Data Quality = Quality AI
speakers
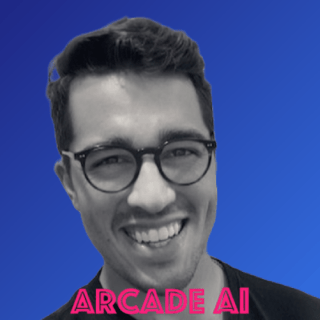
Sam Partee is the CTO and Co-Founder of Arcade AI. Previously a Principal Engineer leading the Applied AI team at Redis, Sam led the effort in creating the ecosystem around Redis as a vector database. He is a contributor to multiple OSS projects including Langchain, DeterminedAI, and Chapel amongst others. While at Cray/HPE he created the SmartSim AI framework and published research in applications of AI to climate models.

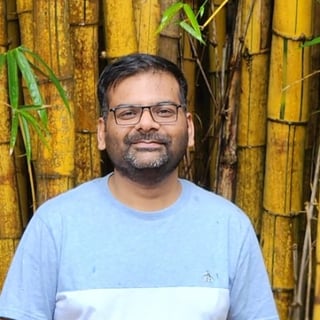
Pushkar is a Machine Learning and Artificial Engineer working as a Team Lead at Clari in the San Francisco Bay Area. He has more than a decade of experience working in the field of Engineering. Pushkar's specialization lies around building ML Models and building Platforms for training and deploying models.
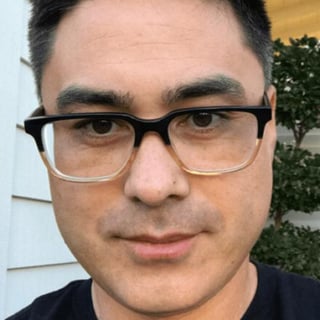
Joe Reis, a "recovering data scientist" with 20 years in the data industry, is the co-author of the best-selling O'Reilly book, "Fundamentals of Data Engineering." His extensive experience encompasses data engineering, data architecture, machine learning, and more. Joe regularly keynotes major data conferences globally, advises and invests in innovative data product companies, and hosts the popular data podcasts "The Monday Morning Data Chat" and "The Joe Reis Show." In his free time, Joe is dedicated to writing new books and brainstorming ideas to advance the data industry.
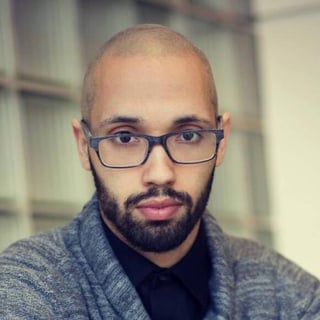
Chad Sanderson, CEO of Gable.ai, is a prominent figure in the data tech industry, having held key data positions at leading companies such as Convoy, Microsoft, Sephora, Subway, and Oracle. He is also the author of the upcoming O'Reilly book, "Data Contracts” and writes about the future of data infrastructure, modeling, and contracts in his newsletter “Data Products.”
SUMMARY
Data is the foundation of AI. To ensure AI performs as expected, high-quality data is essential. In this panel discussion, Chad, Maria, Joe, and Pushkar will explore strategies for obtaining and maintaining high-quality data, as well as common pitfalls to avoid when using data for AI models.
TRANSCRIPT
Sam Partee [00:00:10]: So, hey, everybody, this is data quality equals AI quality. And today you have four of the best people in the space to be able to talk to you about this. We had conversations beforehand about this, and I got to tell you, they have some of the best opinions that I've heard in the space, a space I've been in for probably close to a decade now. And so this is a treat in the sense that you get to hear all four of them at once, which is really awesome. So, to blanket start this, we're just going to start with what data quality means to you and what the definition of that actually is in its applications to AI quality. So, Maria, I'll start with you.
Maria Zhang [00:00:50]: All right. Hello, everybody. Thank you for coming. And it's a great question. What is data quality? It means different things to different people in different scenarios. I actually want to state a failure case. I'm sure you've heard of this expression, garbage in, garbage out. So that's the situation I absolutely want to avoid.
Maria Zhang [00:01:14]: And so that starts with data quality.
