AI For Good - Detecting Harmful Content at Scale
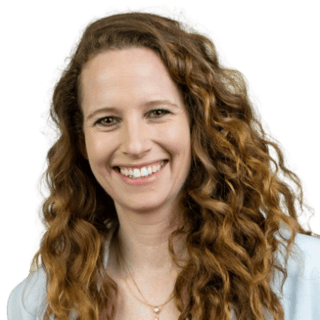
Matar Haller leads the Data & AI Group at ActiveFence, where her teams are responsible for the data, algorithms and infrastructure which fuel ActiveFence’s ability to ingest, detect and analyze harmful activity and malicious content at scale in an ever-changing, complex online landscape. Matar holds a Ph.D. in Neuroscience from the University of California at Berkeley, where she recorded and analyzed signals from electrodes surgically implanted in human brains. Matar is passionate about expanding leadership opportunities for women in STEM fields and has three children who surprise and inspire her every day.
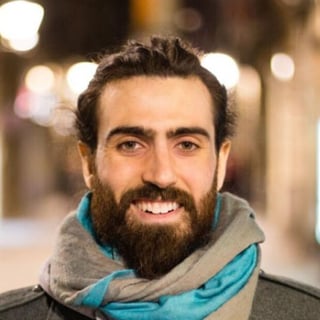
At the moment Demetrios is immersing himself in Machine Learning by interviewing experts from around the world in the weekly MLOps.community meetups. Demetrios is constantly learning and engaging in new activities to get uncomfortable and learn from his mistakes. He tries to bring creativity into every aspect of his life, whether that be analyzing the best paths forward, overcoming obstacles, or building lego houses with his daughter.
One of the biggest challenges facing online platforms today is detecting harmful content and malicious behavior. Platform abuse poses brand and legal risks, harms the user experience, and often represents a blurred line between online and offline harm. So how can online platforms tackle abuse in a world where bad actors are continuously changing their tactics and developing new ways to avoid detection?
Matar Haller [00:00:00]: My name is Matar Haller. Matar rhymes with guitar. I'm the VP data and AI here at active pens. And although I don't, I have three kids. I don't drink caffeine. I drink decaf black tea with milk.
Demetrios [00:00:13]: What is up, MLOps community? You are back for another podcast with your host, aka me, Demetrios. I'm gonna be real with you. Before you go any further, if you are one of those people that does not like hearing about the dark shit in the world, do not listen to this episode. If you get triggered easily, we go into some depths and the thrones of the Internet, the horribleness out there and how AI plays its part in helping to make sure that there is not as much horribleness on the Internet. Matar walked us through these very high stakes AI use cases on keeping hate speech and all kinds of nasty stuff off the Internet and how her company, active fence, is playing its part. I'm going to leave it at that. I'll let you decide if you want to go forward or not. Let's get into it.
Demetrios [00:01:12]: If you enjoy this episode, you know what to do. Share it with a friend who has the ability to listen to this type of stuff or give us some feedback. Spotify's got a cool thing where you can give us a few stars. We'd love that. All right, let's get into it. I'm going to start it with this. We met because you were presenting at the Tekton apply conference, the virtual conference, and there was a ton of amazing talks, but by far the one talk that stood out to me just because of the novelty and the good that you are doing was your talk. And so I want to explain.
Demetrios [00:02:01]: I thought, well, first I have to have you on here, and I want to explain a bit of what I remember of that talk, and then you can maybe fill in the blanks of what it is that you're doing. And so you are using AI to make sure to. I think it's you. You called it AI safety tech. And so you are making sure that when there is hate speech, when there is stuff that we just don't want online, you can detect that and make sure that it is cleaned up. So that's like, first part. Can we start there? I know I just gave a gigantically broad overview of it, but now I'll let you fill in the details.
Matar Haller [00:02:41]: So I don't know what talk you're talking about. My talk was about dinosaurs.
Demetrios [00:02:44]: You're like, I was way off. Wait a minute.
Matar Haller [00:02:50]: So, yeah, that's right. So my talk was about using AI for trust and safety and how at activence, we are an AI trust and safety company, and what that means and how it's done and why it's a super hard problem, but one that is also incredibly impactful and touches us, all of us, every day.
Demetrios [00:03:17]: I think one thing that you did go into pretty in detail in that talk, and we'll leave the link to that talk in the description for anybody that just wants to go directly to that before they come and listen to this. But you mentioned why it is so hard, and you kind of went over all these different use cases on, whoa, wouldn't you think that this should be okay or not okay? And there's a lot of ethics involved. There's a lot of times where I was saying to myself, like, yeah, I think that's okay. And then it's like, oh, you do? Well, maybe you want to think twice about that.
Matar Haller [00:03:53]: And so, yeah, it's so, first of all, I think it's like, besides the fact that it's hard, it's also really, really important. Right? So, like, who cares? Like, why even trust and safety? Why is this even an issue? And I think trust and safety isn't a new concept. Right? Like, if you think about, um, what happens online. So online harm can lead to offline harm, right? Um, I think, like, for me, the most, um, like, visceral and jarring example of that was what happened January 6, um, with, like, all of this, like, you know, this misinformation that we were tracking, uh, prior to elections and that. And that we were notifying platforms about to take down, um, and what that led to with the capital rights and so forth. So, um, there's many, many examples that we can think about where online, online harm can sort of leak offline. And so much of our lives are online. And also, I mean, as a mom, it's something that I, like, care about deeply.
Matar Haller [00:04:46]: I told my daughter, like, she's nine, and all she's asking about all the time is a cell phone. And I'm like, maybe when you're 35, me. But at the end of the day, this is something that's like, it touches all of us, right? Whether it's comments on posts or chats or I videos that we see or images that we share, it's like our lives are online. And although trust and safety isn't new, it's now, I think, after sort of these sort of big events that we've seen, like I said, January 6, and also some really well known trust and safety fiascos that we, if you think back, they'll come to mind. It's now, it's find something like really, really being discussed, right? Like we'll see it in the media and even like, regulators and legislation and the Digital Safety act and, and even users now care about this. Right? It used to be that, like, it wasn't even something that us as users would think about when we went online for a platform. But now, like, we're exposed in a lot of ways to a lot more hate speech or a lot more bullying or, you know, just really vile content. And it could be just because oftentimes content moderation just isn't good enough.
Matar Haller [00:05:55]: And also because it's an extremely, extremely adversarial space. And so we can talk about why it's important to companies and to users. It gives a competitive advantage. You don't want to be exposed to that. But like you said, it's also really, really hard.
Demetrios [00:06:15]: Well, can you break down a few of the use cases? Because I know we're talking a little bit high level, but exactly what is it that some of the things that you're doing so we can get a better sense of why this can be so hard.
Matar Haller [00:06:28]: Yeah. So at activence, we are in the content moderation space. We don't do the moderation right. We flag it for the platforms. We give every item. We have an API. Customers can, platforms can send their content to us and then it gets a probability of risk. And what do we mean when we say content? This content can be audio, video, images, text.
Matar Haller [00:06:51]: The text can be comments, they can be chats, they can be usernames, they can be descriptions, they can be titles, it could be podcasts, it can be short. The space of online stuff is huge. And we deal with all of that. And not only do we deal with that, but also the text can be in many, many different languages, and there's many, many, many different cultural contexts, right? Calling someone a dog in one language versus another language has very, very different meanings. And if we're going to be trying to keep users safe, we need to be aware of that and how slang changes and so forth. So it's difficult because it requires sort of subject matter expertise, right? You need to know the language, you need to know the slang, you need to know in images. You need to know, is this logo of a hate speech group? Is this logo benign? Is this just like, you know, it needs, it's, you know, it's constantly changing. So unfortunately, there's new child abuse content every day.
Matar Haller [00:07:48]: There's new hate speech groups every day. And also the bad actors, they're not stupid, right. They're bad, but they're not stupid. And so they're trying to evade detection. And so they'll use phrases, they'll use lead speech, they'll try to obscure stuff. So that's, I mean, it's a very, I hope, over brought it down a bit from the high level. It's like very concrete in terms of, like, the media types and the challenges.
Demetrios [00:08:13]: Yeah, exactly. And so you're getting a all kinds of input data that is basically coming through the API and you're saying, yes, this looks good. Or maybe like, do you give a scale of how potentially harmful this could be? And then you send it back to the people that send it to you.
Matar Haller [00:08:35]: Yeah. So what we have is basically we have something that we call policy management. And so it's sort of this UI where clients can come in and, and they can basically decide what for them is a violation of policy. So I'm a child. I'm the platform for children. I don't want any nudity. I don't want any swearing, I don't want any bullying. And so I can go in here, I could say, okay, here's a model for bullying, here's a model for swearing.
Matar Haller [00:08:59]: Here's a model for nudity. This is what my policy violation looks like. And then I send in my content, varia PI. And then we basically fan it out. We have our models that run, and then we can provide a risk score. Like, what is the probability that this item is risky? It's not severity, it's probability of risk. And then basically clients can come in and they have a UI and they have moderators, and they can say, ooh, like I. Any item that's like, you know, more than 80% probability of risk, I want to automatically remove it.
Matar Haller [00:09:26]: So they have these codeless workflows where they can just set it up. Any item that's like less than 50, I don't even want to look at it. And then there's this sort of sliver of window where I say, okay, these are things that require an expert to look at. And the idea being is that we care about not only the user's wellbeing, but also the moderator's well being. Right. And we don't want to expose them unnecessarily to harms. Right.
Demetrios [00:09:49]: Yeah.
Matar Haller [00:09:49]: So we can catch the things that are highly, probably risky and just remove them. Then we've spared them from, like, this gory, awful stuff.
Demetrios [00:09:58]: Yeah, that's. I actually heard about that back in the day on how people that were doing content moderation for Facebook. It was like, menial things, 80 or 90% of the time. And then they would be clicking through and, like, whatever, boring, boring, boring, and then, bam. Just getting something that would rock your socks off and give you nightmares for the next two weeks. And so it feels like if you can remove that completely, that's very valuable. And now, going back to the idea of the models that you have, you're allowing someone to choose which models. And I'm assuming when we say models here, it's like policy models, or is it machine learning models or AI models? There.
Matar Haller [00:10:45]: A little bit of all of the above.
Demetrios [00:10:47]: Okay.
Matar Haller [00:10:48]: It depends on the use case, right. So our system needs to be able to handle thousands of requests per second at very, very low latency. Um, if you think about it, um, things are coming online all the time. The only way to deal with this massive amount of content at scale is with models that are able to do the work faster and more efficient and with less, like, mental harm than humans. Um, and so, uh, we do. We have, uh, transformer models. We have, um, uh, ensemble models. We have every.
Matar Haller [00:11:20]: Everything under the sun. Um, we use where our big focus is on ensuring that it's also very cost effective and very accurate. And, um, as much as we can, we want to, like, win on both. And usually we do. Okay.
Demetrios [00:11:35]: So the idea is. Is fascinating to me when it comes to all the different models that you're using, making sure that they are fast and they're accurate, because usually, especially these days with transformers, it's like you kind of sacrifice one for the other, right? You've sacrificed speed at the. Maybe it's not the most accurate, or you want accurate and it's not super fast. How do you think about that trade off?
Matar Haller [00:12:00]: We don't always use the most accurate model. That's the slowest and heaviest, but sometimes that's what's necessary. And we also try to enable our customers to be part of that conversation. Like, what's more important to you? Right? So, for example, for some use cases, keywords are fine. Like, they're totally good. And we have a keyword matching tool that's completely self service. You can, like, put in the keywords that you want, put in the. Even the emojis that you want.
Matar Haller [00:12:31]: Because in a lot of cases, like, for example, in drug trafficking, emojis are super important. In child pornography, also, emojis are very, very important. If you think about it, this is like a very evasive field. Right. And so we have a tool that basically enables clients and also our subject matter experts to sort of put in the emojis or the keywords or whatever in the languages that are relevant and then run with that. And so, and that's super fast, super cheap, super effective. We can do different workflows. And so we could do something like if this triggered, then let's run that and let's see what something heavier picks it up.
Matar Haller [00:13:10]: So we have different ways of handling that. But at the end of the day, also for some models, like a video model, then there needs to also be an expectation of, like, this isn't going to be instantaneous, right. Or an image model that's taking an image apart and breaking it up into components and looking for logos and known bad actors and victims and all these things. It's, you know, let's set an expectation of how long something of that can reasonably take and at what price.
Demetrios [00:13:36]: One thing that I do remember from your talk was how hard the cultural references are. And so how are you making sure not only from like the subject experts? So I guess you have to have somebody that a understands the cultural references and can then translate that into the models. How does that happen?
Matar Haller [00:13:58]: Yeah, so at active fence we have, we're very, very fortunate to have a very large team of experts of subject matter experts, of intelligence analysts. And they're experts in child safety, in hate speech. They know about vegan neo Nazi group in Argentina. They know all these sort of edge cases. They know the news groups, the news, and we work really closely with them. And so when we're developing a new model, the first thing that we have to do is we have to say, okay, what is the policy, what is in and what is outd? Because until, if you don't have a very, very clear policy, then that's going to influence everything downstream. It's going to influence your data labeling, it's going to influence your evaluation, and it's going to influence you when you reach production. We work very, very closely with a policy manager and also with these experts to define what's in and what's out.
Matar Haller [00:14:50]: And then we go out. We have a data ops operation here that can collect the data, whether it's from real traffic or from forums or even generate the data so we can in some cases.
Demetrios [00:15:03]: I was just going to ask.
Matar Haller [00:15:04]: Yes. One thing that we do is we generate data either manually or with the help of an LLM friend. And the reason that we do that is to combat bias. Exactly. For the things that you're talking about. But in order to identify that we even have bias, it's not enough to sort of just look at precision recall. Just now, we had a model that we were working on. I think it was sex solicitation, but it might have been something else.
Matar Haller [00:15:38]: I can't remember where we looked. And we're like, great precision recall. Amazing. And then we ran our functional tests. And functional tests is something that I highly, highly recommend I for anyone that's building a model.
Demetrios [00:15:55]: And what are these? What are functional?
Matar Haller [00:15:57]: So a functional test, what this does is it basically enables targeted identification of model weak spots. What that means is that you basically take subsets of your policy or subsets of your target definition, if you will, and you write tests specifically for those things. So, for example, for hate speech, you can have reclaimed slurs. You could have hate speech expressed using a slur, right? These are sort of two sides of a coin, and you want to have a test that basically ensures that it's, you know, if I say I'm proud to be a. Or I'm the freshest in town, these should always be negative, right? This is like a set of, you know, bunch of examples of reclaimed slurs that I need to make sure that my model doesn't mess it up, right? Because that's, like, one subset of my target definition. If I say, you are just a mmm to me, or I hate or dirty mmm, right? These are slurs. And I'm going to have a bunch of those examples, and I want to make sure that my model is consistently performing and flagging those as negative. And I have a huge bank of functional tests.
Matar Haller [00:17:09]: And then precision recall is important, but I want to look at how I perform on these, because then I can say, oh, great, my model is amazing, except I'm always failing, for example, on her asset and bullying. For a while, we were always failing on wishing someone cancer. We just couldn't get that. And you need to catch that. Sorry. My threshold for things that make me uncomfortable is not normal after working here. So go.
Demetrios [00:17:33]: Sorry. Totally fine. It's just I was just thinking, like, I would never want to get into an argument with you because you probably have the best disses in the world. You've seen all of the force kind of shit.
Matar Haller [00:17:47]: Did I come work here because of that, or did I?
Demetrios [00:17:51]: Oh, I didn't think about that part. Yeah, so sorry, I digress. Where were you?
