Where's Your Pre-registration: A Physicist's Notes from the Cheap Seats on AI's Benchmarking Crisis
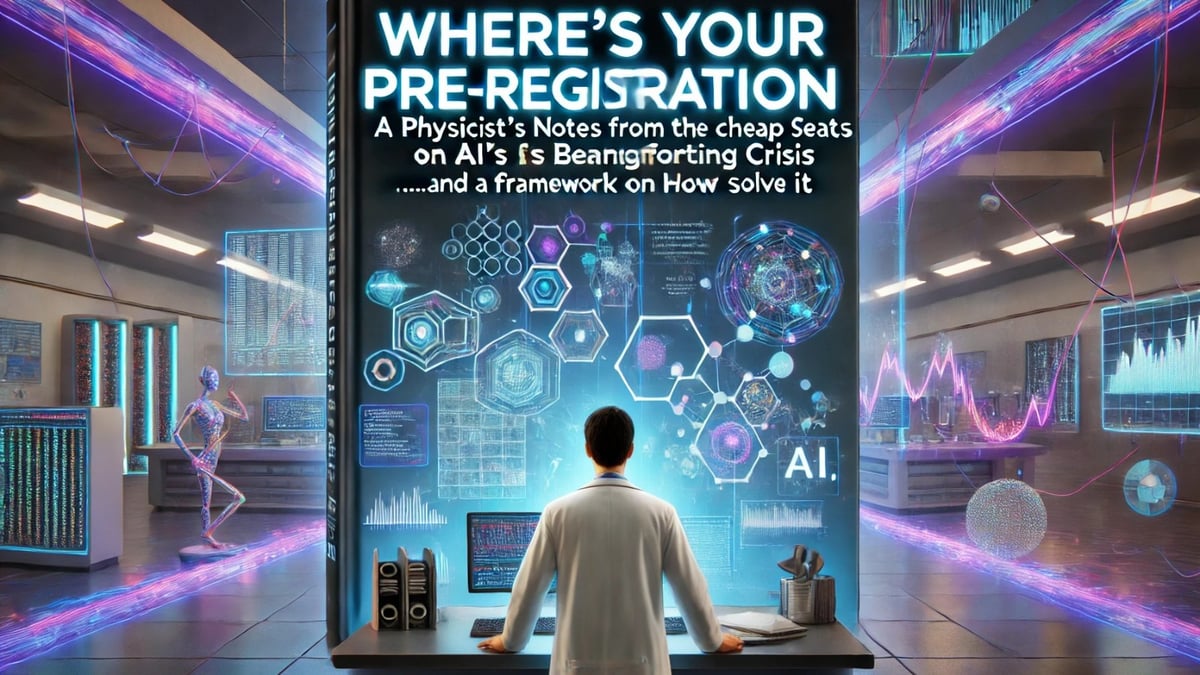
...and a framework on how to solve it
March 18, 2025
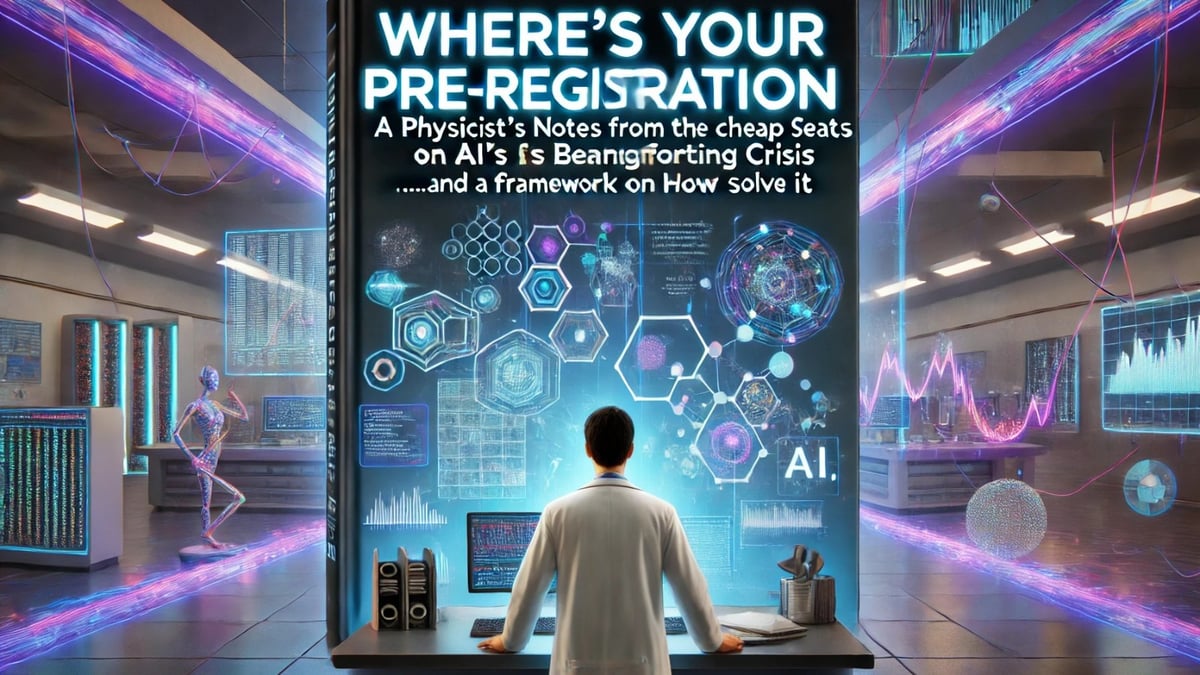
When Physics Meets AI: A Crisis of Methodology
The announcement of the ARC Prize Foundation landed in my inbox like a well-intentioned stone in a methodological glass house. François Chollet* et. al. launched a nonprofit to develop benchmarks for "human-level" intelligence, complete with puzzle-based tests and a coalition of frontier AI labs [1]. It should be exciting news (and to some extent it is!). But it also triggered an uncomfortable realization about the state of AI evaluation which is the topic of this rant post.
You see, a peculiar thing happens when you've spent years in physics before wandering into AI research: you start noticing the gaping holes where scientific rigor should be. The contrast is stark enough to make a physicist's hair stand on end – and not just from the static electricity in the particle accelerator room.
In high-energy physics, before anyone turns on the accelerator, researchers spend years writing detailed papers outlining exactly what they expect to see and what would constitute a discovery. When CERN was hunting for the Higgs boson didn’t just say “we’ll know it when we see it bruh” but specified the exact statistical significance required to claim detection.
The Three Body Problem of AI: Benchmarks, Contamination, and Generalization
Now contrast this with how we evaluate large language models. We create benchmarks with all the permanence of sandcastles at high tide. As soon as a model performs well on a benchmark, we declare it "solved" and promptly move on to creating harder ones. It's as if we're playing a never-ending game of whack-a-mole, except we're not quite sure what we're whacking or why. I completely agree that measuring general intelligence presents unique challenges compared to physics experiments - like trying to measure quantum states while wearing boxing gloves. But that's precisely why we need more rigor, not less. The journey must begin with a clear