Pushkar Garg [00:01:21]: Yeah. So data quality, for me, it looks like it needs to be available on certain metrics, and then those metrics need to be monitored, and then if you're able to monitor on a certain metrics, like data completeness, data accuracy, data validity, timeliness of the data, that's sort of really important into building a good monitoring structure, and then. Yeah.
Sam Partee [00:01:55]: Joe, let me ask you, what happens when you ignore data quality?
Joe Reis [00:01:59]: You get the current state of affairs in most companies.
Sam Partee [00:02:08]: I love it. Chad, What happens when you ignore data quality?
Chad Sanderson [00:02:15]: Yeah, hard for me to say anything much different than, Joe. I think there's a few things that start to happen. The big one is that you start to run into this issue at scale, where it becomes very, very difficult to understand why models are failing, why dashboards are showing the wrong information, why our AI program is not taking off the ground. One of the really common indicators of data quality not being implemented from the very beginning, or at least early enough, is that incidents are happening. And then when you go to an executive sponsor within the company and say, hey, we should fix this, they say, show me the ROI of investing in data quality. That's how you know that something has probably broken down at some point.
Joe Reis [00:03:01]: Can I ask the audience a question here? Who works at a company, how many of you feel like your data is pristine and awesome and okay, otherwise you wouldn't really be.
Sam Partee [00:03:15]: It's not a lot of hands.
Joe Reis [00:03:18]: Yeah, just checking. Thank you.
Sam Partee [00:03:20]: Thank you for the anecdote, Joe. I want to branch off that actually because what you said about ROI, I've worked on a lot of predictive projects where it was easy to measure that, right? You have some regression score or something that, or like time to value, or even in today's world, time to first token, where it's easy to say, the faster that this gets to the user, the faster they get a recommendation, the higher our ROI. But the infrastructure is different in today's world, given that we are now with the generative kind of generation. To be a little punny there, Pushkar, what do you think about that?
Pushkar Garg [00:03:54]: Yeah, I think it's, first of all, most important to be able to bring all of the structured and unstructured data into one place, data lake, lake house, so that you can build some sort of monitoring on everything together. And then I see data quality in, like, two perspectives. One is the data world, and then the second is the ML world. So all the ingestion transformation that happens, I see it lying in the data world, and then once they are converted into features, how do we use. To use them to build models and then do predictions that sort of, like, lies in the. In the ML world, in my perspective. So building, like a data lake where we can get everything together, and then defining a set of metrics that work well for the structured data and then some. Some of them also work for the unstructured data, like data validity, data completeness, timeliness, all those kind of things, which.
Pushkar Garg [00:04:57]: Yeah, so I think that that's really important.
Sam Partee [00:05:01]: Absolutely. I'm curious, does anybody here have a horror story about data quality that they can tell something where, I don't know, there was a mismatched schema or something didn't work right. Or maybe a migration or something. All right, Chad, I see you over there. Come on, tell us.
Chad Sanderson [00:05:17]: Yeah, I mean, I probably have. I could write pages worth of data quality issues that I've encountered in my life of all different varieties and sorts. So I can tell two very quickly. So I used to work at a freight tech company called Convoy that, unfortunately for the world, no longer exists. Thank you, Covid. But we are very heavily model driven, and we had a couple models that were incredibly important to the business, and one of them was our pricing model. And the way that the pricing model worked is that it would essentially, based on data we were getting from our freight marketplace, which was an auction, it would predict a range. It would say, this is what we think the ceiling of this particular shipment is going to go for in an auction, and this is what we think the floor is going to be.
Chad Sanderson [00:06:04]: And then, depending on that range, would say, well, is this within margin or sort of out of band for margin for us? So that model was really, really important. Otherwise, we'd be bringing shipments into our marketplace, and we'd actively lose money trying to fulfill them within the actual auction environment. And we had two really major data quality incidents within a period of about three months. One of them came from a very simple schema change where a junior software engineer didn't understand that a feature, ironically called is dropped, was actually used as a feature within the model. They dropped it just because they're like, oh, well, our application's not using this is dropped column anymore, and it blew up our entire data pipeline. And nobody really knew exactly why for at least a few days until we did a root cause analysis.
