3 Takeaways From Our Survey Of Top ML Teams
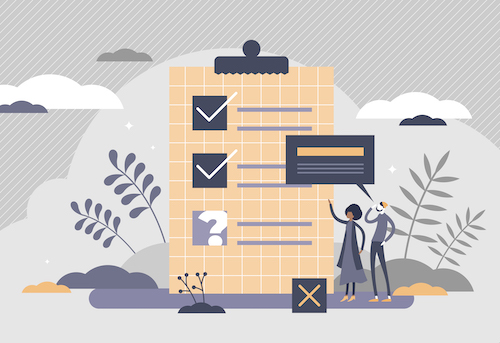
This blog highlights findings from Arize AI and MLOps Community’s recent survey of ML teams
February 16, 2022

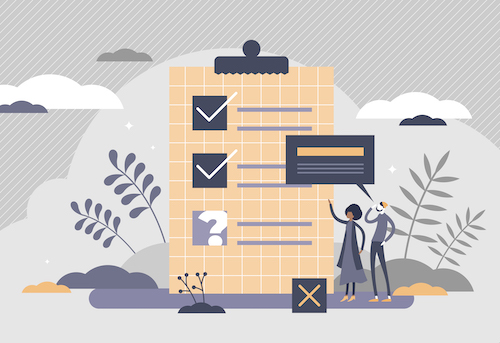
This blog highlights findings from Arize AI and MLOps Community’s recent survey of ML teams. To see the full results, download a copy of the report.
Compared to DevOps or data engineering, MLOps is still relatively young as a practice despite tremendous growth. While it’s tempting to draw parallels to DevOps in particular since some of its best practices easily carry over into MLOps, most in the industry agree there are a unique set of challenges and needs when it comes to putting ML into production. Unfortunately, few robust industry surveys exist to document how teams are faring in navigating these distinct challenges.
To remedy this and give MLOps practitioners insights from peers on how to thrive professionally and ship and maintain better models, we recently conducted a survey of 945 data scientists, ML engineers, technical executives, and others. The results speak to a distinct need for better collaboration across teams and better tools to aid in faster root cause analysis when models fail.
Here are three of the survey’s key findings on ML monitoring and observability – and recommendations about what teams can do about them.
Troubleshooting Model Issues Is Still Too Painful and Slow
Despite progress, ML teams frequently face delays and frustration when it comes to troubleshooting, triaging and resolving model issues in production environments. In all, 84.3% of data scientists and ML engineers say the time it takes to detect and diagnose problems with a model is an issue for their teams at least some of the time – and over one in four (26.2%) admit that it takes them one week or more to detect and fix an issue with a model (i.e. retraining a model in production after detecting concept drift). Delays of one week or more are most common in financial services, followed closely by healthcare and technology.
These issues are likely exacerbated by a post-pandemic environment where drift and performance issues are elevated, according to nearly half (48.6%) of teams.
Recommendation: Evaluate and implement an ML observability platform that helps expose and eliminate AI blindspots.
Virtually all ML teams monitor known knowns – model metrics such as accuracy, AUC, F1, etc. – and most also try to address black box AI (known unknowns) through explainability. However, often what’s missing are solutions that expose the issues teams are not actively looking for: blindspots, or unknown unknowns. True ML observability can help eliminate blindspots by automatically surfacing hidden problems before they impact business results. Rather than writing seemingly interminable queries to get to the bottom of performance degradation, teams using a modern ML observability platform can quickly visualize the full array of potential problems and perform root cause analysis in a few clicks.
ML Teams Need To Communicate Better With Business Executives
Despite the fact that ML models are arguably more critical to business results in the wake of COVID-19, over half (54.0%) of data scientists and ML engineers report that they encounter issues with business executives not being able to quantify the ROI of ML initiatives often or somewhat often – with nearly as many (52.3%) also reporting that business executives do not consistently understand machine learning. Likely contributing to this disconnect is the fact that “sharing data with others on the team” and “convincing stakeholders when a new model is better” remain issues at least sometimes for over 80% of ML practitioners.
Recommendation: Grow internal visibility, increase ML literacy and tie model metrics to business results
Business executives need better access to tools and digestible, relevant KPIs – including, most importantly, a way to quantify AI ROI. By tying ML model performance metrics to key business metrics and giving executives access to dashboards that track progress over time, ML teams can ensure broader buy-in. To help make this a reality, ML teams assessing an ML observability platform may want to consider product capabilities like support for setting a user-defined function to tie model performance back to business results, the ability to compare pre-production models to current production models (champion and challenger) and the ability to dynamically analyze thresholds for probability-based decision models. Additionally, a platform that supports shareable links to charts with filters saved can also be helpful in cross-team collaboration.
Explainability Is Important, But It Isn’t Everything
Although technical executives place a high importance on explainability, ML engineers – who are generally the ones getting models into production and maintaining them once there – rank monitoring and troubleshooting drift as a higher priority, putting explainability on par with monitoring performance and data quality issues.
Recommendation: Do not rely on explainability alone; take a proactive approach to model performance management.
Focusing on explainability in the pre-production phases of the model lifecycle – training a model and validating it before deployment – can be useful. However, continuing to expend the bulk of resources on explainability once a model is in production is of limited utility since it creates a passive feedback loop. While explainability is useful for sorting when troubleshooting model performance in production, it does not help you surface blindspots the same way that data quality monitoring helps proactively catch potential issues before a substantial shift in inference distributions occurs, for example. By setting up automated performance monitors across a given model, ML teams can have a first line of defense — especially if able to A/B compare datasets and perform data quality checks. Drift monitoring across environments or prior periods of production can also be an early signal that model outputs are shifting.
Conclusion
While these are not the only issues facing MLOps teams, they are some of the most salient according to those surveyed. See the full list of findings by industry and role in the report: “Survey: The Industry Is Ready for ML Observability At Scale.”