Why is MLOps Hard in an Enterprise?
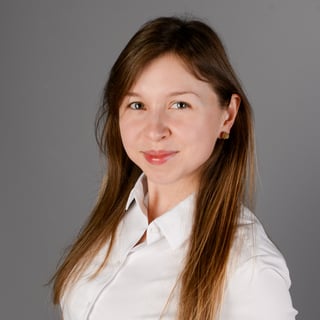
Maria is an MLOps Tech Lead at Ahold Delhaize, bridging the gap between data scientists infra and IT teams at different brands and focusing on standardization of ML model deployments across all the brands of Ahold Delhaize.
Maria believes that a model only starts living when it is in production. For this reason, last seven years, she focused on MLOps. Together with colleague Basak, started Marvelous MLOps to share MLOps knowledge with other ML professionals.
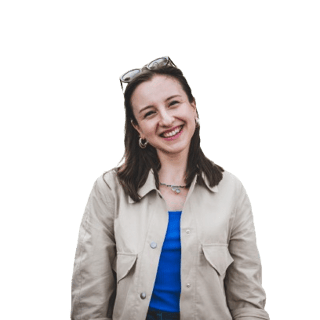
Senior Machine Learning Engineer with 5+ years of experience across diverse industries including banking, retail, and travel.
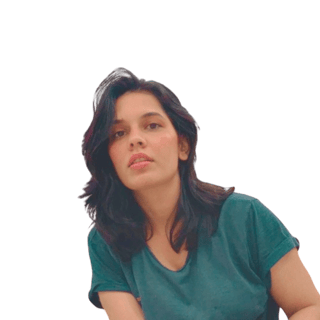
Abi is a machine learning engineer and an independent consultant with over 7 years of experience in the industry using ML research and adapting it to solve real-world engineering challenges for businesses for a wide range of companies ranging from e-commerce, insurance, education and media & entertainment where she is responsible for machine learning infrastructure design and model development, integration and deployment at scale for data analysis, computer vision, audio-speech synthesis as well as natural language processing. She is also currently writing and working in autonomous agents and evaluation frameworks for large language models as a researcher at Bolkay.
Prior to consulting, Abi was a visiting research scholar at UCLA working at the Cognitive Sciences Lab with Dr. Judea Pearl on developing intelligent agents and has authored research papers in AutoML and Reinforcement Learning (later accepted for poster presentation at AAAI 2020) and invited reviewer, area-chair and co-chair on multiple conferences including AABI 2023, PyData NYC ‘22, ACL ‘21, NeurIPS ‘18, PyData LA ‘18.
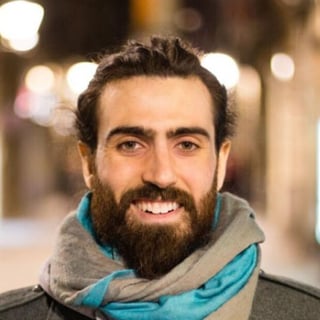
At the moment Demetrios is immersing himself in Machine Learning by interviewing experts from around the world in the weekly MLOps.community meetups. Demetrios is constantly learning and engaging in new activities to get uncomfortable and learn from his mistakes. He tries to bring creativity into every aspect of his life, whether that be analyzing the best paths forward, overcoming obstacles, or building lego houses with his daughter.
MLOps is particularly challenging to implement in enterprise organizations due to the complexity of the data ecosystem, the need for collaboration across multiple teams, and the lack of standardization in ML tooling and infrastructure. In addition to these challenges, at Ahold Delhaize, there is a requirement for the reusability of models as our brands seek to have similar data science products, such as personalized offers, demand forecasts, and cross-sell.
So I'm Maria. I'm a lead machine learning engineer at Akoto. I'm very critical about my coffee. It has to be a latte in the morning. It has to be exactly agent half grams. Grinded coffee that should be not older than two weeks and it has to run exactly for 25 seconds and then it should be a microphone.
So it's very hard to beat it and I almost never like coffee anywhere else. Hi, my name's Basak. I work as machine learning engineer at a Hal Del Highs. I like my coffee, Turkish, actually. I like, uh, I drink Turkish coffee every morning. It is strong enough to wake me up. Hello and welcome everyone to the ML Ops community podcast. I am your host Dimitri Os, and today I'm joined by none other than Abby. What's happening, Abby? Oh, wonderful. Abi. How. A lot of announcements, but basically we have an event coming up June 15th that is happening June 15th and 16th.
We've got the large language models in production, virtual conference, so if anyone wants to learn a little bit more about how to use these large language models, that is going to be two days worth of nonstop, like six hours of streaming. I think we've got three different tracks. We've got workshops, panel discussions, fireside chats, and of course, Talks from some of the greatest in the field.