Sam Partee [00:06:49]: I swear I've made that mistake before.
Chad Sanderson [00:06:51]: Everybody has. So many people have. And then the second is a lot more subtle and, I think more nefarious in some ways, where we had a new feature that was being launched, and this pricing model was trained on the data from our auction. And this behavior within the auction was all human generated, right? So it was a person going in and making a bid and then adjusting their bid and then taking the shipment and so on and so forth. And a very smart, brilliant product team decided to build a feature called Autobid. And Autobid meant all a truck driver had to do was turn this feature on, and a machine would incrementally bid down in increments of $5, $10, $50. So they bid lower and lower and lower. What that meant was, after a certain period of time, we had thousands of machine generated bids in our marketplace, and that was the data our pricing model was being trained on.
Chad Sanderson [00:07:46]: So that was a multimillion dollar incident that took place over a period of about three months.
Sam Partee [00:07:52]: Wow. It's interesting, the power of compounding error like that, when a generated error gets used again and how it compounds, it's specifically something that I feel like a lot of people have run into with now the scope of generative models and their use cases today. Speaking of scope, I'm curious. In enterprise, there's, I feel like a different set of requirements. There's a lot of pocs going out, right? There's a lot of, oh, I built this small rag app, or I built this agent, but at the same time, there's not what I've seen at least a ton or a plethora of enterprise ready use cases. So I'm curious, what about data quality gets you closer to that enterprise readiness? Maria, you have an answer for that?
Maria Zhang [00:08:36]: Yeah, I'm afraid I don't have a perfect answer for that, but I do know that question. I think there is a gap that exists both in terms of the precise understanding of what does it mean? And also from a compliance perspective. What is it compliant? And even the definition of SLA is the first token arrival satisfied? The slaE? And how about guard railing, hallucination, misalignment, and so on and so forth? Is 99.9% error rate okay? Because human being makes errors too, right? We talk about agents. It's really hard to get to 100% accuracy rate. So combine all of these factors, I believe there will be a new definition of enterprise readiness, and there will be a first wave of early adopters or companies that are savvy and that are contributing to this new standard of procedure, per se. But personally, I'm really excited to see that happening and actually would love to hear your thoughts on what does it mean in the era of generative AIH.
Joe Reis [00:09:56]: By enterprise ready enterprise is an interesting one. I wrote about this last week on my blog. I felt like there's really two types of data practices going on. There's product land, where you're using data to make products, and there's also enterprise land, where traditionally data is used in a back office it function. I think when we're talking about enterprise, it's a fascinating one because it's a, I mean, a lot of enterprises, I see, and this is some of the biggest companies around to small enterprises and everything in between. But it feels like data is an absolute dumpster fire and companies are still barely able to do bi at this point. So the question I always ask when I go around the world is, would you be willing to throw a large language model on top of your corporate data set as it exists today, to get, quote, insights? And my second criteria is, would you be willing to bet your job if this doesn't work? I think we asked this in Australia last year. Yeah, I think one person who is working on a large language model raised their hand and the others are like, no, I'm definitely not going to do that.
Joe Reis [00:10:58]: So, I mean, that's kind of the state of affairs. I mean, I've read this moniker for a while. I mean, a lot of companies can barely do bi. But now the thing is, there's a lot of, and there's a lot of motivation to want to throw AI at the problem. So I think there's a huge disconnect between sort of the incentives and the outcomes of what we're about to see there's not a board or a CEO who's going to admit, like, oh, yeah, we're not going to go down AI, right. You'd look like an idiot.
Sam Partee [00:11:24]: Everybody wants to say their board report, right?
Joe Reis [00:11:26]: Right. At the same time, knowing what you all probably know about most data sets and companies, I think you can make the leap. Right. It's not generative AI, it's more like degenerative AI, I guess, would be a better way of putting it. That's sort of the state where we are.
Sam Partee [00:11:41]: I think it also applies to some subsets of predictive modeling as well.
Joe Reis [00:11:44]: It always has. It always has.
