Data Scientists & Data Engineers: How the Best Teams Work // Panel // DE4AI
speakers
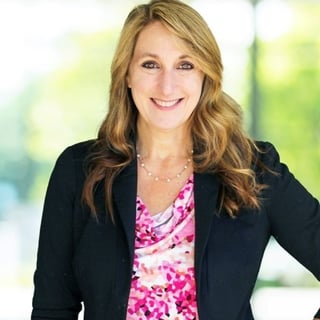
Beverly Wright, PhD, CAP serves as Vice President of Data Science & AI at Wavicle Data Solutions, and Director for Data Science & AI at University of Georgia’s Executive Education.
With a career spanning over 30 years across utilities, financial services, telecommunications, healthcare, consulting, and academia, Beverly has led numerous teams and established herself as a data science & AI thought leader, executive professor, national speaker, podcast host, and a trailblazing advocate for data science for good.
She also serves as Board Chair for TAG Data Science & AI, Board Member for Georgia Institute of Technology’s Master of Science in Analytics program, Past President of the Analytics Society at INFORMS, and podcast host for TAG Data Talk.
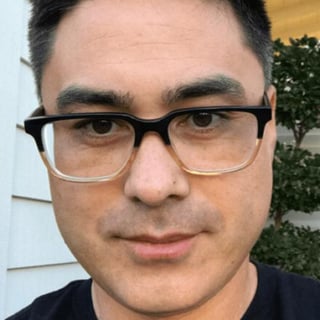
Joe Reis, a "recovering data scientist" with 20 years in the data industry, is the co-author of the best-selling O'Reilly book, "Fundamentals of Data Engineering." His extensive experience encompasses data engineering, data architecture, machine learning, and more. Joe regularly keynotes major data conferences globally, advises and invests in innovative data product companies, and hosts the popular data podcasts "The Monday Morning Data Chat" and "The Joe Reis Show." In his free time, Joe is dedicated to writing new books and brainstorming ideas to advance the data industry.
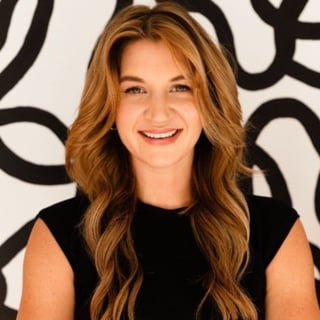
Sadie St. Lawrence is the Founder and CEO of the Human Machine Collaboration Institute (HMCI). HMCI pioneers research and education, to optimize human and machine interaction in the new era of knowledge work with AI, serving leading public and private organizations. Prior to HMCI Sadie founded Women in Data™, a non-profit organization with representation in 55 countries, and a community of over 70,000 data professionals. Women in Data earned recognition as a Top 50 Leading Non-Profit and the foremost community for Women in AI and Tech in 2021. With three degrees in piano performance, psychology, and data science, Sadie's journey from neuroscience research to over a decade in data science and AI strategy showcases her diverse expertise. Her accolades include being named one of DataIQ’s Top 100 Most Influential People in Data (2024), Dataleum’s 30 Outstanding Women in Data (2023), Top 30 Women in AI (2022), Top 10 Most Admired Businesswoman (2021), Top 21 Influencer in Data (2021), and Top 30 Most Inspiring Women in AI (2021). She has also received the Outstanding Service Award from UC Davis (2019). Additionally, Sadie's leadership extends to her role on the White House Council for Equitable Data and AI Training, her contributions as a DARPA community member, hosting the award-winning Data Bytes podcast, and educating over 600,000 individuals through her data science and AI courses at UC Davis, Coursera, and LinkedIn Learning.
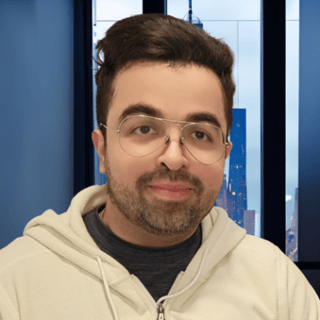
Victor is a seasoned software engineer with over a decade of experience, specializing in data and software development. He is a Senior Software Engineer at Microsoft, leading the prediction engine for a global product that benefits millions of users. His innovative and collaborative efforts were recognized when he won the Microsoft Global Hackathon Award in 2022.