Matar Haller [00:17:56]: Notice I'm saying, so those are the functional tests, right? And so. And so then what we. So. And also what this helps you is like, looking at over time, right? So, like, you have a new model, or we're in an adversarial space, the world changes, and suddenly we're like, falling apart on a specific type of functionality. And then what we can do is, okay, so we've discovered a functionality that we're bad at. So what do we do? So we can go out? If we're like bad on many functionalities, then we can just go out and get more data. Fine. But if it's a specific functionality, then we can generate more data to address that particular bias that we have.
Matar Haller [00:18:31]: Right. And so, and that can be done manually or, like I said, with genai techniques.
Demetrios [00:18:39]: So, so many questions from this, and I love what you're doing and how much depth you're going into your LLM friend. It's not your run of the mill llama. Three, I imagine, because those have been handicapped, they're not going to spit out all kinds of generated data that is going to help you in your use case. Or are you creating your own model that is highly toxic, we could say.
Matar Haller [00:19:05]: So two things. One is a lot of times it's really, really helpful to use the LLM actually to generate the benign side of things. A lot of times, bias is actually, if your negative examples are all slurs about a particular ethnicity, then you want to add data that shows that ethnicity in a positive light. And so that is easy to do with any LLM. Like, give me some examples of, you know, of texts about whatever, about, you know, some religion or whatever, and it'll just give you. And those. Those are good and those are reasonable. And I'll say that one advantage of having subject matter experts working with us and having teams, that part of what we do is we do red teaming for big foundational models, is that we also know how to cause even the best of models to behave better.
Demetrios [00:20:02]: Nice. Yeah, very cool. That's always fun, trying to trick them and getting to do that as a job I find very interesting.
Matar Haller [00:20:11]: Yeah.
Demetrios [00:20:12]: The other piece that I wanted to go down is, okay, so you've got all of this data or you've created this model. I can see various scenarios on how this can become not that useful very quickly. Whether it is new terms that are coming into vogue, we could say, or you have a lot of data that was generated or the model was created, and it's almost like this snapshot in time for up until a certain date, and then it has the cutoff. So the new terms come and then you can't use it. And so you have blind spots there. Are you continuously retraining to make sure that. Okay. And how does that process look?
Matar Haller [00:20:58]: So one thing that we do is, first of all, like, before we deploy to production, I think we do a lot of back testing. We look at it on, you know, product, you know, production data that we had, and we do. And we sort of look at the, even on unlabeled data, we'll look at distribution of scores. Right. You want to see and you want to look at things. If you have things that are labeled, then we look at things that flipped. Right. And then we say, okay, did, like, does it make sense that it flipped? Are we, are we better with our flip or worse with our flip? And then also just look at for on unlabeled data, like, what's my distribution, right.
Matar Haller [00:21:25]: If I have a new model where now things are, like, much, much, much more positive, unlikely. Right. Or much, much, much more negative, also unlikely. So that's like, before we even head out into the world. But obviously your model hits production, and sometimes you fall apart right away and sometimes you fall apart later, but at the end of the day, like, you're nothing. The world is tough and not forever.
Demetrios [00:21:45]: Yeah.
Matar Haller [00:21:46]: And so what we do is we actually have an auditing process. And so we have trained analysts that will sort of do audits of our models in production based on real production data. And also some clients also share with us, enable us to sort of see their moderator decisions. And so we have, like, real labels from how our model is performing. And then we have sort of our policy managers and analysts look and say, you know what? There's a trend I'm seeing here now. Like, somehow you're always missing that when they're talking about, like, food, it's actually not hate speech. And this actually happened to us. Like, we had a food delivery company and we had a hate speech model because they didn't want people.
Matar Haller [00:22:27]: It was like a chat or review system or whatever on their, on their website. And our model went to production, and suddenly we were flagging like, I hate indian food or, like, chinese suck or all these things. And it's like people are talking about food, like, they're not talking about the people. And then, so these sort of, and then, and then we're like, okay, now we need to go into, like, a targeted, like, model improvement session, retraining and so forth, and then, and then bring it out. And we, this is constant. So we're sort of at the same time that we're developing new models and sort of coming up with new sort of policy areas to cover. We're also, like, retraining our models and ensuring that we're up to date based on sort of looking at how the model is actually performing in the real world. And again, we'll also look at the distribution of scores in the real world.
Matar Haller [00:23:14]: Right. We don't need labels to be like, hmm, there's drift here. Like something is going on.
Demetrios [00:23:18]: Yeah. And so, basically, it's me choosing, as a customer when I'm hitting your API, how many different models and protocols I want to be looking at my data from. And so, for each one of these, I'm assuming that it's not just, like, one model each. Maybe you have an ensemble of models for certain types of use cases.
Matar Haller [00:23:43]: Yeah.
Demetrios [00:23:44]: Can you break that down?
Matar Haller [00:23:45]: Absolutely. So one example is, like I was saying, for hate speech or for terror. Terror is an example. So we know how to look for specific components of, like, different objects or different markers within the image. And then, basically, those go into a train ensemble model that's able to give what is the probability. So, for example, we have logos. So Isis and so and Hezbollah and so others. We have faces there.
Matar Haller [00:24:13]: So known bad actors. And this is relevant not only for Tara. Did you know that there's also logos for child pornography studios? Those are other logos that we can look for. And so, in many different situations, we basically can break apart the image, look for known victims, look for nudity, look for things that are indicative of child pornography, hate speech, terror, and so forth.