Sam Partee [00:11:45]: Yeah. It's always been. The data quality piece has always been there. It's just that now it feels almost like it's emphasized because of its impact, its outstanding impact. You know, I think it was Pushkar. I think it was Pushkar, Chad, who was talking about the differences in predictive versus generative and like how that's changing the definition of data quality and model quality. Like we talked about model quality being something maybe like system quality of time to first token, but data quality being completely different. Do you want to talk about that?
Pushkar Garg [00:12:17]: Yeah, sure. So when I see data quality for the predictive side and then the generative side, I think a lot of it applies in like, the intersection of both of them. But there are elements of predictive AI. The data being used to do predictions for predictive AI, those are very, very different emails, call transcripts. Those are some of the things that we look at. So how do you identify tone? How do you identify some kind of action that is needed? So those kind of things, I think, are additional to what you would eventually look for when you're just looking at the predictive side.
Sam Partee [00:13:03]: A comment on that is that I've seen a lot of people try to use LLMs to do things like query intent modeling, and, I mean, I don't know about y'all, but like six years ago I was doing that with Xgboost. You know, you come up with four categories and it's just multi label classification.
Pushkar Garg [00:13:16]: Right.
Sam Partee [00:13:17]: And so it seems like it's almost like we stuffed too much towards the LLM. What do you think, Chad?
Chad Sanderson [00:13:22]: Yeah, I mean, I think that's definitely true. I mean, we're seeing a lot of sort of products and vendors these days that are trying to do traditional sort of machine learning as if it was an AI function. And that's definitely not the right way. But to sort of Pushkar's earlier point, I think, and to Joe's earlier point as well. I think that there are so many companies that are trying to start thinking about investing heavily in AI and AI quality, but their ability to do data quality on relational data sets is horrible, and that is, like, way easier. It's ten times easier than thinking about data quality for unstructured data. And there's a whole host of issues, even the most basic ones possible, like a schema changed for a data pipeline that I used, and it broke me. And these data teams really have no way of preventing or being proactive or even knowing that these issues are coming, let alone more of the complicated data quality issues relating to semantics.
Chad Sanderson [00:14:26]: So something changes in the data, and it doesn't have anything to do with the schema. Like, we're updating a column called datetime now to datetime UTC. If you've worked with data significantly, you know that that's gonna cause a massive issue for you.
Sam Partee [00:14:43]: Always use Unix time, right?
Chad Sanderson [00:14:44]: Always use time.
Sam Partee [00:14:45]: Integer Unix time every time.
Chad Sanderson [00:14:47]: Exactly. But most companies don't even have a great way of understanding that these issues have happened, let alone preventing them. So there's sort of this whole murky territory of dealing with data quality, even in structured data land. And I think trying to make that jump without getting your foundations in place is pretty dangerous.
Sam Partee [00:15:08]: Joe, you talked about anti patterns. That sounds like one of them, treating unstructured data like structured data. Do you have any other. You talked about some of those anti patterns. Do you want to number some of them for us?
Joe Reis [00:15:21]: I think that's a good one. Where do you even begin? Here? Structured data, I found, is always the hardest data set to work with. Right. Has anyone felt the same way? Like, it feels like unstructured data is usually easier, but then structured, you're sort of held victim to your own circumstances of the datasets. Yeah, other anti patterns, I would say. Obviously not having observability early and often on your datasets is a big one. Not knowing what you have is a big one. But I.
Joe Reis [00:15:54]: But again, this is most companies, so now you have to go retrofit all this stuff in, and now you get to atone for your past sins, I would say also the other big cardinal sins that Chad can speak to is just not addressing your issues as far upstream as possible. That's a huge anti pattern. I think all too often right now, we're sort of on the receiving end of it, but you're the receiving end of a sewer, so you can try and clean your water there, or you can make sure things are better upstream but that's your call, not mine.
Sam Partee [00:16:23]: What do you think?
Maria Zhang [00:16:24]: Yeah, I think I'm a little bit more optimistic than my fellow panelists here. I actually think the entire industry has gone through digitization. So I do think the data is stored somewhere, and that's a good starting point. One thing I've noticed repeatedly that people overlook or misunderstand the lack of precise understanding of the data. And that is so critical. Everything starts there. So often people just lump it together is like, this is my dataset, or structured data versus unstructured data, but there's just so much more to it. My suggestion is really to take a much closer and much more granular look at your data set.
