Model Performance Management Done Right: Build Responsibly Using Explainable AI
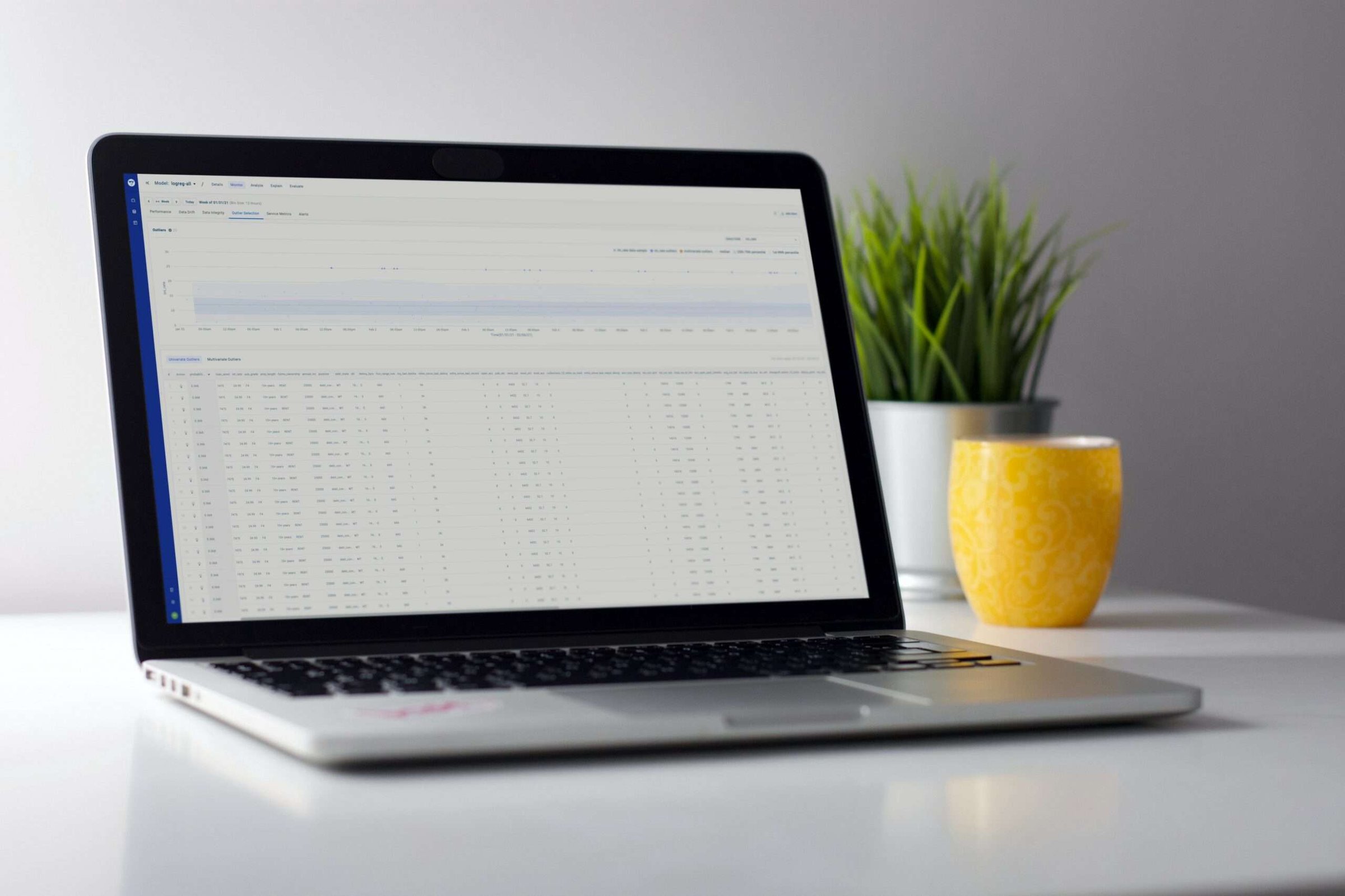
Should I build Responsible AI? The emergence of artificial intelligence (AI) and machine learning (ML) has brought great benefits across different industries, from automating simple robotic tasks to more complex autonomous driving
June 25, 2021

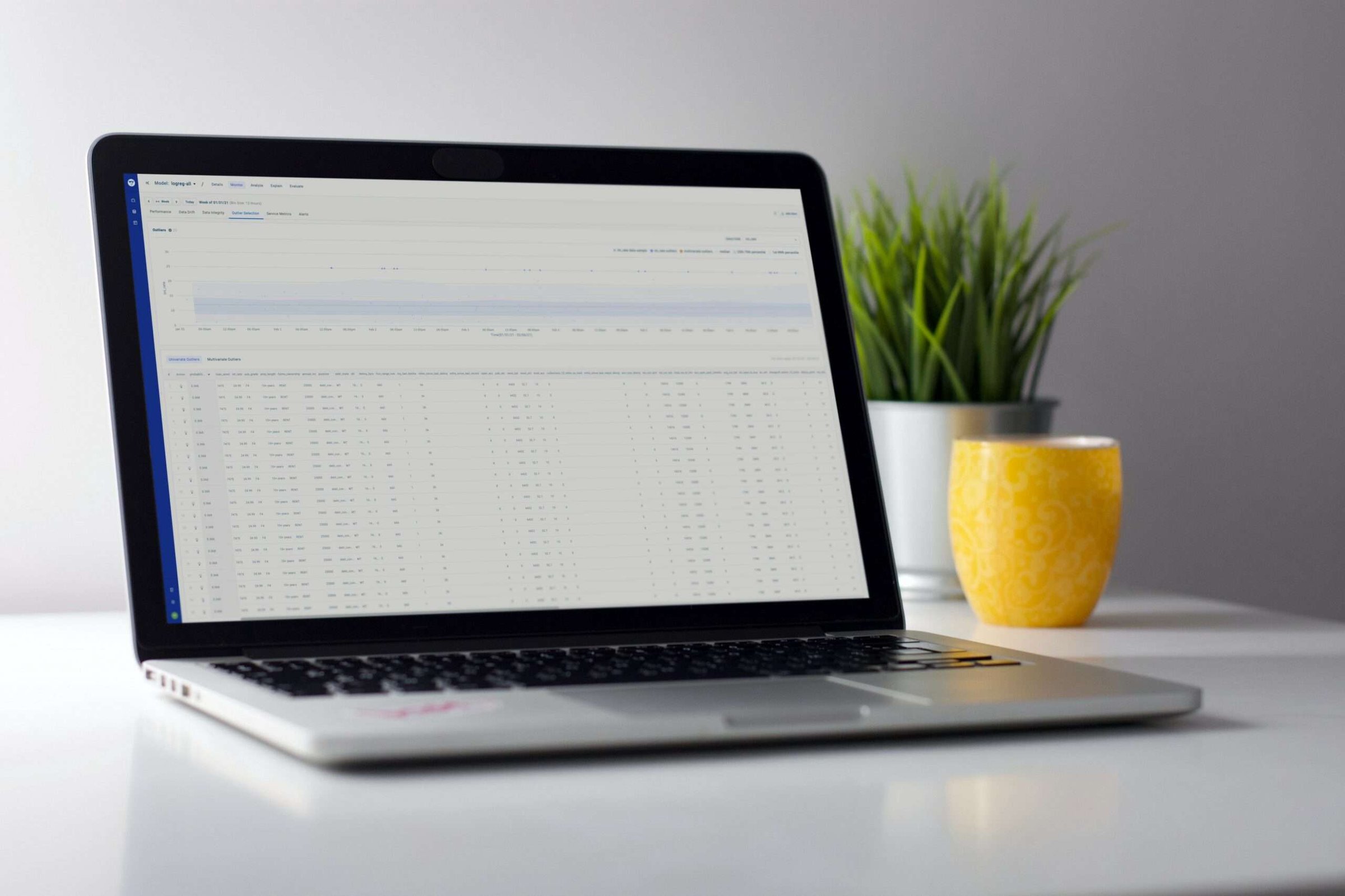
Should I build Responsible AI?
