Doxing the Dark Web // Paco Nathan // AI in Production 2025
speaker
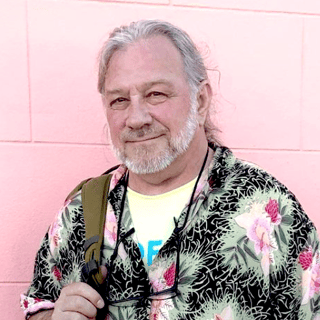
Paco leads DevRel for the Entity Resolved Knowledge Graph practice area at Senzing.com and is a computer scientist with +40 years of tech industry experience and core expertise in data science, machine learning, natural language, and graph technologies. He's the author of numerous books, videos, and tutorials about these topics. Paco hosts the monthly "Graph Power Hour!" webinar, and joins Ben Lorica for a monthly AI recap on "The Data Exchange" podcast.
SUMMARY
The Dark Web: an estimated $3T USD flows annually through shell corps and tax havens worldwide -- serving as the perpetua mobilia for oligarchs, funding illegal weapons transfers, mercenaries, human trafficking at scale, anti-democracy campaigns, cyber attacks at global scale, even illegal fishing fleets. Tendrils of kleptocracy extend through the heart of London, reaching into many of the VC firms in Silicon Valley, and now into the White House. The people who "catch bad guys" – investigative journalists, regulators, gov agencies – leverage AI apps to contend with the overwhelming data volumes. Few of those who do "bad guy hunting" get to speak at tech conferences. However, our team provides core technology for this work, and we can use open source, open models, and open data to illustrate. How technology gets used to stick the moves of the world's worst organized crime, how to fight against the oligarchs who use complex networks to hide their grift. This talk explores known cases, tradecraft employed, and open data sources for fighting against kleptocracy. Moreover, we'll look at where AI and Data professionals are very much needed, where you can get involved.
TRANSCRIPT
Demetrios [00:00:06]: Let's get my man Paco on here. Where is he? Where is he? There he is.
Paco Nathan [00:00:12]: Hi. Hi to meet you. How's it going?
Demetrios [00:00:14]: You're. How you doing?
Paco Nathan [00:00:17]: Gracias. All right. I'm loving. I'm loving all the. The French videos in the woods.
Demetrios [00:00:28]: I want to give you a huge shout out, Paco, because you helped so much in getting some of these great speakers that we have today and you yourself are one of the great. So I feel honored that you're here and I also want to thank you so much for all the hard work that you did.
Paco Nathan [00:00:44]: Oh, thank you very kindly. I've been loving all the talks today. It's just fantastic.
Demetrios [00:00:49]: Cool, cool. Yeah. And then you have to sit through a little bit of this good old random French guy that comes through. But I see that you've got your screen shared already. I'm very excited about docs in the dark money. I am hoping that you can give me some strategies on how I can launder money more effectively. But probably. Yes, that's what this talk is about.
Paco Nathan [00:01:16]: We've got to get hub repo for that.
Demetrios [00:01:18]: Yeah. Oh, nice. I like it. Well, right on, man. I'll be back in 20 minutes to ask you some questions.
Paco Nathan [00:01:25]: Okay, thank you very much. Thank you very kindly. Let me get into play mode. Alrighty. Hopefully that's coming across in full screen and I will get started. My name is Paco Nathan and. Not yet.
Demetrios [00:01:43]: Yeah, press presentation mode. I still see the sides. On the side.
Paco Nathan [00:01:52]: Is that. It's this weird thing where you, you, you don't get the window to select from.
Demetrios [00:02:00]: Yeah, it's a little bit more. Sometimes you can get complex. Oh, you know what this calls for?
Paco Nathan [00:02:08]: What?
Demetrios [00:02:10]: Oh, wait, I think it's in full screen. I still see the slides on the left though.
Paco Nathan [00:02:15]: Yeah, I'm not sure that I'm going to get around that if I. Because I only have one screen for.
Demetrios [00:02:21]: You, so we can. All right, no worries. All right, here we are.
Paco Nathan [00:02:24]: Should be good. Hi, my name is Paco and I'm from Senzing and I'm here to talk about dark money. There's also. I should mention my slides. I, I put a link for. There's a much larger deck and so I put a link for that in chat if you want to grab it off the QR code here also. And I'll put this up at the end too. But this talk is about, you know, what we call dark money there.
Paco Nathan [00:02:54]: There's this web of money flows. It's about $3 trillion per year, estimated in terms of like B2B. And it's really a story about oligarchs and a lot of dirty deeds. A lot of things like illegal weapons trade, human trafficking, even funding illegal fishing fleets. But certainly a lot of bribes and a lot of money stolen from other peoples. And then hey, you know, they go and they buy nice condos in Miami or Manhattan or something. So what I want to talk about though is that, you know, there are people who do this work all day, every day to investigate and try to prevent this kind of thing and, and our company works with them very much. So a lot of the people who do this kind of work in, whether they're in investigative journalism or some areas of intelligence or law enforcement, a lot of these folks probably won't be getting up to give talks because they work with super important areas and you know, it's super confidential as well.