Demetrios [00:24:42]: So the experts here are involved in the curation of data, making sure that you have robust data when you're training these models. But then they're also getting involved in that, like, 50% to 70% that gets flagged as a maybe, or is that just getting thrown back to your clients as, like, you might want to check this out.
Matar Haller [00:25:05]: We do content moderation in terms of, like, showing what. Like, what's the probability of risk? But we don't have moderators. Right. That's. That's the client. But what we do is we do internal audits for ourselves to ensure that our model is still performing, like, how. How they should be. So that, like, 50% to 70% that that's not.
Matar Haller [00:25:28]: That's out of my game, right. That's, like, goes to the client, they can do what they want. I can recommend to them. Right? Like, I can recommend. I can say, look, there's a 90% probability that this is child pornography. But if they don't, if they look at it and they say, not by me or they don't want to. That, that's them. But I do have, on my end, I'm going to be auditing that and, and using it to improve my abilities to detect.
Matar Haller [00:25:47]: I was going to say, I was going to sort of say that all of this we're talking about is just regular content that we see online or whatever. But right now with Genai, the space has changed. And so if it used to be that making high quality, bad content or malicious content, you could do it at a low scale to make it high quality, or you could do it at a very high scale and make it really low quality, like, um, now you can do, you know, really, really high scale and really high quality malicious content, which just means that the need for AI is that much stronger, because the only way to do it at scale is with AI. And the thing is, is that for us, like, like, just like we've been the experts and we've built a lot of expertise in content moderation, like we can now we're sort of transferring that to AI safety.
Demetrios [00:26:42]: And how much of this also is just trying to detect if it is AI generated or do you not care about that at all?
Matar Haller [00:26:51]: So that's for our space. That's less of an issue, right? Because child pornography is bad, whether it's real or gen AI, I don't want that on my platform. I don't want to be exposed to that. Same thing with errors, same thing with misinformation, same thing with hate speech. It doesn't matter. It's bad. And so that means that at the end of the day, regular UGC like, user generated content and AI generated content are pretty similar in the space that we're at, which means that we can leverage our experience and be the experts here as well, basically taking everything that we know from the trust and safety space and just transfer learning it. And so for both of them, you need this sort of, like, multi layer detection, and you want to mitigate in different places in the life cycle.
Matar Haller [00:27:37]: And so there's also, except that there's sort of a twist here, I guess, where with AI generated content, we can enter sort of the chain, the food chain earlier on.
Demetrios [00:27:52]: How's that?
Matar Haller [00:27:53]: So if for user generated content, we can catch it on the platform side, right? Like once it's come to the social media platform or the gaming platform or the dating platform, that's where we're at, and we can help them find it. But with sort of, with AI generated content, we can actually be at the source, right where these jennings are being trained, and actually, we have partnerships with cohere and with Nvidia specifically for that.
Demetrios [00:28:24]: Nice.
Matar Haller [00:28:25]: To sort of help improve the models in red team and so forth. And already at the source, it's obviously not enough because besides the big foundational models, there's also open source models, and even foundational models can make mistakes and so forth. And so we need to be at both places, but we're moving up sort of in the food chain with Genai, with Nvidia, actually, I don't know if you've heard of guardrails.
Demetrios [00:28:54]: Yeah, yeah, yeah. Nemo.
Matar Haller [00:28:55]: Yep.
Demetrios [00:28:56]: Nemo guardrails, yeah.
Matar Haller [00:28:57]: Nice. So you can actually, through Neemo guardrails, you can hook up to our API with an API key and have our models on your prompts and on your outputs to ensure that they're safe.
Demetrios [00:29:11]: Oh, that's cool. So then it's like that added confidence that you're going to not be having anything that you don't want coming through. Now, one thing that I was thinking about on this was like, are you just using cohere models off the shelf, or is everything that you're doing super fine tuned for these different use cases?
Matar Haller [00:29:36]: So cohere is actually, they're a client of ours, so we're helping them make their models safer. So in a lot of the things that we learn with our work with them are useful for us to understand sort of how to, you know, how to make safer models. And so that's like a different kind of partnership with Nvidia. They're using our models, our predictive models, in order to provide risk scores on input and outputs and Neemo guardrails. And then in house, we use a wide arrange of solutions, of solutions if we need to generate data.
Demetrios [00:30:18]: And so the solutions are on, like, let me take a step back. It's like one part of the pipeline is generating the data, and then another part is having that machine learning or AI model or a lot of them. You maybe have the computer vision model that will give you the text that's in the meme that is potentially harmful, and you've also got the model that's going to tell you if that text is good or bad and give it that score. And you've probably got a few other models in there. None of these are like, you being able to send a, send any of that information to, like, an off the shelf model, I would imagine, because it has to be very, very specific to each one of these use cases and, like, built from the ground up. If I'm understanding it correctly.
Matar Haller [00:31:10]: Yes. Correct. Yes, that is exactly right. You oftentimes you'll see open source models for finding hate speech or for finding this or whatever. What we found is they can't work at scale. They're going to fall apart at the scales that we're running at. There's no way they are inconsistent. If we take it back and say policy is important, they're inconsistent in their policy.
Matar Haller [00:31:33]: They don't get the nuances. If we look at all of our functionalities, they fall apart. We take the integrity of our data and the integrity of our labels and then as the integrity of our models, extremely, extremely seriously. These are important decisions, these are important things that we're doing. The quality, the data quality, and then the model quality and the performance at scale in production is something that we take very seriously.
Demetrios [00:32:04]: And for the performance side, I often wonder about this, like if you're using transformer models or LLMs, how are you making sure that there's not hallucinations happening? And because it seems like you have to be very confident of the accuracy. And sometimes with transformer models, they can just say whatever they want, especially if they don't know or they've never seen this particular type of hate speech. So is there, is that where you're talking about, like, you're constantly monitoring for the drift to make sure that whatever you've labeled, you're double checking and seeing if it is true. If it's not, how does that break down?
Matar Haller [00:32:51]: Yeah, so with any model, we want to look at the functionalities. And so, like, if there's a new type of hate speech that we didn't know before, then the policy manager will say, hey, there's this new functionality that we have to make sure that we cover and we can catch it proactively. If we don't catch it practically, because suddenly there's a new slang term or a new style or everything is the same in the hate speech world, but the data is a type of data that we've never seen before, like food reviews or book reviews or whatever, and for whatever reason, we didn't test on that data and suddenly we fall apart. And so that's why we're constantly auditing and looking and improving and we're retraining a lot to ensure that we're sort of non drifting and that our performance stays high. In terms of LLMs, we're not using currently LLMs for inference because at the scale and at the cost that we need to perform at, it just doesn't make sense. Right. We need to make sure that our clients and us, we don't lose an arm and a leg in terms of like being able to predict and to give value at such a high scale. And so we use LLMs intensively beforehand, but at prediction time, we need much more robust solutions.
Demetrios [00:34:07]: Yeah, that makes sense. And it's totally like it's what you would expect almost, right? You don't because the LLMs are a much slower and be a little bit less trustworthy on the output. They can hallucinate or do whatever. I would hope that either you knew some kind of trick and you had some special magic pill or silver bullet, or you're doing that, which totally makes sense. Now, the auditing process for me feels like something that is very rigorous. Can you go into that a little bit more and what the whole audit looks like and how then you make sure to like continuously stay at a very high accuracy.
Matar Haller [00:34:58]: Yeah. Um, so in addition, so even before the auditing, but in addition to the functional tests they talk about, which I think are like really, really critical, um, we also do other types of tests. So we have a go no go test, um, which basically what we say is this is like a set of data that we can't afford to mess up on, right? Like these terms are always positive and these terms are always negative. And if we fail on them, then that's a no go for the model, right. This is like a data set that basically is like go no go. Can this model go to production based on these? Because I can never say have like hello world or I love you, be hate speech. Like that won't work. Or hi, there's things that are just.
Matar Haller [00:35:38]: And so there's that. Um, and um, we also have a false positive rate test. So what we do is we'll take our model and we'll run it on data that is supposed to be completely benign and also on data that's like borderline. Um, and then we can see like what is our false positive rate. Um, so for example, for hate speech, we can run it on, like on Harry Potter or on some book for a hate speech text. And there shouldn't be any hate speech in there. And if there is, then we can analyze the situations where it did get flagged. Like, what are these false positives? And then understand our model a bit better and understand, okay, like why is it flagging this? And what can I do? And sort of retrain again for child pornography or child abuse, we can run it, you know, for, for the benign data.
Matar Haller [00:36:20]: We can just run it on like Google open set, open image. Like, you know, just like a dump of images or whatever. And then, like, make sure that, like, you don't get, you know, a positive score on, like, a picture of a keyboard or whatever. And then, but we can also run it on images that are, like, maybe that are like, a little trickier for the model. Like sports Illustrated, women in swimsuits, mothers kissing babies, breastfeeding, people on the beach, toddlers in diapers, things that are not csun, but they do have bare bodied and children, children on a playground, things like that. And then you want to make sure that none of those are flagged either. And they're sort of more towards the border and more difficult for the model. And so these are all things that we do, like, you know, pre production or out or like before, you know, after retraining to ensure that we're, like, still, like, at keeping our quality high in terms of, like, once we're in production, then, like I said, we can do a couple things.
Matar Haller [00:37:17]: We can. Or a few things. We can just look at our distribution of scores, right, and sort of start to notice, like, are we drifting? Like, our score is suddenly, like, much, much lower or much, much higher? Because probably, like, at the scales that we're talking about, the world isn't suddenly becoming really friendly, right? Like, people are still being mean. People are things. So if words are changing, there's something going on.
Demetrios [00:37:37]: As much as we would like the world to be becoming more friendly, that's usually not the answer.
Matar Haller [00:37:43]: And also we have, like, we'll do audit specifically targeted for customers. Like, let's look at, like, really, really high scores. Let's look at a little bit of scores. The middle. Let's sort of look, you know, the hardest is to find false negatives, but we can also sample within the din and be like, okay, things with this keyword or this sort of things. Like, let's look at all of them and see what the distribution of scores were. And are these negative ones really negative? For example, we used to have something. One thing that we look at is token overfitting.
Matar Haller [00:38:13]: So I like black coffee or I hate black coffee, or I love chinese food, or I hate chinese food. We want to make sure that those aren't being flagged. And if they are, then we need to find ways to combat that. And we can look for that also in production data to make sure that our functional tests are still valid in production.
Demetrios [00:38:37]: Okay. And then you have the audits that you'll go through. And there's the false positive, there's the go no go. There's the functional tests. And then once it's out in the wild, you're just looking at it and you're seeing, did we overfit for some things? Let's look at this distribution score. Let's see where we're at. Do you also have integration tests and, like, unit tests and these kind of things?
Matar Haller [00:39:00]: Yes. Yes. Yes. Sorry. To me, that goes without saying. That's, like, before. Yes, of course.
Demetrios [00:39:05]: Okay, cool.
Matar Haller [00:39:06]: Yes, we do all that. We add end to end tests. We also, before our model hits production, we do load tests. We want to make sure that we're still able to, like that we haven't changed anything so that we can still meet the load of, we look at the price, like what, what instances it's on. So we have a lot of, like, heavy engineering work that we do before a model, like, ever sees the light of day. And also the functional tests and the gonodo tests, they're also run on production data. Right? Like, while the model is out in the wild, like, just, you know, it's not, they're not only done, like, when we've been trained, right. They should be done constantly.
Matar Haller [00:39:40]: And also the audits is that we have our policy managers and we have analysts that are going in and sampling data and looking at, and looking at the data and saying, like, is this a false positive? They'll look at the high scores and say, okay, like, this is a mistake. This is a mistake. And then they go and they sort of summarize it for us and say, hey, guess what? Every time someone talks about food, you're flagging it. I keep bringing up food because it was like the first big fiasco that we had.
Demetrios [00:40:03]: So the process that I would love for you to walk me through is, how does it look like when the data scientist has whatever hate speech model one that they're going to be using, very specific, whatever it may be, and they're like, this is good. I'm ready to bring this to production. I'm ready to. Are you throwing it over the fence, giving it to somebody? Do they run with it? How does that look to take it from the potentially Jupyter notebook if they're playing in that and then now being served as an API at very low latency?
Matar Haller [00:40:47]: So what we have is we serve our models, we use kubernetes, we're on AWS. And our, but basically our inference pipeline, our inference infrastructure is like, is we, we built it ourselves. We were looking at all kinds of different vendors, and we decided that in order to really keep costs low. And at this scale, we didn't want to sort of pan overhead for every inference. It just didn't make sense. And so we were also able basically, to write, at least for our text models right now. And this is something that we're still working on, on this infrastructure, basically to have sort of like a no code deployment workflow for our data scientists. So once they've for like, architectures that we're like that we know and have been load tested and we're very comfortable with, there's like a very easy path for them to basically just deploy to production.
Matar Haller [00:41:43]: And the data scientists, they do that. I strongly believe that people should write code for production, including data scientists. I think that sort of like, if your output is a python notebook, then you lose a bit of the ownership. And even though the data scientists are not the ones usually waking up at night for on calls. Right. That's like the engineers, but it's theirs. They own it, they know it, they're familiar with it. It's part of their ownership.
Matar Haller [00:42:10]: And so it's not that they sort of like just do the research and then kind of like kick it over and say, hey, someone else needs to take this.
Demetrios [00:42:18]: Yeah, you're preaching to the choir. The production code, we actually have a channel in our mlops community, slack, that is called production code, and it is 100% that. Just like talking about how we can make sure that we're writing more and better code, production code, especially those who are data science scientists or have data science backgrounds. And understanding the benefits of that will take you so far.
Matar Haller [00:42:48]: Absolutely. Yep. So the Ops team is very focused on writing the infrastructure so that that can happen seamlessly, but the ownership and put and pushing it, there needs to be, needs to lie also with the data scientists.
Demetrios [00:43:04]: Yeah, it's fascinating, too, to think about, okay, you created almost like this no code solution, so that it eliminates the friction that the data scientists have once they've, once they have the confidence that, like, all right, this model is going to be better, and if passed all the checks that I needed to pass, then they can push it and say, all right, cool, now let's go. And they have that ownership, they can take it across the finish line.
Matar Haller [00:43:34]: I will say that it's not completely seamless, it's not completely smooth. We're constantly learning, like, we're still building. We're a startup. But yes, that part works.
Demetrios [00:43:43]: Yeah, there's other parts.
Matar Haller [00:43:45]: Yeah.
Demetrios [00:43:46]: That are fun and that you're probably digging into. And I can imagine, I can imagine a ton. So there is so much good stuff that we talked about here. I think the thing for me, that is one of the hardest problems that you're dealing with is a ton of languages, a ton of cultural references, trying to make sure that you are not just, like, taking all the fun out of the Internet, because there I, as you mentioned, you could overfit, and now somebody says, hello, world, and boom, that's flagged.
Matar Haller [00:44:28]: Yeah.
Demetrios [00:44:28]: And so how can you find that proper medium that is nothing. Taking the fun out of it and just flagging everything, but also not being too lenient and not flagging the things that definitely should be flagged.
Matar Haller [00:44:44]: Yeah. So, I mean, this is a well known business problem, right, where you have sort of this balance between precision and recall, and a lot of times it's also, it's a client question, right? Like, how sensitive are you? So if you're a child's platform, then you probably want to err on the side of, like, high recall, right. And even at the cost of precision. And you'd want to, like, over flag and, yeah, take some of the fun out, because at the end of the day, I don't care if they're limited to, like, twelve words. They can type. Like, that's fine. At least there's fake. Versus.
