BKFC: An Agentic Workflow for Gathering Knowledge from Google Chat
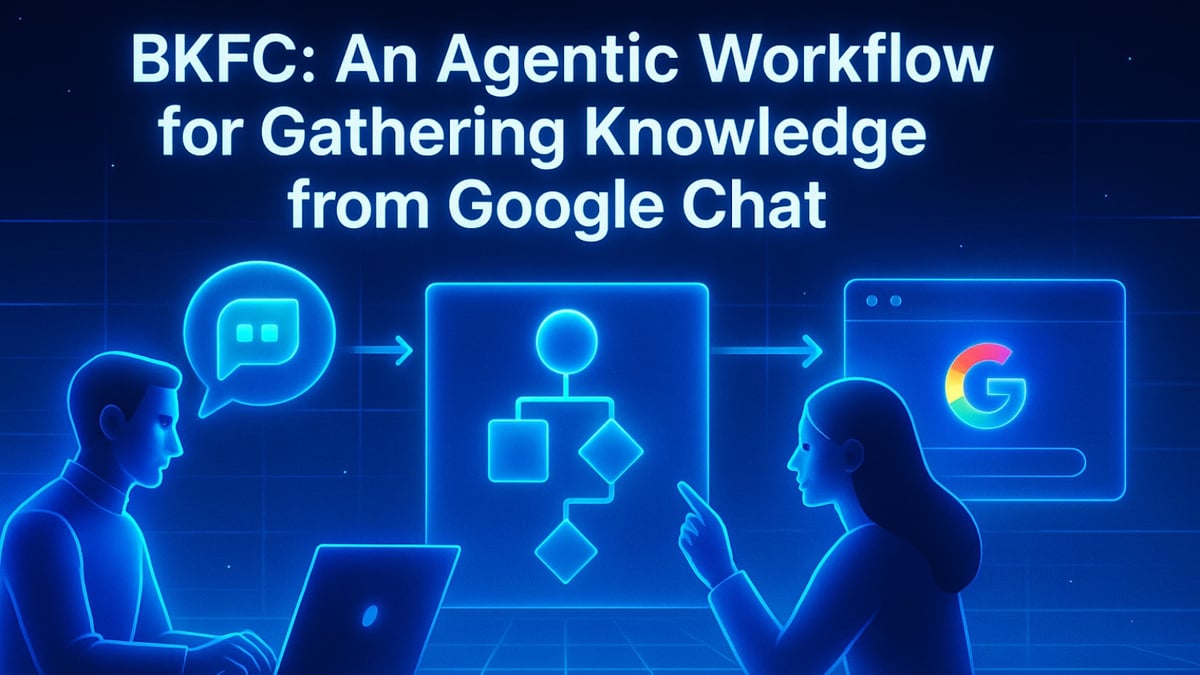
Leveraging AI and Structured Output to Extract Actionable Insights from Team Conversations
April 29, 2025
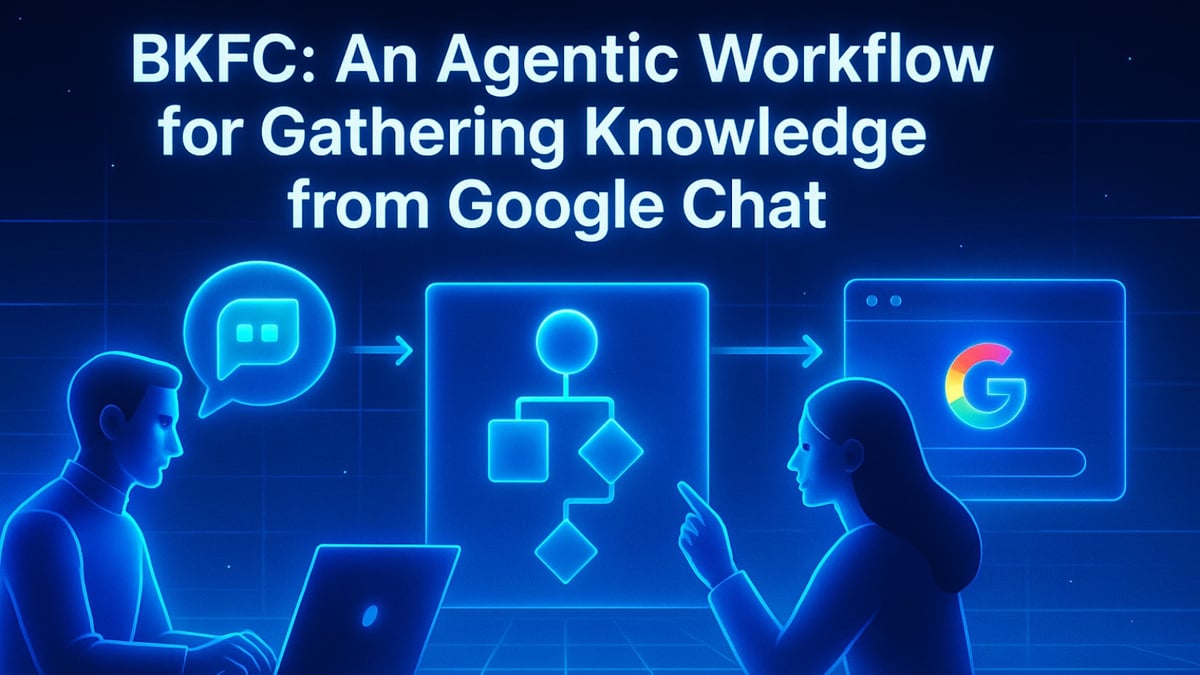
Team collaboration often lives and breathes within chat applications like Google Chat or Slack. It’s where questions are asked, decisions are made, project updates are shared, and solutions are brainstormed. But let’s be honest: extracting specific information from weeks or months of chat history can feel like searching for a needle in a haystack. Important context gets buried, action items are forgotten, and valuable knowledge becomes siloed.
