The Only Constant is (Data) Change // Panel // DE4AI
speakers
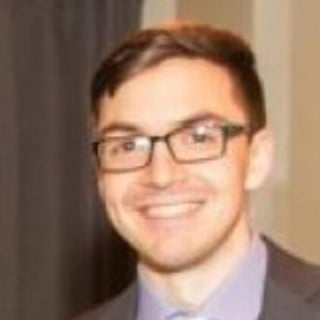
Ben has spent his career focused on all forms of data. He has focused on developing algorithms to detect fraud, reduce patient readmission and redesign insurance provider policy to help reduce the overall cost of healthcare. He has also helped develop analytics for marketing and IT operations in order to optimize limited resources such as employees and budget. Ben privately consults on data science and engineering problems both solo as well as with a company called Acheron Analytics. He has experience both working hands-on with technical problems as well as helping leadership teams develop strategies to maximize their data.
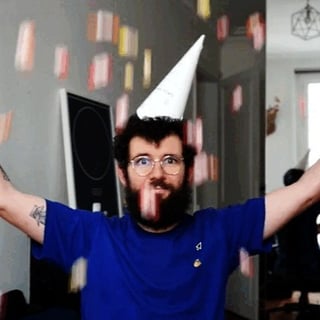
10 years experience data engineer building data platforms for analytics and AI to empower data users and stakeholders. He also creates content about data engineering and has a weekly newsletter.
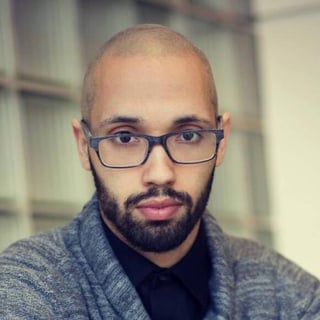
Chad Sanderson, CEO of Gable.ai, is a prominent figure in the data tech industry, having held key data positions at leading companies such as Convoy, Microsoft, Sephora, Subway, and Oracle. He is also the author of the upcoming O'Reilly book, "Data Contracts” and writes about the future of data infrastructure, modeling, and contracts in his newsletter “Data Products.”

Maggie has over 13 years of experience as a data practitioner, product manager, and community builder with expertise in data engineering, analytics engineering, and MDS tooling. She's passionate about building solutions that make data accessible, intuitive, and impactful and enthusiastic about helping others succeed.
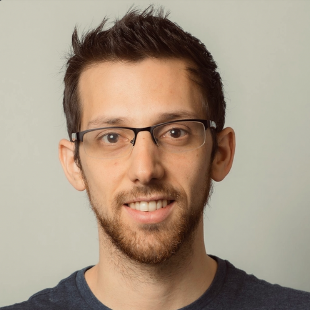
I'm a tech entrepreneur and I spent the last decade founding companies that drive societal change.
I am now building Deep Matter, a startup still in stealth mode...
I was most recently building Telepath, the world's most developer-friendly machine learning platform. Throughout my previous projects, I had learned that building machine learning powered applications is hard - especially hard when you don't have a background in data science. I believe that this is choking innovation, especially in industries that can't support large data teams.
For example, I previously co-founded Call Time AI, where we used Artificial Intelligence to assemble and study the largest database of political contributions. The company powered progressive campaigns from school board to the Presidency. As of October, 2020, we helped Democrats raise tens of millions of dollars. In April of 2021, we sold Call Time to Political Data Inc.. Our success, in large part, is due to our ability to productionize machine learning.
I believe that knowledge is unbounded, and that everything that is not forbidden by laws of nature is achievable, given the right knowledge. This holds immense promise for the future of intelligence and therefore for the future of well-being. I believe that the process of mining knowledge should be done honestly and responsibly, and that wielding it should be done with care. I co-founded Telepath to give more tools to more people to access more knowledge.
I'm fascinated by the relationship between technology, science and history. I graduated from UC Berkeley with degrees in Astrophysics and Classics and have published several papers on those topics. I was previously a researcher at the Getty Villa where I wrote about Ancient Greek math and at the Weizmann Institute, where I researched supernovae.
I currently live in New York City. I enjoy advising startups, thinking about how they can make for an excellent vehicle for addressing the Israeli-Palestinian conflict, and hearing from random folks who stumble on my LinkedIn profile. Reach out, friend!
SUMMARY
If there is one thing that is true, it is data is constantly changing. How can we keep up with these changes? How can we make sure that every stakeholder has visibility? How can we create the culture of understanding around data change management?
TRANSCRIPT
Adam Becker [00:00:04]: Let's see, we have Chad, Christoph, Ben, Maggie, up for the panel. What might be, like, a good, like, dramatic way to introduce you guys? I'm not sure. How about this? We should. Okay, I'm gonna bring one person on first, and then they can introduce the next person. Do you all know each other? I'm not even sure you all know each other. Let's see. Maggie, are you here with me?
Maggie Hays [00:00:26]: I'm here.
Adam Becker [00:00:27]: Hey. Hi, Maggie. How are you?
Maggie Hays [00:00:28]: Hey, Adam.
Adam Becker [00:00:29]: What are you guys going to be talking about today?
Maggie Hays [00:00:32]: We're talking what it takes to work in data engineering these days. We've all been in the space for, I don't know, collectively half a century, I think. So, yeah. We're going to dig into, kind of dig into how the data and data engineering role has shifted and kind of how we evolve with it.
Adam Becker [00:00:51]: The only constant is data change.
Chad Sanderson [00:00:54]: Data change, data change. Okay.
Adam Becker [00:00:56]: I love it. So, next to the stage, maybe we'll introduce Chad. Chad, are you with us?
Chad Sanderson [00:01:04]: I'm with you.
Adam Becker [00:01:05]: Hi, Chad. We got Chad, we got Christoph. Hello.
Maggie Hays [00:01:08]: Kristoff.
Adam Becker [00:01:09]: Hey, Ben.
Benjamin Rogojan [00:01:12]: Hello. Hello.
Adam Becker [00:01:14]: I think we're good to go.
Maggie Hays [00:01:15]: We're good.
Adam Becker [00:01:16]: Okay, I'll be back in a bit. Good luck, guys. Enjoy. And I'll be eating popcorn and watching.
Benjamin Rogojan [00:01:28]: All right, well, we didn't get intros. Do we want to do a quick round?
Maggie Hays [00:01:31]: I guess, yeah, let's do it.
Benjamin Rogojan [00:01:33]: Awesome. Like I said, I'll just start things first. Hey, everyone. My name is Ben Rogojon. Online, I go ast data guy before I fully became the CI data guy. Also, is there a reverb? I feel like I'm hearing myself somewhere. But before I kind of went full CI data guy, worked at Facebook for a few years as a data engineer. Before that, worked a lot in healthcare doing data engineering and data analytics work.
Benjamin Rogojan [00:01:58]: So great to meet you.
Chad Sanderson [00:01:59]: All.
Maggie Hays [00:02:01]: Right, I will go next. I'm Maggie. I'm part of the founding team at Acrel Data. I'm a community product manager for Datahub. Datahub is an open source metadata platform. I've been in the data space for, I think, 14 years at this point. Done everything from kind of individual, you know, ic work as we weren't called a data scientist, but it's basically data science back in the day, then ended up moving into kind of data engineering as a tech lead for a data team, and then made my way into product management. So in the data space for this whole.
Maggie Hays [00:02:34]: The whole journey, it's been a journey, to say the least, and excited to be here.
Christophe Blefari [00:02:41]: Cool. I can continue. So I'm Christoph. I've been doing data engineering for the last ten years, and for six years, I've been, like, in permanent position. And for the last four years, I've been doing freelancing on the side. I also do, like, content creation on Blev FA, where I have a newsletter where I do, like, content creation every week about data AI and so on. And since this week, I'm launching a new company called now to help people doing data transformation without any technical called knowledge, because AI gives super power to everyone. So might be like the next thing.
Benjamin Rogojan [00:03:24]: Awesome.
Chad Sanderson [00:03:25]: Well, I'll wrap it up. I'm Chad. I'm the CEO of a data infrastructure company called Gable AI. Prior to that, I've done a little bit of everything in data managed data engineering teams. I was an analyst. As a data scientist, I worked on infrastructure. I led the data platform and artificial intelligence platform team at a company called Convoy. Part of that, I was a tech lead on Microsoft's AI platform as well.
Chad Sanderson [00:03:51]: So.
Benjamin Rogojan [00:03:53]: Cool, cool.
Christophe Blefari [00:03:55]: And there is something cool to say that I live in Paris slash building. And you three of you like, living in the states, I guess?
Benjamin Rogojan [00:04:04]: Yes, yes. We're all boring in stateside.
Maggie Hays [00:04:06]: I know, I know.
Chad Sanderson [00:04:09]: Well, you guys can speak to yourselves. At the moment, I'm actually in, in London. So I'm in a London hotel room in between, presenting at other conferences.
Maggie Hays [00:04:22]: Hustling out there, man.
Benjamin Rogojan [00:04:26]: Multitasking to the extreme. Awesome, awesome. Well, with the topic of this panel, I kind of posed the first question to kind of just help level set. So I just want to ask, when you all first started in the data world, what did it kind of look like? Both in terms of tools that you used, or maybe just terms that were kind of very standard, like, what was it like for y'all? And maybe I can just direct that towards you, Maggie.
Maggie Hays [00:04:51]: Yeah, so I. My first role was at bank of America. This is where I was acting as a data scientist. I was a market information consultant, which. What does that even mean? But the very first language I ever learned was SAS. I don't even think SAS is still around, but, you know, kind of doing all of our data modeling and analyses in there. I stumbled my way through SQL. Took me a while to figure it out, and then really kind of hit my groove.
Maggie Hays [00:05:19]: But, I mean, this is back. We're working in SAS and teradata. There were days of the month, like the first five days of the month, you couldn't query against the database because they were, like, running all the batch backfill so things were just, like, incredibly slow and managed by a central team of, you know, database. Database engineers who we never really interfaced with. Right. Like the magic just magically, or the data just magically appeared and there was no conversation around, kind of like, how it was created, how was it derived? It was just, like, very pristine and ready for us. And I remember there was. One of my teammates was fighting the good fight to get us to adopt python, and, like, no one knew what it was.
Maggie Hays [00:06:01]: We were all looking at him like, why would we use something else? Sass is everything we need. So just seeing the evolution of how quickly that has evolved has been tremendous. Chad, what about you?
Chad Sanderson [00:06:14]: Yeah, so this is going to sound very basic, but I think my first experience in the data world was Microsoft Excel. There was a lot of Excel work being done. My first sort of real technical job was more on the SSIS side, so working a lot more heavily with the relational databases. And that sort of what I understood data to be was more of the transactional, operational sense. I didn't have that much analytical experience. And then we ended up doing sort of regular FTP based sort of data dumps, and I was sort of managing that process and trying to think about what quality looked like. And quality was zero automation, and it was more just making sure the person responsible for the. For the data dump wasn't significantly changing the schema every single quarter because it caused some pretty significant problems.
Chad Sanderson [00:07:13]: And then from there, started going into r from some of the data science stuff, and then it just kind of exploded. I don't know exactly when it was. Maybe there was a specific year that I could point to on a calendar where it felt like the number of technologies just basically jumped really substantially. I think it was probably post Hadoop era once we went to the cloud, and then you had a lot of, like, vendors and sort of open source companies all pile in at the same time. But, yeah, that's 2016, I think. Yeah, yeah, that's. That's probably right, yeah.
Christophe Blefari [00:07:48]: Yeah. And on my side, I don't know if I came late to the party compared to you, but back in the time. So when I started, like, in 2014, I was. So I started with Hadoop in 2014 in France. And at that time, it was like, one of the first projects in France, like, to build data lakes and so on. I was in a consultancy firm, and at that time, I was, like, every time saying to the clients, yeah, in France, we are, like, three years later before the States, so we are doing the same as they do, and it exploded. On their side, but they started in 2011. And so when I was, like, building the first athletics in 2014, I guess I was like, a pioneer, a pioneer in France.
Christophe Blefari [00:08:29]: And, yeah, it's how it got me into data. I was just, like, graduated, and I was building data lakes, and no one was knowing what it was meaning at that time, to be honest. But, yeah, that's how I started. And you, Ben?
Benjamin Rogojan [00:08:50]: Yeah, yeah. I mean, I started, like, the first company I worked for was a hospital, and we were using a lot of SQL server. I remember there was always whispers of a data lake and Hadoop being built off somewhere else. But for most everyone else, the data warehouse was in SQL Server. And I always say that I didn't know what a data warehouse was at the point because I knew what a database was. I was literally taking a database course in school at the time. I was interning at this hospital. And then they're like, here's SQL server.
Benjamin Rogojan [00:09:19]: I'm like, oh, yeah, this is just a normal database. And I remember I had to eventually figure out in a month or two, be like, oh, this is something slightly different. They don't teach this in school. Maybe now they do, but back then it was just like, unless you took some course afterwards or some certificate afterwards for a bi or data warehousing program, you wouldn't really realize it was different unless someone told you directly or you kind of eventually started to piece together that, yes, you're using SQL and things are called keys instead of ids, but eventually you start putting things together. So that's definitely where I started.
Chad Sanderson [00:09:55]: Yeah, it's actually pretty interesting now that you bring that up, what it sort of feels like back in the seventies and in the eighties, there was sort of a very defined split between sort of the DBA, the sort of relational operational database owner, and you're sort of a data steward that was thinking a lot more carefully around things like data modeling. And they had a strong opinion on whether Ralph Kimball's data marts are the right thing to do, or did they follow Ben Andman? And they were focused more on the data warehouse. And it feels like over time, those two sides of sort of the more production data modeling, software engineering skillset, and the more, like, philosophical data steward, data strategists have started to come closer and closer together. And now as, like, data engineers, it feels like we're having these conversations a lot more. Whereas I think when I was first starting my career, at least on the operational side, it wasn't something that teams were really talking about all that much.
Maggie Hays [00:11:11]: It was a lot of like, a lot of what I started with Washington, you know, kind of setting the business requirements upfront and just handing it over for someone else to deploy.
Chad Sanderson [00:11:19]: Right.
Maggie Hays [00:11:19]: So it's very much kind of like the business was driving, the business needs were driving how that data was ultimately modeled. And what I've seen is it's been really interesting to kind of see that relax that kind of requirement, relax, because it's so much easier to produce or transform or store, kind of get data in and out to where you don't need to. It's like lower risk or lower time to value there. But then on the other end of that, when you have poorly modeled data, it's difficult to actually manipulate. Right. So it's interesting to see you still have people who are staunchly in the Kimbell camp. Right. Like, it doesn't matter what, what your storage layer looks like, it doesn't matter, you know, what tool you're using.
Maggie Hays [00:11:58]: You really need to have the foundational data model, but those things take time, right. So it's like the trade off of having those conversations around really well modeled data for analytics or for kind of data science use cases. And I see that debate just still kind of, we haven't settled that debate on figuring out how we, we kind of sequence all these things together.
Christophe Blefari [00:12:20]: But there is, I think there is an issue because, like, data teams are often seen as cost center, whereas, like software engineering team or like proper product team, I would say produce something that makes money, whereas, like, data team is often like doing like, reporting or stuff like this, but it doesn't make money, it just show numbers. And I think this is something I've said a lot of time that to me, like, a lot of data teams are still in their existential crisis. They didn't find, like, their goal in the company. So they say yes to everything they say they are like, doing shadow it, trying to find their purpose within the company. And so that leads to poorly designed, poorly wrong data models, wrong, no contract, whatever, because they just say yes. They do it on a rush and they want to provide you actually, and still the same. Actually, it was the same ten years ago, it's still the same today.
Benjamin Rogojan [00:13:21]: That's exactly where I was going to go. So it feels like what you're saying is not much has changed. We've just changed. Maybe the platform and the technology, but the people problems remain the same. Like, if you were to ask, like, what's some of the biggest changes right over the last decade or so, it's like just the adoption of cloud has been, you know, it just takes time, right? Like when, whenever, when things were launched and now, you know, things take time and then you're just seeing, I think the other thing is that a larger range of companies now have things like a data warehouse. Whereas before it was like, okay, do you have $5 million to sign up for Teradata? Okay. If not, you're just doing a SQL server, you know, underneath someone's desk and you're willing to pay someone for it. And you can't access your data because you have, like in ERp that either you don't have access to the database or the only way you can access it is through some sort of Excel plugin that lets you export data.
Benjamin Rogojan [00:14:12]: I actually had that for one of the places I worked where it was limited to the row count of I don't know how many powers.
Maggie Hays [00:14:19]: 56,000 or something, right?
Benjamin Rogojan [00:14:20]: Exactly. It was like 56,000. And it was like, okay, so just that, you know, and so you couldn't even export all the data, and it was just such a hassle. So, you know, I think that's kind of when the changes is like the technology platform has changed, but somehow we're still kind of, we're still trying to somehow figure it out. I think in some spaces or what I think sometimes happens is like the, you know, the people doing the data work are, you know, still inexperienced when they're coming in, so they're just learning, and then they're kind of going through the same lessons that someone went through that maybe has that 20 or 30 years of experience that maybe is working either for a larger company or maybe just was like, data is exhausting, so I'm going to go do something.
Chad Sanderson [00:15:04]: I think you're, I think you're, I would agree and disagree. I think that you're absolutely right that the platforms have changed, the technologies have changed, and the cultural problems have. I think there are a lot of the same cultural problems, but I think the nature of the cultural problems have changed as well. So, for example, back in the 1990s when everything was on Prem and you're running teradata or something like that, there was a cost, there was a cost barrier to who could actually have a meaningful data warehouse. You had to be willing to stand up servers and buy these tools and hire DBA, hire data. Architects have, like, some way of doing ETL, which is also a very cost intensive process. And so it limited the number of companies that had sort of real data warehouses and were doing analytics and sort of early stage early days machine learning to your like fords and your nikes and, you know, these like multi billion dollar firms in a lot of cases. And in that world, not only was it limiting the number of companies, but it was also severely limiting the amount of data that you actually had to work with because of that same cost constraint.
Chad Sanderson [00:16:28]: And that lack of data created a very different organizational environment. The data architect, I think, had a much bigger role in 1995 than they do at most companies today. And they were effectively the bridge between what was happening in the transactional systems and what was going on in the analytical systems. And they were sort of thinking about both, like, what is our entity model? What are the domains that we're creating? How does that actually map to our, to our database structure? How are we getting that data to our customers? And what does the catalog look like back then? You could say something like, you could say like, oh, I'm going to join this company as a data steward. And it wouldn't seem weird, but if you said that today.
Maggie Hays [00:17:11]: Yeah, everyone's a data steward at this point, right? And some flavor of it.
Chad Sanderson [00:17:16]: Yeah.
Christophe Blefari [00:17:19]: Actually.
Chad Sanderson [00:17:20]: Yeah, exactly. That's just to say, I think that the cloud has opened, making storage and compute more or less a relatively solved problem, not a totally solved problem. Of course, there's always going to be this long tail of issues and how exactly do we optimize it? But solve to the point that your average business doesn't have to, it doesn't become an impossible wall to climb over. Just to have a basic data warehouse has created a totally different set of challenges, which is, okay, we're living in this new federated world where we're all kind of throwing data over the fence because it's super cheap to do that now. A data steward can't manage that anymore. And I think you start to see the rise of the data engineer in that world. Whereas in the previous world they may not have been.
Christophe Blefari [00:18:09]: Yeah, I think there are two pieces of the puzzle. There is like the hardware part with storage and compute that is like very at low cost today. So you can do a lot of stuff. And there is as well like the pyramid of software, I would say, because back in the seventies or eighties, you could not do like a group by into like three terabytes of data with a single SQL query and do it like in a wink. It's like related to storage and compute, but it's also related to the software and the stuff that people factorized and the stuff that we built. On top, on top, on top, on top with all the software that we have today and the frameworks and the distributed stuff and so on, I guess, yeah.
Maggie Hays [00:18:57]: One thing I'm seeing a lot in the data, or like in the open source community for data hub is a lot of these challenges around kind of like governing or kind of compliance practices to make sure that all the data you're producing is well documented or well, kind of like contained and well understood. I think a lot of the practices that were established in the early days of big data, we haven't really evolved those as much. We haven't kept up with the speed in which you can create net new data resources, plug and play, spin up a data warehouse, connect all of your tools, and suddenly it's there. And so one of the things that I'm really excited to see is kind of how we, how we start to really speed up those processes around or those workflows around governance and compliance, because we're just simply not keeping up. And I don't know if that's anything that you guys have seen on your side, if there's any kind of tooling solutions at the crux of it. I think it is a very human centric problem of figuring out how we collectively govern these assets. But, yeah. Curious if that's something that's popped up in y'all's realms at all.
Benjamin Rogojan [00:20:07]: Yeah, I mean, I think, you know, I've definitely seen plenty of it from a consulting side. You know, you often come in and people kind of are either, either through the fact that they've kind of got what I call like a key person dependency issue, where maybe they had someone who built all the pipelines using some sort of like either local tool or something of that nature that wasn't necessarily built with any form of governance, which just kind of like built, uh, often, or what I've heard it called by Joe Reese is like query driven approach or just in time kind of data modeling where there's no real data model. You're just kind of building it for one specific use case. Um, I, I think that you do, you do see that happen, uh, more often just because, you know, the tools are easier. Everyone can kind of easily ingest data. I think to some degree it's not necessarily bad. It's just you have to figure out how to control, like once something becomes more productive, like this is part of production or this is part of something that's getting used a lot more often. I think then there's this often need to figure out, okay, well, how do we now put this maybe more towards the data engineering team versus having someone in marketing analytics try to manage it? Or is this something that we can model? Because on one side, you want people to be able to do their work and do it well and do it quickly to deliver, but on the other side, you don't want to end up a year down the line and have 10,001 off data pipelines that are now being then depended upon for something else, that are then being dependent on Pog for something else.
Benjamin Rogojan [00:21:34]: And then some small change, like Chad mentioned earlier, someone changes a schema file and all of that breaks down. And that was all somehow connected to some machine learning model that suddenly your ad spends is crazy expensive for some weird reason that you're going to have to go spend time figuring out.
Chad Sanderson [00:21:53]: Yeah, I think there's, I sort of like to think of data infrastructure in three big categories. So your bottom most foundational layer is storage and compute. Then your second layer is data. So it's basically like, where do we put all of our data, and how do we run queries on top of it? And then the second layer is data movement. Like the value of data is you produce it sort of over here. And generally, whoever is the producer of that data does not get maximum utility out of it. That utility comes from sharing that data with other people in the organization, and they may have different use cases for it. So we have to figure out how to get the data from, from this person to a consumer.
Chad Sanderson [00:22:38]: And that includes things like the actual transformation, the loading of the data, something like a streaming would sort of fall into this category, and then sitting on top of that is okay. Now that people can access data really whenever they need it, however they need it, we need some way to manage it. And that includes sort of the quality control, governance, compliance, these types of systems. And then I think of the layer on top of that as sort of like data applications, right? Like, how are you actually using that well managed data in order to accomplish some business task? I think that set of things like those set of categories was totally true. It was needed and necessary in the on Prem world as well. And in the on prem world, because the datasets were so much smaller, companies built their entire internal data ecosystem, and they did all of their hiring around those four things, sort of around the premise that, like, storage is incredibly expensive. Therefore, it's actually cheaper to do all this transformation in one place by a group of specialists. And it's cheaper to have a data steward who is thinking, who is manually adding all the information about the catalog and the PII although that wasn't really a big deal back then, but all the other access control policies and things like that.
Chad Sanderson [00:24:04]: And this is how the modern data organization was formed. And then the cloud happened. And basically every single CTO at every company in the world said, we need to make our data team more like software engineers, because software engineers are actually the ones that are starting to make us more money than these data people. That used not to be the case. It used to be that data people made the company way more money on the technology side than the software people. But then apps became a big thing and, like websites and e commerce became a really big thing, and so it totally shifted. And they said, all right, how do we make sort of our data team and our engineering team that works with data more similar to software engineering? And that means we need to go fast. It has to be super fast.
Chad Sanderson [00:24:51]: Everyone needs to be very isolated and fragmented, and they need to be able to work independently because that's how software engineers build features, right? You silo them and you say you go work separately on your own and you go really, really quick. That's basically what happened. Everyone started organizing their data teams that way. They started organizing data production that way, where every producer is generating data from their database or their events, and they're pushing it into cloud storage, and you don't really know where it's coming from and you don't really know the quality of it. And that makes governance a very, very hard thing. So I think that, maggie, to go back to your question of what have we sort of seen as successful in governing data today? I think what it's going to take is almost like a ground up approach. And that is to say, what does it actually mean to govern data in a federated ecosystem? That's a very different sort of thought process. And I think that's why tools like DBT and five, Tran and Snowflake have been so successful, because they've rethought, I what storage and compute means in a federated ecosystem, or what transformations mean in a federated ecosystem, or what orchestration means in a federated ecosystem.
Chad Sanderson [00:26:08]: And I think now we're sort of arriving at that, taking the same approach to that data management layer.
Christophe Blefari [00:26:18]: Yeah, that makes sense. Actually, there is something that changed as well, like in the, compared to the seventies or eighties, is that the amount of data that we get right now is kind of crazy. Like we generate way more data than years before. And actually it created a requirement that we didn't have before, and so we have to be faster, more efficient and so it created, like, a requirement for, like, our tools to be better. And it's gonna continue, actually. And in that, there is something I love to say when I talk, for instance, about DBT. DBT is a great tool to govern data assets, but DBT is so easy to use for analysts, that creates more assets. It creates a lot of entropy.
Christophe Blefari [00:27:10]: And for instance, if you have a data team with ten analysts, they create, I don't know, five models per week. At the end of the year, it's going to be already a mess. And so at some point, you need someone that say, stop. You have to do a factorization, you have to rethink, and you have to rethink to refactorize, to redo something. You accept entropy for a few months, and then at some point someone say, no, stop now. Do it better.
Maggie Hays [00:27:39]: It is really interesting if we think about in kind of the same software engineering principles, it does empower analysts to move much more quickly if we treat a data set or a model as a feature, there's one specific use case. Cool. It's super easy to go build that out and produce it. I think the part that's so drastically different between an app feature and a DBT model is actually measuring the value or the interaction with that and deciding when to deprecate that feature. If you roll something out to your mobile app, you have a way to monitor, does anybody use it? Are we moving them through our funnel effectively? Are we creating a user experience that encourages people to come back and engage, et cetera, et cetera. Whereas with the data model, it could just sit there. Right? And so what do you do? You look at query activity. You look at maybe how often it's referenced in looker or tableau or whatever that is.
Maggie Hays [00:28:37]: But what I've seen again and again and again, and this is true when I was tech lead for a data team as well, there wasn't really a consequence to maintaining or to keeping them live. And it took more kind of cognitive overhead to say, well, do we need this? What's the strategy around it? So the incremental cost of deprecating it was actually a pretty large, well, we might use that, or the stakeholder said it was really important, so I don't want to deprecate it and make them mad until you get to the point where you have thousands of interdependent models and it's just a nightmare to maintain. So I think on kind of like the software engineering principles of it, I would love for us to get to a point where there's like true pattern, like true development patterns where we say we build this and it's in, you know, you have your staging environment, your dev environment, your prod environment, but then also like your deprecation strategy, because the cost of maintaining just one net new model week over week, not a big deal, but years later, it's so much to untangle. It's so much to untangle.
Christophe Blefari [00:29:41]: Face the reality and look at the usage.
Benjamin Rogojan [00:29:45]: I'm like writing that down. Like, that's a good next, like, article.
Maggie Hays [00:29:48]: Or thought, hey, wait, I'm a co author on that one.
Benjamin Rogojan [00:29:51]: Ben, deprecation strategy.
Maggie Hays [00:29:55]: No, it's huge, though, right? Because it's just, it's a different type of tech debt that software engineers don't. It's a different way of thinking about tech debt that doesn't neatly fit into the kind of software engineering playbook.
Chad Sanderson [00:30:11]: I think one of the reasons that is the case is because software engineers and data teams sort of approach writing code very differently. And I think we're finally starting to realize that these are actually like relatively different disciplines in the software engineering world. It's the things that the code that you write is generally driven by a very clear set of requirements. Those requirements map one, one typically with external facing customer value. So someone says, hey, my customers are asking me for a dropdown list because they're having trouble finding items in their cart or whatever. Then you go out, you build that, you come up with a set of specifications, you deploy it, then you check to see if customers are indeed using it the way that you want. And if they are great, you roll it out. And if they're not using it the right way, you go, crap, let's roll it back.
Chad Sanderson [00:31:08]: That clearly wasn't the right thing. So it's very easy to sort of map software to value, whereas in Dataland, it's not really like that. Data almost always starts with a question, right? You have a question about the world. You say, well, I wonder, I want to understand, x, how customers are interacting with this functionality or how marketing performance has been over the last three or four years. And the answer to that question could be valuable. Like, it could ultimately lead to something that makes a company a lot of money, or it could be totally non valuable where you're like, oh, okay, that didn't really tell me anything. So that's a very different type of approach to building software, to your point, where it's more about experimentation than it is sort of following this very linear trajectory towards deployment.
Christophe Blefari [00:32:00]: There is like another axis, which is a question, is very short lived. Like, you have a question and you want the answer in five minutes, and in ten minutes, it may be too late. Actually, you don't care about the answer. Like, in ten minutes or in one day or one week. It's like, for now.
Benjamin Rogojan [00:32:15]: Yeah, I have questions. So does anyone like, in terms of timing for this panel? Oh, there we go. He's speed.
Maggie Hays [00:32:25]: So you're going to have to cut us off, because we could keep going.
Adam Becker [00:32:28]: I want you to keep going, because, I mean. I mean, I wrote down a bunch of different. You guys think you're co authors, but I wrote down all these new ideas for articles that I'm gonna write after this.
Maggie Hays [00:32:37]: Wait, hold on. We're co authors?
Adam Becker [00:32:43]: It wasn't a fine print. You didn't see it?
Maggie Hays [00:32:45]: Oh, no.
Chad Sanderson [00:32:46]: Is that what this is? Adam, this is just so.
Adam Becker [00:32:50]: Actually, there's a question somebody had here in the chat, or at least a thought. Brian, put this in the. In the chat, you need to have your teammates take turns being data model pruners. Have you ever experimented with that? What? And if so, do you have any thoughts on that?
Maggie Hays [00:33:06]: We did something similar at Braintree when I was a tech lead there, where we kind of cycle through owning those data, like, kind of owning the data modeling approach. And honestly, it was a really valuable experience for, I mean, tenfold reasons. But number one, it really. It forced us to continually refine our kind of core data models that fed into hundreds and thousands of resources. But two, it also was because we're on a centralized team, it was so beneficial because it exposed other team members to other parts of the business. And so there was just, like, so much embedded context sharing in that which really removed it from being, well, this person knows this part of the company, that person knows that part of the company, and if one of them's out of office, we're screwed. Right. So it was extremely valuable to cycle through it, but it was something that, it took a lot of time to build out that practice, and it led to us potentially moving slower on stakeholder requests.
Maggie Hays [00:34:04]: So we had to really show value or kind of like, demonstrate why that was worth the investment. But I highly, highly recommend taking a similar approach. It was extremely valuable for us.
Christophe Blefari [00:34:18]: Thank you.
Adam Becker [00:34:18]: Yeah, I have another. This is, like, another thought that came to mind. We're discussing the difference between data that is often used to answer questions versus features that are much more commensurate, and they react to value that users can get more immediately. Some of the things that you've been talking about is the consequences of this distinction, it can be a little bit more messy and perhaps entropically unstable to just continue to amass more and more of these answers to potentially interesting questions. At the same time, do you think it's more likely to be the case that we will move towards a paradigm where we parse what data actually provided value or so that the data kind of moves to seem more like a feature or the features that we build? And I'm mostly thinking about startups building features in a pre product market fit world where in a sense, you are also asking questions.
Maggie Hays [00:35:21]: Oh, uh oh.
Adam Becker [00:35:24]: Oh, me. Am I back? Am I back?
Maggie Hays [00:35:28]: You're back.
Christophe Blefari [00:35:28]: Yes, you're back.
Benjamin Rogojan [00:35:31]: You're back, you're back, Eric.
Maggie Hays [00:35:32]: You're gone.
Benjamin Rogojan [00:35:37]: Back and forth, back and forth.
Adam Becker [00:35:39]: Oh, no. What's going on here? You know what? I was, I was saying something that might be offensive to some of the data gods.
Maggie Hays [00:35:47]: Yeah.
Adam Becker [00:35:48]: Okay. I'll summarize this quickly as the question. Do you think it's more likely that data will begin to function more like features in the sense that it's very important that we attribute value to each data or model or data set that we put in, or that data, or that we will start to see features as more experimental and conjectural in answering particular questions, business questions. And I'm mostly thinking like, in the paradigm of, let's say, like startups and like, pre product market fit, where almost everything you're coming up with in terms of features is somewhat conjectural. It's, it is. Or at least ideally answering some kind of like, hypothesis. So do you have a sense of which way we're likely to move?
Benjamin Rogojan [00:36:37]: Sorry, go ahead. There's a little bit of a split because there are some aspects that sometimes you do have data that you might be using to build into a feature. Maybe it's for an ML model, maybe it's, there's, the other side where it's like, okay, if it's building a report, or as Christoph mentioned earlier, it's like, we just need to know this question right now, in the next two minutes, and then after that, the value of it dissipates. I think there's, I don't think it's going to push towards any way. I just think there's, there's different places that data adds value, and some of it is in a feature, and some of it is just this ethereal like we know, so we can make a choice.
Maggie Hays [00:37:11]: I kind of think of that. It makes me think of kind of like a metric layer or like a metric store, semantic layer where I actually think we could start thinking about those as features, where it does go through many stages of refinement iteration, but then it becomes a building block by which everything else is kind of framed. Right. So I just, I wonder if we start thinking about kind of like components of data modeling or components of data, kind of like specific calculations or kind of standardized ways to measure, like customer lifetime value, right. You go through it takes you a while to figure out, what does that mean for the business? How do we measure it? What are our inputs? The feature, kind of, that output is maybe something that starts to evolve more into a feature where kind of the, the ultimate output remains the same even, and is more like interoperable with the evolution of your data ecosystem over time.
Chad Sanderson [00:38:08]: Yeah, I think this is a really interesting topic. I would love it if the business started to look at our data model, at their data model, more like a product. Unfortunately, I don't think that will ever happen until you can trace a very clear line from the data model to a dollar in a bank, because that's exactly what you can do with a software feature, and that's how ctos and other engineering leaders make resourcing decisions. They basically say, well, I know exactly how many people I should hire because it's going to get me x amount of ROI on this product that we're deploying by this date. So I think that this is why the data product phenomenon is becoming so exciting for data teams, is because we're moving away from just saying, hey, we're doing data modeling for the sake of it, or we're doing data quality for the sake of it, because it's the right thing to do. And we're actually doing it because data needs to be treated like a product, because it can be traced to that value. My view is that in the same way, like an application has a front end and a back end, right? You have a front end that is a customer interface, and they go in and they type some things and they click on buttons and they get value out of that. And then you have this really big, complex, gnarly backend system with databases and a whole bunch of other things.
Chad Sanderson [00:39:28]: I think that data products will effectively have that same sort of relationship where you've got a front end and the front end might be a dashboard, it might be the prediction of a machine learning model. And the backend is your pipeline, it is your data model. It's all the stuff that ultimately produces the thing that a customer interacts with. And once we start doing a better job, sort of categorizing what are the data products in our ecosystem like? What are the again, the data product is the front end and the back end. So what are the pipelines altogether that are money and creating value for our customers that we can tie to a dollar in a bank? Then I think we're going to start to see this convergence between the way that software engineers sort of take accountability and responsibility and visibility for their products and the way that data teams do. And then, Adam, to your point, I agree. I totally think that engineers will start getting more experimental as well. So there's probably going to be a bit of a bi directional convergence here over time.
Adam Becker [00:40:28]: Guys, thank you very much for this. I promise I will at least tag you or mention you.
Maggie Hays [00:40:37]: Not a problem. Excited to see it.
Adam Becker [00:40:40]: Thank you very much. This was fascinating. I'm sure we could keep going for many more hours.
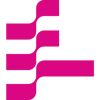