AI Powered Digital Twins of Real Organizations- Aspiration and Reality // Hala Nelson
speaker
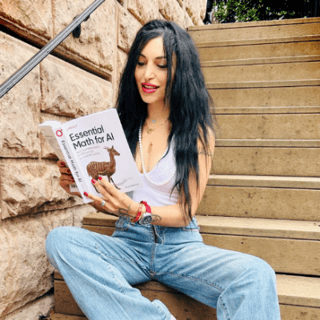
Hala Nelson is a professor of mathematics at James Madison University and the author of two books: Essential Math for AI (O'Reilly 2023) and AI Powered Digital Twins (Wiley 2026). She specializes in mathematical modeling, AI, data strategy, and digital twins. Hala consults for the Department of Defense, Department of State, and the emergency and infrastructure services in the public sector. Her expertise lies at the intersection of mathematical modeling, data, AI, Digital Twins, industrial and military applications, and data and AI strategy and governance. She serves as a link between government, industry, military, and academia.
SUMMARY
Because historically Data and AI have evolved within relatively separate communities, their capabilities, benefits, and adoption strategies are valued differently by different work teams and investment decision makers. Many of us are currently attempting to harness the power of AI technologies at large complex organizations, or small ones for that matter. Initiatives span across a wide range of interests within an organization: AI specialists, data engineers, IT departments, strategists, ethicists, executives, and the people on the ground. How does an implementation team guide a 32,000 person institution to optimally adopt AI within the very short time attention span of an executive who wants an immediate return on investment? Is striking a deal with Microsoft Co-Pilot or OpenAI enough? Can resources be justified for the ambitious goal to create a digital twin of the processes and systems of an entire organization where AI can be applied to drive efficiencies and improvements? Are the required technologies, expertise, and resources available? In this talk I will present my experience on what it takes to move from aspiration to an implementable reality- from math to data to strategy to people to everything in between. We’ll also try to answer the question on everyone’s mind: will we eventually succeed, or will all our efforts end up in the wasteland of failed projects, efforts, funding, and time?
TRANSCRIPT
Adam Becker [00:00:00]: Hala. Good to see you.
Hala Nelson [00:00:08]: Hello. Good to see you.
Adam Becker [00:00:11]: I have been stoked for your talk. Is it true that you're almost. Are you writing a. You're writing a book, right?
Hala Nelson [00:00:18]: I'm writing my second book, yeah.
Adam Becker [00:00:20]: Your second book?
Hala Nelson [00:00:21]: My first book, Essential Math for AI and that's how I ended up in these spaces. And now I'm writing AI Powered Digital.
Adam Becker [00:00:29]: Twins, which I think brings us very nicely to your talk for today. To be honest, I was searching for the book. I wanted to either get it or to sign up to hear about it. I haven't found the link yet, but it sounds fascinating. So once it's ready, let us know.
Hala Nelson [00:00:47]: Yes. The new one. You mean the new one.
Adam Becker [00:00:50]: The new one, yes.
Hala Nelson [00:00:51]: It's being Gutenberg. It's, it's process.
Adam Becker [00:00:56]: It's. It's still in the process, Yeah. I believe you have, you have slides to share. I'm just gonna pull them up right now.
Hala Nelson [00:01:06]: Yeah. Can you see my slides? I share it from my laptop.
Adam Becker [00:01:11]: Are these yours? I think these are yours. Digital twins.
Hala Nelson [00:01:17]: Correct. But you share. Did you share them from yours or from mine?
Adam Becker [00:01:22]: From yours.
Hala Nelson [00:01:24]: From my computer, yeah. Okay, so I'm gonna do this now.
Adam Becker [00:01:29]: Awesome. I'll be back in 20 minutes again chat, Write questions in the chat and I'll be back soon. Thank you very much. Hala, take it away.
Hala Nelson [00:01:42]: Thank you. Thank you everybody for coming. I'm gonna talk about AI powered Digital Twins, but I'm going to focus on digital twinning of real world organizations and I'll say why as we progress through the talk. So I am a math professor. I wrote a book, Essential Math for AI with Ill Media two years ago and I did not know at the time that we were going to the AI field was going to blow up as much as it did because generative AI succeeded right there and then. And then I ended up being on all sorts of committees, task forces. I consult for Department of Defense, Department of, of State. I, I'm.
Hala Nelson [00:02:31]: I find myself always at the intersection of academia, industry, military and government. So this is a pretty unique. Gives me a unique space and vantage point. And so I'm gonna tell you what I've observed as organizations are trying to deploy AI into their. To move from prototype and development and proof of concept into a large scale organizational deployment. But first I'm going to talk about the elephant in the room. We are. Is AI going to take our jobs? The answer, my first answer is no.