I just confirmed the Microsoft Deep Speed team is gonna be there. We've also got Matt Tay from Databricks, given a keynote. And Abby, you're gonna be talking there. You're gonna be on a panel, right? Leading the evaluation panel? Yep. But I am pretty excited about recording this introduction today where we had a conversation with Maya and Bashad.
can I just say one thing before you jump into who Maria and Bakka are. They hands down, have the best coffee intro. I think that we have heard they are very particular and I like that they know what they want when it comes to coffee. So who are Maria and Busak? So write down both of them are working, uh, hold.
Uh, which is one of the biggest grocery chains across Europe. And Maria for one, has really interesting background. She worked as a data analyst and then tun to Rice, and currently is now a manager working in ML engineering in Bash. She's currently working as a machine learning engineer at ul. Her background, again is as a data scientist.
And she worked at banks and stuff, so they have such clarity when it comes to like the thinking about lops frameworks and adoption at organizations. I was so surprised that how mature they were when it comes to the machine learning life lifecycle, it is so cool to see this because.
They talked about, and I don't want to give away too much, but when they jumped into how they were able to standardize and make sure that everyone at Holtz was on the same page and make sure that there were some kind of standards that people were abiding by and. Looking at the maturity levels of each one of these groups in their little silos and what was consistent across all of these groups, and what were things that people were asking for.
And on top of that, what was so cool is that they were referencing a few times in this podcast how they had been thinking about writing a blog or that they were, they had this maturity assessment. Questionnaire that they were giving out to their coworkers, and we asked them in the podcast if they would be open to sharing it with the rest of the world.
And it is very exciting to say now that that is in blog form and it is on the Lops community blog. So we'll leave a link , in the description to that in case anyone wants to check out the maturity assessment levels that they put together and how you can correctly. Assess where your machine learning efforts are going, and especially if you're in a big company, this is just like gold.
I really appreciate them giving this to the rest of us. My best part about this conversation was especially when we asked Mario on which tool comes out to be expensive versus cheaper, and she said, it's not really about the tool you use, it's about how you use it. And I think that was one fantastic. At least like a very different point of view and probably the right way to think about M'S maturity and M'S tooling in this space.
We'll, uh, get into this conversation already. Huh? Let's go
Today. I really wanted to get into some of the challenges of. Of doing ML in the enterprise and particularly ML ops. And so Maria and Basak, I'm excited to have you both on here to talk about your experiences and maybe we can start off, Maria, can you kick us off with just how you ended up. Having such a colorful history and know a little bit about this topic at hand.
Yeah, that's a long story. I think I was always passionate about automating things. I started nine and a half years ago as a data analyst. A data science wasn't really a thing, so you could not get hired as a data scientist. But I was building some churn mold. Was it a telecom company in Netherlands, kpm.
And I was writing scripts in R back then, so I didn't know Python, but I knew some R and we scheduled, uh, jobs on the server standing in the room and it could get locked with a key. So it was, it was really funny. We didn't have any servers, I think fancy, but I wanted to do things in automated fashion already.
Then I moved more into data science, uh, building my first APIs. And there was no one who could put things in production for us. So I started doing it myself, just learning how would you do such a thing? Talking to software developers in different department and, uh, getting our first a p I live and we also built something called Model Factory.
Back then it was pretty cool. Basically we were doing the lops already six years ago. Uh, Melos wasn't a thing back then. Model Factory. It sounds like an ML op tool that I have heard of, but I can't remember where something. if you Google it, you can still find our block on Model Factory back then. And I think Mobile Factory is not really about what tools you use, but it's more how to get it working together. So I really love the Melos and I build a version of Model Factory like four times all over again with different tools and also one here at the Jesuses.
So I want to dive into why and how it evolved on these four times like you iterated on it. But basak, I also want to hear your story, . Mine's slightly shorter.
I, first started working as data scientist and uh, I realized, that numerous notebooks were being treated as data science products and many actually, uh, projects were remaining at M PB M V P stage. Uh, because of operationalization problems, I made a decision to become a male engineer cuz it made more sense to fill the gap between data scientists and.
The ops engineers, and that's how, how I actually, uh, started working as a metal engineer. So my previous company was a, a Dutch bank. Um, there I, uh, developed models but also deployed models. And then I, realize the standardization is actually key when it comes to big enterprise. there we also try to, um, create common solutions for, uh, operationalization parts.
and now I'm at Ahol Del HAIs. Uh, here we actually, uh, managed to standardize many things. I think we'll dip, dive into that, um, later in the podcast. I find it very fun to actually, get things in production because, uh, notebooks, uh, make no value unless you productionize them.
It's one of those podcasts that I'm realizing I should have blocked off two or three hours of our time because just in these opening words, I see that there are so many things that I want to talk about. Not only what we have that we. Mentioned the themes that we were gonna talk about, but now thinking about standardization or thinking about building a model registry and the evolution of that and how those two things go together.
