A Decade of AI Safety and Trust

Co-founder & CEO at LatticeFlow AI, building the world's first product enabling organizations to build performant, safe, and trustworthy AI systems.
Before starting LatticeFlow AI, Petar was a senior researcher at ETH Zurich working on the security and reliability of modern systems, including deep learning models, smart contracts, and programmable networks.
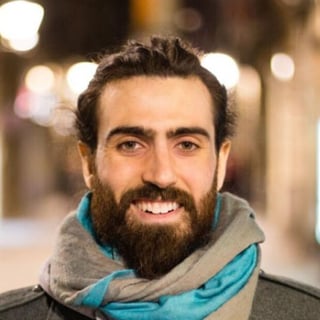
At the moment Demetrios is immersing himself in Machine Learning by interviewing experts from around the world in the weekly MLOps.community meetups. Demetrios is constantly learning and engaging in new activities to get uncomfortable and learn from his mistakes. He tries to bring creativity into every aspect of his life, whether that be analyzing the best paths forward, overcoming obstacles, or building lego houses with his daughter.
Embark on a decade-long journey of AI safety and trust. This conversation delves into key areas such as the transition towards more adversarial environments, the challenges in model robustness and data relevance, and the necessity of third-party assessments in the face of companies' reluctance to share data. It further covers current shifts in AI trends, emphasizing problems associated with biases, errors, and lack of transparency, particularly in generative AI and third-party models. This episode explores the origins and mission of LatticeFlow AI to provide trusty solutions for new AI applications, encompassing their participation in safety competitions and their focus on proving the properties of neural networks. The profound conversation concludes by touching upon the importance of data quality, robustness checks, application of emerging standards like ISO 5259 and ISO 40001, and a peek into the future of AI regulation and certifications. Safe to say, it's a must-listen for anyone passionate about trust and safety in AI.
Petar Tsankov [00:00:00]: Hi, my name is Petar Tsankov. I'm the CEO and Co-founder of LatticeFlow AI. And I drink my coffee, so it's not a special kind of coffee. I drink espresso, but it's interesting how I drink it. So I always get up and drink my first coffee very fast. Think of it like, you know, two minutes, and then immediately, then jump on the second one. So that's how people recognize me of the way I drink my coffee.
Demetrios [00:00:29]: What is up, mlops community? This is your host for the next hour. I'll be taking you on a little bit of a journey. My name is Demetrios, and it is a pleasure to be here with you, talking with Petar today about all of the things that go into AI trust. Wow, what a deep dive. And I've got a few key takeaways before we get into this conversation that I want to bring up and highlight for you so that hopefully they stick in your mind as we cruise on by them in the conversation. The first one is how AI research has traditionally been about optimizing one metric, and that metric has been accuracy. But now, when we take these models into production, there's so many wrenches that can get thrown into the system and so many X factors that we need to be cognizant of. And that is what Peter has been thinking about day in, day out, how to really make your system bulletproof, make it robust, make it all that it can be.
Demetrios [00:01:37]: I want to reference a song right now, but I'm not going to. The other thing that I will bring up as a highlight that I felt like was pretty spot on was how you cannot decouple models from data. It is almost like a trope that we've gone over time and time again, but your model is only as good as its data. And he was speaking about how, in LatticeFlow, the product that he has built. They are very interested in everything that happens upstream from the model because you're not able to create the best systems without being able to go upstream and make sure that the data is solid. So he had this really cool feature that he spoke about where you can see the model blind spots so that you can create a more robust system. And with LatticeFlow, you can test which data it just does horribly with my model, basically, how can I figure out where my model is doing bad so then I can go back upstream and give that model what it needs when it comes to data, so that it won't whiff it so hard? This was an awesome conversation. We got into all things trust and AI.
Demetrios [00:03:11]: Trust, I think, is the word of the day. And I really appreciate LatticeFlow coming and being a huge supporter of the community. If you are interested in this conversation, hit up Petar. And as always, if you like it, share it with just one friend or leave us a review. It would mean the world to me. Let's get into conversation now. We have to start with the fact.
Petar Tsankov [00:03:45]: That.
Demetrios [00:03:48]: The last time we spoke, you were in Switzerland. Now I think you're not in Switzerland.
Petar Tsankov [00:03:52]: That's correct, yes. Well, we started expanding a little bit. So we did recently open a second office in Sofia, which is Sofia in Bulgaria, which is where I'm from. And it was not a random decision, so it was quite strategic. So one of my co founders, actually, Martin Vechev, so he has a really, really big initiative here in the region. He raised 120,000,000 to basically bring world plus science into eastern Europe. And he's doing this quite successfully here. So we thought, well, that's a great idea.
Petar Tsankov [00:04:26]: With LatticeFlow AI, we have very cool deep-tech topics, so we have to have an office right next to this structure. So basically, we're now literally on the same floor with all the researchers in the research labs that are working on these topics.
Demetrios [00:04:40]: And have you seen any tangible outcomes already?
Petar Tsankov [00:04:45]: Not yet, I would say. Still, the vast majority of machine learning team skills is something that we based in Zurich, which is just purely by realizing that Zurich is one of the, for me, the best place in the world to build deep tech teams, especially focusing on machine learning. So not this kind of outcomes yet, but we hope that's coming very soon. So, again, strategic. So it's an early investment and this will also come in the future. Yeah.
