Why Shadow AI Hurts Your Ability to Scale Production-Ready AI Models
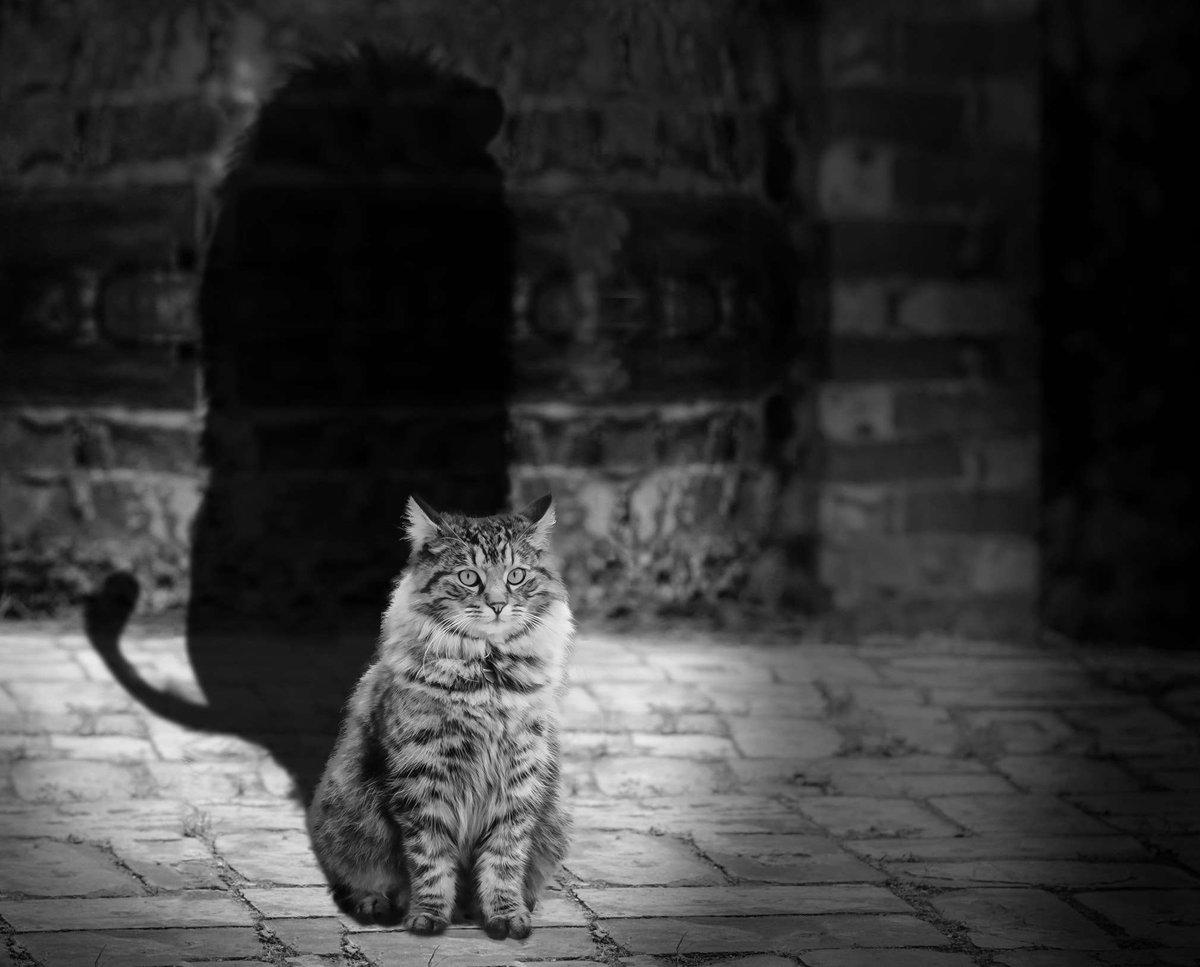
Run:ai’s 2021 State of AI Infrastructure Survey revealed that 38% of respondents are spending over $1M a year on AI infrastructure (hardware, software, and cloud fees), with 74% of respondents saying that they will increase that spending next year