So maybe Maria, like I imagine you also think strongly of standardization, cuz I saw you Bob in your head and when. You were creating these tools, these internal tools, and you saw the evolution of them, how did standardization play a role, if at all, Yeah, so basically Model Factory or my ML ops framework, it's all about creating the golden path of how to do things because data scientists, most of the models, they're just the same.
So they are doing the same thing all over again when it comes to bringing that to production. So, They have developed a script, they have to run it somewhere, and somewhere is usually just one tool that you have to pick. So for example, now at, we use Databricks a lot and we, uh, run jobs on Databricks.
Before at KPN we had a server called As that was the product of Teradata, but KPN bought it and we had to use it because well didn't have any other choice back then. We also migrated that to Kubernetes. So we were running jobs on Kubernetes and um, also we were trying to do the same thing on AWS using SageMaker.
So it's, there are many tools you could use to do the job. It's just, uh, picking the ones that you have in place already. And in large organizations you already have a lot. So basically you need to have a version control and that there is already a tool of choice. So we have GitHub, for example, JE and for C I C D we use here, um, GitHub actions at KPN used Jenkins back then.
So it's really, there are so many tools and you just have to see what you have and combine them together in a way that is easy to use for data scientists so they don't have to think about it. So the way, how I see that, They can use the reusable workflows that are developed, um, by a central team. So for example, our team and, um, they can just have something up and running in five minutes.
They don't have to think about service, user service principles. So all of those things, um, it should be minimal for them to start working, start bringing demos to production. That's how I see it. what is also important, uh, as Maria mentioned, I think it needs to be easy when it comes to a solution design for, uh, AI products, data science products.
It's not about combining 15 tool together and then have a very large um, End-to-end solution. It's actually more about you choosing what you need, cuz you already need certain functionalities. You don't need, three tools that has the same functionality. Yeah. So we, um, what we focused here when you, when it comes to standard solution is choosing the key elements and combining them and having a very simple end-to-end solution.
And we provided that as a golden path. Also, what does like standard task for you look like? Uh, the reason I ask this is because, again, depending on the task, you would choose very different models. And depending on the models, the pipelines could sometimes differ. Yeah, I think it really depends on the industry. If you look at our, we have two types of, uh, pipelines. It's a bad job. So we run some kind of pre-processing and we train a model, uh, maybe once per Rico. Once per day, and we create productions every day, for example.
So that's one type of a task. And then this, uh, predictions that are created by the model needs to be delivered. So some. And system. so, so that those predictions can be used in sending the emails, for example, or they're picked up by some server in another country so that it's being used, for demand forecast in the stores and other type of models that we have.
Um, those are basically APIs. So when you are on the website of. One of our shops, you are at the basket and then you get suggestions of the products that you may want to buy based on your basket. This is an API that we developed, cross-sell api. And for that, um, the pipeline will be a bit different, of course, right?
You have to train a model, but you also need to put that model behind an api. And right now we have standardized deployment on Kubernetes. So that deploying the same model, uh, to another brand, for example, is a very easy task. The deployment process itself, of course, you have to still fine tune the model, uh, to make it work because the data is different.
But the putting it to production, it's literally couple of minutes and now we are simplifying it a bit further. By using Databricks Server, a API deployment. Uh, I know you had a podcast with people from Databricks recently on this topic. so we really like that solution because the way Hub Kubernetes is used across different brands of our, it's a large retail company, it's different, someone.
Some have push type of deployment, so you use cube, Dell commands and some, some have pool type of deployments with Argo City. So you see already a huge difference in how you would bring that to production. But with Databricks, it's just the same. Maria mentioned, uh, about deploying to other brands. Maybe It's actually good to elaborate on that because, all Del HAIs differs from, um, maybe other enterprise organizations in the sense that it has 19 brands and all brands.
Um, some has data science teams, some has, uh, no team at all, but they all want similar data science products because they all have e-commerce websites. They all have, uh, grocery stores. So when it comes to, uh, standardization, actually for us it was even a bigger goal to standardize things so that if one brand develops a model, let's also give it to other brand and the other brand can just leverage it.
And what does your workflow look like, once you stop working on a project, I know you're a little bit further into the journey right now. Where you've already built the models and you've already chosen your stack, but when you sort of started out in, in this entire journey of building the envelope solutions for a hold, uh, what was, how did you choose, what were the key tools for you and what was the tech stack going to look like?
Or did somebody else previously choose that for you? Yeah, so when we started to work at a, we did MLS maturity assessment. And there are multiple versions of it you can find on, on the internet. Uh, then develop our own questions and, uh, we ask these questions to different people within the organization per project.
So already based on that assessment and uh, talking to people, we knew what kind of tools were used and it was very clear that everyone was using Databricks for training models. So, um, and Databricks was already within the company for a number of years. So it did make sense to stay with it and, well as any solution.
