Sign in or Join the community to continue
10 Types of Features your Location ML Model is Missing
Posted Oct 07, 2021 | Views 525
Share
speaker
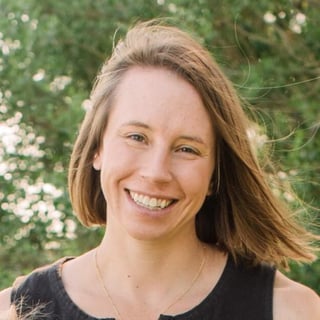
Anne Cocos
Director of Data Science @ Ask Iggy
+ Read More
SUMMARY
+ Read More
Watch More
Tour of Upcoming Features on the Hugging Face Model Hub
Posted Jul 26, 2021 | Views 563
# Hugging Face
# Huggingface.co
How to Systematically Test and Evaluate Your LLMs Apps
Posted Oct 18, 2024 | Views 14.1K
# LLMs
# Engineering best practices
# Comet ML
Building LLM Applications for Production
Posted Jun 20, 2023 | Views 10.7K
# LLM in Production
# LLMs
# Claypot AI
# Redis.io
# Gantry.io
# Predibase.com
# Humanloop.com
# Anyscale.com
# Zilliz.com
# Arize.com
# Nvidia.com
# TrueFoundry.com
# Premai.io
# Continual.ai
# Argilla.io
# Genesiscloud.com
# Rungalileo.io