Demetrios [00:05:16]: Well, talk to me about Zurich, because I know that you've had some experience working with the very famous. I don't even know how to pronounce it, like ETH Zurich, or.
Petar Tsankov [00:05:30]: I mean, I got into Zurich after. So I did spend five years in the know to study, to focus on computer science, and then I did want to come back somewhere in Europe, there are not that many good options. So I kind of had to choose between London and go to Imperial, Imperial College London or fantastic places to study and everything. But Zurich won me as a city just very well organized. And then when I got to Zurich and I did my master PhD, focusing on security reliability, I did have a pretty clear focus to go back to the US. I was kind of missing this. Not randomness, but the US is a little bit wild. So there's like a broad spectrum of obedience and everything.
Petar Tsankov [00:06:16]: So everything is extremes. Right. And growing up also in Bulgaria, where she also has quite a lot of extremes. So I was missing a bit of this in Switzerland, but again, Switzerland is just amazing in terms of building teams. So right now I'm kind of stuck there with ability to also commit to Sofia. So that's also.
Demetrios [00:06:36]: Yeah, yeah. You wanted more chaos that you couldn't get in Switzerland.
Petar Tsankov [00:06:42]: Well, if you grow up, like think, I think that's actually important also for entrepreneurs for creating new things. So you do need this kind of randomness, crazy ideas that are floating around. So they do help kind of. Yeah, I think they do help come up with the next big thing.
Demetrios [00:07:00]: Yeah. The diverse opinions, definitely. You think outside the box and talk to us about AI safety. I know you've been working on it for a while.
Petar Tsankov [00:07:10]: Yeah, almost ten years now. So it's been a while. AI safety broadly or trust? AI trust, I prefer better is the umbrella term. So the main story kind of how everything happens in the safety space, I would say we have seen basically a whole decade of really having AI research being focused on improving accuracy. And this was driven by all these golden data sets, like imagenet, for example, where you would kind of fix the data and just get the models to make them as accurate as possible. And that has been the focus for decades, actually. Even pretty deep learning. We were trying to optimize this and kind of then what happened is, well, we figured out how we can really get highly accurate models once you fix the data.
Petar Tsankov [00:08:00]: And then everybody got super excited. They said, oh, that's great, we can build vision, speech language model that are superhuman. So now we're going to crack all the problems around the world and just build this super intelligence models to solve everything. And that's where also safety kicked in, because it's a very big gap. There's a very big gap of building something that works on your data in the lab to something that you actually then deploy to solve mission critical tasks. And it actually works well reliably in these new environments. So that all these topics kind of reliability, meaning that it's not just accurate on your data, but also as the environment changes or something else changes, the models kind of has grasped and learned these kind of good features to solve the task in these environments. Then that of course, becomes a big topic because the world changes in the deployment environments are different.
Petar Tsankov [00:08:53]: Safety also. So a lot of these models, especially if you deploy them to solve mission critical tasks, which in my view, is where the big value of AI is, right? We don't want to be solving tiny problems. We want to solve problems in business, in medical and financial services and so on. Then a lot of those models, they affect people's lives. So then how do you make sure that also the decisions don't harm people? So kind of all this kind of hitting everybody and so on.
Demetrios [00:09:23]: So if I'm understanding this correctly, it's very much about how we've been optimizing for one metric, let's say accuracy.
Petar Tsankov [00:09:31]: The example. Yeah, kind of. The data set was fixed, and then you're optimizing how to make it highly accurate, meaning that your models are giving you the expected outcome on this data than to really going beyond this right.
Demetrios [00:09:47]: Now that we don't have the fixed data sets anymore.
Petar Tsankov [00:09:50]: Yes, it's a whole different, whole different set of challenges and even spans. I think if you talk broadly about trust, it spans beyond just pure reliability of these models. It's also about ethics. Are there biases that we don't want to have? I mean, we are about 27 February now. So my feed has been full with a lot of anger from the world about the foundation models that are popping up.
Demetrios [00:10:20]: I guess you're not following that have been produced. This image generator has gone awry and people are up in arms about it. Definitely there's some things there, and I think that's almost like a good case study as to trying to make things too politically correct and too safe in a way, so that you over optimize or you almost overfit for these other types of diversity metrics.
Petar Tsankov [00:10:56]: Especially, I would say, when you talk about using other models, which is something that we see more and more, meaning that it used to be that machine learning was this task where you in a company or whatever it is, you would gather your data and start playing in the lab and building models. But now things are transitioning to third party models. So you have a bunch of good models and you start building applications on top of them. So they don't have to be foundational models to do some. They could be some also custom specialized models. And like in the case with the Gemini, if somebody has built this model and you lack some transparency on how this model was trained, what kind of biases and everything was used to produce it, then I think people would appreciate if they know, if they expect this. But if this is coming unexpected, then that's really a no go for, I think, many, many users around the world. And this, of course, contributed, I would say, a loss to this whole AI safety space because this is now not about a company deploying a model that doesn't have the right kind of accuracy and under delivers in terms of some business metrics.