It has pros and cons, but we found it works quite well for what we need to do because we use park a lot for pre-processing. So that solution made sense. Um, also, uh, multiple brands were using already, uh, GitHub actions for C I C D. So, and also it's a policy of Arge to use that for software development.
And I think model is kind of software in some sense. So, Uh, we also use, keep using that and GitHub was for version control and Kubernetes was already used for API deployment. So we kind of, looked at what is being utilized at the moment and um, just to simplify the adoption of what we build, it makes sense to stay with tool that already used.
We are also on Azure, Azure Cloud, so we also, um, have some Azure native solutions. That be used. And so I want to dig into this like ML test score that you did, because I know there was that famous Google paper that came out back in the day and Microsoft also has their own version of it. And so a, what did you do?
You just sent links to people with a, a survey and you had them fill that out? Or did you go and shadow people for a day and watch how they were doing things and what their workflow was? What did that look like? And also how many teams did you actually have to interrogate? So indeed, we walk through the papers available out there and, but these papers are more on organizational level, not really on product level.
So we made it really product level like. Do you use version control for your, for your model? Like as simple as that? when you deploy a model, can you look back what code was used from what model artifact was used, what data was used for this particular deployment? So this kind of question, there are 70 of them, I think.
Um, oh my God, 70 Yes, we have like also standards. There is a standard document that we use. Uh, so we had 70 questions and we, uh, just had interviews with people. We scheduled call calls with them, and, uh, we asked those questions. some also, uh, we sent. This Excel sheet and we shared Excel sheet at SharePoint and they were filling it themselves.
This was, I think one and a half years ago when we started interrogating people and, uh, we're gonna measure your envelopes maturity. Uh, initially they were scared and, uh, later they were also not happy to see the results because, uh, obviously among those 70 questions, the max I think they achieved was 60% and they were not very happy to see that.
but I think it was also good reflection of their work. So eventually, um, they liked it as well. So purely hedonistic, any chance you would open source those questions to the community? I think we can do that. Oh my God, that would be so amazing because I know how many people have come through and asked for something like that.
Like how do I measure what a best practice is? Or how do I look at what I should be doing? Or I should even be focusing on. We can definitely do that. We also have standards document, and we, I, I wrote, uh, the best practices of data science methodology. It's like 20 pages document. I guess we could open, open source that, because there is nothing regarding our alcohol in it.
So we'll talk later about that. one thing that I.
I would also like to learn is when you are sharing, because you mentioned, oh, we have a model and maybe we wanna share this model with another team. How do you view a, data sharing concerns like data access, model sharing, all of that headache that I'm sure there's a lot of DevOps people that are listening and they're.
Just they're wondering that it's dying. They're dying to know like what is going on in this, in that case, well, we don't share data. Uh, we don't share models. The only thing we share is the code. So, uh, we have a centralized repository for each type of the project. So I'm was mentioning cross sell model.
Cross sell model is deployed for different brands. And there is one central repository with the logics of the model. so, and each repository for brand, it's, uh, actually using this package, the, the central package, and there is a configuration file. Uh, because the data locations, of course, different, but also hyper parameters of the model can be different.
And a model is trained separately for each brand in separate repository. But because it leverages the central package and central deployment framework, um, it actually makes it really fast to deploy things. To add on what Maria said, um, when we develop models, uh, we also keep in mind that multiple brands might use it.
So, uh, we develop in a way that it can be configured and parameterized. So that's why the central package, um, is more like a Python package imported in every brand ripple story and, uh, used to train and deploy models. one of the things I'm fascinated by, because you have this very interesting structure, uh, within the organization itself, where you have 17 different brands.
How does the code review happen internally inside the organization and what does the process look like? How many people are involved, which is like, how many tier engineers are there? How many ML engineers, how many data . And how long does it take to per, not just technically how long does it take to put the modern in production, but how long does the inception through the deployment look like?
Yeah, I think it really, uh, depends. So our team, um, is a team that helps the brands so, uh, to become more professionally in the machine learning and data science field. Uh, some brands don't have their own data science capabilities, so, We have, for example, supermarkets in Greece, Serbian, Romania, they don't have data scientists at all.
So, uh, our team develops models for those brands and we have, um, four data scientists and two machine learning engineers. So that's pretty much the whole team working on those solutions. And we also have one external machine learning engineer from another team that also helps us, and we have a platform team.
The platform team is, can be also different per brand. Uh, but the Greece, Serbian, Romania and Belgium, they share the same platform team and we have an agreement with them how things are deployed from infrastructure point of view, because that is also important. That's the key actually, to bring things to production fast.
And there are multiple architectural choices that can be made, how those, uh, environments are set up. So in our case for historical reasons, we are on Azure Cloud. And so resource groups are shared across the projects per brand. So each brand has separate resource group per environment, so production, pre-production, uh, development, uh, accept, um, test environment.
