Distributed Training in MLOps Break GPU Vendor Lock-In: Distributed MLOps across mixed AMD and NVIDIA Clusters
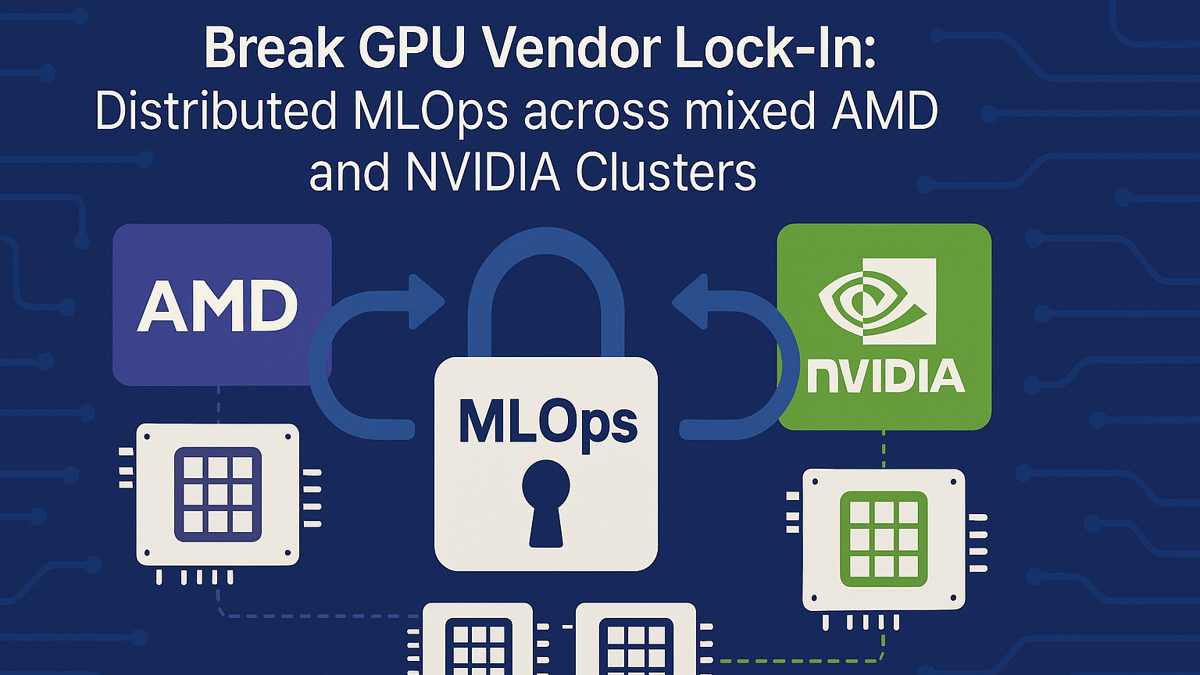
# MLOps
# Machine Learning
# Kubernetes
# PyTorch
# AWS
Unlocking the forbidden cloud hack: Running distributed PyTorch jobs on mixed GPU clusters with AWS NVIDIA g4dn and AMD g4ad instances
April 7, 2025
Rafał Siwek
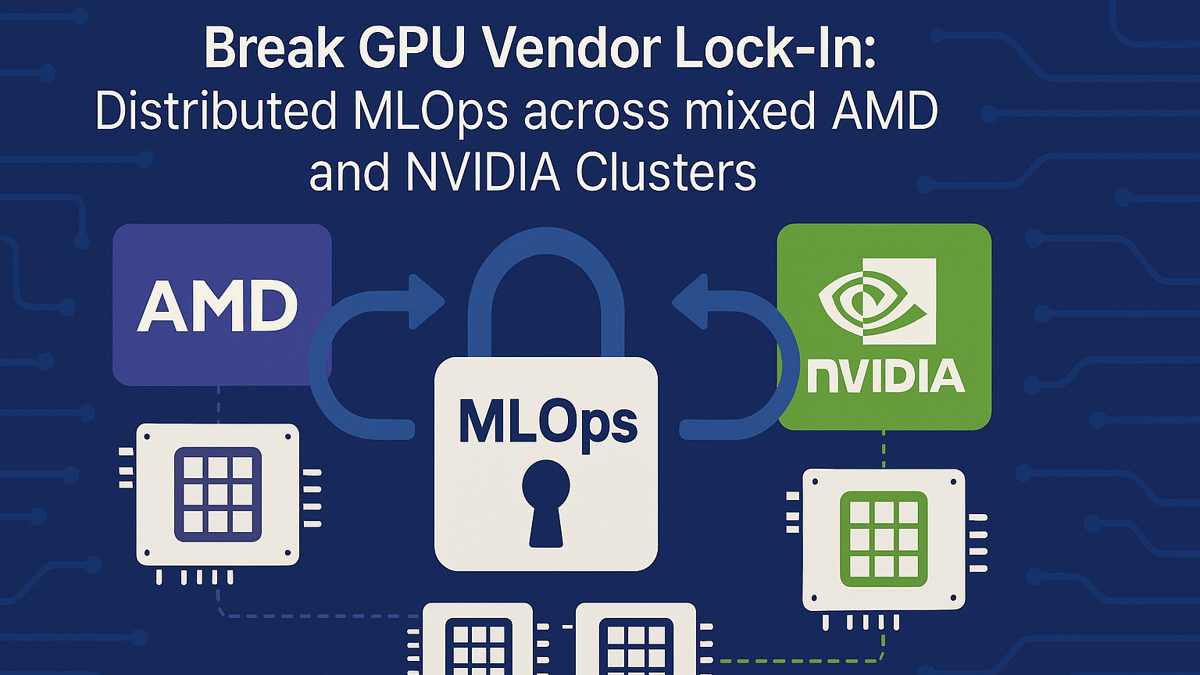

The unexpected alliance: AMD and NVIDIA GPUs for distributed machine learning, together (AI-generated image using Ideogram 2.0)
By 2024, tech giants like Apple, Microsoft, and Intel have acquired over 60 AI and machine learning companies, according to a report by the Center for Security and Emerging Technology (CSET). These acquisitions aim to boost innovation and consolidate expertise in scalable machine learning, where efficient GPU integration is crucial for high-performance AI infrastructure. However, acquiring competitors or merging with partners often results in mixed hardware clusters. An organization might start with NVIDIA GPUs but face challenges after acquiring a team set up on AMD GPU clusters. Overcoming vendor lock-in and unifying heterogeneous clusters is essential for cost-effective, distributed training solutions.
Vendor lock-in intensifies integration challenges by confining clusters to isolated ecosystems. NVIDIA’s CUDA and AMD’s ROCm rarely interoperate, which forces teams to silo workloads and leads to underutilization of available infrastructure. As models grow larger and budgets tighten, replacing GPUs every 2–3 years becomes unsustainable. The solution is to leverage heterogeneous clusters that utilize all available GPUs, regardless of vendor.
In this article (Part 3 of my series on distributed processing in MLOps), we explore how to configure devices for distributed training with PyTorch across mixed AMD/NVIDIA GPU clusters on AWS. By bridging CUDA and ROCm, we learn how to:
- Utilize mixed hardware without rewriting the training code.