definition of what we're measuring and why it matters. Instead, we're caught in an endless cycle of buzzword bingo. Today's AGI becomes tomorrow's ASI, which transforms into next week's AMI, while we're all pretending these alphabet soup terms actually mean something concrete. It's like renaming a particle every time it doesn't fit our theories, rather than admitting we might need better theories.
This doesn't just muddy the waters - it fundamentally undermines our ability to measure actual progress in AI capabilities. When GPT-4o crushes a benchmark that stumped GPT-4, what have we actually learned? That newer models are better at taking tests? That throwing more compute at the problem helps? We're collecting scores without accumulating understanding and our models are quickly becoming star students who are becoming great at taking tests but unable to come up with any real innovative solutions.
Additionally, there is another elephant in the room: test set contamination. Remember when we used to joke about students memorizing past exam papers? Well, our language models are doing exactly that, except on an internet scale. With models training on increasingly larger swaths of the internet, our carefully crafted test sets are likely already part of their training data. We're essentially giving open-book exams to models that have photocopied the entire library.
The traditional response to test set contamination has been to create new benchmarks. But this creates a perpetual cycle: create benchmark, watch it get "solved" (or contaminated), create new benchmark. Rinse and repeat. We're running on a treadmill and calling it progress.
The Generalization Challenge: Beyond Memorization
What we really need is a rigorous framework for measuring out-of-sample generalization - the holy grail of learning both human and machine. The fundamental question isn't just whether a model can solve a problem, but whether it truly understands the underlying principles enough to apply them to novel situations. It's the difference between a student who memorized every physics formula and one who can solve never-before-seen problems by understanding the core concepts.