Maria Zhang [00:17:15]: What's dynamic, what's factual, what's slow changing, what's human labeled with subjectivity, what is factual. When you have a more precise understanding of all this data at a column roles level, then you need to give them the proper treatment and the treatment is going to look very different. It's the classic divide and conquer. If you put everything together, it's going to look really messy and you feel like you can never get it right. But if you look at, okay, this is my dynamic data, I'm going to measure latency, I'm going to measure completion. I can make it synchronous, so on and so forth. And, you know, you're good there. And if this is slow changing, factual type of data, I don't need to worry too much about it.
Maria Zhang [00:17:59]: Right. Then I measure change. It shouldn't be changing too much. If it changed drastically, something went wrong. Yeah. So I see it over and over again. People overlook and try to kind of summarize, like just put all of these issues into one giant basket and then they feel overwhelmed to come up with the right solution. So take a closer look and really break it down.
Chad Sanderson [00:18:21]: Yeah, actually, just to sort of comment on that, because I think that's super relevant. This is a metadata problem, right. And it's a metadata problem that's existed in data for the past 50 years. Cataloging is a huge component of data management. Like, what is my data? Where is it from? What does it mean? What are the semantics? Who are using it? How is it changing over time?
Sam Partee [00:18:44]: There are whole companies built on just storing the metadata for your snowflake database.
Chad Sanderson [00:18:48]: There are whole companies built only around this. And so I think applying it in the context of unstructured data, structured data, AI, it's a thoughtful exercise that folks need to undertake. One of the activities that data scientists spend the most time on relative to anything else is just figuring out what the data means and where it comes from. And if you don't have sort of an automated system in place to help you with that, then ultimately you end up relying on the goodwill of whoever the producers of the data are to tell you accurately what this information means. And what I find is that there's an enormous amount of institutional knowledge that gets embedded in the engineers that are responsible for creating these data sets that data scientists depend on. And if you don't do a good job documenting that institutional knowledge, then, like, you're asking to be subject to data quality issues in the future.
Sam Partee [00:19:38]: Yeah, that's really interesting. We talked about, too, getting data scientists and machine learning engineers as the canonical sense of the two terms, like getting them to work closer together, and how it's more important that the people creating the systems and the people that are creating the data and the people that are cleaning the data all work together. Do you all have any tips or practices or methodologies or anything that you know of that gets that group of people to work better together?
Pushkar Garg [00:20:09]: Yeah, I can take that. So what I have seen is like, implementing things at the platform level is sort of always the best way. Implement as much as you can at the platform level and then make frameworks available. So like data quality in the data pipelines, right. How do we implement that? Bake in operators, in airflow or whatever. Your orchestration tool is like great expectations or any other data quality related tooling, and then make those available generally to the data scientists so that they can, when they're building their data pipelines, they're able to utilize those and then make their lives easier because they don't really like working with terraform and other like, you know, tools like that. So making their lives easier is something that I think we should go by. I'm talking from a platform ML platform person's perspective.
Pushkar Garg [00:21:09]: Yeah.
Sam Partee [00:21:11]: If you see a data scientist deploying terraform, you let me know, because I want to hire them. That person is multifaceted. Chad, you want to go?
Chad Sanderson [00:21:22]: Yes. Yeah, sure. I think there's a lot of things that you can do to better improve sort of the relationship between the producers of data and the consumers of data. The sort of foundational, the model that I use is that data is very similar to a supply chain. And in a supply chain you've got a producer and the producer is generating some raw input, and then that input is transformed many times, and then at the very end of the process you have a product. And I think that's true for the data pipeline as well. You have a raw data source, you have some ETL ELT mechanism, multiple steps of transformation, and then at the end you have a data product and AI might be one of those data products. And in the supply chain world, what's super important is this idea of end to end supply chain visibility, so that each person who's responsible for a transformation understands how that transformation is going to impact the owners of the data products downstream.