October 28, 2022

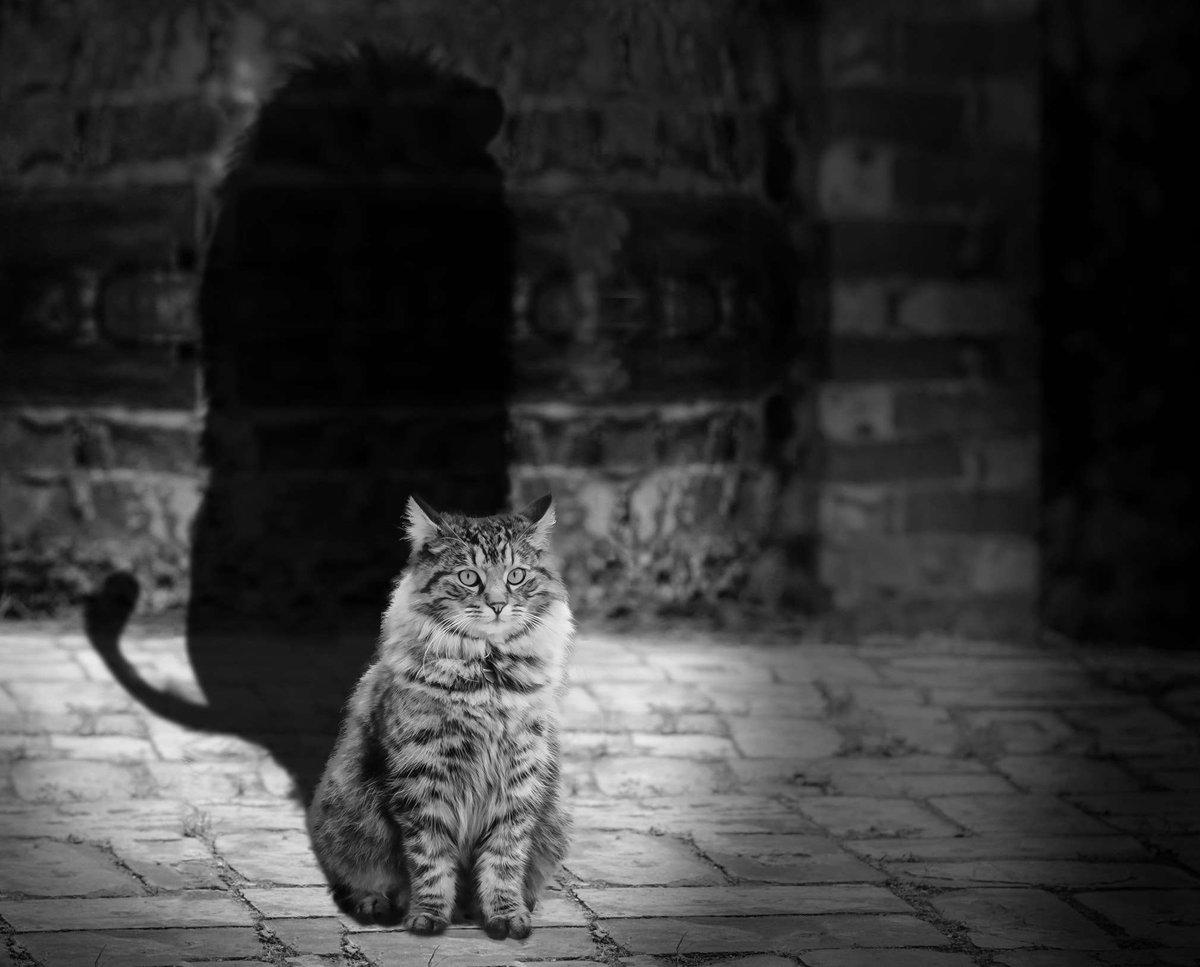
Run:ai’s 2021 State of AI Infrastructure Survey revealed that 38% of respondents are spending over $1M a year on AI infrastructure (hardware, software, and cloud fees), with 74% of respondents saying that they will increase that spending next year.