So, uh, those, um, have. The same service principles that are being used by multiple projects, and we keep secrets in as organization secret that are shared across repositories, but we also have some guardrails in place to make sure that those secrets cannot be misused. And that's something that, uh, we have in place as a product that our team provides.
, our team is slightly centralized as well, given that, , we are, uh, not, uh, attached to any brands. we are working as more consultants to brands from a global, uh, team. So this already gives us the chance to actually, um, develop models for multiple brands already, since the beginning. And, um, you asked for quote reweaving.
Well, our team develops the models. Our data scientist develops the models. There are also some data scientists from different brands they can also contribute. So it's more like a, um, open project within our organization that people can contribute and the people contributing to that project. already follow of certain, uh, software engineering best practices.
And I think it's also important to mention that the, the data is of course, important part of the whole thing. And there are data engineers, uh, on the platform site that, uh, make sure that the data arrives, to the prepared layer that is being used for the modeling part later on. The young sung heroes, those data engineers, making sure it arrives in the way that it needs to arrive and when it needs to arrive.
All of that good stuff. So there is something that you all mentioned, uh, a few minutes ago that I wanted to dig into also, which is not having this tooling sprawl and really looking at the user experience and recognizing that the user. Doesn't want to have to choose or go between 15 tools to get that whole end-to-end process done.
And so it seems like you decided on a select amount of tools. But one thing that I always think about when I look at the lops tooling landscape is how. Vague it is. There's like one tool that will have one value prop, but it also does these five other things. And then you have this other tool that has this other value prop and it does these five other things and there's a lot of overlap.
And so when you are choosing which tool you want to use and how you want to interact, how do a just end up on, okay, this is the absolute minimum that we should have. And these are the majority or these are the most important. Pieces that we should have, like these tools are going to get us 80% of the way there or 90% of the way there.
Y yeah. So, uh, I think we are coming back to this, uh, lops maturity assessment questions, right? So we have these questions and we want to go as high as possible in the percentage of the questions answered as yes. Yes. So we want to be as mature as possible, and then we need to define how do we do that with the tool that we already have need to understand in a corporate environment.
It's not easy to get yet another tool, even though that will cover a hundred percent for you. if that tool existed, I don't believe it does. Um, zero tools to all the magic for you that you need to need to do. Um, so getting any tool is, is a big struggle. So you have to do with whatever you have at the moment in a corporate environment.
And so we happen to have Databricks and actually, uh, Databricks helped us to cover quite a lot of the points. Of course, you need to you any tools you can use in the right way, and you can use it in the wrong way. Databricks makes it easy to use it wrong. Like you could create a notebook without any version control and you could schedule it, right?
You could, and you can claim, well, model is in production, but it's not really following any standards, right? So it's not about the tools, but it's also how you use the tools, I believe. One of the questions I had, Ruth, uh, there, you mentioned briefly about, uh, guard rails that you have in place. Can you go a little bit more and expand on that?
What are the kind of guard rails that you have while productionizing your models? Yeah, so I think I was mentioning the guard rails regarding the organizational secrets that we have in, uh, GitHub repositories. So, um, these secrets are being shared across multiple projects, but we need to make sure that, uh, those secrets cannot be just used by any repositories.
So, uh, we can only add a secret, uh, repository in the scope of organizational secret if it follows certain convention. So if it has a certain naming convention, and not everyone can just edit there, it can be only added by specific workflow and only certain people can trigger that workflow. So you are being checked whether you are part of certain team in, in GitHub, and if you are, then only you can trigger that workflow.
So in that sense, it's based on, uh, whether you are part of the right team to be able to. Add your repository to the scope of the secrets, and also if secrets are getting expired, uh, like all secrets, do we have a certain process in place that is run by our, platform team to renew the secrets? It's, it's really quite technical, I guess, and depending on different tools that he use, your guard, rest may be different, I believe.
But that's using the tools that we have, that's how it would look like. But that, that makes a lot of sense because again, this is one of the problems that I've seen, which is basically secret sharing, and the second is credential sharing, which has some sloppy processes that I've seen across organization where you have to have like, defined rules on who can exist, what.
And why it sounds prohibitive early on when you start implementing things, eventually the value delivery is really high. One of the things I wanted to know from you guys was what was the career for working before these envelopes processes were implemented? Yeah. You can sit like a nightmare. You want to know?
Yeah. Uh, that, that was really bad. Um, I think I'm coming, uh, from a comp. Bernie, where I was in quite luxury position in the sense that, well, telecom company I worked for, they were already using all the best practices. And what I have seen when I started working here, Uh, that there were some external companies, uh, building data science solutions, and then they would want to put that in production and they would literally zip the code and create the wheel files and send it over by email to a DevOps team to deployed.
Uh, yeah, I was in shock. yeah, well, you can imagine, right, the environment where a data scientist, uh, well create these wheels and, uh, run the code. They're also bit different than the DevOps engineers environment. So the errors would occur and then they would send over those errors messages in the email back, and then you would try to reproduce them so it can take two weeks.