Petar Tsankov [00:12:12]: This is about end consumers that are playing with these models and just pure disappointment of what's going on.
Demetrios [00:12:18]: Yeah. And that goes back to that trust piece where we can't even trust it to generate an image that is more or less actually correct. How can we trust it on these very important problems and life changing issues like you were talking about? Yes, indeed. And so how do you feel like those types of things can be combated? Is it just being more public, being more outfront with it, if there are signs in these models that are generating different types of biases and making sure that you get in front of it? Or is it trying not to have that happen at all?
Petar Tsankov [00:13:04]: Yeah. So maybe I'll take a step back and try to capture what is the main problem in a way, in AI, safety and trust, reliability to address. And while I do think these models that are making quite a lot of news today because, again, because they do touch the end consumer and everybody can see their mistakes and get really upset about this, the vast majority, I would say, in terms of pure problem to solve is a big part of it is in supervised machine learning, classical supervised machine learning models, where you would build custom models to solve really well. A given task could be in a bank, could be in medical, could be in other application. And I would say that while it's really interesting also to solve the biases and security and other products in the generative models, and we sure is something a big topic to address there is right now. Also, we do see this from an industrial point of view, a very big push to also solve the safety reliability issues in the classical setting, because a lot of these kind of classical, and why this happening. The reason is that when you do build these custom models, supervised models, to solve a specific task, they're very useful because they run on autopilot, you just run them and then they solve something end to end. It's not like I have to read the output and then kind of optimize my performance as a human.
Petar Tsankov [00:14:34]: So they're really valuable and very often also very business critical because they solve very important tasks and still reliability challenges. There are just tremendous meaning that we're talking about definitely more than majority of the models just not making it into production because of these issues.
Demetrios [00:14:58]: So it's funny you say that. One thing that I've been noticing is that there is so much interest in generative AI that it's almost like, the traditional ML systems have been thrown out the window, and people that are doing traditional ML have kind of like, taken a backseat. I have a friend who is hiring for ML engineer, and they aren't doing any type of generative AI. And he said it's so hard to find someone that wants to work on the problems that we are working on because everybody is trying to work on generative AI problems these days.
Petar Tsankov [00:15:43]: Yeah, I think that's true. So we also see it. People want to work on specific topics, especially the ones that are right now very trendy. And I mean, don't get me wrong. So there's definitely very high value. These are really impressive models. They're very useful. But it's not to underestimate also where the value is.
Petar Tsankov [00:16:02]: And typically, I think, especially when it comes to new technologies, it always at some point boils down to the value. Right? Like, why am I doing this? Why am I investing hundreds of millions and billions? Like, what am I getting in return? And the reality is that indeed, that's where a lot of the value is, and it will be in the coming years. So I think that would kind of slowly not come back, but there would be this kind of realization that we really have to grasp this technology also for supervised machine learning and figure out how to reliably deploy these models, because otherwise we would just miss out so much if we don't crack this problem.
Demetrios [00:16:43]: Yeah. Now there is this feeling that I've had that because of the explosion of generative AI and specifically like chat, GPT and Sam Altman going and testifying in front of Congress and the hugging face CEO, I can't remember his name, but he also went up there, and next thing you know, it's like AI is on everyone's minds. Do you feel like that has just blown up the idea of trust and safety when it comes to AI?
Petar Tsankov [00:17:18]: Absolutely, yes. So this definitely contributes a lot to kind of developing this area again, because once you reach the end consumers, then it's literally all over my feed now, like, all these mistakes, issues, biases that are popping up, which was that would not be the case that my LinkedIn or Twitter feed xfeed would be filled with biases because defect inspection model for some semiconductors has a particular bias. That's not something that people would be boasting about every day. But awareness was raised. It definitely also reached. What we do see is a lot of these models, like high stake, mission critical models deployed in enterprises, also supervised models. So definitely managements and executives are also very aware now of the challenges, and that they would very explicitly require more validation, more trusts. Again, especially when you go to third party models.
Petar Tsankov [00:18:20]: We may be interesting here to give a more concrete example. We recently completed actually such an assessment for a third party model used by Migros bank, which is one of the largest banks. And what they do is they're using externally built models to provide just a much better car leasing service. So you can predict what would the value of a car be in the future and give the best possible terms to the user so that they can ultimately lease this car and use it. And so this is all great and nice, so they can use this now massive data sets to build these models and offer this service. But ultimately, this model, you have to understand it's responsible for managing hundreds of millions of swiss friends. So how do you make sure this works right? And that always has been the big challenge at this level. How do you first get it to work really well? And how do you also kind of give this trust also to other people to rely on that this model would work bad well.
Petar Tsankov [00:19:24]: And I would say this kind of general topic of just gaining trust in the models and in new AI applications has been around at the board loans for quite a while. That's actually to a large extent also how LatticeFlow AI started. So we initially did not start with idea to build the company. We were just a bunch of research people that worked on safety verification back then, and we had good success in building some of the scalable frameworks in this space. And then this was back in 20, 15, 16. So quite, quite a long time ago when everybody was still discovering the brittleness and challenges around these models. And even back then, a lot of these large companies like Boeing, Siemens, Bosch, I still remember, they would ping us to our emails, research emails, and they would ask, well, this wave is coming right now. We built classic software systems, we have processes, we have standards, we know how to ensure that they work.