The emergence of artificial intelligence (AI) and machine learning (ML) has brought great benefits across different industries, from automating simple robotic tasks to more complex autonomous driving. Some use cases we’ve seen are credit card fraud detection for financial institutions, sales lead scoring for both B2B and B2C companies, and job matching for HR teams. However, as more AI applications were put into practice, various problems arose due to the nature of AI being hard to interpret or being a black box. For example, Amazon’s AI recruiting tool favored men over women due to the biased resume samples and this was discovered by an employee who got suspicious after a year. Another well-known example is Apple Card’s discrimination case that was revealed by a software engineer who questioned the different credit limits on his and his wife’s cards.
Unfortunately, these examples scare people, including customers. Because AI is making decisions that put some groups at disadvantage, the media and general public view AI as a super-intelligent being that cannot be trusted and turn away from benefits. How do we turn this aspect around? According to Accenture’s report on Responsible AI, Federal agency leaders get these three questions the most:
- How do I ensure that AI solutions are designed to operate responsibly?
- How do I employ AI to act in a manner that is compliant with stakeholder expectations and applicable laws?
- How do I use AI to unlock the full potential of my workforce?
While the report points out government officials, any business leader with AI initiatives would ultimately have to answer the same questions. But what does it mean to build “responsible” AI?
There are different approaches to building “responsible” AI. One way is to focus on risk management to only take responsible actions. However, there is another method that is applicable to broader practice: utilizing Explainable AI, or XAI, in Model Performance Management (MPM).
Why use XAI to build Responsible AI?
Some people might ask, “Where does Explainable AI fit it to building responsible AI?” The answer is, “Everywhere.” Once XAI is incorporated into MPM, it adds numerous advantages. Let’s go over some of the benefits.
Trust and Transparency
The most apparent reason is the transparency provided by XAI and the trust that can be built with stakeholders. By showing how the model was built and is making predictions, stakeholders can better understand AI. For example, XAI allows regulators and auditors to verify that the models are operating under the required laws. The transparency and trust can go further and help train non-technical people to use more AI applications throughout the organization, increasing efficiency and productivity.
Increased Productivity with Efficient and Faster Debugging
The gain in efficiency and productivity also applies to technical members at an organization. As more models are put into production and used in making business decisions, being able to diagnose and debug models are becoming more important. Especially for those doing business in real-time, finding a performance drop and finding the reason for the decrease can be a daunting task. Was there a data drift? Is it a data integrity issue? With so many places to look, XAI can assist the team to narrow down the scope — finding the cause of the drop and related features — and minimize the loss that happens with the troubleshooting time.
The benefit of fast troubleshooting is an enormous productivity gain but there’s one more. As with any business that handles ever-changing data, an ML model will decay at some point and require the team to retrain the model with an updated dataset. Unfortunately, the timing to retrain has traditionally been very reactive. However, with MPM providing 360-degree observability with XAI, a data scientist or a machine learning engineer will be able to proactively find model performance drop due to decay and retrain the model to maximize the performance and business ROI, rather than trying to minimize losses.
Effective Collaboration and Accountability
The associated benefit of knowing the cause of the problem with Explainable Monitoring is a dramatic increase in teamwork. With multiple teams working together on maintaining the ML models in production, communicating to the correct team or personnel reduces the overall debugging process. For instance, a real estate market and consumers change their behaviors constantly and datasets change overnight. In hindsight, a business user might upload a dataset with a new feature to the outdated AI application, get an inaccurate prediction, and offer irrelevant information to customers. With XAI working behind MPM, the team can catch such incidents in real-time, get the right stakeholders together, and assist everyone to find the most appropriate solution quickly.
Fairness
Fairness and bias aren’t just for regulated industries. More companies are looking into implementing more responsible AI due to customer demands. For example, an HR company expanded their data science team to develop better explanations for their job-candidate matching model because more candidates wanted a transparent fairness report. Since the job-candidate pool is constantly expanding, XAI and MPM together can effectively handle different use cases listed above, including fairness and bias, from model validation to production.
Model Performance Management powered by XAI fuels Responsible AI
As illustrated, implementing Explainable AI into Model Performance Management to monitor AI lifecycle enables an organization to become one step closer to building responsible AI. Businesses will gain complete visibility into how the models are performing in production and extract the full value of AI — improve your business process workflow, make better decisions for internal and external customers, reduce inefficiencies — by empowering the entire organization to build and use ethical AI applications in a trustworthy manner.