Hala Nelson [00:03:21]: We are understaffed and under resourced and we can be aided by automation. For example, we don't have enough truck drivers. So autonomous trucks can help us fill the gap. There's a lot of gaps in our resources and we need to fill them up. AI does increase productivity and satisfaction of employees. I think all of us are using AI in one capacity or the other nowadays. However, yes, AI will completely replace employees whose work is solely task oriented. If you are doing, if your job is doing one task that can be automated, you are going to, your job is going to be replaced.
Hala Nelson [00:04:04]: So also I want to acknowledge that there are way too many tools, too many tools, too many. You don't know what will work for your organization. So what we need is what organizations need are technology, communication, partner who will know their business, know what tools and your current landscape and what's your current infrastructure and whether it needs to be replaced and design solutions, look for platforms to help you suit your needs. So basically you do need. Because of the hype, because of the market that's flooded with all kinds of stuff that promise to solve anything, there is a big confusion and hesitation and security concerns out there in the spaces that I've seen. There's also the aspiration versus reality in terms of deploying AI into the organization. The aspiration is of course everything is automated, efficient, secure and ethical. The reality, and this is a reality that we work in and this is not a rant and we have to design strategies and deployment and implementation routines that work with the reality.
Hala Nelson [00:05:27]: So the realities include the people are culture and culture. There's turf issues. If you have an AI deployment team and they usually need to work across multiple deployment come from multiple backgrounds and expertise. Who does this new body report to? I mean this is, this has been a hurdle for, for large scale organizational adoption. There's always policy and regulations. This change the technologies that, that the AI is going to pull information from AI incompatible, they don't work together. There's in interoperability issues and of course there's hype and you don't know what, what can be accomplished. What not those.
Hala Nelson [00:06:19]: Sometimes the administration in the organization that's trying to do these changes and then the whole priority, the whole AI initiative goes into the back stage and then what do you do in that case? And also people who come on AI deployment teams, they are trained differently. Even if there could be software engineers, they could be AI specialists, they could be strategists, they could be so, so even, even the technical training itself is different because you're, you're, are you talking, are you software engineer? Are you computer scientist? Like what, what all of These have to work together in the AI age, while traditionally they operated in silos. And of course there's insufficient resources and that's what it's a state. So, so what, what we find ourselves operating in nowadays is, is we have a 500 billion Stargate Initiative and I'm going to talk more about digital twins in a second. The current industry size is 11.37 billion, but is projected to grow into 137 billion by 2030. We have a lot of security concerns, so we have sovereign AI and data center initiatives and we have national energy emergency to how are we going to power the data centers that will satisfy the demand? And, and of course there's, there's all kinds of data and AI acts and executive orders that are being rolled out and we have to comply even as we develop the technology. So this is a landscape we operate in. But because I also exist in the cyberspace and the cyber policymaking space, I have to say that there's more than that.
Hala Nelson [00:08:15]: There's competition and AI race, there's competition for the markets and there's national security concerns. And the AI race could not, AI age could not highlight that more. Of course, like you saw what happened with the markets when China's deep SEQ was rolled out. It's open source, cheaper model, smaller model. And of course like, okay, we have to assess civil capabilities, we have to address security concerns at all layers. It depends what organization you're deploying into your. And what kind of, Is it a highly regulated industry, is it highly secure industry? But then what that taught us is smaller and cheaper models are the goal for us and we're nowhere near that. And I'm a mathematician at heart.
Hala Nelson [00:09:07]: I do actually my specialties in optimization. So just looking at the whole AI supply chain, which we will, you discover that there's opportunities for optimization at all layers and we can do better. And of course we were thinking nowadays of edge compute and wearable devices, AI. So the idea today is to move from pilots and prototypes and overcome growth challenges, deliver value to real organizations. This is an AI humanoid that I met yesterday at this here in Las Vegas. It was pretty human like. And this is a prototype. And soon I think if we optimize, if we deploy at scale, I think we'll be seeing a lot of all kinds of AI, whether software or robots, walking around us doing all kinds of stuff.
Hala Nelson [00:10:10]: Here's the AI supply chain which when we look at it in its wholesome shape, we can optimize. We can optimize hopefully at all levels. There's data models, hardware. There's the communication channels and networks, data centers and energy and power supplies. This is how you do AI. And of course there's a system design layer, the data engineering layer. Engineering in policy, regulation. I'll talk about the standard of care in a bit.
