this conversation with Peter. Hope you all enjoy. And as always, it would mean the world to me if you share this with just one friend. Just one friend. Let him know. Mlops community, we out here doing it. Let's get into this combo.
Demetrios [00:05:24]: Peter, we gotta start, man, with the exact same way that I started the call with you a week ago. Tell me about that skateboard, because it is the centerpiece. I can't stop staring at it.
Peter Guagenti [00:05:35]: Okay, so I was a derelict as a teenager. I was a sponsored skateboarder as a teenager, grew up in New York City, and skateboarding was my outlet. Actually taught me creativity, actually. That community taught me diy and entrepreneurialism. And so its always been my roots. And the board specifically behind me, its a recreation of a very famous skater, this guy, Mike Valali, out of the east coast. It was one of the first double ended boards that was sort of the shape that became the primary shape all the skaters used. And so when he reissued it, he did a limited run, autographed and numbered by him.
Peter Guagenti [00:06:14]: I had to do it. I had to do it. I had the original of that deck. I used the hell out of it and ended up in a bin, I was sure, at some point. So it was great to get the recreation of it.
Demetrios [00:06:26]: Were you skating pools or parks or handrails? What are you talking here?
Peter Guagenti [00:06:32]: Yeah, I was a street skater, so I grew up in New York City. So I was a skater in the late eighties, early nineties, sort of at the heyday of street skating. It was street skating, so everyone was born. This is pre X games. They did the first X Games just as I was getting out of the sport. So this is when skateboarding was not cool. It was sort of the dead zone in between the era and the X Games era. But a lot of folks, you know, like, a lot of the kids I grew up with, these are the founders now of supreme.
Peter Guagenti [00:07:01]: These are the guys who ended up running skateboarding at the big companies like Levi's and Adidas and others. Um, so it was. It was a great hour to come up, right? It really was.
Demetrios [00:07:12]: So now, you said it taught you entrepreneurship. You had and have had and are continuing to have a bit of a run when it comes to starting companies. Maybe let's just start there. Like, when did you first get the bug? What was the first company? Break it down for us. Yeah.
Peter Guagenti [00:07:30]: I like to joke that I was born an entrepreneur. Even when I was a skater, I always have a little side hustle. I did. I did a little clothing brand and a skateboard deck brand for a little bit. That was just low production. Sold in the local shops and in the tri state area in New York. But when I was in school, so I was in university in the mid nineties, and when I was in school was the birth of the web, right? It was the start of all this stuff. And I had been doing.
Peter Guagenti [00:07:55]: I had been paid more through school working as a photojournalist, and was doing content creation and other things, and had an NEA grant for a publication that I was doing. Someone came up to me at a computer lab in 94 and said, hey, you should put this on the Internet. You reach such a bigger audience. I was like, what the hell is the Internet? It was 1994, so by summer of 95, I was doing a bunch of web development, and I got an opportunity to do some contract work. So I built the first website for People magazine, did a bunch of work for some of the record labels or other media companies in New York. So in 96, I ended up starting a digital agency. So two worlds simultaneously building, building websites, building web applications, doing sort of the earliest hours of digital transformation, and then doing digital marketing. That was a very new thing.
Peter Guagenti [00:08:47]: I built and sold that company at a year and a half and then went and did it again. So sold that company, real holding company, and then moved to the west coast, joined a group of guys who were trying to do the same thing and took that company for 20 to almost 300 over a few years and sold that to one of the big holding companies, and that was it. That could have sort of gave you the bug. So I've been in tech now for almost 30 years, just a little shy of 30 years. Always in digital transformation, always in transformative technology, and loved it. I've always loved it. So fast forward to today. I was in services businesses for years, then switching to software companies.
Peter Guagenti [00:09:29]: I've been in some companies that folks would know. I was early employee at Acquia behind the Drupal open source project. That was a huge, huge win for us. I was at Nginx and built that company up from just a russian open source project to being the powerhouse that they became. I've been in data companies, AI companies, and now I'm at an AI for software developer company, and then I'm an investor and advisor at a number of companies and mlops and AI and in other core parts of data infrastructure, which I think is really interesting.
Demetrios [00:10:06]: It always amazes me when I hear these stories about people back in the day building websites and how easily it was that these gigantic companies were just letting kids build their websites, because it was almost like the Internet was so low stakes that you were like, yeah, all right, we kind of need something there you could go. And I can only imagine what the conversations you had with people magazine were like, hey, we should probably get you a website, right? You want that?
Peter Guagenti [00:10:36]: And actually, it wasn't as low at stakes as you might think. I remember when I first put people.com live, I convinced them in 95, I convinced them not just to put the regular publication up, but to do custom content, which was radical at the time. And their big thing at the time was sexiest man alive, sexiest woman alive. They're all these great things. And I convinced them that we should put up the extended galleries of these people because they have all these great photos that they took. So I put up extended galleries of these people, not just what was in the publication, which was just one photo of each. And we got over a million uniques in one week on the property, which in 95, there were not that many people on the freaking Internet. And there was not that many people with actually fast enough access to be looking at all of this stuff.
Peter Guagenti [00:11:27]: So it was not as low stakes as you would think it is. Although it is really funny. I was 18 years old when I did that. I think about it now, like, there's no way in hell a top 20 media brand would let some 19 year old get access to their platform nowadays. But at the time you nailed it, there were very few of us who understood it. There were very few of us who were doing it. I definitely was more mature than my years at the time, but a lot of it was just, I was in a space that was really interesting and exciting, and I was one of a limited number of people who was and who cared about it. I'll tell you, it's easy to look back now and yourself and maybe many of your viewers are folks who aren't even, they weren't even born when the browser started, right? But I remember in the mid nineties, the nineties, having conversations with CEO's and saying, this is the future.
Peter Guagenti [00:12:22]: This is not just another channel. We are going to do everything through the Internet, because why wouldn't we, right? And it was impossible for them to understand. And then you fast forward just ten years and you think about the whole world flipped on its ear, particularly when the iPhone came out, because the iPhone was not a phone. The iPhone was the first truly personal computer, right? It was, it was the fulfillment of steam jobs vision, you know, 30 years earlier, of putting a computer in everybody's hands, right? It just happened to literally be in your hand, right? And so, you know, I remember going through those, those errors, and I feel like we're going through the same thing with AI right? Now. I've been at AI companies now for eight years, and I remember having these conversations even four or five years ago, like, you don't understand. AI is going to change everything. Every app will change, every business will change, all of our processes will change. And people will tell me crazy, right? There's no way.
Peter Guagenti [00:13:14]: And I think now we're finally starting to see it.
Demetrios [00:13:16]: I can only imagine what the hosting costs for the people.com extended galleries were.
Peter Guagenti [00:13:23]: They were.
Demetrios [00:13:25]: So I agree with everything else you were saying. So forgive me for continuing to harp on this in the nineties. But that is probably ridiculous.
Peter Guagenti [00:13:35]: Oh, yeah, no, they had their own data center. They had their own data center. It was, it was pretty remarkable. I'll tell you just how you think about like DevOps. I mean, how far DevOps has come and even scripting, like I used to actually have to be in the office at 910 o'clock at night to push the site live every week.
Demetrios [00:13:55]: Then moved to California, got involved in the same kind of thing, built another company, sold it. You thought, hey, it worked once, why not test my luck, see if it works twice? Built the company, gigantic company and up to 300 people. Impressive stuff. I know you mentioned having a few tips and tricks when it comes to selling companies and teaching. Almost like helping us learn from your experience.