Petar Tsankov [00:20:28]: But there's something coming so soon that there would be a big transition in the industry where we would be relying on just building these massive models on data, and we have no idea. And this would be business critical by definition. Like, if you want to be part of this next generation businesses, you have to be understanding how to get a grasp on this technology. And a lot of them were asking exactly these questions, well, how do I make sure it works? How do I get it to work? What is the process? What standards do I follow? And that's where it kind of also hits me as a researcher. Well, that's a pretty big transition. We better get something better get started working on this and deliver some solution for these companies.
Demetrios [00:21:13]: There is just a little side note that I wanted to mention. When you were talking about how Twitter and the consumers are getting to red team all these models, it reminded me, I think I read somewhere that you were doing red teaming before red teaming was actually a thing. And did you write a paper on red teaming?
Petar Tsankov [00:21:39]: We did safety competitions. So competitions on AI safety, that was back in 2020. So before red teaming, and then we did participate also on some classical red teaming. There was a very nice competition organized by the White House on this topic.
Demetrios [00:21:55]: So bringing that back around to what you're talking about and this transition of going from, okay, I understand how my software systems work. I know that things are predictable, especially in these big enterprises. But then you start sprinkling data and predictive modeling into it, and it's like, I'm not so confident in what the output is. You were red teaming and you realized, you know what, maybe we can almost, it's almost like the white hackers, I guess, is the inspiration. And you said, I think we can figure out a way to show people how robust and how strong and how well put together their systems are. Is that kind of the catalyst for the start of LatticeFlow?
Petar Tsankov [00:22:46]: Yeah. So maybe to give a bit more background. So we were really working on this kind of. So how did it all start? So people are building in research all these accurate models, and then at some points, several smart researchers from Google and Stanford, they were trying to understand how these models actually work. What do they learn? How do they make decisions? And a very easy way to kind of play this exercise is you tweak with the inputs and you see what happens. And then actually for a long time, they thought that there's a bug because you change a little bit and then output just goes through the roof. And it's really completely different, which is totally unexpected. If you have a model that is, I don't know, 97% accurate, and then suddenly it's behaving so odly.
Petar Tsankov [00:23:31]: But it turned out that's a challenge, and there's properties to check beyond accuracy. And then everybody started working on this topic of how do I ensure robustness of the models? And then that's when we also got into this topic. And what we started working on is, I would say, on the far end of deep tech, how can I prove even basic properties about neural networks? And this is typically how kind of people deal with complex systems. We don't know how they work even in software in software, if you have a large code base, you don't know what's going on, but what you can always do is you can express some simple properties of some things that you expect that the model does and then you can verify them and even in some cases mathematically prove them. So we started with, this was the original entry research, and then a lot of the industry was actually really curious because they would read the papers and then it would say, well, I have a mathematical proof that your model is working. And they're like, oh my God, that's great. Now I have the ultimate trust on my models, which is not entirely the case because there's other issues around this. You cannot fully specify what a model should be doing.
Petar Tsankov [00:24:46]: That's kind of the whole point of machine learning. You're learning very complex tasks that you cannot specify, you cannot code, you have to learn them from data. But that's kind of how everything started. And then industry was interested on how do you get this to work? And that's how we kind of got into the space and then started, I would say, this whole journey of learning, what does AI safety and reliability really means in an enterprise, in an industry environment, which was quite different from this kind of standard robustness testing that research was doing at the time.
Demetrios [00:25:22]: Yeah, let's dig into that a little bit more because it does feel like there is a gap and you have the research side of things and you being able to say, like, I can promise you your model is doing what it should be doing. And then the industry where, as you mentioned, there's so many different X factors, how do you see that Delta? And what are some ways that you can bring that trust AI into the industry?
Petar Tsankov [00:25:50]: Yeah, that was a really fascinating journey in the company because we started from the robustness angle and then we got, I would say, broadly speaking. So industry was highly influenced by research at the time because AI was really, AI meant Europe's ICML papers and industry would pick on specific topics in research, like robustness, explainability, you know, oh, that's going to solve all my problems. And we did got a bunch of those requests like, hey, come here, can you verify my models? Correct. And then you go and you check, wait, that's far away from even working. There's all sorts of other challenges. Like the data is very different from what you have in production. The labels are wrong, there's biases in the data on this kind of data. Your model completely doesn't work.
Petar Tsankov [00:26:38]: I'm not proving anything here. First you have to go back, fix all these challenges on the data in the model side, and then we can talk about even deploying these models. And that was, I would say, a journey that the industry was going through as well, because they would want to kind of eager to deploy something that would not work. And it's very unclear what went wrong and what you should do to even fix these models. And then kind of at the same time, in parallel, also at the company, we were expanding capabilities of the product to ultimately build something that's across the AI lifecycle. Because you do need to address data quality. You do need to address, how do I validate the model to find where my model is fading, to kind of close this loop and iteratively improve and get better models. So it has been, I would say, joint journey with a lot of bunch of interesting and excited companies that want to go in production and really building the practical side of it, of what you need to do to get these models to work.