There are two potential paths forward here, and frankly, we need to pursue both:
- Developing methods to probe model behavior on novel inputs—much like how we observe increased glucose consumption when human brains encounter unfamiliar problems versus routine ones. By measuring activation patterns in our models, we might find similar signatures of genuine learning versus mere recall.
- Pushing for training data transparency, though this seems as likely as finding a massless electron in today's competitive AI landscape. While CERN freely shares every detail of their particle collisions, most AI labs guard their training data like dragons hoarding gold.
(If you're working on either of these problems, or have ideas about how to crack them, please reach out—my inbox is ready for your mathematical derivations and late-night research proposals.)
This isn't just an academic exercise. Without solving the generalization problem, we're essentially building increasingly sophisticated pattern matchers while convincing ourselves we're measuring intelligence. And unlike physics, where we can isolate variables in controlled experiments, we're trying to measure something far messier - the ability to learn and adapt to truly novel situations.
The Standard Model of AI Evaluation: Towards a Unified Theory
Let's stop playing whack-a-mole with benchmarks and build something lasting. We need a framework as robust as the Standard Model in physics—one that brings together multiple disciplines and perspectives. Here's what that looks like:
At the foundation lies basic language understanding—sentiment analysis, entity recognition, the building blocks. But we need cognitive scientists to ensure these metrics reflect genuine human language development, not just statistical patterns. Think of it like measuring a particle's properties: we need to know what we're actually observing.
Above this sits contextual reasoning—the quantum mechanics of language, if you will. Here's where we need philosophers of mind working alongside AI researchers. When a model maintains context across a thousand tokens, are we seeing genuine comprehension or just really good prediction? The distinction matters.
At the apex: generalization and knowledge transfer. This is our unified field theory—measuring how well models can leap from training to novel applications. We need evaluation experts to develop metrics as precise as those used to confirm the Higgs boson.
But perhaps the most exciting insight comes from cognitive architecture researchers: these capabilities might not develop hierarchically at all. Like quantum entanglement, improvements in one area could mysteriously enhance another. Creative generation might boost abstract reasoning, or vice versa.
The Implementation Challenge:
- Cognitive scientists: Map the territory between memorization and understanding
- AI researchers: Design contamination-resistant evaluation methods
- Philosophers: Define markers of genuine comprehension
- Evaluation experts: Quantify the "distance" between training and novel application
The fundamental shift? Moving from difficulty-driven to hypothesis-driven benchmark design. Instead of asking "What's a harder test?" we ask "What specific capability are we measuring, and how do we measure it with better accuracy?" To do this we need to understand the true invariants of learning and problem solving.
From Theory to Experiment: Making It Real
Had these principles been in place, the recent developments in AI evaluation would look very different. Instead of chasing benchmark scores, we'd be building cumulative scientific understanding. The ARC-AGI situation perfectly illustrates why we need this change: When OpenAI's unreleased o3 model achieved a qualifying score by throwing massive compute at the problem - after specifically training for these types of puzzles - what did we actually learn? That with enough computational brute force and targeted training data, we can solve pattern-matching puzzles? If we're really testing for general intelligence, shouldn't the model be able to handle these challenges without specialized training? Color me unimpressed. The fact that the same model might drop to under 30% on the next iteration while humans maintain 95% accuracy without any specific preparation should be a wake-up call to anyone claiming we're approaching AGI.
The Final Test: A Call to Scientific Rigor
Here's my challenge to benchmark developers and every major AI lab: Before you release another benchmark or claim another breakthrough, show us your pre-registered hypotheses. Tell us exactly what you expect to learn from your evaluations. Explain how you'll prevent data contamination. Define what success means before you start testing, not after you get results you like.
Because yes, this will be harder than our current approach. It will require more upfront work, more careful thought about what we're actually measuring, and more discipline in sticking to pre-defined evaluation criteria. But if we're serious about understanding AI progress - and not just generating impressive-looking numbers - it's work we need to do.
After all, if we're building systems that might fundamentally transform human society, shouldn't we be at least as rigorous in evaluating them as we are in testing new particles? Or are we content to keep playing colored-square puzzles while pretending we're measuring intelligence?
Originally posted at: