Transforming AI Safety & Security
speaker
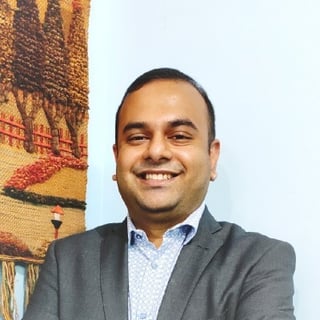
Manoj is the Founder, CEO, and CTO of AIShield, a Corporate Startup of Bosch with a mission to secure AI systems of the world. He is a serial corporate Intrapreneur, and AIShield is the second one. He and his teams have built several innovative and successful products and solutions using multiple classical and emerging technologies resulting in billion $ portfolios. He is an award-winning, experienced, seasoned Technologist with 25+ patents and 13+ papers. He holds engineering and management degrees and his alma mater also includes IIM Bangalore, UC Berkley, and HEC Paris.
SUMMARY
The rapid adoption of large language models (LLMs) is transforming how businesses communicate, learn, and work, prioritizing AI safety and security. This captivating and insightful talk will delve into the challenges and risks associated with LLM adoption and unveil AIShield.GuArdIan – a game-changing technology that enables businesses to leverage ChatGPT-like AI without compromising compliance. AIShield.GuArdIan's unique approach ensures legal, policy, ethical, role-based, and usage-based compliance, allowing companies to harness the power of LLMs safely. Join us on this riveting journey as we reshape the future of AI, empowering industries to unlock the full potential of LLMs securely and responsibly. Don't miss this opportunity to be at the forefront of responsible AI usage – reserve your seat today and take the first step towards a secure AI-powered future!
TRANSCRIPT
All right. Hello. You disappeared, but now you're back. I had any issue with the system. Uh oh. Okay. Cool. Well it's so nice to see you. How are you doing? Doing fine. Thanks for asking. Please. Yeah. Okay, so I'm gonna pull up your slides. Um, and I think if you can just let me know when to move to the next one.
I'll make it out. Yes. Can you see them? Yes, I can see them. So, okay. Thank you. So you have to, uh, be a clicker for me today. So thank you, uh, as well. So, great. So thank you, uh, for having me today. My name is Manoj. I'm a CEO and CT of ai. She, ai she lives in a corporate startup of Bosch. And what I'm going to talk today is about how to transform the AI safety and security.
And I'm going talk you through with a very specific, uh, uh, A slide deck where I'm going to introduce you to the overview of an, uh, the problem and then the technology and the product, and use cases in similar orders as well. Uh, next slide, please. Uh, next. Next,
yes. So, uh, AI issue. Uh, Working for a long time into securing the AI systems. We had a own a traditional product, which essentially does the security of all of the non generative AI related AI systems. What I'm going to talk today is with respect to AI Guardian, which ensures the safe and compliant and enterprise usage of generative ai.
And this is mainly the security solutions works almost as an militarized zone between the LLM and applications where it works as a guard rail. Probes the data and then ensures that during the deployment and the usage sites, the users are protected as well. Uh, next slide please.
Yeah, we have close to 30 plus customers for both of our product offerings, uh, worldwide, and we are one of the leading vendors in the terms of an AI application security, which is the newest category which has been created, and we work with many of the partners across the wide spectrum as well. The next slide please.
Yeah, so Guardian is the most important topic as, as many people are talking about, is having a robust to enable safe and secure, secure adoption of generative AI in enterprises. And this is the topic that we would like to, to, uh, discuss today. Next slide, please.
And it is very funny that everyone talks about that having a benefits and risk as well. And you see that this are some of the numbers which are coming out where it's, it's essentially that everyone wants to use or planning to use. The generative AI is fascinating. It's such a new technology and everyone wants to use it.
And then on there is another end of the spectrum where the people are talking about the risk of it. I just want to highlight some of the risks that is already available. Next slide, please.
So we have already seen all of those kind of, uh, news articles, which are there, right? Employees are feeding sensitive business data to chat g bt basic security concerns or prone to open source, port related vulnerabilities, privacy threats, and many more. And essentially what we have realized is that the generative AI is a double edged sword.
The best way is that you understand and navigate this risk in the enterprise VR, where you really want to use the AI technology, but not having to worry about them so much when not being in the newspaper articles at least, or the one of the leading sites as well. Next slide, please.
So, Now there are, there are two camps, right? Essentially the opportunities who wants to leverage the AI benefits of generating ai, grow productivity gains, and people who wants to create a competitor advantage also with investment in adoption. And then there is another side, which is a risk. They're worried about the risk of loss of valuable intellectual property.
P i I, trade secrets. Also a lot of other aspects with respect to the compliance violations, the reputational issues, the damage due to NIA or bias outputs and the business boards. And when you have these two kind of an accounts, there are only two options which are there today. Next slide, please. Which people are taking, people are completely ignoring the, the risk.
Uh, and using the chat. And then they're assuming this kind of an risk, the brand reputation loss of high legal and compliance issues. And then there is another camp which completely wants to prohibit the use of an en large language model. And then they say no notice, and then they missed out onto the opportunity to improve their business, uh, in terms of the top line, bottom line.
And they'll not be able to compete then. Then that famous say, uh, kicks in also. For the businesses, which is, it's not about that the AI is going to replace your business, but the people or the businesses who are using the AI is going to replace you. So these are the two current dominant positions that we have seen.
Uh, and that is where it is very, very tricky. The space is very tricky and organizations literally have no other options today. Next slide, please. And this is where the, we need to think about how do we balance this. That means we don't have a lot of risk of using the large language models. At the same time, we are able to gain all the benefits of a large language model, and we need something in the middle that brings the best of both worlds.
And this balance is this space that we are innovating as well. And this is the balance is what the guardian does, which essentially balances the risk and ensures that you can have. The best of the benefits, which are coming up from the large language models. Next slide, please.
So we talked about the risk and then there are benefits. Guardian is the solution and a product which can ensure the security data, policy control for any kind of an L L M that you want to adopt in your organization. It provides a specific configurable solutions and ensures that you can do safe and compliant usage, including, uh, the experimentation.
Uh, with this, when we say that it is designed for the enterprises when, specifically the enterprises who wants to use the third party or in-house apps, Uh, which are built with any kind of large language models and APIs. We fortify those particular, uh, usages and we ensure that the output, uh, at the application level is always compliant.
And it is compliant, means that is safeguarded against the legal policy, role based, usage based violations as per organization's policy, which they have, uh, configured. And by doing, this organization gets that the best, uh, benefit in terms of a middle. And we enable the responsible and careful experimentations, they get a compliance with our national's policies rule, their IP gets protected and they're safeguarded against the P i I league.
And it also has a benefit because it's an entire tool, uh, which gives the automations and productivity gains as well. Another question is how does it work? And it works in very simplified debate. Next slide, please. It is, uh, DMZ solution, which is a demilitarized zone. Uh, people who are not familiar with it is anam, which is coming up from the network security, uh, layer as well, where you create an buffer network before you allow anyone to enter or anyone to go outside your network, uh, uh, from your organization's network.
Similarly, the Guardian, which is there on the, the right hand side here, which essentially means that. The user application is there on the one hand and on the another hand in the blue color. What you see is the LLM providers, and then the yellow color is the guardian with six, like in a firewall or as an ized zone.
And if your user is sending any kind of an input, it analyzes it and checks against though your configured policies if it is allowed or not allowed. If it is not allowed, it'll simply block. And nothing happens. And if it is, uh, allowed, then it'll unblock that particular u uh, input that is a prompt or the question that you have asked.
It'll go to the llm. LLM will do its magic, and then the response comes back. And when the response comes back, the one more time, this analysis happens again for the output side policy there, the settings, and if everything is fine, okay? The output is given. If not the output is blocked. You might ask that why this thing happens, why the input is also analyzed by output is analyzed.
The problem or the situation here is that the context and the data or the queries prompts, which are generated, they have the power. To violate the things, and that means you need to have a very powerful solution here, which can understand and analyze each and every query in terms of its context, in terms of its usage and in terms of its role nuances, legalities, and other aspect.
The Guardian is already in a working solution. Uh, what you see besides is in a simple demo, uh, where something question is asked and input gets blocked, which also follows the philosophies. Prevention is better than cure, so it gets prevented. The, the harmful questions never leaves the, let's say, organization and never hits any kind of an l lm.
And second is that, let's say the questions are okay, but the answers might have the violations, then the answers are again checked by the guardian, and then the output also gets blocked in this case. And for, in all cases, nothing happens. So it's as if that it doesn't exist. For the people who wants to use it responsibly and safely, but however it creates in a guard as well.
Uh, next slide please.
Yeah, so now I'm just going to go into the use cases, uh, uh, uh, with respect to the Guardian, that what are the use cases that we have worked upon so far? And that includes the LLM based virtual assistant, uh, documentation search and, uh, L l M assisted software development across various industries. And we have already, uh, launched our design partner program.
Back in the month of, in March, we finished our first cohort, and this was in a very interesting, uh, journey for us where we have learned and when we have, uh, updated in a lot of features as well. Now give you the very specific example onto the next slide, which is with respect to the virtual assistance for the medical use.
And here is the very specific example on the next slide, please. Uh, which is with respect to the doctor patient interaction, which is far empowered by the. Large language model, which is coming up from the organization where we have deployed that particular things and in place of not having any kind of a guardrail when the questions are asked, there are issues with respect to ethical risk and issues with respect to data privacy and confidential, which are there.
Here, the questions is that my patient has an addition behavior, and then what is the maxim oxycontine that can be prescribed to considers a substance abuse case? Ethical risk. And in another case, it is the issue of getting an address of the 10 surgeons, uh, where this particular doctor is not even authorized for, uh, or has a consent for this next slide, please.
And then when the, the guardian is implemented, uh, in this case, what we see is that when this kind of questions are asked, uh, the input gets blocked. The, and the answer is given that the question is considered harmful. And this is how we mitigate the, the issues of an ethical risk, which is, again, contextual heavily.
And then the second one is with respect to privacy, which is confidentially breach is also mitigated because the particular operator is not allowed to have this information. But that is not the only case. Somebody in the organization still needs this information, which is on the next, uh, slide that we see, uh, that when the doctors gets blocked for the similar question.
Like an Oxycontin, but the compliance officer in the hospital who needs to check each and every prescription in order to understand whether none of them is violating, uh, or the auditors who wants to check, they get enough full fledge information. So here the, the magic of policy appears, which is role based as well, uh, and which needs to be established here, and I'll show you that, how it actually happens.
Next slide, please.
This is another use case which is coming, which is with respect to the code, uh, how to use the l l m for the software development. Uh, where the, the guardian does a very simple thing. If you ask for any kind of an, uh, questions which are, uh, considered from coding point of view, the harmful, it'll be blocked.
If you try to put your proprietary code there, then again, it gets blocked, which violates the against policy. And in some cases, if you ask the question and the code, uh, gets generated and comes back in that case it again, uh, sanitizes and, uh, does not provide you the code because in order to safeguard the user against the potential, uh, copyright, infringements, and other aspects as well.
Next slide please. Okay, and then this, this gets automatically extended to the contextual interpretation of an immediate use cases. The next one, which you see is the, uh, is the manufacturing use case where the person asks the questions that how to put achieve part in a boiler machine gets an answer. But if they ask that, uh, anything other question that how to use non-approved part, and by the machine, they don't get an answer here.
And this gets blocked. On the next slide, what we see is that the multi-language support of a guardian, uh, very specifically here, I come from the India, so I have just taken an example of an Hindi, which is the, uh, let's say the, the language which is spoken by many in India. And then this guardian also is able to have the, in a privacy violation, uh, for this kind of an a setting.
And it is a multi, uh, lender support as well. On the next slide is one of the famous examples that people talk about with respect to Jane Breaks. Uh, and the guardian has an mechanism that it has able to identify all of their, uh, the jailbreaks here and simply blocks this jailbreaks. And these are some of the nastiest jailbreaks.
I'm going to show you our early results when we are done. The jailbreak, uh, benchmarking against multiple LLMs, uh, with Guardian and without guardian as well. On the, now we have seen this entire journey that what are the use cases, how it have delivers a benefit, what it looks like. I want to open the hood and go back there a little bit under to see that, how the product looks like and how it really works and how it gets free.
On the next slide, uh, please. And after that, one more slide, which is, uh, feature support and after that, one more slide. So the third slide, it is, as we have seen, that it is just, uh, uh, Let's say, uh, middleware, which is almost like A D M Z. So it comes with a very simple python, kind of an SDK that we have developed for, to make it easier for developers to use it.
And you can start working, uh, with a guardian, with just two line of an code, uh, very simply. And you just have to configure the policies with the Ready Python sdk, which is simplified with the three cross three mapping, uh, as well. And then you have this, uh, The dynamic policy enforcement that happens logging off all of these explanations in the offline mode.
It happened and it works across all type of LM and deployments, and it partially supports the large vision model Also today, uh, especially for the textual, uh, violations, and this is to, in this is to give you the feeling that how easy it is at many places to, for developers to use this, the difficult part is to generate this kind of an entire.
Uh, system and the product and solutions as well. On the next slide, what you see is the integration example, uh, which is coming up with an l l M, uh, that how the, the Guardian works, which is a zone that you, uh, have and then post, post this aspect of, let's say you have deployed it here. Guardian, guardian is also working with L LM and search and natural lynching and, and lot of other things.
Uh, what is important here is to see that it works. Uh, uh, it works exactly like the middleware and it integrates very easily. So it's a passthrough, uh, for everyone. However, if anything, uh, under what needs to happen, then the guardian takes care of that and stops this kind of an interactions. On the next slide, I want to, uh, focus onto the, This will be deeper architecture than what the guardian looks like.