Demetrios [00:27:42]: And you're talking about some serious issues that we've seen time and time out with, making sure that your system is running properly and making sure that you're thinking through each piece of the system as you're talking about there, thinking through these challenges. It is so crucial. How can I make sure that the data I have or the data whoever is building the model has access to is the same data that is out in production? How can we make sure that the labels and the data is clean and it's actually useful data? How can we just make sure that the people that are messing with the data have access to that data? There's so many what you could, quote unquote, call data ops challenges. Right? And then that data preparation, creating a platform seems very smart because it's upstream issues. And the problem that you were trying to go in at, you can't hit the question of, is my system robust? Can I explain the model output if you don't solve those upstream issues first?
Petar Tsankov [00:28:58]: Exactly. And what you also uncover once you get deeper into this is that, purely speaking, you cannot disconnect these kind of problems. For instance, one thing that we do is ability to find what we call model blind spots. Like I have built a model gives you some aggregate performance. How do I find on what kind of data performance is really, really bad? And this you can do in various ways, too, and so on. And this particular problem you cannot detach from, for example, data curation, because very often what happens is maybe it's a bias, maybe it's lacking data, and then you go back and just fix your data set. So you just cannot, for example, what this tells you is you cannot separate data work from model work, one model validation. So these are tightly connected things, and that's why you have to put them under one umbrella so that you could comprehensively solve this.
Petar Tsankov [00:29:54]: And that's, I think, very also important to note.
Demetrios [00:29:58]: So that's so cool. So basically, you can see, and I think I saw in a meetup that we did last summer where you were showing off some of the features of this model blind spot where you can say, look across this distribution of data, the model performs really bad. What is going on there? Let's try and debug that. And so it gives you that insight into being able to debug your system much more. And then I'm guessing you can be like, oh, well, it's because we don't have the proper data. Maybe we can go and either gather that data or we can synthetically create that data, do all of those different things. Right, exactly.
Petar Tsankov [00:30:41]: And you do want to kind of be connecting these things. And what's really interesting about specifically, since you mentioned model blind spots, is that it's a quite fundamental problem. People often talk about the unknown unknowns, right. How do I find out about biases that my model has that influence performance? And I didn't even think about them. So it's very easy if I have some idea in mind and I easier to go and check it. But how do you kind of also automate this task of discovering those? So that's really interesting and very important also from a purely, basically from any mission critical use case you can think about, you do need to be doing this kind of analysis before going in production. Yeah.
Demetrios [00:31:31]: Because it's almost like you're red teaming before opening up the floodgates and you're making sure that you can try to get, you're covering your back before you let it out there and then see what happens almost. And you can never be like 100% sure, but at least in this case, you can see more of, you're like stress testing the model and you're stress testing your ability on the system to be able to say, okay, we did not realize there was a blind spot here. Let's go and work that out before we update this model or push it into production.
Petar Tsankov [00:32:14]: Yeah. And what's also very interesting as a kind of transition or trend in the whole space is that, again, as monos are moving away from, I built my model myself and I'm going to use it to, hey, somebody else built this model and I'm going to use it now. So how do you kind of then bridge this gap? So how do I ensure that whatever data was used is good, that there's no model blind spots, that there are no biases, that best practices were followed? And that's where I would say a lot of the work on AI safety reliability is on framing this problem. How can we ensure that there's a baseline of best practices that are always used for certain kind of applications? And then that's where kind of all the standards are now that are being defined are really attacking this. So we recently had a very nice event at the boss at the World Economic Forum on this topic, and we saw NIST and other people, and that's basically what they aim to do. And how do you define systematically what are the general properties that you need to check on data? Because then this helps you solve this problem as a third party. If I'm a vendor giving my model to some other people, I can just say that, look, I apply this, whatever, ISO 50 to 59, and then I followed all the best practices. So that's why I claim that my model should be okay.
Demetrios [00:33:42]: So anybody that is putting out a third party model provider like we just saw yesterday or two days ago, Mistral came out with their API, right? And making sure that we can trust that API there in some sense should be that it's just kind of right. Right now we're just taking it at face value, like, okay, this model is good, I guess, let's evaluate it. Let's see if it works with my application, and then let's put it out into the public. Let's put it into production and see if it doesn't totally mess up.
Petar Tsankov [00:34:17]: Yes. So this would definitely not, this try and error would not work for any of the mission critical use cases. So this is going to change. And there's kind of two parallel tracks of work that are happening. On one end, you have the legal side, I would say, which is what the EOAI act is trying to do in Europe, is how do they even define the classified use cases on what needs to be adhering to stricter standards, quality standards, and then that's one part of it, the legal aspect. And then the other part is, well, now that I am actually in one of those mission critical use cases, what steps do I actually take to kind of show others that I have followed best practices and that's where standards come into play, where they would list all the potential risks, possible mitigations, or maybe if there's no mitigations, that's something that you have to tell to whoever is going to use this model. That's a problem. So if you're concerned with it, just you have to live with it and so on.
Petar Tsankov [00:35:21]: All this space is kind of being now actively being structured to provide this kind of basic level of trust so that it's not just, hey, here's a model, go and play, and maybe it works. This is not a very good idea.