Before joining Microsoft, Victor was the founder and CEO of Kyklos, a startup focused on payment software and data analytics. He has a strong technical background, with expertise in Azure, SQL Server, C#, Python, and ISO8583. His extensive experience is complemented by multiple certifications and awards in machine learning and cloud computing. Victor thrives in dynamic, diverse teams and is passionate about continually expanding his skills and embracing new technologies.
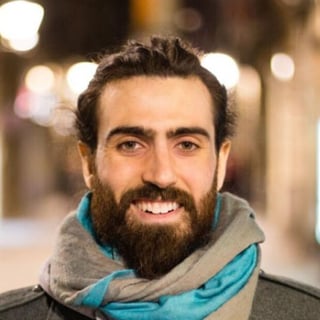
At the moment Demetrios is immersing himself in Machine Learning by interviewing experts from around the world in the weekly MLOps.community meetups. Demetrios is constantly learning and engaging in new activities to get uncomfortable and learn from his mistakes. He tries to bring creativity into every aspect of his life, whether that be analyzing the best paths forward, overcoming obstacles, or building lego houses with his daughter.
SUMMARY
There are clear patterns that make the highest functioning data teams work so well together. In this panel we will explore what data scientists and data engineers need to know about each other's responsibilities to speak the same language and align incentives.
TRANSCRIPT
Demetrios [00:00:02]: I'm gonna bring on our panelists for the next session. And who do we have coming up first? My man Joe. Where are you at, dude? This up. Wait, can we see your shirt real fast? Are you? Are you rocking this?
Joe Reis [00:00:21]: I'm not rocking it today.
Demetrios [00:00:22]: Oh, you're rocking. Sorry.
Joe Reis [00:00:26]: You don't rocking your beard, though, so I guess we're even. I was like, I I'm wearing mine.
Demetrios [00:00:32]: It's okay.
Joe Reis [00:00:32]: You are.
Demetrios [00:00:33]: It's okay. So I I got you on this panel. We've also got Beverly on this panel. Where are you at, Beverly? Hello. Uh, next up, where. Sadie? Sadie. Hello. How you doing?
Sadie St. Lawrence [00:00:49]: Doing good.
Demetrios [00:00:51]: All right. Hey, there's Victor. Can you see us?
Joe Reis [00:00:56]: She's moving now.
Demetrios [00:00:57]: But the main thing on this, and I might have to do hot potato over to you guys, which I fully trust you're capable of doing running your own panel. So the idea of this panel is data scientists and data engineers and how they can best work well together. And I think, Sadie and Joe, you are both coming from the data science world, right? And now doing a lot of data engineering stuff. So, Joe, I'll kick it off to you first if you have some burning questions. Victor, we see and hear you. All good, man. It's all good. I think you might be asking questions in the chat, but basically, the reason.
Demetrios [00:01:45]: Let me give you a little background on why I wanted to create this panel, especially with you folks. I feel like a lot of times, many things can be fixed if we have shared terminology and if data scientists understand, like, what data engineers goals are and what they're dealing with every day, and if data engineers do the same with the data scientists. And so I wanted to explore that kind of space for a little bit. I'll kick it over to you, Joe, and I'll let you get rocking with it first.
Joe Reis [00:02:28]: Yeah, I mean, it's an interesting. Can you hear me as well?
Demetrios [00:02:31]: Yeah, yeah, yeah.
Joe Reis [00:02:34]: So it's great. Yeah. I mean, it's an interesting one. I mean, I got into data science before. It was data science and data engineering before, I guess that was so. I sound like the ultimate hipster. I can talk about how many days I crossfit as well, if you'd like, but it's an interesting one. I feel like if you go back, I was actually on a hike today talking to somebody about this.
