AI/ML Product Management
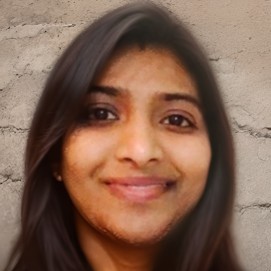
Amritha is an accomplished technology leader with over 12 years of experience spearheading product innovation and strategic initiatives at both large enterprises and rapid-growth startups. Leveraging her background in engineering, supply chain, and business, Amritha has led high-performing teams to deliver transformative solutions solving complex challenges. She has driven product road mapping, requirements analysis, system design, and launch execution for advanced platforms in domains like machine learning, logistics, and e-commerce.
Throughout her career, Amritha has been relied upon to envision the future, mobilize resources, and achieve business success through technology. She has been instrumental in helping shape product strategy across diverse sectors including retail, software, semiconductor manufacturing, and cloud services. Amritha excels at understanding diverse customer needs and leading data-driven efforts that maximize value delivery. Her passion and talents have led to her spearheading many greenfield projects taking concepts from ideation to national scale within aggressive timeframes.
With her balance of technical depth, business acumen, and bold leadership, Amritha is an invaluable asset ready to tackle dynamic challenges and capitalize on new opportunities. She is a principled, solutions-focused leader committed to empowering people, organizations, and ideas.
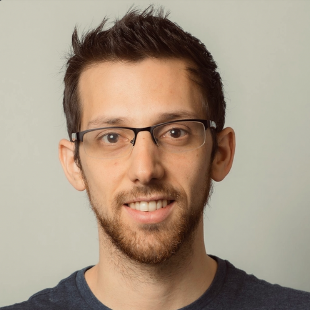
I'm a tech entrepreneur and I spent the last decade founding companies that drive societal change.
I am now building Deep Matter, a startup still in stealth mode...
I was most recently building Telepath, the world's most developer-friendly machine learning platform. Throughout my previous projects, I had learned that building machine learning powered applications is hard - especially hard when you don't have a background in data science. I believe that this is choking innovation, especially in industries that can't support large data teams.
For example, I previously co-founded Call Time AI, where we used Artificial Intelligence to assemble and study the largest database of political contributions. The company powered progressive campaigns from school board to the Presidency. As of October, 2020, we helped Democrats raise tens of millions of dollars. In April of 2021, we sold Call Time to Political Data Inc.. Our success, in large part, is due to our ability to productionize machine learning.
I believe that knowledge is unbounded, and that everything that is not forbidden by laws of nature is achievable, given the right knowledge. This holds immense promise for the future of intelligence and therefore for the future of well-being. I believe that the process of mining knowledge should be done honestly and responsibly, and that wielding it should be done with care. I co-founded Telepath to give more tools to more people to access more knowledge.
I'm fascinated by the relationship between technology, science and history. I graduated from UC Berkeley with degrees in Astrophysics and Classics and have published several papers on those topics. I was previously a researcher at the Getty Villa where I wrote about Ancient Greek math and at the Weizmann Institute, where I researched supernovae.
I currently live in New York City. I enjoy advising startups, thinking about how they can make for an excellent vehicle for addressing the Israeli-Palestinian conflict, and hearing from random folks who stumble on my LinkedIn profile. Reach out, friend!
Discuss how the role of product managers is evolving to incorporate AI/ML - Share insights from your experience as an AI/ML PM - Provide 3 key strategies for building impactful AI products - Explain how PMs need to expand skillsets to lead AI teams.
AI/ML Product Management
AI in Production
Slides: https://docs.google.com/presentation/d/1XJ8p2-501LaRJ3-A1QYtH7VnyKYY8wvR/edit?usp=drive_link
Adam Becker [00:00:05]: We have Amrita here. Amrita, are you with us?
Amritha Arun Babu [00:00:09]: Yes, I am.
Adam Becker [00:00:10]: Okay. Good to have you. Amrita has been a product leader at places like Amazon and Wayfair. You have screen to share with us here it is. Awesome. I'm going to let you have the stage and I'll show up soon. Thank you very much Amrita.
Amritha Arun Babu [00:00:30]: Hey everyone, I'm Amrita. I'm here to talk about how we can strategically determine which problems need an LLM investment. A quick introduction about myself. I have decade experience in building product solutions across companies like Amazon, Wayfair, Rubrik to name a few. I have built product solutions in data exploration, ingestion framework and as well as international supply chain platforms. Today, companies face a challenge of determining we have all these list of problems. Should we deploy LLM as a solution for all these problems? I face similar situation and I've come up with a framework that helped me determine what are the key factors that we need to keep in mind when making such investments. In this session, I'll quickly run down the framework and give you examples into how I have applied this framework.