Peter Guagenti [00:14:24]: Yeah.
Demetrios [00:14:24]: Of selling companies, building selling companies, particularly selling companies, is quite interesting. I can imagine. And especially in this environment, maybe there are companies out there that have raised too much or that are just ready to get out of the game.
Peter Guagenti [00:14:41]: Yeah.
Demetrios [00:14:42]: What are things that you learned when you sold your various companies?
Peter Guagenti [00:14:46]: Yeah. Well, you know, the craziest thing about this is you never go into a company, expect them to sell it. Right. That's never the thing. The first agency I sold, I knew the company I sold to was inside of one of the big advertising holding companies. When they were starting to roll all these companies together and they were friends, they knew me, they knew my work, they knew my team. I had a team of 30 people doing really interesting work, which is actually how I was able to sell it and not stick around because they knew what they were getting, because they knew me really well and they knew my people. And the same thing with the second agency sale, I was actually pitching services to a big Fortune 50 brand, actually.
Peter Guagenti [00:15:30]: And it was in their offices and it was myself and my CEO and a couple others. And we won the business. But in the room was a senior executive from their traditional ad agency who actually was responsible for M and A. And so she called us a week later and said, I was really impressed with you guys. You're amazing. Would you be open to us acquiring a piece of the business? We want to expose you to more people. We want to get you into other accounts. We want to help you scale and we think we could do better together.
Peter Guagenti [00:16:03]: These things happen when you least expect them. Software was different. Acquia was a sale of private equity. And it was a good sale, right? It was. Vista ended up acquiring the company. The company had built a really strong independent business. It was cash flow positive, but was missing that next level of scale that it wanted. Vista came in and it was a great thing.
Peter Guagenti [00:16:27]: Or Nginx was a similar story. The company had been scaling, it was growing at a healthy rate, but there were all these big install base companies that were in controlling that category in the Fortune 500 and could walk Nginx into 50 times the number of customers that they could do on their own. And so the best way to sell a company is to not try to sell a company. The best way to sell a company is actually to go and build a healthy, independent business that's differentiated in space and has customers who love them. This is the secret of every business. The purpose of a business is not to create a product. The purpose of a business is not to create revenue. The purpose of a business is to create and keep customer relationships.
Peter Guagenti [00:17:07]: And if you have customers who love you and you have deep relationships with them, you will generate profit, you will generate healthy revenue, and you will have an attractive business that somebody will want to be part of. So I think that's the focus now when you get to a level of Duress where you need to sell, which I think is happening right now, I think a lot of people raise money to try to build businesses. It was so easy to raise capital even if the idea wasn't good. Right. Or so easy to raise capital even if maybe you didn't have the skills to do it. That's a fair thing to admit, right? As an entrepreneur, I have my strengths. I also have things that I'm terrible at. Some people don't make it because they can't get the right staff.
Peter Guagenti [00:17:44]: Maybe they don't actually get to that breakthrough. That's a different point then. My best advice to people when they're in that situation where they have to sell is if you still love your baby, you still want it to thrive, then reach out directly to the companies that you think that your team and your product could thrive in. I've seen that happen. I've had some companies that I've advised and invested in over the years that had to do those sales. I had an AI company that did that. But their thing was they're super happy with where they ended up because the reality is they're surrounded by people who get their vision. They're surrounded by people who really understand them and understand what they're trying to do.
Peter Guagenti [00:18:22]: And they were able to keep chasing their dream inside of a large company instead of trying to do it on their own inside of a small software company.
Demetrios [00:18:31]: Yeah. And can you speak a little bit more to people not having the skillsets to execute on their vision. You mentioned not being able to recruit the talent as being one. I've been through my fair share of startups, and I feel like each time I encounter a new problem as to why. But I also am kind of backseat driving. Right? Like, I don't have the stress or the pressure of millions of dollars on my back. So it's easy for me to be like, well, this guy doesn't know what the fuck he's doing, or this person is way out of line with how they're running this business, because like, I, I just can't know what I would do in that situation. And so I feel like you've seen, seen it firsthand, and maybe there are things that you have felt as you developed as an entrepreneur that you recognize now, 20 years ago, you did not have that skill set.
Peter Guagenti [00:19:33]: Yeah. I'll give you my sort of foundational theory of successful startups. All right? If you're going to be in a tech company, then there's two core functions that every person in a software startup needs to fall into, and you need both equally, they both need equal weight, and they're hackers and hustlers. And what do I mean by that? So it's technology people and go to market people, right? But more importantly, it's the right mindset of technology people and go to market people. Hackers say yes, right? You need people in a technology role, in startups that aren't going to tell you all the reasons why something can't be done. They're going to sit there and hustle and figure out, like, what is the way I do make it happen. How do I make that real? Because the act of creating a business is the act of generating something from nothing. And if it was an easy thing to generate, that a business would already exist, somebody will already be doing it.
Peter Guagenti [00:20:36]: Right? And so you have to embrace this mindset of, I'm going to make it happen no matter what. And that sort of hacker mindset, not the negative breaking systems, hacker definition, but I'm going to just dIY until it's real. That mindset, I'll tell you, I've been able to work with some really, really remarkable, remarkable computer scientists over the course of my career, some of the top 1%, and they all shared that. They all shared that mindset of, I can see the way forward, I believe in it, and I will bring it to life. I will reach hurdles along the way, but I will come over those hurdles and I will make it happen where I feel like software has gone wrong for the last. I mean, gosh, it's been at least 1015 years now was the diminishing of the go to market side of the house, right. Because there's this whole thing of Mark Andresen was famous for pushing technical founder CEO's, but if you come from a technical background, you don't know how to sell, you don't know how to market. In fact, I would always argue with some of my peers at some of the tech companies I've worked at as a software engineer.
Peter Guagenti [00:21:50]: Your world is math and physics. Your world is formulas that balance out, right? They come down to a simple thing, but sales and marketing is psychology and sociology, so the formulas don't balance out. And in fact, you don't really know 100% for certain why anything happens. Right. You know, and an example I just always use of my technical founders is, you know that higher education rates in a society reduce crime rates, right? Okay. No one's been ever able to actually show you a direct causation in that. No, no one's ever been able to actually trace that out. Right.
Peter Guagenti [00:22:28]: But it's just two data points. That's what it is. The marketing and sales is the same way. Why did you know? If I spend money on awareness advertising, the business grows. Can I show a direct correlation? No. Until I can put a chip in someone's brain and keep track of everything they've seen and felt, I'll never know for sure.
Demetrios [00:22:46]: Right.
Peter Guagenti [00:22:47]: But you have to have both sides of those coins. And the hustler mindset says, I'm going to build a brand, I'm going to build a business, I'm going to make money no matter what. And the irony of all of this is the most successful businesses in technology over the last 50 years usually were not the best product. They were the best go to market. Very rarely have we seen product that won, very rarely. The iceone being another great example of best product and best go to market combined. But I guarantee if you look back over the history of even products youve used, I love that thing, and they didnt make it right. This other company ended up taking the Caligori right 100%.
Demetrios [00:23:30]: There's the graveyards are littered with incredible products. And I find it funny too, like just reminiscing on a lot of conversations that I've had with startup founders and how they're very much like, all right, we're just going to product, product, product, and then once we have the product right, then we'll turn on the go to market like it's just a light switch that you can flip on and off, and it's like you need to be kind of thinking about that at the same time. You know, it's not something that you can just turn on and. And then all of a sudden, the leads just come funneling in. Yeah. So it is. It is interesting to think about that. And on the other side, what you're talking about with the hacker mindset, especially in the startups, I have seen that, and I think those people, when you encounter them in startups, it's a joy to work with them, because a lot of times what you'll get are the other type.