As someone passionate about AI, Generative AI, and MLOps, I’m always looking for practical ways to leverage these technologies to solve real-world problems. The challenge of unlocking the latent knowledge within Google Chat seemed like a perfect opportunity. How could we quickly surface key insights without manual scrolling and searching?
This led me to create BKFC (Build Knowledge From Chats), a Python notebook designed to act as a simple yet powerful insight-gathering agent for Google Chat. My goal wasn’t just to extract information, but also to demonstrate how quickly valuable, focused agentic workflows can be built using modern tools like Google Colab, the Google Chat API, and Vertex AI’s Gemini models.

The Motivation: From Chat Chaos to Actionable Insights
In many teams, Google Chat spaces become informal knowledge repositories. You might find:
- Questions asked and answered.
- Updates on ongoing projects.
- Implicit or explicit action items.
- Valuable technical discussions, code snippets, or configuration details.
- Feedback and suggestions for improvement.
Manually scrolling through this history is inefficient and error-prone. Key information can be easily missed, especially as teams grow and conversations multiply. I wanted a way to automatically distill recent conversations into structured, actionable summaries.
How BKFC Works: An Agentic Workflow in Action
The notebook follows a straightforward, agentic process: Fetch -> Process -> Analyze -> Report.
Setup & Authentication: The first step involves standard Google Cloud setup. This means enabling the Google Chat and Vertex AI APIs in a GCP project and creating OAuth credentials (specifically for a Desktop app) to allow the script to securely access chat data on your behalf. Colab’s secrets management handles the client ID, secret, and project ID securely. Authentication uses the gcloud auth application-default login flow, granting the necessary chat.spaces.readonly and chat.messages.readonly scopes.
Fetch Data (Perception): The agent’s “perception” phase involves using the googleapiclient library to interact with the Google Chat API.
- It lists all chat spaces the authenticated user has access to.
- It filters these spaces to only include those active within a defined recent period (e.g., the last 100 days, configurable via SINCE_DAYS).
- For each relevant space, it fetches messages created since that period, respecting API pagination (PAGE_SIZE).
Process Data (Preparation): The raw message data needs some structuring before analysis.
- Messages are sorted chronologically within their respective spaces and threads.
- They are then grouped by the parent chat space.
- For each space, the formatted text of the messages is concatenated into a single “page” or document representing the recent conversation history for that space.
Analyze Data (Reasoning & Action): This is where the Gen AI magic happens, powered by Gemini via the Vertex AI API (python-genai library).
- Structured Output Definition: A key aspect is defining what we want to extract. I used Pydantic models to define a clear schema (ChatInsight) for the desired output. This schema includes fields for:
- summary: A concise overview of the discussion.
- questions_answers: Pairs of questions and their corresponding answers found in the chat.
- unanswered_questions: Questions asked that appear unresolved.
- projects: Mentioned projects and their status/details.
- action_items: Tasks identified, potentially with assignees.
- feedback_suggestions: Ideas or critiques shared.
- technical_insights: Specific mentions of MLOps, AI tools (like Vertex AI), code, etc.
- API Call: For each space’s conversation page, a prompt is sent to the Gemini model (gemini-2.0-flash is a great choice for speed and cost-effectiveness here). Crucially, the API call specifies the desired response_mime_type as application/json and provides the response_schema (our ChatInsight Pydantic model). This instructs Gemini to format its response according to our defined structure.
- Parsing: The library automatically parses the JSON response back into a Pydantic ChatInsight object.
Report Results (Output): The final step is presenting the extracted insights.
- The structured ChatInsight objects are iterated through.
- Readable Markdown summaries are generated for each chat space, organizing the extracted information under clear headings (Summary, Q&A, Projects, Actions, etc.).
- The raw structured data is also saved to a jsonlines file, which is ideal for potential downstream processing or integration with other tools.
The Value Proposition: Why Bother?
This simple notebook, acting as a focused agent, delivers this added value:
- Rapid Knowledge Retrieval: Quickly get summaries and key points from recent chats without manual searching.
- Action Item Tracking: Surface potential tasks or commitments that might have been missed.
- Project Awareness: Get a quick pulse check on mentioned projects.
- Identify Unanswered Questions: Highlight areas where follow-up might be needed.
- Technical Knowledge Sharing: Easily find references to specific tools, techniques, or solutions discussed.
- Efficiency: Saves considerable time compared to manual review.
- Demonstrates Practical AI: Shows how easily accessible Gen AI models and APIs can be combined to build genuinely useful tools today.
Conclusion: Simple Agents, Tangible Value
The BKFC notebook is a practical example of how agentic workflows, even simple ones, can provide immediate value. By combining the power of the Google Chat API to access data and the reasoning capabilities of Gemini models guided by structured schemas, we can transform conversational noise into actionable insights.
It highlights that you don’t always need complex, multi-turn conversational agents to benefit from AI. Focused, task-specific agents like this can automate tedious processes and unlock information trapped in our daily communication streams. As AI and MLOps practitioners, building these kinds of targeted solutions is becoming increasingly straightforward, enabling us to enhance productivity and knowledge sharing within our teams.
What other routine information retrieval tasks could be automated with a similar approach? Let me know in comments!
- Github Repository:
- Colab Notebook: https://github.com/fmind/BKFC https://github.com/fmind/BKFC/blob/main/BKFC_Build_a_Knowledge_base_From_Chats.ipynb

Originally posted at: https://fmind.medium.com/bkfc-an-agentic-workflow-for-gathering-knowledge-from-google-chat-b521cba535d7