- Maintain high-performance collective operations — such as all_reduce and all_gather — that efficiently aggregate and share data (e.g., gradients) across AMD and NVIDIA GPU nodes for synchronized training using UCC and UCX.
- Run Distributed PyTorch Jobs on heterogeneous local and Kubernetes Clusters with AWS g4dn (NVIDIA T4) and g4ad (AMD Radeon V520) instances.
See other articles in this series:
Part1 — Accelerate MLOps with Distributed Computing for Scalable Machine Learning
Enable your MLOps platform to train bigger and faster — because your models deserve a “team effort”
Part2 — How to Efficiently Use GPUs for Distributed Machine Learning in MLOps
How Industry Giants Slash Machine Learning Training Costs and Achieve 5.6x Speed Boosts
Cloud infrastructure setup, code, and detailed instructions are available in my repository:
GitHub — RafalSiwek/distributed-mlops-overview: An exploration of distributed processing solutions…
An exploration of distributed processing solutions for MLOps. — RafalSiwek/distributed-mlops-overview
Cluster Heterogeneity
Cluster heterogeneity spans a continuum from mild to strong, with each level necessitating distinct management strategies in distributed machine learning and high-performance computing environments. These clusters, which predominantly rely on GPUs as primary accelerators, can also exhibit variations in CPUs, memory configurations, storage and interconnect technologies. This chapter concentrates on HPC clusters with GPU heterogeneity, addressing mild variations within a single vendor’s ecosystem and pronounced differences in mixed-vendor setups.
Mild Heterogeneity
Mild heterogeneity involves variations within the same vendor’s ecosystem, like NVIDIA V100s with A100s or AMD MI50s with MI250X accelerators. In this example, these GPUs share architectures, drivers, and communication libraries, allowing frameworks like PyTorch to manage discrepancies through abstraction layers.