Hala Nelson [00:10:45]: But, but to optimize and fulfill the technology potential, all these layers must coordinate with each other. So what we need is all of these have operated in silos throughout. But to actually deploy AI, we have to remove these silos. So we have to have experts at all kinds of intersections here and perhaps experts that kind of know what happens at the full stack. What changed in the past two years? The data engineering has matured. Generative AI has removed access barriers. It enables adoption at scale. Anyone can even deploy an AI agent now to do all kinds of stuff using generative AI.
Hala Nelson [00:11:31]: So it's an AI agent using generative AI, which is insane. But this also opens the road for security concerns because if a lot of people can do stuff, then a lot of people can do good stuff, but also a lot of people can do bad stuff. So it's, it's an imperative for an engineering standard of care. And also the massive investment in AI have, have enabled a lot of people to want to do this and of course the potential. And we have public private partnership that will enable things to keep going. But what also changed is from an engineering perspective, before we had data sources, they would, the data would go to data to data warehouses and data lakes or data lakes or data lake houses. And then in the process of transforming, loading, et cetera, it would lose context. And of course, and there was also, when you wanted to apply this to whole enterprise, there's always modeling conflicts.
Hala Nelson [00:12:38]: How do you model the enterprise data? What's changed now with the AI agents they plug directly into hundreds data sources at their, at their, at the source. So, so agents can generate, execute action plan trigger alerts. Right? So, so it's kind of taking advantage of the reality on the ground, the lay of the land. The, the enterprises are not mon monolithic. The reality is decentralized. And AI, by plugging directly into these data sources, hundreds of them is able to work with this reality. So we have thousands of AI agents are being deployed to get all kinds of tasks. And this is for those of you are going to get the slides later.
Hala Nelson [00:13:27]: I put my definition from my original book of what an AI agent is. Everybody asks, but I saw today really good talks on how AI agents work, the engineering background. All right, so what we are headed toward is Building digital twins of systems, buildings, organizations, processes. You can twin anything you want because we do have this engineering infrastructure. But with that we have two imperatives. The imperative for engineering standard of care and the imperative for end to end native AI approach. So first, for those of you who are wondering what a digital twin is, it's exactly what it says. It's like you have a physical object or a, a biological object like a cell, a human or a process like that is something happens in your organization, someone places an order and then what happens afterwards to fulfill that order.
Hala Nelson [00:14:27]: That is a process. So a digital twin is a description is a digital saving of that. It could be a dashboard digital rendering of that process or object. But, but, but what I will of what AI is when AI powers a digital twin, it moves it from being a static digital representation into a representation that you can manipulate that you can do test with that you can even blend it with your physical environment. You can ask something of the digital twin and then the physical object response, which we've had all of that before for except that with AI it's it. It can do more complex tasks, more realistic and also more dangerous engineering standard of CAG because of the scale at which AI can do good or harm. I mean it. You can, you can for example think of our critical infrastructure.
Hala Nelson [00:15:32]: You can attack which has happened attack our water lines using just by a stroke of a keyboard. We have to build an engineering standard of care. And I'm not saying standard of care in general. I'm not to to think about the ethics of AI. That's a huge big philosophical questions and people ethics are different than each other. What's ethical to one group might be not to another but however when you do engineering standard of care, it's more focused and you think about engineers when they're building actual buildings, they follow standard of care imperative. So we don't have that for our digital systems or digital twins. And this is the time for us to develop the standard of care.
Hala Nelson [00:16:21]: So there's also an imperative for an end to end AI native approach. Now we have the ability now to come to this field from an AI native approach. Not, not like our training in the past. However, before we do that we need to how. How are we going to devise models that we can base explainable decisions on while we don't have full mathematical clarity about what we can conclude from adding more data and computation what we can so we do not have like the, the actual. If you add more compute and add more data, are you getting better results? Maybe it's better to engineer the data and instead of putting models on top of unanchored data, it's better to actually engineer the data and think about the process. You want to model what data goes to it and how you're going to use that before you actually put more bad data on even bigger models. So my point is we have a lot of optimization opportunities and this end to end AI native approach can pave the way to more powerful small languages, language models on smaller devices and more optimized AI workflows.
Hala Nelson [00:17:44]: For the sake of time, I'm going to skip this and I'm going to say that most organizations I've noticed I have absolutely no strategy to deploy AI at a large scale. They are still in pilot phase and so this is a strategy to overcome growth challenges. You can take a picture of this slide. I will skip to an example because I started a bit behind time and I will hopefully finish right on time. When you build, you want to build for replaceability because the technology changes rapidly. The back end users, the change in the back end should be as simple as plug and unplug. Users don't care for users only care for addressing their pain points, automating their own workflows and increasing their productivity. They don't care what's behind the scenes powering their models and what data stack happens to be in the background.