Right before the issue is resolved, it's a nightmare. And it took us a year, uh, to get, uh, all permissions. We need to deploy ourselves, like getting this organizational secret for service principles and defined, um, in GitHub. So to agree on that, it took us a year. Yeah. In a nutshell, the biggest change we made, uh, with Maria is.
To actually break the wall between data scientists and DevOps engineers and get the responsibility of deploying models ourselves because, we can create the models and we can deploy the models within the same team so the ownership stays in the same team. And the, the errors won't have to be emailed from one team to another team.
And he also had something to say about the distrust. The distrust between the DevOps engineers and data scientists. Explain a little bit more about that as well. Yeah. So, um, I believe data scientists, they typically come from econometrics. Background, statistic background. You see a lot of them, well, I'm one of them, I guess.
Um, and you see DevOps engineers and know all the best practices, uh, how to build software data scientists. Don't always know about these practices. So, and when Dell engineers see data scientists doing something that they wouldn't necessarily do, there is distrust that appears. And you need to build that trust between these different teams, that data scientists actually can put things in production themselves because no one would give just permissions like that.
Right. As I mentioned, it took us a year. Um, so you need to build that trust that you also can handle that your yourself in a production environment. do you think there's a difference between lops and DevOps? What are the key differences occurring to you guys between both the fields and how does that change between the two different kinds of models that you're putting on or between the two different systems you have where one is the forecasting models and the second is the API models?
What are the key differences when you're thinking about envelopes and both the things. Yeah, so I believe there is quite a big difference between, uh, Mel ops and DevOps. And one comes, uh, with, it's not just, um, the code that you need to, to run, to be able to put things in production. It's also the data part, right?
And the data part makes all the difference here because. Even if your covid, uh, didn't change, your data did change and aeros may appear. And, um, therefore getting access to production data in development environment might be very important for data science product. And that's also another struggle that I believe many people have because of all the rules to get the red access to production data in a, in environments different than production.
so that's also the struggle that we had, for example. And, uh, it took some convincing to actually get permissions and everything. Uh, another one is, um, checking for the quality, the monitoring. Check it for the quality of your model when it is deployed. Cause when whatever software is, is deployed, there are also of course, some type of health checks that you need to perform here.
The quality of your model can deteriorate. The model can get worse. And if you don't have those checks in place, you just wouldn't know because, well, from the software point of view, everything is just fine. So these two, uh, difference, I think are the biggest ones. The classic question that we tend to ask on the monitoring pieces, who gets the call when the models deteriorate at 3:00 AM?
Well, I believe that depends on the model. So if you have a model that is critical for the business in the sense that if it's not working for some reason, you lose a lot of money. So we had such a use case, um, at previous company worked at that. Um, it was, um, bad debt model. People would go and get subscription for the mobile phones on the website.
And the model will either accept or reject the person from getting a mobile phone with the subscription, and if the model was not answering, it would cost 10,000 euro per hour. So you can imagine this kind of models are critical, right? You need to have some checks in place, and there should be a team that, uh, fixes it as soon as possible.
And I was also called in the weekends to fix that model, for example. but we personally right now don't have such use cases where pigs would be needed at three o'clock in the night. Really, really, uh, because if a cross town model is not working well, there are some costs maybe involved. But in the night, who is ordering online groceries?
Anyways. Yeah, probably not so many people. drunk people, so I can imagine drunk people. Well, unfortunately they don't get delivered instantly, so it doesn't make sense for drunk person to order the night anyways. It would be delivered like in two today's neighbor and the next day, oh no, in the best case.
So, yeah, the cost of not, of things not working are not that high. Good. Yeah. So in that sense, um, No one gets cold in the night, but monitoring is still a key. Um, yeah, we are also working on our monitoring component because it's definitely important to keep track on how your model is performing. Um, in our previous way of working before we arrived, we have seen models predicting, um, ridiculous suggestions and not being even recognized.
Uh, this cross model, for example. Imagine an e-commerce website and you just keep seeing trash bags suggested all the time and why, and this has not been even, uh, realized for a long time. So it is not a very expensive cost when it comes to not functioning data science product, but it is still, uh, important to keep monitoring.
Yeah, I think it's also the trust of the customer, right? If the customer sees stupid suggestion, they wouldn't pay attention the next time. So that's, uh, like a future cost, accumulative cost? Well, I, that comes with it. Yeah. I remember one time we had a, one of our first meetups ever in the lops community back in March, 2020, Favio came on, and he was saying that at one of the companies he was at, They recommended to every single user no matter what.
The user was the same item, and I think it was like women's high heels. And so it ended up costing the company a ton of money because the money that they usually get from these extra buys when you have. A recommended item that someone clicks on. They lost all of that because they were recommending it not just for like a few hours, it was 18 days straight before they realized that anything was wrong, and that ended up being something that they realized, wow, this is actually more important than we had originally thought.