Joe Reis [00:02:58]: But if you look back at the origins of data engineering, it sort of came about, I would say, at least in this recent incarnation, kind of by accident. There's always a big tech version of it, but it was very much to support data scientists in ways that data scientists couldn't support themselves on their own or were not given support. Right. Namely setting up the infrastructure and the foundations to upon which to do data science. And so that's the original incarnation of it. But then you fast forward to today, and data engineering is a pretty popular and useful job title. Obviously, we're having a whole talk about it, a whole conference, actually, about how data engineering can work with AI. So it's come a long ways.
Joe Reis [00:03:40]: I'm more curious about what happened to data scientists. I would love more people's opinion on that at some point in the conversation, but that's my answer.
Sadie St. Lawrence [00:03:47]: Can I add on to what you said, Joe, because I feel similar, which is, I don't know if I became a data scientist before it was a thing, but definitely ten years ago. So it's been a little while. And I will say at first was like data science was this kind of full stack developer in data science, right? You were the data engineer, and then people realized like, that's too much. And so data engineering got split out. And then I think everybody realized that actually data engineering really is the meat of what data science was and that we need way more of that than we actually need of, like, the model building. Right. And I think that one of the key problems is, like, a lot of the data science education didn't teach fundamental principles of data engineering. Like, for me, in my master's program with data science, it was so hard on the model building and the visualization.
Sadie St. Lawrence [00:04:40]: And yes, you took a SQL class, but then I got into the real world and was like, oh, it's just all SQL and all pipelines. And I think that part of the problem from it came just like they were trying to pipe out data scientists as fast as possible, because ten years ago, that was all the rage and really missed a lot of, like, the foundational concepts of data engineering, which is why I think we've seen more of the rise of data engineering these days versus data science. But I'd be curious what your opinion is.
Joe Reis [00:05:09]: Yeah, I agree. Like, I, if you look at the, I think around the time you were getting into data science, I think it was, the curriculum is very model heavy, right? So it's probably, I'm guessing, Python, Skler and all that fun stuff. And then, uh, maybe some matplotlib thrown in. Did you learn SQL? Is that one of the things, too? Were they, that was also when sequel was sort of like, thrown under the bus is like, oh, we don't need this anymore, because obviously, Python, I did learn.
Sadie St. Lawrence [00:05:35]: I did learn it. It was, like, one class, and I think, as it was so light, is what encouraged me to go and, like, teach a class on it, because I was like, this is the core of what you need to know. Right. But then there's also this whole idea for a while of, like, well, is no sequel gonna be the big thing? And, you know, so people were still a little bit on the fence of, like, do we commit fully to sequel or not?
Joe Reis [00:05:56]: Yeah. Hi, Beverly.
Sadie St. Lawrence [00:06:04]: I got nothing.
Demetrios [00:06:05]: Me neither. Oh, dang it. Something's happening there. All right, well, I don't know what's going on with that, but we can try behind door number two.
Sadie St. Lawrence [00:06:20]: Victor.
Demetrios [00:06:23]: Yeah.
Victor Cuadros [00:06:24]: Oh, finally.
Demetrios [00:06:31]: So, I imagine, Victor, you haven't been hearing what we were chatting about, but one key thing that I think both Joe and Sadie were saying, mainly Sadie, is that when she learned data science, it was very heavy on the modeling piece, but not so heavy on the data engineering piece, and so that kind of left this big hole in her understanding as she got into the industry.
Victor Cuadros [00:06:58]: Yeah, yeah, definitely. The same stuff kind of happened to me, because, for me, I've been doing it way back, and I didn't even hear about, like, designs, like, since five years ago. And I was like, oh, there's a whole new world about this stuff, and there's a lot of people knowing this stuff. And there was a huge gap to understand what were even the models, what we were targeting. And for me, it was, like a huge experience to learn this other stuff. And it's like a, you know, when I used to be software engineer, too, only. And it's like this gap between back end engineer and front engineer. You have to find a way to communicate, to go to there.
Victor Cuadros [00:07:39]: So it's basically. It's like a cornerstone for a good pillar to go ahead with the.