And this is the deployment architecture along within a block diagram, which is there. So the one side you have a user on the, another side you have l l M. And in the center is the entire, uh, block that how, what the Guardian has. So Guardian has an simplified policy table with role mapping that we have seen.
It has the, its own fine tune guardian, L l m as well as the domain specific fine tune guardians. Set up an uh, uh, purpose built foundation models, has an prompt engineering aspects. As well as the, there is a, the decision aggregation mechanism, which is there, and then also the classification models. With respect to the custom board, which has been designed, the whole idea is to look into the various aspects, to understand the context, decide, uh, based upon the policies, and then also do an a fair assessment and aggregate it, and then decide whether it is violating, whether the input is violating, the policies that you have set up, or whether the output is violating.
The policies that you have, set it up and then all of this data gets locked as well. So this is the, the under the hood, uh, which is there, what is there in terms of a technology is also in a very important, when I said that the custom finetune models, uh, if you just pass forward to most life skills, you will see that the, uh, there are various stages of a guardian that we have, uh, done as in a fine tune, uh, as well, which was the one of the important, uh, model which you see, which is a custom, uh, purpose build.
Fine tuned models. We have used the OIGs Moderation dataset and trained the base LMS from the gpt jcs, and this is what we call it as an amazing capability, which is mainly for the safety and security focus. And then for the various domains like healthcare, finance and software engineering, there was a domain specific, uh, fine tuning, uh, which was done in adaptation, which was done and in parallel, all of this thing was also supported by the various prompt engineering aspects in order to ensure that there is a resistance against the various, uh, chain breaks as well.
Uh, next slide please. Here is, uh, with respect to the, uh, jailbreak. I just wanted to give you, uh, some of the early that are there when we are tested with the various, uh, large language models. So Azure Open AI without Guardian, with Guardian. What kind of an settings? Similarly, only we two. And then Alfa newness, which are there.
Uh, from the information that I can share today, you see that without Guardian, uh, Azure Open AI APIs almost. Only it is able to stop 11 out of the 50, uh, jail breaks, which are there that we have specifically crafted. That means it has the only a 22% blockage rate with Guardian. This goes up, it goes 74%.
It does not go a hundred percent. There is a work which still needs to be done. That is why we find is an early result. But this is very promising things that we are able to lift the, uh, add additional 50% of the, let's say, the resistance. Inside the, the models. Similarly on the Dolly v2, uh, there's no inherent resistance inside the model with respect to the jelly brake.
So everything, it lacks nothing. And with Guardian, uh, we were able to boost to the 44 person and then we are going to see that how further that we can, uh, do it with this. And this is some very interesting results that are, are coming from our side and we are continuously working in and striving to improve further.
The next slide, and I'm now going to wrap it up my presentation, uh, which is essentially that by using the guide rate, uh, there are benefits. You can realize all of your benefits very easily, that you have thought about, uh, with respect to compliance, responsible care for implementation and safeguarding and all of these aspects, and.
The path for implementation of balancing is hard is what we have figured it out. Like training the l l M is hard path for balancing is also hard, uh, which requires a lot of self implementation. It's very ex expensive. You need to have a lot of knowledge and skills and there's an then power issue that you already see apart from the computer power related issues.
And Guardian is precisely that we have built for the large organizations as well as for every month in order to ensure that they can. Create the benefit of the L l M and have the realize their advantage along with this journey, which is a very fascinating journey as well. Last slide please,
and the now until the last slide. I just want to give the, the summary, which is like generic AI and can transfer productivity and efficiencies, which are there and everyone wants, should use it. Uh, I think there is not, you know, stepping back from that aspect. However, at the same time, everyone need to be aware also about the damaging risk and hindering this implementations that comes along with those particular risks.
That means depriving the organizations, the customers, and then the end users, the benefit of the l l m. So please be aware about that particular things. And finally, uh, the Guardian is one of the answers, not the answer, but it's another, the answer to ensure that we can work in the best setting to mitigate this risk and still, uh, enjoy the benefits of generative AI and unlock the proper potential, uh, for businesses to sustain, thrive and grow in the future.
So, Simple request. Use guardian with, uh, generative and l lm and unlock your business potentials. Thank you for your attention. And if you have any questions, I'm here. Woo. Yay. Thank you so much, Monas. That was awesome. I think you have a question in the chat, so I'm gonna send you over there though, because we have a couple of people queued up.
Okay. But thank you so much. This was an awesome talk.
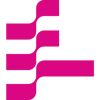