Demetrios [00:24:26]: That's like going to tell you all the reasons why we're resource constrained, and you can't do that thing. That crazy idea that you came up with at 06:00 on a Thursday night when everybody was having dinner together, right? It's like, no, that's not possible. But then you find that person on the team that's like, oh, yeah. Like, hold on, I'll see if I can throw something together. I think we could do it maybe like this. Yeah. Next thing you know, it's happening. And like you said, you're literally creating something from absolutely nothing.
Demetrios [00:24:56]: It was an idea on a Thursday while you were having some Dal and indian food, you know, like, and then Friday, it was kind of in motion, and then next Tuesday, it's actually a thing that you're talking about and you're touching, and maybe you're demoing.
Peter Guagenti [00:25:13]: So I've run a bunch of different functions in my career. I re product for years at companies, and there's two types of product people in companies. There are those who come bottoms up. They're usually software engineers, or engineers by degree, and they think requirements and features, and they sort of build products from the foundation up. And then there are go to market product people, right? There are the people who their mindset is, there is white space in the market, there is a need in the market. I understand enough of how the technology works that I can see a path forward. I was always that guy. Now I was.
Peter Guagenti [00:25:50]: I broke code early in my career. I am very technical. I probably would have been a software engineer had I had a different upbringing and knew that was an option. Right. I probably would have done something else. Instead. I became osler instead of hacker. But what's really interesting is I think there's a certain amount of joy in knowing enough about the technology to know that it is possible, but not so much that you stop yourself and say, well, but it's really hard, or, oh, but this other thing is in the way.
Peter Guagenti [00:26:17]: There's a certain amount of value and ignorance in that, and that is entrepreneurialism. Entrepreneurialism at its core is just looking and saying, I'm going to make it happen. I believe in it enough. You have to have this sort of suspension of disbelief where you're not afraid of failure, you're not afraid of getting it wrong, you're not afraid of hitting a wall. You just say, I want it to be, so I'm going to do it. What's really interesting is my earliest forays into machine learning and AI products came from that because I understood I was trying to accomplish this thing. But if I could get an algorithm to do it, instead of a simple logic of tree in an application, it'd be this much better. Knowing it was hard, but be able to look and say, but it's so much better.
Peter Guagenti [00:27:02]: It's worth trying. It's absolutely worth trying. And then just making it happen. I'll tell you, when I was at Acquia, we created this product called Acquialift, which was a personalization and targeting engine for media companies, and it used machine learning, it was a multi arm bandit algorithm to do it. At first when I proposed this product, I meant nothing but resistance internally, nothing but CEO shut it down. Said, I don't see the value, I don't get it. It couldn't see it. He also and then the engineers were like, well, thats really hard, and how would collect all that data to feed the algorithm and what wed do with that? So there were a handful of us who really believed in it, like really, really believed in it.
Peter Guagenti [00:27:41]: It was one of my engineering managers, myself, the guy who ran my sales engineering team, and we set this thing called gardening days. So every three sprints we would have a dead sprint in between. So the fourth sprint was you could do whatever you wanted as being a big open source company. Most of us contributed back to open source. When that happened, we used the fourth sprints to build the product, but we just believed it. Two sprints. And we had a functioning mvp, fully functional, and came to the company and said, I know you said no, but we believed in enough. We built it and here it is, and it works.
Peter Guagenti [00:28:17]: And it became like two years later, it was a third of the level of the company. And it's a great example of like, if you really believe in it, then pardon my french, but put your ass on the line and just do it. Just go and make it happen. We get to polish rough edges. And there were some things we changed about it, but that product ended up evolving and continuing to grow and is now a core part of that company's business.
Demetrios [00:28:40]: Exactly. And you get to see how putting something into production so quickly gives you that feedback that you were looking for. And now people can't talk about why they can't do it, because it's this thing that's in the air and it's not scoped properly, or it is something that is intangible. Right. When you get something that's there, you throw it down. It's tangible. Look here, we're getting feedback from it. It's a whole different conversation.
Peter Guagenti [00:29:08]: Exactly, exactly. You can take it to your big. For us, it was our biggest customers. I can go to it instead of saying, I have an idea for a thing. NBC was one of our biggest customers at the time, who was really great at giving feedback and really was like a good partner who wanted things to work, and he would pull no punches on his feedback at all. And so he was the first person we took it to where as soon as it was functioning, we're like, oh, by the way, we mocked it up on your site. We're using your data, all this other stuff, and he's like, this is amazing. These are the ten things that I don't like.
Peter Guagenti [00:29:39]: And we just iterated it. Ideas are silver, code is gold. Just get it in production, assume it'll change, and you'd be amazed at what you can accomplish.
Demetrios [00:29:54]: Yeah. And that iteration speed is what makes startups so dangerous, because you can't do that type of thing. There's a million reasons why you can't do that when you're at a big enterprise. Right?
Peter Guagenti [00:30:08]: Absolutely.
Demetrios [00:30:08]: And all that resistance that you faced, maybe when you're at a startup, you face the resistance from a few people on the team. But just the fact that there are not that many people at the company, you can't face that much resistance. Whereas if you're at an enterprise, you're having to jump through so many hoops and jump through hoops of people's jobs, it's only their job to, like, kill your dreams.
Peter Guagenti [00:30:32]: Yeah, it's true. It's true. And it's politics, because I think enough. It's, it's politics that's the problem. Right? Because you see it in big companies. Yes. I worked, my biggest customer when I was an agency exec in the two thousands was Microsoft. And this is the bomber era.
Peter Guagenti [00:30:50]: This is the Kevin Turner era. This is when Satya was just running the online services group, which owned Bing and a couple other things. And it was remarkable at how good they were at saying no to everything and telling you why everything was bad. And it was politics. It was literally just like, I don't want you to win, because then it may diminish my wings. And it almost killed that company. That attitude almost killed that company. You look at what happened, it required them actually having a near death experience and having Google overtake them in a bunch of categories and having Amazon overtake them in software infrastructure and it infrastructure before they finally woke up and said, we can't do this anymore.
Peter Guagenti [00:31:29]: And all the politics got pushed aside. But I've seen that even happen in small companies. It's very easy for people to give feedback or to make decisions that are not actually about the best interest of the company and the best interest of what you're trying to achieve. And I think that's the most important thing you can achieve as a leader in one of these, is don't let that stuff creep in.
Demetrios [00:31:52]: All right. Real fast, I want to tell you about the sponsor of today's episode, AWS, tranium and inferencia. Are you stuck in the performance cost tradeoff when training and deploying your generative AI applications? Well, good news is, you're not alone. Look no further than AWS's tranium and inferencia, the optimal infrastructure for generative AI. AWS, tranium, and inferencia provide high performance compute infrastructure for large scale training and inference with llms and diffusion models. And here's the kicker. You can save up to 50% on training costs and up to 40% on inference costs. That is 50 with a five and a zero.
Demetrios [00:32:39]: Whoo. That's kind of a big number. Get started today by using your existing code in frameworks such as Pytorch and Tensorflow. That is AWS, tranium, and inferencia. Check it out. And now let's get back into the show.
Peter Guagenti [00:32:54]: When. When people start saying no to things, ask, why? Why are they saying no to it? Is it actually because they are protecting the business, or is it because they're protecting themselves? Right. And that's something I've seen that happen in, you know, 50,000 person companies, but I've also seen it happen in 500 person companies. And so, you know, that'll become a bigger hindrance to your success than anything else. I used to always joke, politics will kill a company faster than a bullet.
Demetrios [00:33:18]: That's so good. So there is another piece that I wanted to get into, which was all around dealing with data teams from the business side of the house. So as you mentioned, there's the software side of the house, and then you've got the go to market side of the house. And I know that you've gone through your career and you've been the stakeholder. That's like what we would say on your, like the non technical stakeholder, although you are technical in your own right, but in these cases, you're the person who needs things from the business side of the house and you're working with machine learning and you're working with the different software teams. Yeah. And I want to hear from this side because I feel like in the 205 podcasts that we've done, you're the first person that we talked to. And it makes me feel like a little bit ashamed that it took us this long to talk to somebody in your position, because it is very valuable, and we hear people beating the drum about it all the time, how important it is to take care of your stakeholders.
Demetrios [00:34:31]: Your stakeholders are your customers. At the end of the day, especially if you're an ML engineer, you got to look after your data scientists. You've got to look after your so many different pieces, like your analysts, your marketing team, whatever it may be, whoever's got their finger in the pie on wanting to see the results of what is happening with the data products that are going out, that is you. Like, you've seen the other side of it. And so now what can you tell us about that?
Peter Guagenti [00:35:04]: Yeah, it's a great question. And I feel like I've lived on both sides of the house, both in building the products for a business, both in my agency days, as well as in product development, then being on the other side as a consumer of these tools and as somebody who has set the requirements and said, listen, this is what I'm trying to achieve. I feel like the biggest mistake that gets made by most teams is they get too deep, too fast, and they start getting into a level of specificity around how something is going to be constructed without really understanding why something is being constructed. You think about the data world, you think about operational analytics and machine learning and these applications of machine learning and AI. It's very easy to work bottoms up. Like, I have an algorithm, I have a certain way of reporting on things, or I have a certain data set or data structure that starts to drive what it is. And I see this all the time in marketing, for example, because marketing is just overwhelmed by tracking data. The amount of things, the touches we track and the interactions we track and the user data we track and all these other things.
Peter Guagenti [00:36:19]: It is a soup of just tons and tons of data. Data is not insight. Insight is not action. Right. And what you're actually trying to get to is an action. Right. I don't care about the raw data. Under all of that interaction, I'm trying to understand how I get more people to buy my product.
Peter Guagenti [00:36:41]: I'm trying to understand how to get focused so I can dominate a category as opposed to just spread like peanut butter across a larger universe. And I feel like what happens is a failure on both ends. I feel like the people asking for these things don't ask the right questions, and the people building the systems don't actually understand what it is these people are trying to accomplish. So my biggest advice to data engineering, data scientists, analysts, people building these things is ask the five whys. There's this interrogation technique around asking why, and then when they get an explanation, asking why that is true or why they feel that way. I think there's a, there's a sort of lost arc for business requirements. We used to put so much emphasis on it pre agile, where we'd really unpack and understand and really document requirements, not just at a surface level, but why agile broke it. Agile is so popcorn.
Peter Guagenti [00:37:41]: It's like I'm just going to move fast, I got to just go build stuff. It ends up sending us down rabbit holes sometimes, or it sends us down forks in the road that are in the opposite direction of where we're actually trying to go. I think data engineering and data science teams can do a better job of just stopping and asking, what is it youre trying to get to as an outcome? What are you trying to achieve with this? With an ideal state? How would the business operate more effectively if I were able to solve some of these things for you? Because what you start to find very quickly is their company with requirements and they're actually asking for the wrong things. Right? Or they're asking for something and they're articulating it really poorly. Right. The other side of it, though, I push on the business teams all the time to also do a better job of leading with those things, but then also ask you a better question is about what is possible and what's available. Right. I just went through this at tab nine, where we have a really great data team that tracks a ton of really interesting data about usage and how are people actually living the product and what they're applying it for and where they're successful.
Peter Guagenti [00:38:55]: And so it's all anonymous, but it's really robust data. We used to tune the product and I just sat down with them instead of coming in directed and saying, well, I'm going to do some product led growth initiatives and I'm looking for X, Y and Z. I didn't start there. I started with, what are you tracking? Why did you start tracking that? What does that tell you? How much data we have around that? Because by me understanding even those foundational elements, it starts to give me better questions to ask and better requirements to put back on them. Right? And there's this sort of funny thing around data and insight when you're not a mathematician, when you're not a statistician, when you're not an engineer, where you don't know what you want until you see it sometimes. So I encourage businesses very often to do things like look over the datasets they have and just play where's Waldo? What do I have here? And what does this tell? That's really interesting. And it starts to inform the business in a way that is really striking. I wrote an article five, six years ago when I was at a company called then called MemsQL, now single store, which is operational analytics and ML and AI datastore.
Peter Guagenti [00:40:13]: I wrote a piece for CEO's and it got published. I can't remember which business publication published it, but it basically was calling them out and saying, if data is the new oil and you as a CEO don't know what data you collect and how and why you're failing, you're absolutely failing as a CEO. Because if we were in the nineties, we'd be talking about supply chain. If we're in the two thousands, we'd be talking about digital channels. Both of those required you to have a level of insight around what was happening in a portion of your business that was critical to its success. Today, that's data. That's 100% data. If you don't know the data sources and what you have and where it resides and how it can be leveraged, you're going to make bad choices.
Peter Guagenti [00:40:54]: And I say that for the CEO. Now imagine if you're the head of marketing or the head of sales, or you're somebody in the head of product and you have all this instrumentation around usage and your user. What do you do with that stuff? So I do think it's a two way street.
Demetrios [00:41:10]: There is one. There's a lot to unpack there. Let's just start with that. I really appreciate all the insights. And speaking of insights, I wrote this one down because I felt like it was a powerful phrase that you said, and it is. Data is not insight. Insight is not action. And so it's like the end goal.
Demetrios [00:41:30]: If we're looking at it like, what's the outcome we're looking for? What's the result we're looking for here? We want the action. The data is not. It's like a means, it's a stepping stone to get us to that action, but we can't get caught up in that data. And so the other piece to this, though, is I almost have like a. How do you juxtapose these two ideas that just earlier we were talking about how you were able to build a feature in two sprints, get it out there, you got people using it, iterating on it. But then you also just said that sometimes we need to slow down and really make sure we're asking the right questions and not go deep into the how.
Peter Guagenti [00:42:15]: Yeah, yeah, it's a tough one. The way I think about it is how much effort does it require for you to pull back the bow before the arrow fires? Right. And I think agile encourages a ready, fire, aim mentality when even if you're moving fast, it needs to be ready, aim, fire. Right. Not because you should have a perfect answer. Don't mishear me. I'm not saying you need to have a perfect answer before you proceed. In fact, quite the opposite.
Peter Guagenti [00:42:48]: Like, it's okay to move forward when you only have a limited view of what's ahead of you. But two things are critical. One is you don't want to waste energy on stuff that's not going to go anywhere. And actually, because there's opportunity cost, it's not just the cost of time, but now you're pulled down a rabbit hole and you're trying to unpack it, and then you end up in situations where people want to justify the work they did before, even though the evidence is saying it needs to be somewhere else because they invested so much time and effort in things, and that stuff is dangerous. Right. We talk in software all the time. We should fail fast. I've never met a single company in Silicon Valley that actually was comfortable failing.
Peter Guagenti [00:43:28]: So how are you going to fail fast and be okay? It's really uncomfortable to say you went down a bad path and it's a dumb idea. It's really uncomfortable to say that. Just admit it. It's really uncomfortable to say it. I think there's a lot of value in just asking the whys enough that everyone feels like they're on the same page and everyone feels like, they understand the problem and maybe not perfectly. You say, well, I understand the problem well enough to do my initial foray. I know it just well enough. And then what I'm going to do, though, is I'm going to assume that this is written in crayon, not in stone, and we're going to use it to continue to unpack the requirements.
Peter Guagenti [00:44:07]: And maybe we throw it out. And that's something that engineering teams are really terrible at. Engineering teams get emotionally invested in their code. It's bits. Delete it. It's okay, you can throw it out if it got you to where you are. There's more than enough value in the information and education it gave you that you don't have to maintain the code. And that's something that, as a product leader, I feel like was probably my biggest success was early days and things saying, no, no, no, this isn't where we're going to land long term.
Peter Guagenti [00:44:42]: This is just to solve this one customer problem today. We're going to re architect this thing from scratch right away. But we know we can get this out fast. We know we can solve close enough of the problem that we're not going to freeze ourselves in place and say, well, I have to wait two years until the final product is done. No, I've got a near term answer, I've got a medium term answer, and I've got a long term answer. You've got to kill your loved ones. I hate to say it, but, like, if you really want to be successful as an entrepreneur, you have to be willing to kill the things you love, right. If there's something better on the other side of it, that a customer is going to be more successful with it and you're going to end up having a better result from it, then don't be afraid to throw away the things that you created.
Demetrios [00:45:24]: That's it. Don't be afraid to delete that code. It's all chat GPT written anyway. Let's be honest.
Peter Guagenti [00:45:32]: It's okay.
Demetrios [00:45:34]: Yeah. Incredible. Now, there is something else that I think is important to call out, too, when it comes to the interrogation of the data and almost like the interrogation of what you have, I've noticed that with myself when we are doing things and I can sync with people on what we have, it gives me strokes of inspiration on what we could do. So it's like being able just to understand, okay, there's this, there's this, there's this, and then it's like you're able to put two and two together and say, you know what? If I could know that, it would be very exciting. And I think about it a lot with like, I'll try and give a concrete example here. For example, if you've got your cloud platform that you're trying to get people to use, and you can get information on who is using the cloud platform, but you want to know what the journey is to activation. You want to see like a, what's the biggest metric that shows us that if people do these things, then we're able to say, like, you know, 80, 90% of the time they'll go on to becoming a paying customer. So we need to optimize for that person within the first 30 days to do these things.
Demetrios [00:47:03]: Yeah. How can we just give them all the information that they need? How can we make big pop ups on the product so that it points them to doing that? And all of that is huge. But you don't know what, like, if you're starting from scratch, you don't know what the journey is. You have to figure out, do we have that data? How can we find that data? How can we know what those metrics are that we should be tracking? All of that is like, sometimes I think when I look at it, I'll get overloaded because there's so many potential things and then it's choosing those one things and saying, okay, we're going to make a bet that this is the metric we need to be looking at. So I guess there's not really a question in there, but I feel like you may have some wisdom and you've done that before.
Peter Guagenti [00:47:50]: Yeah, well, I have. And actually I think you just threw out a really critical insight, which is you don't know what you don't know. Right. But if you approach it with the right mindset of, I'm going to start off with a potential answer and I'm going to be observant and really pay attention to what it's telling me. I will start to understand what I don't know that actually has value. And so I'll give you an example. So the most common modeling use case that I do in marketing. So we talk about marketing as the right message at the right time to the right audience.
Peter Guagenti [00:48:23]: But the irony is it's inverted in value. We spend a lot of time thinking about, create a very right message, but actually, like 85% to 95% of the value is targeting the right person. If you just target the right person, even with a mediocre message, you tend to get more sales, you tend to get more product growth. Right time is the next five to 10% of that. The last few percentage points is the right message. We always spent our time on the modeling side on ideal customer profile. How do I identify the right customer for me? Because you don't want 2% of the market. You want 100% of the customers that you're an ideal fit for.
Peter Guagenti [00:49:03]: You want to dominate something. And so I've worked in a bunch of, you know, I built these models myself from scratch when I was a management consultant for, for companies. I've used tools to do this. My wife works at a company called Mat Kudu that does this for sales and marketing professionals around, like enterprise sales. And what we've always discovered is the same thing. So when you first build one of these predictive models, like I'm trying to figure out who my ideal customer is, and the sales and marketing ask is simple. I want to put 100% of my time at a customer base. That is the highest likelihood to convert.
Peter Guagenti [00:49:35]: I don't want to spend my time on 100 people to convert five. I want to spend my time on the five. That's the ideal state. But it's a spectrum. When you first build these models, the best thing to do is collect as many characteristics about the customers you've been selling to as is humanly possible, because you'll know what is, what's going to generate Lyft. You don't know which ones are actually the indicators. I remember when I first started doing this enterprise software, I would just collect everything. Firmographics, hiring data, technographic profile, anything about their product, set the verticals they sell into any piece of data I could collect.
Peter Guagenti [00:50:15]: A pattern started emerging in all of this. It's been consistent for all the software companies I worked at in emerging technology that the technographics are the things that always are the most important. So literally, what else do they have in their stack that was always the most important. So I'll give you an example. This at Cockroach we landed on. CockroachDB is a high performance, high scale, unkillable system of record. So it's a system of record database. Traditional OLTP, the dead giveaway of a customer that was an ideal fit for us.
Peter Guagenti [00:50:48]: Had Oracle and Mongo. They managed their own data center and they ran kubernetes. Those four things would feel like they're opposites to each other, right? But they actually worked because this was somebody who had high scale, which is why they had their own data center. They had legacy applications, which they didn't want to mess up, but they were really forward thinking. They were modernizing, their business kept evolving, they kept changing, which is why you'd have Oracle and Mongolia, because you had unkillable system of record in first gen and this unkillable but not agreed system of record that you try to use on the other end. And so what was interesting about that is when I first started doing these things, when I first started seeing technographic data popping up as high lift, what you realize very quickly is how much you don't know. Like the very first time I built it, we didn't track who was running kubernetes, we didn't track what cloud providers they were on. We were tracking whether they were hiring for data center roles.
Peter Guagenti [00:51:47]: We didn't have any of these things, but once a couple of them started popping, we were like, wow, that's really interesting. If that's generating Lyft, I wonder if the wonder if then gave us requirements instead of people, let's go get this data, let's go see if it helps. Then some of it helped and some of it didn't. It was great. I think that's true. Timing is true for that. Messaging is true for that. I think that's actually true for even other things outside of marketing.
Peter Guagenti [00:52:14]: When you think about the AI stuff we're building at tab million, we use rag in context. So when you're writing code, you're working in chat, you're asking for a recommendation, I need a function that does this. We started actually giving context awareness to things like documentation, to things like your requirements, docs, not just code. We started giving exposure to other things and we discovered was, oh, interesting. Context awareness around things like your geo instance is actually more useful than context awareness around code. You don't know what you don't know until you start experimenting. And then you have to be unafraid to say, okay, I'm going to add data, I'm going to add these things, I'm going to try it, I'm going to keep tuning. But the one thing that ever changes is what are you trying to achieve? That ideal customer profile example I gave you, when I roll it out of Nginx, we were getting a shocking number of inbound leads.
Peter Guagenti [00:53:07]: Shockings. Nginx, as of now, I think 70 or 80% in the year runs on. So we had this massive pool, we just have to keep trimming. And my CEO came to me one day, we were getting it focused. Down he goes, I want to cut the number of leads that the sales team touches in half without changing the outcome. That was his goal to me. And we did it in two segments, and we kept getting smaller and smaller where their conversion rates just kept doubling every time we'd make changes.
Demetrios [00:53:32]: Wow. Okay. It's almost. Yeah, you're just following breadcrumbs in the beginning, trying to see what are the biggest drivers and what is going to give us the idea of who our ICP is. And then once you can zone in on one, it's like, huh, I see this, whatever it may be, Kubernetes users and Kubernetes users that are running their own data center. That means they have scale, that means that they're doing that. And then, oh, add a little sprinkle of oracle on there with MongoDB, we're golden. We have found our fit.
Demetrios [00:54:07]: But you didn't get that on the first try. I really like that you're honest about saying it took you a while to dig through all of this data, and maybe you had one little nugget of information and you probably found, okay, people that are using kubernetes like us. And I think for me, as I was listening to that, I probably would have stopped there and been like, all right, let's go get all the Kubernetes users. But you're saying no slice down, segment down as much as possible so that you can find that 100% of the market that you can dominate. Yeah.
Peter Guagenti [00:54:42]: You know, so there's another famous example of this, which was Netflix's recommendation engine. And that's something that everybody, I think, can really relate to. You know, Netflix's recommendation is really good. It's really, really good. When it first started, it was 100% based on likes. So it was like, did you thumbs up or thumbs down? Right. And what they discovered was. And I have to find the report, but I'm sure your viewers can search for it.
Peter Guagenti [00:55:06]: They discovered, actually, that whether or not you played something all the way through, and if you played it multiple times, was actually more valuable data than what you explicitly said. So they discovered your behavior was so much more useful than your stated view of something. And that's another one. It's not.
Demetrios [00:55:24]: Yeah, actually, I was thinking about that when you were saying it. It was like features, right? Like, for recommendation systems. This is exactly what you're. You can use that as a parallel here. What you're talking about for, in this marketing sense of segmenting down and being able to slice down for the ICP, it's the same thing for, like, features if you're trying to build a recommendation engine.
Peter Guagenti [00:55:49]: Yeah, yeah.
Demetrios [00:55:51]: So there is another place that I wanted to take us. It's a little bit of a change of gears, but bear with me because you're building, you kind of drop some hints, but you're building a coding assistant that has access to so much more than just your code. Yeah. And I know that I can only imagine how happy people are that an LLM can read their epics and their stories and it can give them context. And maybe one day you can have some agents that will go and just do the epics and stories for you. But I don't know. Are you there yet?
Peter Guagenti [00:56:31]: We're there, actually, we're not released, but yes, we have that. We. Never mind. All that stuff already built and will be rolling out over the coming months. I'll back up and tell a little bit about why this company and what they were going after and then why I joined. I've only been in the company now a few months. So Tamdyn is the originator of the AI coding assisted category. So the company actually released its first product five years ago now.
Peter Guagenti [00:56:57]: And it was basically in parallel with the growth of llms. Right. So as llms started to really demonstrate their power, the company founders had been focusing on developer productivity and automating as much of the developer software development lifecycle as possible through other data intensive means. But LLM had the most promise. And it's obvious, right, if you think about a large language model, what does it need? It needs structure in the language, it needs repeatability in the language. It needs clear rules. The english language is a mess, which is why it's taken so long for generative AI to work within the english language. But programming languages are very, very rules driven.
Peter Guagenti [00:57:39]: It works really well. So the company built originally an LLM to do code completions in the IDE. So as you type, basically we'll type ahead of you. Really powerful, actually, really, really powerful. Because when you're trying to write a function and you're trying to try to add data in fields and everything, it knows where you're going based on the context of what you have open, where it's going, though. And the reason why I joined the company is AI. My belief is AI's going to change every job. Right.
Peter Guagenti [00:58:10]: Every single thing we do today will look a little bit different five years from today. It's the same thing. We went through a digital transformation. It's not all scary. I think some of it feels a little scary, but my view of it is it's not going to be, it's not going to be a job replacement. I think about a lot of these things as an Iron man suit for the mind. Like they're really about accelerating you and accelerating your skills and your capabilities, and automating the way the mundane. That's the truth.
Peter Guagenti [00:58:35]: That's what we're actually seeing. These AI tools for software development have been around, like I said, three to five years now for the majority of the category. So we've seen this pattern. And what I really liked about what Tab million was doing is they were looking across the entire SDLC. It said, if I had an AI agent that lived in the middle of everything, that lived in the IDD with you, but also lived in jira and confluence, and also lived in Datadog and lived in your other APM tools, and then could read your logs and could do all those other things, how smart would that AI agent be? So when you went to write a test, instead of saying, I'm going to start writing a test, it would say, we're going to write a comment that says I need a test that does this thing. And I wanted to be able to assess for these factors and I want to be able to know it succeed or fail based on these outcomes. It can actually do it because it has all the systems available to it. It's not just, I'm going to write a dumb test, I actually know.
Peter Guagenti [00:59:29]: Oh, you mean you want to actually track the outcome from datadog so it will pull the data that comes back and tell you what actually happened. Now, some of this stuff is still in progress, but that's the vision. The vision is you should have an AI agent who knows how to do testing with you. You should have an AI agent that knows how to write good requirements with you. You should have an AI agent that does things like deployments and actually sits alongside you as a DevOps professional exposure and tell you if it's successfully deployed based on real world data. All of those things, when it works really well, it's your patterns of behavior, it's the things you would normally do, but instead of you having to go to five different systems to do it, you've got this partner that works with you and actually doing those things. The vision for the company that we have is in order for that to happen, in order for that to be done. Well, there are a few requirements.
Peter Guagenti [01:00:21]: The first one is we believe privacy matters. Unlike a lot of the public tools like Copilot and others, we don't collect any of your data. We will not share it, we will not change the model based on your data. So we are completely private you want to deploy completely air gapped. We do that. You want to go and deploy in a private SaaS. We do zero data retention. We're really focusing on privacy because that's the only way we earn the trust to talk to all of those systems is if we are fully private.
Peter Guagenti [01:00:54]: Fully private. We also really focus on copyright. Our models, unlike the public models, have not been trained on any non licensable data. A lot of these larger chat, GPT and others, there's all this discussion or copyright because they've trained on stuff they don't have the right to. And you can debate whether or not do they have rights? Do they have not? A lot of that stuff will get settled at a court of law. But from our perspective, it was not even a law issue, it was an ethical issue. If you said that your code is GPL and anything that gets generated needs to stay GPL, we're not going to train on GPL, we just won't do it because there are other ways of getting to that answer. We see it.
Peter Guagenti [01:01:36]: We see maybe a slightly lower performance for us versus others, but we actually have switchable models. So our platform can run across multiple models and we've tested it. So if I go to a CIO and say, well, I can give you seven out of ten answers perfectly with tab nine, or I can, using the OpenAI models, get you eight and a half out of ten perfectly, but one of them is legally safe and the other one is legally questionable, which one do you want? They always set a seven out of ten because the additional benefits aren't worth the risk. Right now, I'm hoping a lot of this stuff gets resolved. Our view of personalization is we can get to that eight, nine out of 1010 out of ten by trading on our customers data, by trading on other things. We can license, which we own, licensing from third parties, but we think it's our mindset to have with this stuff, because AI is going to penetrate every function in every company, and you have to do it in a way that really respects the requirements of the total business, not just the task at hand and what that is. And we believe that's really critical.
Demetrios [01:02:41]: One thing I find fascinating about the ability to ingest from all these different places like you were talking about, maybe it's your datadog instance, maybe it's your CI CD pipeline, you've got visibility into that. Maybe it's the Jira, all of that and a little bit of a sidebar. I 100% understand why privacy is so important. I don't want you just sending out data to chat GPT about my roadmap. And all of a sudden, GPT five now has my nine months ahead roadmap. Well, I guess by the time GPT five comes out, it'll be obsolete by then. But I think you more than anybody understand why that is. That is not cool.
Demetrios [01:03:28]: But coming back to the .1 thing I find fascinating about this is that when it comes to code, evaluating if it runs or if it doesn't run is so much easier than evaluating. Like marketing. Totally copy that. Is that good or is that not? Is that going to get somebody to buy better than what I could have created? I don't really know, but I guess it gave me a bunch of cool ideas and I can riff off with those ideas.
Peter Guagenti [01:03:55]: Yeah.
Demetrios [01:03:55]: And when it comes to code, you know, it's very black and white.
Peter Guagenti [01:04:00]: Yeah, yeah, very much so. Very much so. And even where it's not black and white, like I had this conversation with a, with a partner, another big tech company that we're starting to work with, and they said, well, there might be three answers that work, but one of them is preferred, one of them is better. That's where context actually really helps. We can actually set some guardrails and say, when a developer asks a question about x, the answer is always y. Because, for example, I need a function that accomplishes this and does it in this way. We always use this API for that, we always use this system for that, and we always secure it in this way. As a company, you can actually set those parameters.
Peter Guagenti [01:04:45]: That's stuff that we're rolling out later this year. We call it expert coaching or expert guidance, where you can set some parameters for those things. And that is possible. Now that's more of the gray area. But this is where the machines with the right context get smarter and smarter. This is actually something that users don't understand. I can't tell you how many times I see users complaining about how the system is broken. This is true what we get for feedback in tab nine, and we see it with our competitors, but I see it with people just prompting chat, GPT, and getting bad answers.
Peter Guagenti [01:05:17]: And when you look at it, you say, okay, the issue wasn't the system. You didn't give it enough context. It's like going up to a stranger on the street and saying, help me find a car. Okay, wait a minute. Like, who are you? What do you need it for? Who are you carrying? How often are you driving? Do you care about performance? Do you care about fuel economy? Do you care about size? Are you a breeding? Are you a muscle car guide? Like, there's a million questions you have to answer in order to do that. And we feel like our learning around how to use AI is still stuck in this era of we expect an omniscient bot that's going to give us a perfect answer with minimal input. They're not there yet, so we're going to close the gap two ways, right? We're going to close it by being better consumers of these tools and giving them more to work with. But actually, I think this is where companies are really going to win over the LLM providers, is we can shape that.
Peter Guagenti [01:06:12]: So, for example, when someone starts asking for a function, we look at the workspace and we look at what everything else they're doing, say, oh, you're writing that function. You always write it this way, and that's the right answer. So really we're going to give you that. So it's, instead of having like a stranger on the street, this is somebody who knows you, who's worked at your company, who's been part of your team for a while, so they have a different context. So I think that's what's really exciting about AI, because there are three components to all these things. There's the models themselves, there's the data they train about, and then there's the user experience. And the user experience includes prompt engineering, the shaping of all this stuff, what goes in, what comes out, all those other things. I think the models are going to get democratized very quickly.
Peter Guagenti [01:06:51]: My belief, and I've seen this in every other part of technology in the last 30 years. I think the models are all going to be equivalent within five years. There's going to be no differentiation because they're all open source white papers. We're all building the same stuff. The data sets, I think, are going to become more curious. We're going to all license the appropriate stuff. We'll have access to the right things. People will get better about protecting their own data and using their own data to train their models.
Peter Guagenti [01:07:14]: So everyone gets access to everything. I think really, the space for entrepreneurs and for people building these products to win is still the uxed. It's going to be in crafting the right experience so that you bring to it what is appropriate for you as a user to bring to it. We don't make you jump through oops, and instead we provide that additional context and we gather all that stuff. And the AI agents are great for this. I'll ask you questions, you ask me a question and ask you a couple questions before I give you an answer. That's going to be really exciting because that's where the things will really start coming together.
Demetrios [01:07:47]: It's so funny you mentioned that because I was going to ask you, are you baking the philosophy that we were talking about earlier on, making sure you're asking those five whys into how you're dealing with the prevailing amidst AI?
Peter Guagenti [01:08:00]: Yeah.
Demetrios [01:08:01]: So, yeah, I figured as much. And earlier today I was reading a blog post about the way that you evaluate coding AI, and I was remembering a conversation that I had probably a half year ago, maybe eight months ago now, on how one of the strong evaluation metrics that people will use, especially when it comes to coding, but just in general, is if you accept the output. And this article that I was reading today was talking about how when they did a study, and I have to figure out what it is and cite the paper and stuff, but there was a study done on the amount of code that stayed in the code base from suggested chat GPT. Yeah, two weeks or four weeks after it was accepted. And what they've been seeing is there is a lot of tech debt that gets formed by just accepting copilot's suggestions. And then later on you have to go back and realize, oh man, that was a bitch. You wrote this code. How did anybody let this get through?
Peter Guagenti [01:09:16]: You know, so I know exactly the paper you're talking about because I just posted it myself on social media. It was a great piece. It was a great piece of research around code called and my response to it, and I'll share with you exactly what I said when I posted it, which was, it reminds you that these are not a replacement for a developer. Once again, I use my commodore. They're an ironman suit for the mind. If you are a terrible developer, you're going to insert terrible code. Whether you wrote it yourself or whether an assistant coached you on it, you still have to actually make a good choice.
Demetrios [01:09:51]: Yeah. Whether it's from stack overflow.
Peter Guagenti [01:09:53]: Yeah, I mean, this happens all the time. By the way, I can't tell you how many people copy pasta out of stack overflow and injected. So this isn't new. The AI bots are just making it faster to do it. That's all they're really doing. And so there's a couple of answers to this. First and foremost is as engineering managers, you have to be diligent. Who is on your team and what are they accepting and what are they putting in? It doesn't replace code review.
Peter Guagenti [01:10:16]: The AI bot can help you with code review, I suppose specifically you have parameters, you give it. But if you have a bunch of very, very poor developers, all you're doing by getting them these tools is making them generate poor code faster. Your answer here is to get rid of the poor developers or train. It's one or the other. You either need to make them more effective or need to get them out. The AI coding assistants, by the way, do on average save 20% total developer productivity, 20% total across everything. When you talk about writing code, writing tests, writing comments, evaluating code, explaining code, all these other things, all the studies, I've got a white paper on this that we created at tab nine where I didn't do any research myself. I just went and said, what did McKinsey see? What did IBM see, what did Cambridge see? There's all these studies that have been done and what you see very interesting is the data.
Peter Guagenti [01:11:09]: All is very similar and very consistent and it's about 20% setting up. If you have 20% productivity savings on your mid level software developers, that gives you more time to coach the junior folks, or it means you can fire 20% of your junior staff, one or the other and get back to quality. Now the interesting thing is, I do think AI has an answer to this above that, which is when the chat suggestions come out, like when we actually suggest something, we do track whether it was accepted or not, because that helps us shape it. You can overweight who is valued in the response. So if you know that this is a senior developer, this is a coach, then their weight is given much higher priority over others. This is where that coaching context I think comes in really helpful because we can come in and say, actually this is what good code looks like when you're recommending a function. If you have three options, generally from what's available in the wild versus this is what an expert developer on the team built bias towards that. That's where things start to.