Demetrios [00:07:49]: So good, so good. So, all right, the other pieces that I've been thinking about just in this conference while we're here is how there's so many things that the data scientist doesn't recognize from the data engineer's point, but also vice versa. Right? So, the data engineer, I think a lot of times they're serving the data scientists, but maybe they're not really that empathetic to their needs. And I would love to chat about, like, what you all have done or things that you've seen work well to make sure that you're best serving the data scientist.
Victor Cuadros [00:08:37]: Yeah, sure. I agree. One of the big topic over there is, like, the. And he has worked for me recently. Is the pair programming knowing a little bit of each world? Because if you don't know a little, if you don't know data science, you won't know, like, basically your customer needs and vice versa.
Joe Reis [00:09:02]: Empathy is important. I think maybe it's something it's better explained by anti patterns. So how would you cause division and animosity and hatred amongst the two groups? I always like to look at things through. What would you want to avoid doing? Let's see, where to begin. There are many, probably that would be a whole conference in itself, but some big ones is treating data engineering and data science is two separate silos that may get together during the annual company pizza party and not talk to each other. Apart from that, similar to how software engineers and data engineers often communicate or don't communicate, more to the point, which is where when you have upstream dependencies, like software engineers are an upstream dependency of data engineers, data engineers are often an upstream dependency of data scientists and so forth. When you create silos and kingdoms, and especially when product managers or project managers aren't aligned across these different functions, I think that's when you start getting a lot of the problems that occur, right? And then you don't have the empathy, you don't have the team collaboration and building. You don't have the paraprogramming that Victor mentioned.
Joe Reis [00:10:20]: There's no. There's no pair, there's no pairing of anything. So it's very balkanized. And so I think that that's a good way. If you wanted your data projects to ultimately fail or have, you know, done everything in your power to, you know, make things very difficult for yourself, then you should. You should do what I just advised, which is, like, set up highly siloed, highly balkanized teams that don't talk to each other, and you should totally do that. That's a good idea. What do you think, Sadie?
Sadie St. Lawrence [00:10:54]: Yeah, I mean, I think one of the biggest issues is just, like, a lot of people don't understand what the bigger picture is that you're building for. Right. I mean, whatever you're building, data scientists have a piece of it, data engineers have a piece, and that piece adds up to the whole puzzle. Right. And it's like people are working as a team to create fill in the puzzle, but nobody saw the end state of what that puzzle looks like oftentimes. Right. Or maybe even worse, you have, like, a PM who did. Who poorly communicates it to each of the technical teams.
Sadie St. Lawrence [00:11:26]: Right. So not to, like, throw the PM under the bus in this situation and be like, it's not the data science for data engineers, it's the PM. Right. I feel like there should be a teacher or something that's like, it's not my fault, it's the PM didn't fill out the ticket properly kind of thing. But I say that happens so often is like, even the data scientists maybe don't understand the full picture or the data generators. And so just as much as you said of like, yes, not working in silos, but like, we have to see what that end puzzle looks like for what each piece we're bringing to the table. And then we have to collaborate as data scientists and data engineers to see where do our pieces fit together. Right.
Sadie St. Lawrence [00:12:06]: But it's so hard to even determine where your pieces fit together if you don't know what the in state looks like that you're building. And that is where I just see so many teams fail time and time again.
Joe Reis [00:12:17]: Yep. Yeah, but just blame the PM. That's easiest to do, but yeah, it's all their fault. No, that's a really good point. I think it helps if you've worked in other areas, like you come from software. Victor. I've worked in software and data science, so forth, and I think it's good to have that kind of experience either downstream so you know what good looks like, or upstream so you kind of understand, okay. Like, this is how the data is probably going to be generated downstream and these are the unseen impacts or things that data engineers are probably not even aware of that I can bring to the table.
Joe Reis [00:12:54]: So I think if you've worked in the other positions, it certainly helps, I think, with that piece of just understanding. Okay, so what do the stakeholders need that I'll be providing data to?
Sadie St. Lawrence [00:13:05]: Yeah, I'm curious what you guys think if there will be more of a rise of a full stack, in a way. Data engineer, full stack data scientists coming forward. Given the fact of AI tools and what we can do. This last weekend I was playing around with relet and cursor and I'm just like, I was never a full stack developer and feel like I am now. That is only going to soon cross over into our data world and our data tech stack. Does that mean we're going to switch back from like these siloed roles to more of like a full stack data professional role? What do you guys think?
Victor Cuadros [00:13:42]: Yeah, I agree on that one. That's something that has been ringing my belt last couple of years before we used to be like a half, as I told back engineer, front end engineer, and all these tech is like, it got easier to be mix both worlds together. I think as data science and engineering get more easier to handle, we will have two worlds merge together. And it is a big impact, to be honest. And I think the bridge is already there, and it will be more narrow and narrow between those two professions. I won't be surprised in the future if we will have a full stack. I don't know how we would call it engineer sciences or something like that, but, yeah, it's basically, it is amazing how it will work, but I'm more than sure, especially with AI tools right now.
Joe Reis [00:14:35]: It's an interesting one. I mean, so OpenAI just announced today the release of o one, the new model, and it got me thinking about kind of the broader context in which all these things sit, because you mentioned you're able to have, like, somewhat superpowers, Sadie. And they, and we wrote about this in the fundamentals of data engineering, where it felt like this was before chat GBT, but it felt like the roles were actually going to converge to what we call the live data stack. So the rise of streaming, for example, and that becoming, I think, the next first class citizen in the data world, and the fact that products are becoming more modularized and commoditized, and the missing piece is interoperability. But assuming that's solved, the feedback loop between software data and Mljdehe, it shrinks, which means use cases get tighter. And so it does make me wonder, for a lot of use cases, do the roles converge? I would argue probably yes. But with the rise of these new tools, again with strawberry or one model today, it can apparently do PhD level reasoning on math science problems. The broader question I had is, okay, what does that do to science teams at companies? Do you just not do it anymore? And so, you know, I know a lot of people who are starting companies right now who are basically just leveraging, you know, AI API calls for a lot of the work.
Joe Reis [00:16:03]: So it's pretty shocking. Like, one of my friends is trying to raise money for is a startup right now, and some VC's like, well, how big is your engineering team? He's like, oh, it's just me and Claude Aihdeende.
Sadie St. Lawrence [00:16:14]: Yeah, I think we're gonna have to start naming, like, the agents and stuff to put them, like, on our team chart.
Victor Cuadros [00:16:23]: Right.
Sadie St. Lawrence [00:16:24]: Because it's just like, no one will believe you if it's just one person. But, yeah, I agree. I think one of the things that it's gonna, I don't think people are gonna lose their jobs in ways too. But I think our scope of what we manage is going to expand. And the main reason for it is because in the past I would, you know, put in a request to have this data model or to have something built and then wait a few days and then see what I got back and realize it's not right, you know, make some changes, work back and forth. Now all I see that's happening is we're still putting in requests to these models and these agents to help us in building, but we get immediate feedback almost. I mean, that's what I was loving building with replic this past weekend was just, I would put in a request and it, you know, within like 10 seconds it'd show me a preview of my web page of what I built. Then I'd say, okay, move this box over here, and immediately Leah would change it and happen.
Sadie St. Lawrence [00:17:15]: And so for me it's like maybe that back and forth of the problems that we're facing within data science and data engineering teams of like the miscommunication or misalignment could be solved by some of these tools, maybe replacing the data scientist or replacing the data engineer and having that one person work directly. Because instead of waiting a day or two, I'm getting instant feedback from the work that's happening.
Victor Cuadros [00:17:41]: Yeah, one of the things that I most is like that jobs won't be lost. Like it would evolve. Because in the past before, you know, Azure AWs, we had people building data centers for each of building servers, and this was way different. Right now, the same people that focus on that switch to, you know, they have, they build everything in the cloud, everything is, they have the knowledge, they have how to connect all this network. So it has changed a lot. And I think that for example, for me, when I was digging into data science world, for me it was hard at the beginning. I didn't understand quite a bit, but with the GPT, all this stuff, it was way easier to understand. You can even tell it, hey, explain it for a five year old how this will be and it's easier, it gives you good concepts.
Victor Cuadros [00:18:29]: And then after that I was creating models and it got way, way, way easier to do all this stuff. I think one of the basically biggest challenge that we are facing right now, it could be the remote communication that we have between those big careers that were there because it's hard, like remotely, we have to get used to it, like working with people for other time zones, other countries. That's how far we have went before this open world remote work that we have. And communication has not been that good. And I think the different strategies or cross work that we do between those two fields, it will help. For example, cross teams work, it will help. And the pre programming I mentioned before too, it kind of closed and out of the gaps because for you, it's like, as I said before, like the data science is your customer, but at the same time you're the customer of the data science and you need to provide each other with enough feedback and information to accomplish goals together. What do you think about the remote works and all this? How to impact you new lives?
Joe Reis [00:19:44]: I mean, I grew up working in a traditional office environment, so I remember the days of cube farms. Really bad pleated khakis and yeah, pizza in the break room, people cooking fish in the break room. Disgusting. I remember what that was like. I can't say that I miss it. At the same time, you know, when I visit offices, which I do once in a while more to give talks and give high fives and stuff, it's interesting because I feel like there's a sense of camaraderie that it's really hard to get with remote work. And there's a sense of collaboration, I think that lends itself in an environment like that. My opinion is you should do both if you can, I guess on a hike today with somebody who flew in to see from New York City to go see his team because he has an office here in Salt Lake City where I live.
Joe Reis [00:20:38]: Why not just keep that all Zoom calls? Why meet your team at all? Why have an office at all? That's kind of a weird thing. But he does and because it, because it worked for them. But I think that to paint, I often see a lot of big CEO's say, well, remote works stupid and everyone has to come back to the office or, you know, office work is stupid and we need to stay remote. I think it depends on the company culture you have and like what works best for the culture and for the specific team or department you're in. I don't think there's a one size fits all for any of this stuff. But for hard problems like data, I think it does help to physically meet and whiteboard. But you can solve a lot of this now with stuff like loom and other products that do, I think a fairly good job at asynchronously helping with this. But I think as well though, I mean, how often do we just stare at screens like this? All day, being on video calls.
Joe Reis [00:21:26]: Right. It needs a bit of a fatigue at this after a while I feel like I'm at an office meeting right now, I don't feel like we're on a webinar, but, yeah, definitely.
Sadie St. Lawrence [00:21:35]: I think there's just something so magic about the white. The whiteboard and, like, virtual whiteboards do not, like, compare. And there's something that just is, like, magical about being in a room with other engineers, data scientists, and each having the ability to write on the whiteboard that I haven't found anything that can replace that quite yet. I was hoping with remote work, it would be better, because it'd be like, documentation is better, or how people write, you know, Jira tickets is better, that type of thing. But I think the whiteboard still trumps everything, and there's still a place for teams coming in collaboratively.
Victor Cuadros [00:22:15]: Yeah. Nobody reads documentation unless, if it exists, nobody misses.
Joe Reis [00:22:21]: Well, you mentioned the empathy part. Sorry, Beverly, what did you say?
Beverly Wright [00:22:24]: I was going to say, people ask me all the time, what's your. What's your favorite tool? And they kind of laugh because I say, the whiteboard. It's my favorite tool. It's my favorite collaborative tool. But it's also a way to think through the data engineering and the data science. Like, how do you go about hypothesizing a model before you start putting hands on keys? So, yeah, I've always found that to be a really useful tool.
Joe Reis [00:22:48]: Well, and you mentioned earlier, Victor, the pair programming and empathy part. Right? You tried pair programming remotely, I'm sure.
Victor Cuadros [00:22:56]: Yeah, yeah, definitely.
Joe Reis [00:22:57]: Have you tried that in person as well?
Victor Cuadros [00:23:00]: Yeah, yeah. I mean, it's way different experience. I recently, I had, uh, I went to the office, uh, and one of my peers that I just met, first time in person, they were, like, always good communication. There was no need communication. But when I went to the office, it was funny because she was like one, you know, one that's awake. And when she was like, oh, I have a question. And she. The solution was like this once.
Victor Cuadros [00:23:25]: Different from, uh, you know, teams or other way of communication, chat. It is harder to. You have to wait. Probably you lose the track of mind or train the thoughts that you will probably have. But in programming, obviously, in person, you can sit between two persons. You learn from each other at that moment, and ideas, just brainstorming at the moment. It just comes out. It's easier in that sense, even though the tech has go to a place that we can call that other person, it's not the same, because sometimes you.
Victor Cuadros [00:23:57]: You want to do a follow up, I don't know, ten minutes after the call, and you have to send a message, and it's way different.
Beverly Wright [00:24:08]: Yeah, well, it sounds like we've talked quite a bit about remote. And what I'm wondering, too, is what is your final piece of, of advice for how data engineers and data scientists can better collaborate? If you had to leave the audience with one piece of advice, what would you say?
Victor Cuadros [00:24:31]: I would say prep program and improved communication between the two areas. That's the takeaway that has worked so far for me. And the empathy about the setting. Sometimes boundaries is good, especially if you are in different countries, because sometimes your time is way different. I have worked with people that is 9 hours ahead of me, six people 6 hours ahead of me, or 3 hours before me. So it's different. So that, that, that sense of empathy, as we mentioned, the programming, it can go a long way into improving that type of communication.
Beverly Wright [00:25:09]: Yeah.
Sadie St. Lawrence [00:25:12]: I would just say whatever side of the fence you're on, like, do the other person's job, or at least try, you know, and then you'll just one, you'll learn the terms of how to talk to those people better. You'll, to Victor's point, have more empathy for what they do. Right. And, you know, take the time to really get to know what is that big picture that you're trying to build and, you know, try something new out. Don't get stuck in your own way of doing things. Who knows? Maybe you'll switch over to the other team, too. Like, you may end up being like, actually, this is better, right? And be like Joe and be a recovering data scientist and switch over to.
Victor Cuadros [00:25:48]: Definitely.
Beverly Wright [00:25:49]: So try to walk them all in their shoes and build some empathy. I had a colleague, he's the chief data analytics officer, and that's what, that's one of the things that we talked about on a podcast interview. He said, I don't think there's enough empathy for data engineers to understand what data scientists do, for data scientists to understand what data engineers are doing. So I get that point completely. Any other final thoughts or advice?
Joe Reis [00:26:16]: I don't have much to add that hasn't been said. That's all awesome stuff, I would say. If you're able to go get lunch with somebody, I think best bonding moments at work have always just been getting lunch with people and getting to know them, you know, um, I think nothing, uh, helps more than just, I think people seeing each other as people and not just as somebody who is an avatar on slack. So, um, you know, which, unfortunately, the world we're in right now, everything's become depersonalized. And I think that's, uh, taken away a bit from the, um, you know, the experience of building great things together. So, yeah. Let's get back to simple.
Beverly Wright [00:26:55]: There's a lot to be said for it. Excellent. Well, thanks for all about this. Data engineers working together.
Demetrios [00:27:04]: Yes. Thank you all for coming and doing this. It was very cool and nice to see Beverly, thank you for sticking with it and making it here. I appreciate that. We had some fun, and it wouldn't be a virtual conference or a virtual event by any standards, if we didn't have a little bit of technical difficulty. We made it happen.
Joe Reis [00:27:29]: You got a new sweater.
Demetrios [00:27:31]: I got a new sweater. Yeah. Because it's good.
Joe Reis [00:27:34]: I. Yeah.
Demetrios [00:27:36]: Joe, thank you for noticing. I appreciate that.
Joe Reis [00:27:40]: Anytime.
Demetrios [00:27:41]: Now I'm kicking you off the stage. You're out of here. All right. See you later. See ya, everybody. Later.
Victor Cuadros [00:27:47]: See y'all.
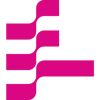