Hala Nelson [00:18:50]: And they also care about the bill, who shoulders the dollars. That should be a huge part of your strategy that is here that I just will give you in the slides. So at the organization the easiest step is to popularize the individual use of your AI tools. And then more work is to create the resources and training resources for your pilots. Approve AI that actually saves a lot of hours. We just saw a talk about exactly that. The hard work but worth it is to model and document the institutions processes, operational administrative processes. That's the hard hard work.
Hala Nelson [00:19:31]: And I will see you, I will show you. The take home lesson is the business process guides the data model and not the other way around. You don't data model your organization and then try to deploy AI, you actually model. You start with your business processes that are your most pain points that you want to automate and then you pull in the data. And this is an AI native approach that's different than before. So where to start? Choose one specific administrative process. I have one use case succeed. Then assign each department, then assign each department should have an AI IT consultant that works with the knowledge, with the people who are who are not technical but they know exactly what's the right answer? How to train this and then scale.
Hala Nelson [00:20:23]: Each office identifies and prioritizes use cases. So here's an example of business process. A lot of people were losing employees on that. The small purchase credit card in a large institution, it had to go through so many departments. Training employees is very, very difficult for something like that or a reimbursement. Again, there's so many involved processes. So once you document all the involved processes that go into these, you pull the data for it. However, I have seen three approaches in terms of how to model a business process and deploy AI successfully on it.
Hala Nelson [00:21:06]: One bad approach that I've seen is you just throw the data in a knowledge cloud and then you deploy a language model on top of it. This is a bad approach because the data, any organization is always constantly moving and shifting. The regulations change who's going update the knowledge cloud and how is it always going to be close to the truthful. It's better to keep the data where it belongs, okay? Where it is closest to the source of truth as possible. However, you have in that case security and access controls who can access this data and then you can throw AI agent on top of it. So again, this is a better approach. However, it gives unguided answers because you're just deploying a language model or a generative AI or an agent on top of that that's out there that the agent deciding which data I would need. Actually the best approach I've seen so far is faithful to the business process as the connective tissue for your purposes in your organization.
Hala Nelson [00:22:10]: The business processes are the connective tissue between all the different data sources. So actually that is where you start. You model the business process as an executable graph or a flow chart with all the involved components and then you keep the required data where it is. With security and access controls. You only create virtual data schemas and you throw an AI agent on top of it. So that approach has been that I've seen has been the most faithful to the most rewarding, the most, the better that has has fewer problems. So I am going to say, I'm gonna stop and I'm gonna say this is a big hump. This is an exciting and hum.
Hala Nelson [00:22:59]: The biggest lesson I've learned is this is an exciting and humbling world. Mathematical modeling, that's my background is a superpower. But neither the superpower of math or I AI models or good foundation and whatever engineering you're doing that, that's not enough. We have people, regulations, administrations, we have to work with the reality we have to keep flooding and. Yeah, I'm gonna stop now and I will give you the slides. I skip a lot of words, but I tend to be more worthy. That's why I'm a writer.
Adam Becker [00:23:37]: Hala, this was packed with insight and I think Jacques has a thing to say about it. Let me try to pull him in.
Hala Nelson [00:23:51]: That's.
Adam Becker [00:23:52]: That's my feeling exactly.
Hala Nelson [00:23:54]: Thank you.
Adam Becker [00:23:56]: It's packed with ideas. Honestly, like we don't have time to just to dive much deeper, but I would love to. We should find some time. How do people connect with you? Because I feel like, you know, just, just this idea of building or almost using as a lens AI to parse through business process and then being AI first in the manner in which we parse the business processes and then onboarding them slowly as opposed to just like a massive kind of transformation that starts with what our technology can do. Instead, let's just figure out what the actual bottlenecks are and what the challenges are and then work backwards. That feels to me what you're saying.
Hala Nelson [00:24:35]: Right, that's exactly what I'm saying. And that's the opposite, the exact opposite of what's been happening historically and taking years to deploy. And even when it deploys, it doesn't serve the. What it's intended because of AI. And because AI is able to interact with data sources, with all modalities and from all places, through API, through agents, etc, we're able to reverse the process and have the business process as a connective and the. Basically the engineering baseline and then build on that and then you start small and then people are going to. The departments themselves are going to guide who, which cases they're going to model first. And then it really removes a lot of bottlenecks, creates excitement.
Hala Nelson [00:25:21]: Yeah.
Adam Becker [00:25:23]: Thank you very much. This is incredibly wise. So I think this. I hope folks will tune in for the Digital Twins book. Yes, best of luck with that.
Hala Nelson [00:25:35]: Thank you. Thank you.
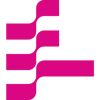