Paco Nathan [00:04:01]: I work with a lot of these folks. I can get up and show use of open data and open models and open source to illustrate what's going on and try to highlight this and also provide some resources. We have some Demos here on GitHub we can show you also a lot of resources if you're interested in getting involved in this. But the main takeaway is that there's a lot of crime happening and it happens behind networks, it happens behind graphs and the used networks like shell companies, so that you can think of the order complexity of investigation. You know, it goes up dramatically. There's just an overwhelming amount of data and then they try to scramble the links between the data as well as part of the tradecraft for committing crimes. So what we're going to talk about is using entity resolution graph technologies and also the downstream AI apps that are being brought to bear to kind of focus a lens on what's happening with dark money flows, how this is powering kleptocracy and how we can leverage AI tools to bring it back to human scale. Also a lot of what I'm talking about, it's getting used in anti money laundering.
Paco Nathan [00:05:12]: But the same kind of techniques also work for customer360 or patient360 or understanding hotel loyalty programs, things like that. As far as who I am, I'll drop this in here. I have a weird background going back decades and got to being on the ground floor. Early work in neural networks, early work in neural network hardware accelerators, early work in cloud. I was a databricks when we all fit in one room. And now I'm at sensing. Okay, so my talk is about investigative graphs in particular. And you're going to see a lot of this in popular media.
Paco Nathan [00:05:51]: There's some great books. There's Autocracy Inc. By Anne Applebaum and also Moneyland by Oliver Bulow 2 that I highly recommend. There's a Netflix movie called the Laundromat which kind of goes through little vignettes, but it covers a lot of it, has Gary Oldman and Antonio Banderas as like the bad guys. You've probably heard about Panama Papers, Paradise Papers. There's a organization called icij. We'll be looking at some of their data. And more recently, I highly, highly, highly recommend Carol Cadwalader.
Paco Nathan [00:06:24]: She's the investigative journalist who wrangled a lot with Putin and also with Farage. She's the one who broke the story about Cambridge Analytica way back when and the Russian influence campaigns to push Brexit. And now she's got a new series called how to Survive the Broligarchy, which highly, highly recommended about this area. So let me just focus on what's called Azerbaijani Laundromat. This is a case from about 12 years ago. It was about $3 billion of money laundering. And that is to put it into perspective, this was over a two year period. So this represented 1/20 of 1% of the money laundering that's going on in the world.
Paco Nathan [00:07:10]: And yet it was $3 trillion or $3 billion worth. And the, the gist here was that leaders in the Azerbaijani government, they were buying weapons from Russia, they were getting kickbacks personally under the table from Russia, and they're also stealing money off of public projects. And then they would funnel that through about 400 different companies, scramble it around through their shell companies and then dump it out into four shell companies in the uk and then they went out and bribed people. And nobody would have known except for there was a whistleblower who leaked 17,000 transactions about money transfers from Danske Bank. And there's a really great interactive series about this. It's powered on link curious, but it's by an organization called the Sentry, which does investigative journalism. So if you want to dig in and see a graph that you can work with, here's the link. I'm going to focus more though on, you know, what, what we're doing to look at the data and similar kinds of cases.
Paco Nathan [00:08:07]: So I want to point to a talk by a friend of mine, Friedrich Lindenberg, friend of ours we worked with a lot. He's at Open Sanctions based in Berlin. And Friedrich, in this talk is talking about how there's, there's a kind of general data model that we see. On the left you've got risk data. So this is like known people who are known to be doing dirty deeds or organizations that are known to be doing dirty deeds and they're on some sort of sanctions list or watch list. And then in the middle you've got link data that says you'll hear this talked about in terms of like benefit, ultimate beneficial ownership, UBO or just understanding like which individuals own what shares of what companies, which companies own other companies, who's a director, who's really wielding influence and that helps to build a graph. And we've had excellent graph talks today. I'm not going to go too much into the graph side because this is all about graphs.
Paco Nathan [00:09:06]: And anyway, shout out to Amy and David and Alessandro and Weidong and others and Jessica, all who've done really excellent graph talks. On the right hand side though, we talk about event data. And so you really need to put the risk data, the link data and the event data together to get a full picture of the crime, the event data. Unfortunately, banks do not release their money transfer data or even like suspicious activity reports inside of a bank. When one of those gets filed, it's about €50,000 to get processed. I was told to try to integrate some of that data. I asked what the schema was. I got yelled at for asking a bank what the schema of their SAR was.
Paco Nathan [00:09:46]: It's just so highly confidential, it's really crazy. But we have, from a Whistleblower, we have 17,000 transactions we can leverage to investigate. So I work in an area called entity resolution and it's a bit, it's foundational for this. And let me explain why. Our company, it's called Senzing, the founder got a start. Jeff Jonas started out at the Mirage casino in Las Vegas back in the 80s. Even before it opened. A lot of the people, the core team has been together, working together on these kind of problems for more than 20 years.