The increased AI infrastructure spend is giving rise to “Shadow AI,” a term that describes building AI infrastructure and tooling without IT’s input. In the short term, AI and IT teams may succeed with a decentralized approach, especially as they get started with building new models, and have fewer data scientists and AI teams. In the long term, Shadow AI is likely to lead to the failure of building the infrastructure required to scale.
by Ariel Navon
The 4 Problems with Shadow AI
Shadow AI has many drawbacks for all AI stakeholders (IT, data science, and business leaders), including:
- Lack of visibility, control, and access to compute resources. In the survey, 35% of respondents stated that they do not have access to on-demand GPU compute, and 43% require manual requests to gain access to GPU compute. It is common for IT teams to be in the dark about GPU usage unless someone updates a spreadsheet or submits a ticket. AI and research teams often have to sit and wait for access to GPUs. The lack of visibility, control, and access leads to a slow-down in building new models and a constant experience of frustration and delay.
- Inability to align AI infrastructure to business goals. As many business leaders can attest, it’s not just IT and the data scientists that suffer. The lack of access and productivity has a massive impact on the business. If IT can’t set priorities around which teams, users, and projects get access to GPUs, companies can end up focusing on low-impact initiatives.
- Inefficient use of current AI infrastructure spend. Shadow AI exacerbates the expensive growth of AI infrastructure spend, as 83% of companies admit to idle GPUs or only moderate utilization. As AI needs scale, the increased demand stretches existing siloed infrastructure capabilities. Without the ability to see usage across teams and departments, IT is forced to either spend more or limit a team’s access to compute resources, which impacts their ability to train newer models and push them to production.
- Added IT management overhead. When each team has its own tech stack, there’s a lack of standards across the organization, as well as process inefficiencies and waste. For example, if multiple teams research various AI tools, there is redundant work being done. And IT is forced to monitor and support a variety of AI tools and services —each with their own access, administrative, and process workflows.
The bottom line is that organizations can’t rely on shadow AI to bring production-ready AI models to market. To scale, IT needs to centralize their management of AI infrastructure.
Accelerate ROI and Scale Production-Ready Models with Run:ai
Run:ai enables IT teams to accelerate ROI by enabling the business to scale production-ready models. There are many benefits for all stakeholders when centralizing AI infrastructure with Run:ai:
- Increase efficiency and performance of AI infrastructure resources. Run:ai pools all compute resources and optimizes GPU allocation and performance. With Run:ai’s unique GPU abstraction capabilities, organizations can “virtualize” all available GPU resources and ensure that users can easily access GPU fractions, multiple GPUs, or clusters of GPUs which drastically reduces the percentage of idle GPUs and enables a wider range of access to compute resources.
- Accelerate time-to-market for different AI models. Run:ai helps MLOps and AI Engineering teams to quickly operationalize AI pipelines at scale. It also allows them to run production machine learning models anywhere while using the built-in ML toolset or simply integrating their existing third-party toolset (MLflow, KubeFlow etc).
- Create an on-demand and flexible GPU-as-a-service. Run:ai allows for efficient and automated management of these resources and enables IT departments to deliver AI-as-a-service. The platform offers a simple way for IT and researchers to interact with it using built-in integration for IDE tools like Jupyter Notebook and PyCharm. They can easily start experiments and run hundreds of training jobs without ever worrying about the underlying infrastructure.
- Unify visibility and unlock insights for AI infrastructure. Run:ai visualizes every aspect of the AI journey, from infrastructure to model performance, giving every user insights into the health and performance of AI workloads. These dashboards allow IT to have the visibility needed to set policies around which AI teams, users, stages, and projects get access to GPUs. This ensures that organizations remain focused on projects with higher business impact.
If you’re an organization increasing AI infrastructure spend and placing your business goals at risk with shadow AI, learn how to centralize your AI infrastructure with Run:ai today.