We also had this example where we, where the, the personalized model gave a very expensive gin as a suggestion to all customers, and we had no clue why it happened. And since it was also. Before envelope standards, we were not able to track why.
Um, but the, we don't have a purpose of getting everybody drunk, that's for sure.
It's classic and that's why people are ordering things in the middle of the night. Now we know it's cause you suggested, oops. But there is one thing I wanted to follow up on and it's. You mentioned before about notebooks and how there is now a process around it, and I think that is one of the most contested and hot topics of the ML ops community.
And might just be because I bring it up so much, it's a little too cliche now, but what does the process look like from that exploratory phase in notebooks to actually then productionizing it? so it, it really depends what kind of notebooks you use. So since we use Databricks, notebooks and Databricks are not exactly the same as I iPads and notebooks, luckily they are not.
So, uh, they basically do just look like Python files and there is. Mark down command that Databricks understands is an notebook, so it makes your life a lot easier. Uh, so our data scientists love notebooks as all data scientists do, and they do start developing in an notebook most of the times. But we encourage them to move all functions, uh, and all classes that they write outside of the notebook.
And Databricks has a nice feature called Databricks repost that allows. To, um, to use these classes in, in such a way, and you can also package it. so we also encourage to create, uh, patent packages as soon as possible because the way how we work with Databricks is in production. Uh, we build the wheel and we upload the wheel to Dbfs Bs.
Uh, but to test it, uh, in a notebook, it's just very easy with this, with this repo feature. So we also used, uh, now vs code extension for data risks a lot, I believe. I personally have not used it that much, but I guess Bash has more experience with that. Maybe you can tell a bit more about it. Yeah, we allow our data scientists start from notebook.
We don't have any grudge on notebook, and they can start exploring, especially in Databricks, where they have direct access to data. But we also, what, uh, what we also tell them is to start writing production ready code. I think this is also one of the important, um, takeaways, uh, from what we learned. Is that train data scientists in a way that they write modular functions, they write production ready codes, um, they start learning some software best practices.
And, uh, with that, when it comes to actually going to production, we can easily migrate our. Code from the notebooks to a GitHub repo story. Uh, here. Also, Gith repos plays an important role because it allows us to synchronize GitHub repository stories to Databricks environment. Um, now also with another feature, uh, recently released from Databricks called VS.
Code Extension. They can also develop things locally, but run in database clusters via VS code. I think this also allows them to already start writing, um, in an ide, uh, while running on database clusters. So this already, uh, encourages them to write more mature codes. Yeah. And we also of course emphasize on importance of unit testing.
Uh, I think that's a key, uh, and also documenting things properly. Uh, one part of our pipelines is also, uh, doing checks on code quality. So you basically cannot deploy if your code doesn't look well. And that's, um, if you use our, uh, workflows that we provide. Uh, so that's something that we also kind of try and to enforce, I guess.
And how, what does the process internally look like when you tell somebody, you know, you want to write production of code? Do you provide people a template internally often for a good code for a model look like? Or is it to like constant feedback on their existing code? Yeah. So, uh, when a data scientist starts with the project, we recommend to use our cookie cutter template.
And it's not like using a cookie cutter template. It's a, a workflow that they can run from the repository that we have of cookie cutter repository, and it create repository for you with all permissions already. So basically your repository will be added to the scope of organizational secret, and it'll have code that can run, it'll have with Hello World in it.
Um, and then, uh, they can deploy it already, this hello World Code, but they can also adjust this code. And then the C pipeline to check the quality of the code, um, that that will run. So those things we already provide, uh, as part of this template. Uh, we also have of course examples of the existing projects where they can see, and we also have some conference documentation from how to do that.
Um, and we try to talk to them a lot. I think that's also important piece. Yeah. Having examples is already. Good guideline for them. But I think there is also a bit of, uh, trust between us and them. We rely on them to write, uh, need codes and follow the guidelines we show them. So when you look at the whole process that you have now and what you've been able to accomplish, what are the areas that you feel like still need work or you have blind spots in?
Yeah. Uh, so one of the things that we already started working on, um, is, uh, the cost management. I think that's a very important thing when you are on a cloud, because costs can go high without you even knowing about it. So especially on data. Yeah. Well, Yeah. Data brick can also get expensive if you choose the wrong type of clusters.
Um, anything can actually, uh, so we tag things properly because resource groups are shared across multiple projects. This workflow that we have, they tag tag things. So we tag data brick jobs, and when Databricks job jobs are attacked, they will promote the tech to whatever resources Databricks job creates to be able to run the job.
And then you can see in Azure cost management, uh, how the costs were associated with different runs. Um, that's something that is now been handled by another team, but we would ideally want to have our own dashboard with all information about all the runs, with some metadata about the runs, but also with associated costs.