Demetrios [00:35:37]: I mean, correct me if I'm wrong, but it feels like it is not that transparent at all. Like what you're speaking about sounds awesome. It sounds very far away from where we're at.
Petar Tsankov [00:35:47]: Oh yes. So we uncovered this just a few months ago when we did the first assessment for the swiss bank. And so we did take the ED slices, latest ISO standards. They're quite nice, they define a bunch of properties, but the gap between the high level requirements discussed in these standards to the actual technical objects you have to be running on these projects, on the data and the model, there's very, very big gap. So thinking about, they would all talk about data has to be representative, it has to be relevant and all these things. But what does this even mean? So there was a lot of back and forth with, well, these actually things, these are quite generic, so we can check those, but we have no idea what relevance means. So then you go to the bank in this case and say, hey, what data is relevant to you? And then kind of encode those. So that's going to take quite a few, I would say, iterations, until this is becoming something that everybody can do right now.
Petar Tsankov [00:36:52]: It requires quite a lot of know how basically to bridge this gap because it's just so white that you cannot just read and implement.
Demetrios [00:37:00]: Well, it does feel like that is a case for using open source, large language models because you can control them a little bit more, but even still you don't know how they were trained. And there may be those unknown unknowns that are just kind of hanging out in dormant latent space. I'm sure you saw that paper, I think, cohere put it out, anthropic put it out where it was like, oh, there's just this stuff that can happen because in latent space if you touch it, all of a sudden it's like this bomb that just went off in your model. And I'm doing a very poor job of explaining the paper, which I'll try and find later. But the shit idea there is like you don't have the understanding of how it was trained and how that model was built. And because of that you don't have full understanding at what is possible and capable with that. Are you thinking about trying to retroactively do things like that where, okay, we're never going to be able to work with every open source model provider. You would probably love to work with meta and Mistral and whoever else comes out with their next open source model.
Demetrios [00:38:18]: Right. But in the case that you can't do that, is there the possibility of being able to pop in a model and then test it after the fact?
Petar Tsankov [00:38:29]: Some things yes, others no. So there would be certain things that you could test just in a black box manner, meaning that robustness would be an example of this. Right. I can test some of those properties, but if you talk about, let's say then data relevance representativeness, and I have no clue what data this model was trained on. Well, good luck. You just don't have enough knowledge, knowledge to run these checks. So I think open source, of course that's useful because she does give you some visibility, but it's not sufficient. And in a way that doesn't preclude the need for doing this kind of additional assessments and validations because just look at open source code itself, right? It's not like people would not blindly trust open source code.
Petar Tsankov [00:39:18]: In fact, actually one of the big challenges for the industry was you have all these great open source projects and every developers love it because it's just import, compile and run. But how do you know that means that you're now relying on third party developers pushing commits and you have no idea what kind of vulnerabilities you will be importing. So that was also in the software space that was open source was not a solution, it was just something that was useful because it accelerated developers. But still, there has to be all these additional tooling and systems that would need to track vulnerabilities across versions and so on. So actually builds reliable software. So models would not be any different here.
Demetrios [00:40:05]: Yeah, exactly. I'm not trying to make the case for you grab an open source model and then you're good. It's almost like the open source model, you can play around with it more or have a little bit more insight into it and then pop it into your system and have more control. But I hear you with like, yeah, you can have more control, but at the end of the day, if they're not giving you the data on what that model is trained on, then good luck. You can fine tune it all you want, but you're not going to be able to really get down to the nuts and bolts of it.
Petar Tsankov [00:40:42]: Yeah. And what's also interesting is often you would not want. So for a lot of these companies, data is the IP. So you don't want to just then put up your data and say, hey, look, that's what it is. So then this whole domain of independence assessments come into play and that's in fact how it worked with the swiss bank. So it was a third party vendor building these models that they would definitely not go and publish all the data to the bank because then the bank could just train their own models. So then we had access to the vendors data, training code, everything. So we could plug them in, run the checks and then deliver this to the bank.
Petar Tsankov [00:41:22]: But we are kind of an independent provider that gets this access and ultimately ensuring that IP in data in particular is isolated from the consumer. So that's definitely something, not something to underestimate in terms of kind of dynamics and how this would work. Does it make sense?
Demetrios [00:41:41]: Yeah. And thinking about that a little bit more, you're not talking about a third party provider as the traditional, what I would think of it as a third party provider as like OpenAI or anthropic. You're thinking of a much more specialized third party provider that is building out a machine learning model that is specific for this leasing use case that you're speaking about.
Petar Tsankov [00:42:08]: Exactly, yeah.
Demetrios [00:42:09]: And it's less of like a gigantic llm that you can grab and it's OpenAI's version. And good luck figuring out what's going on there and working with them on that, which I can imagine makes it just even more difficult to have trust in your systems if you're using something like an off the shelf OpenAI instance or just the.
Petar Tsankov [00:42:38]: I mean, there are interesting checks. We have been looking into this also in collaboration with some of the research teams at ETH. Basically, how do you kind of compile some of the checks in the AI act? But again, many of those you just cannot run unless you have access to everything, which is the case today for many of the models.
Demetrios [00:43:04]: And do you feel like safety will be only taken seriously when there's the regulation that is put around it? Just like you have the sock two and you have the ISO and all of that fun stuff? Or is it like because there's a lot of money on the line and because somebody needs to be able to cover their ass, especially if it's dealing with serious amounts of cash, like in this bank example, it's already at the forefront of discussion.
Petar Tsankov [00:43:37]: Yeah, that's the latter case, definitely so again, when we were in Davos, actually, we did have one of those vendors coming to speak and he was upfront, he said, look, I don't care about the regulation. I care for two things. I'm building these mission critical models. I have to make sure they work because otherwise my customers would lose a bunch of money. And second, I have to instill trust in whoever is using these models because otherwise they would just never put, you know, they'll never use them. So that's really where we do. And I think that's very healthy dynamics to observe on the market because you don't want stuff that are purely regulatory driven. I think that's not overly exciting.
Petar Tsankov [00:44:18]: So people do realize that there is real need for ensuring that stuff works. There's real need to demonstrating this to others. And then that's why I always say that you have to focus on getting things to work before incorporating it into standards and regulations because it should not be backward. So what standards and regulations should do is, again, they should kind of go and put this baseline. Once you understand how things should work out, this is the baseline for all these applications in this domain. We want to make sure that these things are checked. That makes sense. The second thing that the regulations are very important is also kind of the whole redlining of use cases, because we cannot ignore that this is super powerful technology and you can do just bad things like mass surveillance.
Petar Tsankov [00:45:08]: Do we want this in Europe? Probably not. So I think that's kind of always been my position, focus on redlining things that we agree on and that's good to do for everything else. Just kind of follow what the industry already needs just to put this baseline, to make sure that some vendors are not slacking off by ignoring these things.
Demetrios [00:45:31]: It is not a surprise that money drives this innovation much more than regulation does. And regulation in one way, shape or form is almost a little bit behind. And you spoke about some of these very vague regulations that are out right now. And I'm wondering if you feel like there are certain regulations that are actually very useful and that you like to see, like the surveillance one could be an example of that. Or maybe there's other ones around the robustness that you were talking about.
Petar Tsankov [00:46:08]: Yeah. So in terms of regulations, I've read them. So these are legal documents, so they are still vague. I think those are typically, again, not an expert, but these get settled in court to see what did this mean? Is it this or that? And then after a few. That's why we always report to cases to show how things were interpreted. So I think there will definitely be a big iteration of this kind to concretize a lot of the ambiguity on the regulation side. I am interested in the standards. I think they are very useful because these are more down to earth technical documents that risk real technical risks and mitigations.
Petar Tsankov [00:46:47]: And those already do provide useful frameworks. So they do exist. Many of them are, we're talking about here, for example, ISO 50 to 59, which tackles data quality. There's 24. That is about model robustness. There's stuff on model governance, and they are well thought out. So I think they would definitely be adopted in some way and they can create enough so that experts can take them and start implementing the checks and so on.
Demetrios [00:47:23]: And so the difference there is the regulation is just the government saying, you have to do this by law. The certification is where you say, I'm going to jump through these hoops so that I can show and I can prove that I've thought about different areas where my model can fail. It's not by any means a must, but it is something that I want to do so that I can get that money and I can get that contract.
Petar Tsankov [00:47:51]: Yeah, and they would be. So I think regulations would require some of the standards to be, that you would need to be certified for certain things, but we're not there yet. So I think that will still take at least two years based on if you look at the UAI act. So that's April, so two years afterwards, if it happens on time, so there will be some time until this takes effect.
Demetrios [00:48:17]: And what were the two certifications you mentioned? 2024, ISO 20?
Petar Tsankov [00:48:22]: Yeah, there is one, it's 52 59. So that's a very useful standard for data quality. There's 40,001. This is about AI defines how to do AI management system effectively. So these are the ones that we see most kind of discussions around those and things that would likely become something that's implemented by the industry going forward.
Demetrios [00:48:48]: And what is the 4000, what is it around how to do AI management?
Petar Tsankov [00:48:54]: Yeah. So this is about just properly documenting what models exist. How do you upgrade models? So defining the kind of, it's around the process on managing models as opposed to drilling down and then checking a particular project, is it actually safe? Does it comply with specific data quality and model robustness checks? So that would be then different set of standards that would apply for these checks.
Demetrios [00:49:25]: And these certificates, they aren't new, are they? It's not like they just came out this year.
Petar Tsankov [00:49:31]: Yeah, they've been in the workings for several years. Now, some of them are very close to final or final. So this is all happening basically right now.
Demetrios [00:49:44]: Incredible. Okay. Yeah, because it does feel like I've heard these being talked about before, but I didn't realize that it is not quite finalized. And it's something that people are actively thinking about. They're trying to incorporate just to get ahead of the curve. So as soon as it is finalized, you can go and get that certificate and say, look, we are thinking about this and we're actively working towards it. And so then boom, you have a certificate.
Petar Tsankov [00:50:14]: Yeah. The thing is, because even if they are not final, it still gives some methodology to it. So let's say for this vendor, for the bank. So if we just say, well, look, I thought of five checks, I think they're good. So that's why the CRO in the bank should trust me, I think that's not a very good argument. Right. So you don't want to rely on something that's more globally acknowledged and accepted by people. So even if not fully final, they're still valuable.