Amritha Arun Babu [00:01:39]: So the key value framework or the LLM value framework that I call it as is basically around focusing on what is the accuracy that a particular problem requires when we build any solution there? And also can we try the impact of this solution to the key business metrics? I know when we are looking into designing a solution for any given problem, we talk about understanding what is the business problem here? Why are we solving this? What is the need to solve this? Currently those are still relevant. In addition to that, it would be great if we can apply an LLM focused framework wherein you determine this is the list of accuracy or this is the accuracy threshold that we need. And here is how I would tie the impact driven by LLM to my business need and also dive into understanding what is the infrastructure cost that I would incur to either build the LLM? Do I have all the data that I need? And what does the LLM infrastructure look like? Whether to build, integrate with the LLM or integrate with my applications? One of the use cases that I use this framework is about how do we get PIi information? And I think all of you can relate to this because every organization faces this challenge that we do not want the customer PIi information to be shared downstream. Let's say you are a product manager or you're a leader who is solving problems about. Let's say you want to build a music recommendation system for your customers. And in order to do this effectively, you're going to interact or share your data sets with the third party playlist here. You do not want to share your customer name, location or any other PIi information. Then in this scenario you would need a highly accurate model and you would want to measure the accuracy, that is the precision or recall metrics of the model tied to our customers churning out because of privacy issues.
Amritha Arun Babu [00:03:57]: How many scenarios do I have where we have violated the customer privacy, so on and so forth. Those are some of the ways that you would measure. And then do I have access to clean data? In past when I worked in this scenario, we had both structured and unstructured data. So we spent a lot of time getting access to the unstructured data, had to spend a lot of time transforming the data, and incurred a lot of costs due to that. But we also had a well established ML platform where we could deploy the model. And then in this scenario we were able to determine that yes, LLM is the right solution and we were able to achieve about 87 plus percent north of that precision. And this was mainly to gate name Pii. And we did this by training a model for a name gazette.
Amritha Arun Babu [00:04:56]: So that is one of the use cases that I would like to share. The other use case where I encountered similar situation is about automating our contract negotiation. So if you are in any part of any vendor management teams, you are well aware that you would have to negotiate periodically, like contracts with your suppliers, with your vendors. So often negotiating contracts is a long drawn process because you would have to understand market dynamics, understand what are the products, how is the supplier or the vendor support here, and what are the levers that you have that based on your previous contracts or if this is a new contract, how would you navigate this negotiation deal? So in those scenarios, it is because of the time constraint and because of the legalities, it's extremely important. Any solution that we built has quite high accuracy and it's always very hard to tie a solution that you build to a metrics which says that, okay, here is the NLM that gives the x input, but what did it reduce into? Did it reduce my time to negotiate or did it reduce inability to churn out quicker contracts, smarter contracts? What did it lead to? That's hard to determine. And also with respect to data and infrastructure, there's always a requirement of quite diverse data. And again, this goes much deeper into like as you start dealing with country jurisdictions and any local legal data that you need. So in this scenario, we decided that it's not right, at least for now, to build an LLM solution.
Amritha Arun Babu [00:06:45]: Rather we went down the path of building a standardized contract template system and that set foundation to build a consistency around how does our contracts look like so that we can eventually build contract or negotiation assistant AI that enables our vendor management team to get insights on, let's say one of the countries that we operate in and then based on that we would improve or extend it to other countries. So that's the thought we enabled here. Just to recap. So yes, every one of us is eager to dive into and harness the potential of llms, but at the same time, it is very crucial now to make sure is that the right solutions. And we could do that by determining what is the accuracy needed for the particular scenario, what is the business metrics that I'm going to tie, and how am I going to measure the performance of LLM and tie to the business metrics? And lastly, what is the infrastructure set up or the cost? And is it worth it, and will that give the return on investment that I'm expecting? Thank you.
Adam Becker [00:08:10]: See, why am I here? Okay, Amrita, thank you very much. It's refreshing and I feel probably very frustrating for some people that really want to use llms for certain purposes, come out of this framework recognizing that maybe that is not the best tool for the job or maybe just this type of problem. It doesn't lend itself to an LLM solution quite yet. It feels like you probably have to manage people's expectations and feelings, and it's not that easy to use a framework like this. But at least if you turn it into a framework, it's not just one person's hunch, right? It's a little bit more robust.
Amritha Arun Babu [00:08:51]: Agree? Yeah, it provides a structured thought process. This is not like a magic bullet, but it definitely adds to it saying that hey, we understand these are the parameters against which we are evaluating our solution, in addition to any nuances that comes with that particular problem, that you're saltly 100%.
Adam Becker [00:09:12]: Amrita, thank you very much.
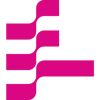