Challenges in mildly heterogeneous clusters:
- Compute imbalance: Older GPUs lag behind newer models, creating bottlenecks.
- Memory mismatches: Devices with smaller VRAM limit batch sizes.
- Interconnect variability: PCIe Gen3 vs. NVLink/NVSwitch affects data transfer rates.
Solutions:
- Utilize the Parameter Server distributed strategy for a more fault-tolerant distribution workload
- Dynamic Load Balancing: Implement smart workloads with device utilization tracking, assigning smaller batches to slower GPUs.
- Gradient Compression: Reduce communication overhead for bandwidth-constrained nodes.
- Containerization: Use Docker images with CUDA/ROCm versions tailored to specific GPU generations for compatibility.
Collective communication remains effective in mild heterogeneity due to vendor-specific libraries like NVIDIA’s NCCL or AMD’s RCCL, which are optimized for their respective ecosystems.
Strong heterogeneity
Strong heterogeneity involves clusters blending devices like GPUs from different vendors (e.g., NVIDIA and AMD).
NVIDIA CUDA and AMD ROCm are designed for their hardware, using different instruction sets, memory management, and driver interfaces. This lack of a common foundation means that load balancing — which relies on shared communication backends — and FSDP — which depends on uniform memory semantics — cannot function across both platforms.
Currently, no standardized solutions exist to tackle the challenges of strong heterogeneity. This gap calls for proposing strategies that enable transparent utilization of mixed-vendor clusters while achieving near-native performance by minimizing communication overhead between AMD and NVIDIA GPUs. This defines the following goals:
- Transparent Utilization: Run distributed training without rewriting model code or segmenting clusters by vendor.
- Near-Native Performance: Minimize communication overhead between AMD and NVIDIA GPUs, approximating NCCL/RCCL speeds and enabling efficient distributed techniques with RDMA-capable collectives and GPU P2P communication.
In the following sections, I will detail my efforts to enable collective communication for distributed training with PyTorch on AWS G4dn instances (equipped with NVIDIA T4 GPUs) and AWS G4ad instances (equipped with AMD Radeon V520 GPUs). The focus will be on leveraging available Collective Communication Libraries to tackle the challenges of strong heterogeneity.
RCCL port of NCCL?
NCCL (NVIDIA) and RCCL (AMD) are GPU—optimised Collective Communication Libraries, integrating low-level optimizations that directly exploit GPU Direct RDMA and, when necessary, socket transports.
The first optimistic sign I came across was when I studied the RCCL changelog — Compatibility with NCCL <version>. But no matter what version configuration I used or what optimization changes were applied, I always
got:
NCCL WARN NET/Socket: message truncated: receiving X bytes instead of Y.
This was revealed to be a dead end, as it appeared that even though RCCL is a port of NCCL, many under-the-hood changes prevent heterogeneous usage of RCCL and NCCL across a cluster. The libraries rely on low—level hardware integrations and the reliance on distinct kernel-level optimizations, memory hierarchies, and IPC mechanisms would make compatibility non-trivial.
An efficient communication middleware is required.
Unified Communication Framework
While searching for a suitable solution, I stumbled upon the Unified Communication Framework (UCF) — a consortium of industry, laboratories, and academia with the mission of unifying HPC communication.
The product that sparked hope— Unified Collective Communication (UCC) — an open-source project offering an API and library for group communication operations used in high-performance computing, artificial intelligence, data centres, and I/O. Its goal is to deliver efficient and scalable collective operations using algorithms that consider network topology, straightforward software methods, and hardware acceleration for in-network computing.

UCC works with a transport layer middleware — Unified Communication X (UCX), using its fast point-to-point communication routines and utilities. The UCX design is based on lessons learned from several projects, including Mellanox’s HCOLL and SHARP, Huawei’s UCG, open-source Cheetah, and IBM’s PAMI Collectives. And what’s most important — both UCC and UCX implement support for ROCm and CUDA

UCC is implemented as an experimental backend flavour in the PyTorch distributed module. It can be specified as a direct backend for the PyTorch distributed module or as a backend for collective operations in OpenMPI. For this, building MPI-aware torch from source is required and OpenMPI-backed distributed jobs must be executed using the mpirun launcher.