Chad Sanderson [00:22:19]: If you don't have that visibility and the awareness you're operating in a black box, you make a change. You might end up blowing up someone who's running an AI system, or maybe not, and it's totally fine. It's more or less impossible to know. One of the technologies I'm a huge advocate of is data contracts. The data contract is basically starting to apply an API to your data. And that's not like a literal API, it's an API in the sense of like there are certain expectations and SLA's that you have for your data assets. You always expect the schema to look a certain way, you expect the contents of the data to look a certain way. There's data quality rules that need to be enforced.
Chad Sanderson [00:22:56]: We would always expect like 1000 events to be emitted over this time period, and never five. And the goal is to have that check be as close to the producer as humanly possible. So if the failure happens, you can communicate that to all the other contracts downstream. And now your data supply chain is connected by contracts and not these disconnected people. So that's what I recommend. And it's something that some of the larger companies in the world are starting to implement across their entire data ecosystem, and it's very effective.
Sam Partee [00:23:24]: Joe, what do you think about that supply chain?
Joe Reis [00:23:27]: I like supply chain.
Sam Partee [00:23:28]: Supply chains are good.
Joe Reis [00:23:29]: It's pretty cool. I think also to solve the problem of making everyone work together, trust falls and group activities are good. I'm sort of kidding, but nothing. Yeah, the supply chain thinking is interesting. I actually come from a supply chain background. My background's in lean, so it's interesting to see how many principles from lean are actually adopted, starting in software and now in data, and now AI. This is an ops conference that gets its roots in lean. And so I think if you actually a good thing to go study, if you all really want to read a great tome, is the goal.
Joe Reis [00:24:07]: It's a supply chain book, I think written in the late eighties or nineties. Actually the eighties it was, it still holds true today. I would say that this is an awesome place to start. That'll give you a lot of the reference you need, but it's about continuous flow, reducing bottlenecks, collaborating with people, single piece workflow. I think if the industry can master these principles, it actually goes a long way towards reducing defects. The way you get into errors really is the way we do it now, which is you lob things over walls in supply chain, this is the old way of doing stuff. This is mass production. Accumulate a bunch of stuff in batch, which is anti pattern in lean.
Joe Reis [00:24:41]: But this also results in. Now you have to go and rework a thousand possible things, versus one where you can catch the error and fix it. So I think adopting those principles would go a long ways towards, I think, fixing data quality, which people like Chad are working on, and others. But it's really a process question more than anything. If you go back and read supply chain, like Deming, for example, in the sixties, he already called out that 98% of the problems are usually process related. It's not people.
Sam Partee [00:25:08]: That was not on my bingo card, but I loved that. I'm not sure who had that on their bingo card. Supply chain tome, this is what happens.
Joe Reis [00:25:14]: When you're inviting to a.
Sam Partee [00:25:15]: What do you think, Maria?
Maria Zhang [00:25:18]: Yeah, I agree with everything you said, but there is a human element, and that's what I call holistic ownership. Right? Even, you know, I work at some of the largest companies, and of course there are different departments, different functions. And my philosophy was always take ownership of it and not toss it over the wall and feel like, oh, I'm done with my job. Let me give you a simple example. So, of course, developers need to log activities, consumption, metering, so forth, and eventually it becomes billing to customers. And if there was a mistake, either you overcharge your customer, which is not nice, and when they find out, they're furious. And two is you under bill your customers, and there's massive revenue loss. So I actually got these two teams together.
Maria Zhang [00:26:10]: They actually never met on this side. You're creating these logs and supply chain. Five points later, dollars came out of there, and I could just see, and there were issues. Of course, it's never perfect, and the developer felt so bad because it was a. And then I feel like he's taking his job from a different lens. Like the work I do, like every piece of data, because sometimes it feels meaningless, right? I'm logging all these things, we're talking about billions and billions of events on a mainnet business, right? They're just like, whatever, right? And now he's like, okay, I'm going to take my job seriously, and I was like, start talking, start communicating. Do a monthly review together. Do error injection together.
Maria Zhang [00:26:50]: So really have this sense of holistic ownership and really understand where your data is going to deliver and end to end.
Sam Partee [00:27:01]: Well, I think that just about does our time. So I just want everybody to thank these awesome panelists for coming and sharing their expertise.
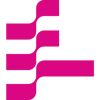