Matar Haller [00:45:13]: If you're a dating platform, you're going to be a little bit more tolerant of, like, you know, some. A little bit more exciting talk. And so it's a business question. We have our policy, and, like, when we building a model, like I said, we're, like, very, very clear about our policy. The policy manager is, like, works very closely with our data scientists. He's at all of their dailies. Anytime there's a question, there's, like, it's an ongoing conversation, because sometimes the policy will say something, and then we'll be like, yeah, but what about this example? Like, is this, is this not. And the labelers are always asking, and we're always asking.
Matar Haller [00:45:46]: So it's sort of this, like, constant conversation to make sure that at least in terms of our policy, our data and our data quality, our labeling is, like, very, very high quality. And that's also something that we can, that we, you know, um, communicates the client. Like, this is our policy. If you're more sensitive, then you can add a keyword, right? So if, for example, our hate speech policy, reclaimed slurs, are not hate speech. So if I'm saying, like, I'm proud to be a. Then that's not hate speech, it's reclaiming, right? Or don't call me a, that's not hate speech. That's, like, victim, like, you know, asserting themselves. But for some platforms, any slur is not okay.
Matar Haller [00:46:24]: Like, I don't want you to use that word at all. And so that's, that has nothing, like, my model will not, was not going to be, like, tuned to that particular, you know, sensitive level. And so for that, we have our keyword tool. Feel free. Add the keyword in, describe how it is, and then just add it to your model. And then, like, that'll, that'll be the right sensitivity level. And then if you want, you can take all the fun out that way. I have a, some level of fun.
Matar Haller [00:46:49]: Um, and so it's, again, and then also clients can set their own threshold, right? So I give the probability of risk. And then if you're, like, very, very, very sensitive and you don't want anything, you'll set that threshold really, really low, and then you'll make sure that anything that's, like, at a, even a little bit probable of risk will get flagged. And if you're not sensitive, then just set your threshold really high and go have all the fun you want.
Demetrios [00:47:13]: Yeah. Well, it does feel like the main thing that you're probably doing continuously is labeling data. Is that safe to say? Like, you are you or the teams, I would imagine. Like, it feels like 90% of this happens at the data labeling phase to make sure that whatever goes through the pipeline and is then on, the output gets flagged or is, is caught. I.
Matar Haller [00:47:41]: So, yeah, so I think there's two things that make our solution our models, or three things that make our solutions, our models, so powerful. The first is really the subject matter experts and the intelligence analysts and the fact that we, we really know this world, right? Like, we know how to build a policy. We know what it means to be hate speech, what it means to be harassment, bullying, what it means to be suicide, self harm. Like, we know this really well and.
Demetrios [00:48:03]: In different languages, right, and different cultures.
Matar Haller [00:48:05]: Yes, many. And we also have cultural context. So someone calling someone a dog in one language is not the same as calling them a dog in a different language. And so we're sensitive to that and we know how to do that. And what that means is that our data labeling quality is, our data quality is very, very, very high because it's so linked to our intelligence analysts and to our policies. And we're also very aware that, like, that's what gives us an edge, right? Like, our data is our gold, right? Like, this is what like, we've invested a lot of this, and this is what it's, you know, it's what our models are built on. And then the other part of that is that we just have killer engineers and killer data scientists that are able to take this, you know, this data and turn it into something that's able to make a real impact in the world at scale.
Demetrios [00:48:50]: There's. It's two parts of the puzzle, because you can have all the data in the world that is very high quality data. But if you can't operationalize that with a machine learning model, then it's as good as nothing.
Matar Haller [00:49:03]: Right, right. So that's like, the raw materials. We need to know what to do with raw materials. And that's why also I said that there's, like, a lot of open source models out there, and oftentimes they fall apart of the data. Right. Like, they can throw, like, a really big, cool model at something and then it won't run at scale, it'll fall in production. And also it's based on poorly labeled data or inconsistent labeled data. And then it doesn't matter, like, how amazing the algorithm is.
Matar Haller [00:49:23]: It makes sense.
Demetrios [00:49:24]: Yeah, well, yeah, and it seems like the other piece, for better or worse. Like, I. I can imagine it is hard being some of these subject matter experts because you have to go into the depths of the worst parts of the Internet.
Matar Haller [00:49:40]: Yes. So we take the well being of our labelers and of our experts very, very seriously. We have a psychologist on staff. We have well being programs. This is something that's not taken lightly. Like I said, we care a lot about this. This is another reason putting AI in the loop here. It's not only because it's the only way to combat this.
Matar Haller [00:50:06]: There's the sheer scale of things you can't do manually, but it's also because we want to offload and focus human energy only on where it's going to be most important and most effective.
Demetrios [00:50:20]: Yeah. The questions and that burden, it makes a lot of sense. So incredible. Thank you so much for coming on here and talking to me about this. I am thoroughly fascinated by it, and I think you're doing so much great work. And as a father of two daughters, I know I told you this before, but I'll tell you it now that we're recording. I am so grateful that people like you and active fence existential, because it is just the. I know the Internet isn't going to always be like 100% safe, but it does feel like at least you're trying to do something to make it a little bit safer.
Matar Haller [00:51:02]: Thank you. It was great being here. I was really excited to get to speak to you and thank you for inviting me, and I hope I get to keep doing great work. Important. Excellent.