Demetrios [00:50:42]: Yeah, it reminds me of like certified organic food. And you want to make sure that you're hitting the certifications on that or any kind of certification. It makes complete sense. So does LatticeFlow help in that regard? Like, are you actively building into the product ways that it makes getting certifications easier?
Petar Tsankov [00:51:10]: That's a good question. So this is something that's indeed actively happening, because we did start with this general framework for improving data quality and model performance safety based on intelligent workflow, for prepixing data and model issues. But now that kind of the market is also shifting towards using third party models and so on. This whole validation piece is becoming really key. And these things are very tightly connected, again, because they should be. It cannot be that to get a model to work, you have to do these ten things and then standard talks about something completely different. That would be no sense. So basically, now these things are being codified into best practices, into these standards, and it's becoming kind of packaging those kind of packaging the checks that you would normally do as an ML engineer or as a data scientist to improve your project.
Petar Tsankov [00:52:06]: It becomes a kind of checks that you would package then to show to somebody else, as opposed to acting on them to fix certain kinds of issues. So defed is something that is evolving and we are, let's say, very actively also looking at it and playing on these markets.
Demetrios [00:52:23]: So basically being able to expose and show off what you're doing in a way so that it's not behind closed doors, and it is very visible for others and getting that transparency into the workflow so that we go back to the whole idea of, yeah, how are you going to trust something if you don't have these transparency pieces?
Petar Tsankov [00:52:48]: Yes. And that also helps a lot, communicating across different levels in the organization because it's one thing to talk about, I don't know, data biases and labeling errors and the engineering wrong. It's another thing to go to a CIO or the chief risk officer in the bank and then talk about these topics because these are standard frameworks and ways that the industry already works. This is putting this structure so you could actually communicate between these different levels.
Demetrios [00:53:20]: And that was one question that is popping up in my head is how much are you seeing engineers jumping on board with this type of stuff versus the compliance and risk versus any other stakeholders?
Petar Tsankov [00:53:36]: Yeah. So compliance and risk, not yet. So this is still very early. I would say again, this is not something that's developed now. So we still focus on engineers. So a lot of slow AI is used by engineers to get their models to work, but there is this transition going on on the market and that this is also becoming a market on its own. But it's not. Definitely not something that's kind of fully developed yet.
Demetrios [00:54:08]: Yeah, just give it time. Let Air Canada keep selling no refund policies, and Chevy keep selling their cars for a dollar. And all these mistakes. Gemini, keep generating the black forefathers and you'll be fine, man. Compliance and risk will want to get into the conversation before you know it.
Petar Tsankov [00:54:30]: I think so, yeah. I think this year 24 would be. I think last year 2023 was really a lot about the awareness of these challenges. Now, this year 24 is a lot about delivering value with these models as well as kind of making progress with this validation that you could entrust in or instilling trust in the, in the.
Demetrios [00:54:52]: Models, how far do you think we can push the whole deep learning approach to more mission critical applications?
Petar Tsankov [00:55:03]: Yeah, I think that's a really. It was also something that we actually discussed in Davos with the roundtable of experts because there is certainly things that we can make progress on. So all these topics around ensuring safety, reliability for specialized custom models that solve kind of in controlled environments, I think that's definitely in the realm of things that we would grasp. And now the question is, how can we make this systematic and have this widely deployed for everybody? I think the main limitations are wide, well known still, like deep learning, handling the long tail. It's very hard to handle the edge cases, get the model to generalize, to work universally, well across your distribution, all the challenges around, like, I can a bit tweak my inputs and get the model to output something. These are fundamental things, so this would not disappear magically, no matter what kind of safeguards, guardrails, you put around these models. And that was also a comment that one of the participants, his name is Apostle Vasilev, he's a security expert at NIST. He made a really smart observation that I liked.
Petar Tsankov [00:56:18]: He said he kind of brought an analogy from traditional security worlds, where normally you would want to have a secure design. So something in cryptography, for example, is known as information theoretic guarantee. So you know that if you implement this, it works, and then securing the system boils down to just implementing it correctly, meaning we don't have bugs. And that's very important because some of those are design issues. So you can get the model to outputs no matter what. So you cannot just safeguard it by plugging in another model or something else. You need to do something fundamentally different so that the design has a good foundation, and then you can solve it. So, in general, there's a very big difference, and that's important.
Petar Tsankov [00:57:02]: So to basically differentiate benign environments, where you're deploying against something in controlled environment, where people are not trying to attack you, versus something in a fully adversarial environment, where I have full control, and I'm going to do whatever it takes to break your model. And these are things that would not be solved with current approaches. And that's important because, again, these are the risks that we have to know. There's no solution, and then we just assume them or don't deploy.
Demetrios [00:57:31]: If only it could all be benign environments and rainbows and flowers tie dye would make everybody's life a lot, huh?
Petar Tsankov [00:57:42]: For sure.
Demetrios [00:57:44]: Excellent. Well, Peter, this has really been informative for me on the trust AI front. I know that you're knee deep in it, and I appreciate you teaching me ton about it.
Petar Tsankov [00:57:56]: Thanks a lot for having me, Demetrius.