This proved to be a breakthrough; with guidance from the UCF team, I successfully identified a viable configuration and ran a Multinode Distributed Data Parallel job with PyTorch and MPI
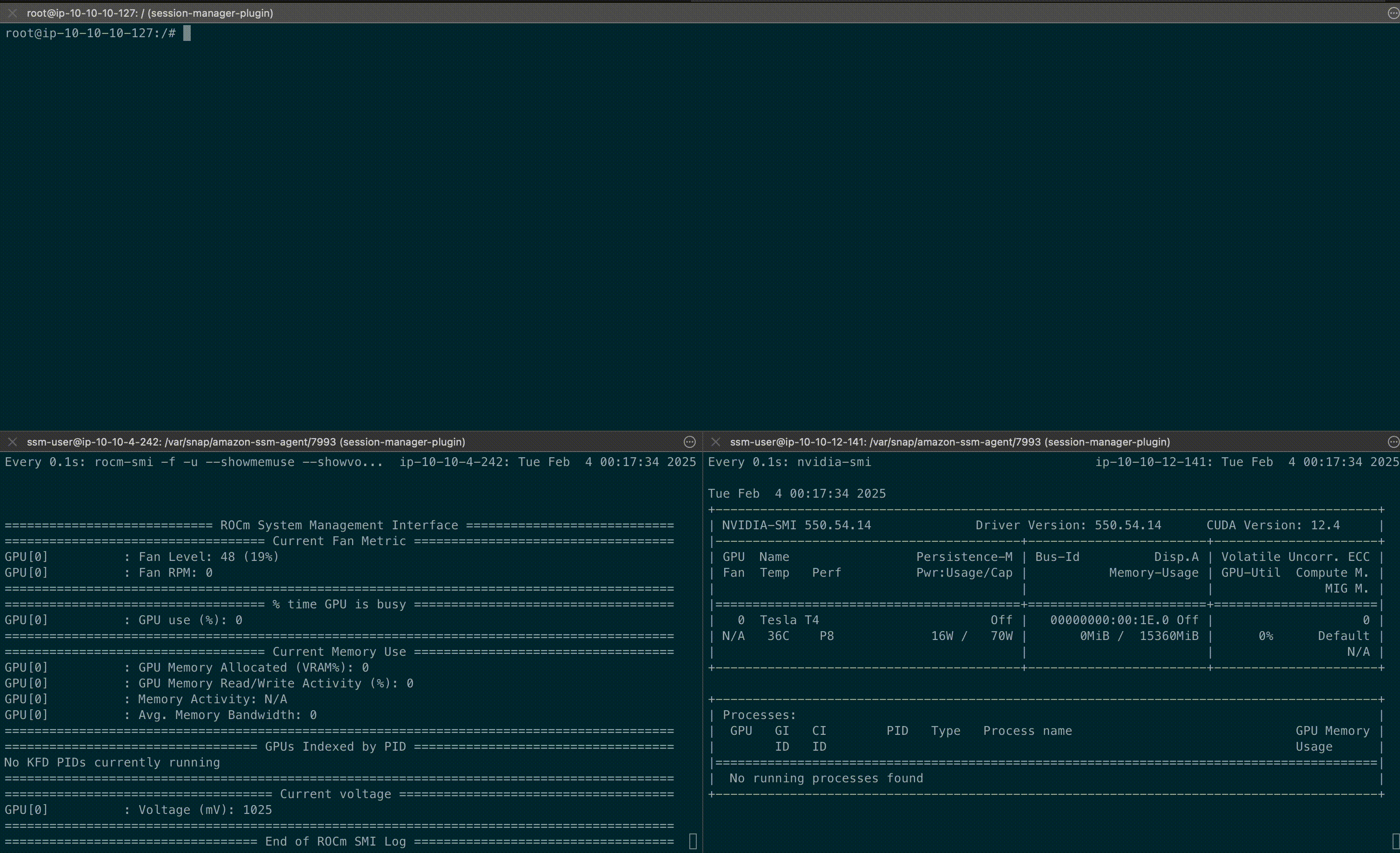
Distributed Data Parallel PyTorch Training job on AWS G4ad (AMD GPU) and G4dn (NVIDIA GPU) instances.
By using UCC and UCX, it appeared that mixed-GPU clusters aren’t a distant dream but an achievable reality. This breakthrough could trigger organizations to unshackle their hardware investments, turning fragmented resources into cohesive supercomputers.
All low-level details on environment configuration and the research and testing process are documented here:
GitHub - RafalSiwek/troubleshoot-heterogenous-distributed-operations: A repo describing my…
A repo describing my struggles to run distributed training on a heterogenous cluster …
Enabling Heterogenous Kubernetes Clusters
When managing enterprise-scale infrastructure, organizations face the challenge of supporting teams with the required resources. They also require enabling fast and efficient machine learning workloads at any scale, including small experiments and long-lasting training jobs of trillion-parameter-size models.
Kubernetes becomes a cornerstone for distributed machine learning for orchestration and its ability to maximize resource utilization and harmonize diverse hardware.
To schedule distributed training jobs on Kubernetes, regardless of using the Kubeflow MPI Operator or Pytorch operator, the job manifest requires using either the AMD or NVIDIA device plugin-provided resource:
# NVIDIAresources: limits: nvidia.com/gpu: 1
# AMDresources: limits: amd.com/gpu: 1
Specifying a strongly heterogeneous job would require either a CRD or a mutating webhook handler that would unify the resources with a general name like heterogenous.com/gpu: 1 or manually deploy each pod separately.
VolcanoJob, a Kubernetes CRD provided by the Volcano scheduler, simplifies this process. Volcano is designed for high-performance batch workloads, offering gang scheduling to ensure atomic execution of distributed tasks (i.e., all pods start when all required allocation resources are available or none do) and plugins to automate infrastructure setup. Unlike Kubeflow’s Training Operators (e.g., MPIOperator), which enforce uniform resource templates across workers, Volcano allows per-task pod definitions, enabling precise control over heterogeneous resources.
To launch a mixed GPU distributed training workload on a heterogenous Kubernetes cluster, the following VolcanoJob CRD features have to be configured:
- automated SSH configurationThe ssh plugin generates a ConfigMap with pre-shared SSH keys, enabling passwordless authentication between pods. The sshd setup in each container leverages these keys, eliminating manual certificate management.
- worker pod DNS resolutionThe svc plugin creates a headless service, assigning predictable DNS hostnames. Pods resolve peers via Volcano-injected environment variables (e.g., VC_MPIWORKER_AMD_HOSTS), which the master pod uses to construct the mpirun hostlist.
- resource-specific task groupsEach task defines a unique pod template:— The mpimaster orchestrates training, using MPI and UCX flags to optimize GPU communication.— mpiworker-nvidia and mpiworker-amd specify distinct resources and vendor-specific container images.
- gang schedulingminAvailable: 3 ensures all pods (1 master + 2 workers) are scheduled together, preventing resource deadlocks in mixed clusters.
- job completion definitionpolicies field with the CompleteJob action key allows configuring the event that puts the job into the completion state. In this case, it is the mpimaster task TaskCompleted event.
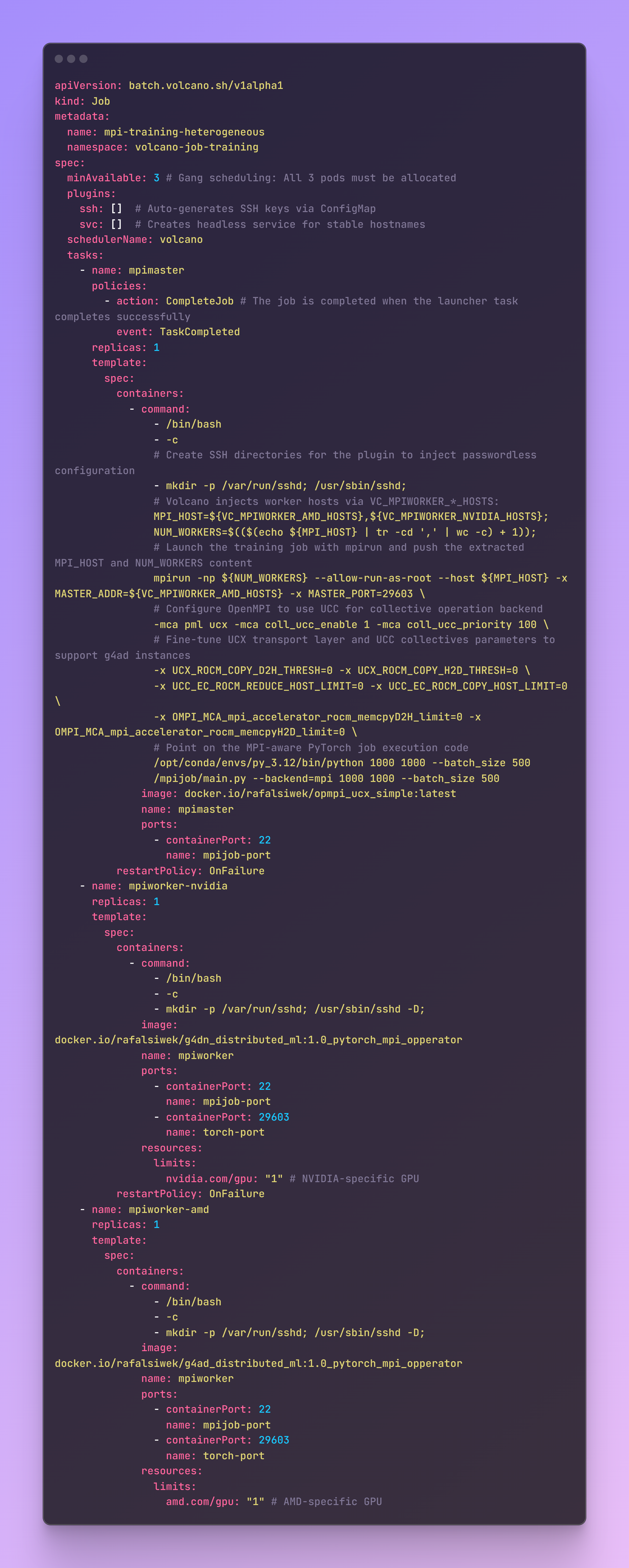
Running PyTorch distributed jobs requires worker environments with GPU-type aware UCC, UCX and MPI libraries with PyTorch build that links its distributed module to these libraries. The launcher only requires UCC, UCX, and OpenMPI, however since its collective operations do not involve GPU-specific processing, they do not have to be built with any GPU-awareness. For this setup, the libraries and PyTorch must be built from source. Dockerfile recipes are provided in my repository. Additionally, pre-built images for specific worker instances are available on my public DockerHub registry:
By enabling mixed-GPU clusters on Kubernetes, organizations could transform fragmented hardware into a unified force for innovation. This approach eliminates costly vendor lock-in, maximizes existing investments and increases GPU utilization. Whether scaling trillion-parameter models or juggling mergers with disparate infrastructures, heterogeneous setups empower teams to train faster and smarter — without overhauling hardware.
Limitations
- Lack of RDMA Validation: Due to AWS EFA’s unsupported status for g4ad instances, proper RDMA compatibility remains untested. UCX also lacks official AWS EFA compatibility for zero-copy RDMA operations, necessitating reliance on TCP.
- Suboptimal Communication Performance: The sole use of the TCP transport layer significantly reduces communication bandwidth and latency, as evidenced by OSU benchmark results (see here).
- ML Framework Integration Requirements: While PyTorch and Horovod support MPI backends for collective operations, Horovod remains untested in this implementation. Furthermore, most frameworks require explicit MPI backend integration, which is not universally available.
- Limited MPI Backend Support in PyTorch: PyTorch’s MPI-flavored collective backend is narrowly scoped, prioritizing NCCL/Gloo backends and supporting only Distributed Data Parallel (DDP). Advanced strategies like Fully Sharded Data Parallel (FSDP) rely on operations such as allgather_base, which remain unimplemented in PyTorch’s MPI backend.
Conclusion
The ability to execute distributed training on multi-vendor GPU clusters presents a compelling opportunity for organizations seeking rapid scalability in ML/DL workloads. However, achieving this today demands significant engineering effort due to a lack of native support in mainstream ML frameworks.
The development of open, standardized implementations could democratize access to heterogeneous hardware ecosystems, enabling cost-effective flexibility without compromising performance.
...
Popular
Dive in
Related
Blog
DISTRIBUTED TRAINING IN MLOPS: Accelerate MLOps with Distributed Computing for Scalable Machine Learning
By Rafał Siwek • Mar 11th, 2025 • Views 185
26:35
video
Challenges in Accelerated Training and Deployment for Complex Tabular Models
Aug 23rd, 2022 • Views 939
Blog
Distributed Training in MLOps: How to Efficiently Use GPUs for Distributed Machine Learning in MLOps
By Rafał Siwek • Mar 19th, 2025 • Views 243
Blog
DISTRIBUTED TRAINING IN MLOPS: Accelerate MLOps with Distributed Computing for Scalable Machine Learning
By Rafał Siwek • Mar 11th, 2025 • Views 185
Blog
Distributed Training in MLOps: How to Efficiently Use GPUs for Distributed Machine Learning in MLOps
By Rafał Siwek • Mar 19th, 2025 • Views 243
26:35
video
Challenges in Accelerated Training and Deployment for Complex Tabular Models
Aug 23rd, 2022 • Views 939