Peter Guagenti [01:12:20]: This is the UX stuff I'm talking about. This is where things start to get really, really interesting because this is not a difference in the model, this is a difference in what we do with the prompt, the context, and then what we recommend back. And you can get very specific if you really want to in these things.
Demetrios [01:12:36]: So then it makes complete sense that you would have these senior developers be weighted much higher. And it makes me think about how, yeah, like of course you would. You want that to be the golden evaluation set. You want to be taken abat to the lab and training with what they're accepting and what they're saying is, okay, so, yeah, look, I'll leave you with.
Peter Guagenti [01:13:04]: One thing on these AI tools, because I don't think it's just for us. I think it's going to be true in a lot of these other categories. I think there was this, there's this universe of tools that are trying to be all things to all people, right? And Gideon Cobalt's a great example. They don't want to be focused on a certain type of developer. They want to get the lowest commons denominator, as many as possible, because that's best for Microsoft's cloud business. If they do that, they'll get a lot more people burning compute on Azure. Whereas someone like us who's focused on enterprise development teams, like high performing enterprise developer teams, they're going to want things that are more bespoke. They're going to want things that are a little bit more focused on them and how they work and the things that matter.
Peter Guagenti [01:13:45]: So that's why we make personalization such a critical part of what we do, because we don't care about being all things to all people. I want to go and make every engineering team more successful as a unit. I want to make that entire engineering team more successful, not individual developers modestly more successful. Your example or our code quality is a great example. I do not want to accept just generating more code. I want to know that the code that we are generating as an engineering organization is more successful. That is absolutely part of the parameters of these things. I think that's where we're going to start seeing the difference in AI in general.
Peter Guagenti [01:14:23]: You can go and just tell Dolly to generate you an image. Where you go to adobe that actually has better curated content, knows what you're trying to do, knows stylistically what you want, is going to ultimately focus on generating something that isn't just, oh, look, I made an image, it's, oh, this is an ideal image for my use case. I think that's where this expertise and the UX side is really going to come to the fore in the next five to ten years is companies like ours are going to say we care about the outcome, we care about the output, right? And it's at the right level of quality.
Demetrios [01:14:54]: That user experience is a great thing to flag, and I hadn't thought about it until you said it, but it makes me think about how that is the differentiating factor. At the end of the day, you have to have that strong user experience when everyone has access to the model, unless you're just adding a feature into your already high performing product that isn't leading with AI. If you are leading with AI, though, you got to make sure that the user experience is the strongest because that is the differentiator. That's your moat right there.
Peter Guagenti [01:15:33]: Yeah, agreed.
Demetrios [01:15:34]: I want to end with one last thing, which is you've had some time in marketing positions and you've specifically marketed to devs. You talked about how you really were looking for these three different pieces of information. But I want to dig a little bit further and ask if you found anything specific when it comes to marketing to developers. Have you seen any insights that you can share?
Peter Guagenti [01:16:08]: Look, I think I have, yes, I have marketed to developers, but I've sold enterprise software, right. So I think there's an important thing to note with large scale developer tools, which is they're not bought by developers, they're bought by their boss, or they're bought by their boss's boss. And what you learn very quickly is selling complex technical products. You don't sell to one person, you sell to a sphere of influence. You sell to a group of people to make the decision together, and they all have different goals. And that's probably the biggest breakthrough I made early in my career. And something I advocate for anytime I talk to anyone going to market in enterprise software is don't think you have one customer, you have a champion who really wants this product in because they have a problem they're trying to solve. You have a decision maker who's going to say yay or nay.
Peter Guagenti [01:17:00]: You're going to have somebody who controls the budget, which might be different than the decision maker. You think about procurement in a large company and then you're going to have users. And those users, they can either push you in the business or they can keep you out of a business if you don't win their hearts and minds selling to developers. A lot of my career has been, can I build a groundswell of trust and belief among my end user? Do they believe in this? Do they feel like this is something they want to have in their lives every day? Developers almost never have budget with that. Can you activate them to become champions for you? Can you get them to push you in an organization? And then can you go to the engineering manager? Can you go to the CIO or the CTO? Can you go to the architect or the principal software engineer? Those people usually are decision makers. They are champions or decision makers and help them understand the value you add. And so even when I coach, my salespeople like, look, know who you're talking to and know what they care about. Like if you talk to a developer coming out of out of the AI place, for example, developers don't care if that code is licensable or not.
Peter Guagenti [01:18:10]: They don't care like they don't care if the code is being pushed out to open an AI. They don't care about any of that stuff. Most developers don't last more than two to three years in a job. So they're not thinking long term, they're not thinking about these things. They're just thinking, look, I've got ten jira tickets I have to work through in the next five days and I really only have time to get seven of them done. They're very focused on like, you gave me a job, I'm trying to get through this job as fast as possible, right? So when we talk to developers, we're like, look, I'm going to help you get through the job as fast as possible. Now I am going to do it in such a way that doesn't get you scolded in the future. That is part of it, because the code quality is bad, because you issuing code that it wasn't licensable, those sorts of things.
Peter Guagenti [01:18:53]: But when you talk to the CIO, they don't care about developer satisfaction. I hate to say that, but they don't. Those are machine parts to them. If you have 10,000 developers, they're like, yeah, I'll just hire another thousand. But they do care about productivity and efficiency. We do remind them that you don't want to churn developers because that's expensive, you know, so you got to really know your audience and make sure that your value propositions resonate with each of them. Like, are you really solving something that they all care about? Because if you're solving something that only one of them cares about and the other's a resistance to, you're never going to build a business.
Demetrios [01:19:29]: You just hold shoot me straight. Peter, you good at golf?
Peter Guagenti [01:19:35]: No, I don't golf. I was a skateboarder. Do you think I golf?
Demetrios [01:19:40]: I thought I was about to say you sold out on us and now you're golfing, selling to these CIO's.
Peter Guagenti [01:19:48]: Actually, you know, I thank God that most of the guys in charge in software decision making, in the United States at least are jet x ers who grew up the same way I did. So I actually, my biggest hobby, I race cars as a hobby, and I probably know more executives in the software through racing than I know through work. So, you know, it's, I feel like adrenaline. Sports are the new golf for my generation, at least. So I'd be more likely to find, you know, find.
Demetrios [01:20:19]: There we go.
Peter Guagenti [01:20:20]: Buddies on a kite board or on a snowboard or in a racetrack than on a golf course, which I'm grateful for.
Demetrios [01:20:28]: So there is one thing I want to leave us with, which is I know you are hiring right now. You've got an incredible team of developers that are working in. You've got an engineering team remotely all over the world, I think. And then you've got a home base. Also. You've got a team in Israel. You're based in California, right?
Peter Guagenti [01:20:48]: Correct.
Demetrios [01:20:49]: So if anybody out there is looking to join the rocket ship and let us know, hit up Peter. Tell him you heard him on the podcast. It's been great talking to you, man. Like, there's so much I've been. I've been scribbling notes a ton, which I normally don't do because I try and give my full focus and attention to the guests. But here, there was a few that I was like, well, I have to remember that so that I can use it later and incorporate it into my life and how I do things. Thank you for coming on here.
Peter Guagenti [01:21:20]: Always want to be on the phone.