So that's something that, uh, we want to still improve on. And I think overall monitoring, um, there is, um, yeah, scattered, uh, tooling for monitoring that we used across the organization. So some, uh, use a lot Grafana, uh, prime use Azure Data Explorer. Uh, elastic Storage is also part of the STACK Team. So it's really everything you can find we have and, um, I think it is important first for the platform team to make the decisions on what the tooling we gonna have, because standardization is a key, uh, also for monitoring.
And we want to, uh, build integration layer with this monitoring tool of choice for the platform. But because we are waiting for this decision to be made on what, uh, tools are gonna adopt for the whole platform, it's a bit, um, dirty at the moment. I would like monitoring to be improved, but yeah, for that you need to have a tool of choice first, and that goes beyond the mals.
So this tooling just for everything, right? Not just for machine learning in addition to. The cost management? Uh, well actually since we started focusing on the cost management, we started seeing how much actually a model training cost, let lane euros. And, uh, this also motivated us to calculate how much value we actually bring because if we are.
Spending more money than we actually are making money than what's the purpose of ha having a data science model? And, um, when it comes to recommendation systems, it's a little difficult to calculate the actual revenue you get because, uh, it's not a direct, um, transaction. But we still found our ways to calculate our KPIs, um, the, the value in terms of revenue, uh, on our websites.
This already, um, allows us to have a clear image of how much model costs and how much value brings. Not every model brings as much as value, um, as models like demand forecast, for example, but it's still nice to see. And compare. Yeah. And we did already a lot of cost saving, by the way, because, uh, we mentioned there were models developed by the third parties, and the way how they were deployed is just crazy.
There was a process in place, uh, an Azure function that would create in Kubernetes cluster, and it'll then, it, it'll way too big, bigger than it's needed to run a thing. Then it'll run a thing and then it. The Kubernetes cluster will be killed. So, and every day, any Kubernetes cluster will be created. But if a job failed, uh, then Kubernetes cluster wouldn't be deleted.
So you end up having those Kubernetes cluster without any monitoring, having huge amount of costs. Uh, so by doing the mail ops in the way, in this standardized way, using the framework that we built, we were able to reduce the cost by 90%, 90. Um, that's huge. And also in general, we have seen a lot of processes, uh, where we could improve.
Like we've seen processes where interactive clusters and are being used for Databricks. Uh, so it makes sense to move them all to job clusters because of, uh, smaller cost on Databricks side. And also to optimize for the VM sizes and VM types on Azure. Uh, so we want to move from V2 types to V4 V five, which have better, uh, performance price, performance ratio, but also pick just right size of the cluster to run a job instead of having a bigger cluster that can accommodate for running multiple types of processes.
So, um, and by that we also, uh, To save a lot of money. I think it's a good moment. To repeat what Maria said initially, any tool can be used bad, or any tool can be used good. Uh, any tool can be very expensive or any tool can be very cheap if you use it wisely. So Databricks can be very expensive or you can actually, uh, find a way to make it cheaper.
Such good wisdom. That is so cool to hear about. And really, I love that you are taking this approach of, Hey, we need to prove our worth and we need to show people that what we're doing is actually making the company money. It's not a cost center, it's a, it's a profit center and that gives you so many more resources to be able to champion new projects and show your worth.
Uh, I want to know. How many people are coming to you asking to use AI and chat g p T on new projects? Oh, well actually zero. I've never heard anyone asking us to use, uh, chat, G p t yet. However, we have, uh, a teammate on the principal data scientist. She loves chat, G P T, and she's using it a lot. For pretty much everything.
Um, but I believe it has not created that hype yet within our organization, at least I believe within many other organization. Indeed, though we also had a lops conference recently and we had one talk on, uh, how chat G B T is being used now at ikea. So, um, There is some change happening in because of C G B T, but I cannot tell yet about our own company yet.
I started using co-pilots on GitHub. I think that's a really nice tool. Um, but indeed we haven't heard many C G B T Reers in our organization, at least not yet. So you're still untouched by the entire generative L L M hype. Well, we are touched, but not, not as organization, I believe. Not yet. Fantastic. So I think this is a good time.
We should wrap up this call or interview. Uh, but it was really wonderful talking to both of you. I, I, I think I can comfortably say, and I've not said it live yet, which is, this was my favorite interview til it, and start. Well, thank you. In case you guys were so open about all the things, including what your processes look like.
as brothers talking about modern maturities. These are, and these are things that I do find a lot of people talking about. Again, the revenue question is something everyone talks about, which is, Hey, as a data size team, we need to make revenues, but nobody really puts that in perspective. Some too. But still I will find that missing, at least from a big picture.
Rain doesn't make sense. So I really love this conversation. Thank you. I really enjoyed it. It was really fun. I definitely enjoyed.