Paco Nathan [00:10:20]: If you've ever seen the movie 21 starring Kevin Spacey, we were the bad guys in that movie. There was a movie about MIT math students who go and do card counting in Vegas and they get busted. We got our start by busting people like that and we got acquired by IBM, but then we got spun out in 2016 and now we've got about 35 people. 60% of what we do, 60% of our end use cases are in air gapped environments. So for public sector these are in skiffs. And it adds a lot of complexity to MLOps kinds of considerations when you're working in a completely air gapped environment. So let me just describe what we do. If you look at this Diagram, I've got three records, I've got Bob Smith II, Robert Smith Jr.
Paco Nathan [00:11:08]: Bob Smith, aka Bobby Jones as a known alias. And you've got those three records, but then a fourth one comes in for Bob Jones and there's some matches on addresses and phone numbers and whatnot. If you're just trying to match record to record and you're just doing like fuzzy string comparison on the different fields, you'll miss this. You won't see that these are connected. And so that's really the problem with trying to illustrate. We take an approach called entity centric learning. It's a more holistic view where we look at all the attributes and features across multiple data sets, we kind of triangulate on them and we build out an overlay of entities and other entities they might be related to. And then also pointing to data records which are evidence because a lot of this, you know, you're going to have to go in front of a judge and and provide evidence for why you want to send out investigators.
Paco Nathan [00:12:00]: So again, trying to take a more holistic view of it. And to illustrate this, this is a kind of tradecraft that gets practiced in money laundering a lot. Let's say you've got Bob Jones and he's known to do some bad things. So he's on a list. Here's his address and his phone number. He's going to go out and use an approach called channel separation where he opens up multiple accounts on your system. Each time he opens up a new account, his PII data is going to drift just a little tiny bit, enough to sneak in under the wire. But eventually in the middle there, he's opening up a new account.
Paco Nathan [00:12:35]: And by this point the data has drifted so much that he's constructed a completely different identity by using entity centric learning approaches. Then we can triangulate on this and show this is one person. He's on a watch list. You need to take action. And the edge cases are where it's really hard because working globally, like consider, you know, here's someone named Abdullah Kardash. If he's made a pilgrimage, he may have a prefix al Haj. If his parent is someone famous, he may have a suffix bin Amir. And by doing entity resolution, we can drill down and figure out these are different names represented for the same person.
Paco Nathan [00:13:14]: But I mean, if I were to take Abdullah Kaldash and translate from Alokothara rabia into English, that translation could go several different ways. So like string matches just don't work. But then down at the bottom, I should mention about the majority of voter registration in the United States gets handled using our SDK. And so look at like John R. Smith versus John E. Smith. If you think about that in terms of voter registration, those are different people. And so the takeaway here is that cultural norms determine what kind of comparisons have to be made.
Paco Nathan [00:13:48]: And we support 80 different globalizations for these kind of cultural norms. And a lot of them are not intuitive, believe me, not what you would guess. And similarly, you know, here's an address, I've been by this address in Singapore, I've driven by, I will say I'm not a customer, if you know, you know. But here's like two different representations and basically there's different cultures in Singapore. So the street abbreviations get really weird. We're able to show that, okay, these are the same addresses for the same business. And then we can take a look at the bottom. Here's this, a third representation for that address compared to one of its neighbors, they're only two letters off, but they are different addresses.
Paco Nathan [00:14:32]: So it's really mind bending to try to understand how to handle the entity resolution. There's a lot of big name customers and whatnot, but I really want to point to the data providers because we work a lot with open data and commercial data providers. Many of them make slices available if you want to get in and start working in this area and try it out. In particular, we've recently published some that are mixes of different data in say Moscow, Vegas, London, etc. The thing is that when you run entity resolution across multiple data sets, you're looking for the footprint of entities across multi data sets. But the way we think of it is it's a way of generating the building blocks for constructing a graph or at least constructing the trusted backbone for our graph. So the output when you run any resolution is you're going to get entities and relations and properties, you're going to get pointers, relations out to other entities that maybe we don't have enough data to say it's a match, but if you are doing an investigation, this would be a good lead. So think about building blocks for generating knowledge graphs out of any resolution.
Paco Nathan [00:15:45]: And there's some great case studies. Alessandro from graphaware was giving a talk earlier. David Hughes from Enterprise Knowledge was giving you a talk earlier. Plenty of others I want to show. There's a couple that I'LL point to here. This is a case study turning congressional hearing transcripts into knowledge graphs by Waihau. And they used our entity resolution to try to understand the different people who wall being mentioned and sorry. And then here's another one recently from Ernst and Young also they're doing work with us to modernize county court systems.
Paco Nathan [00:16:23]: So, you know, if you've ever had a traffic stop and they find out that you have multiple parking tickets and you know, suddenly your participation in the legal system is getting escalated. But then there's some data problems and nobody knows when your court data is going to be. This is kind of what happens on the ground. And ey is using any resolution, knowledge graphs and AI apps to go in and clean up a lot of that data and try to make it more fair. Another thing that I'll point to is just real quick, this is out of the Panama Papers. There's a kind of pattern that we see with graph analytics. Not necessarily graph queries or graph rag, but graph algorithms and visualization. We see this kind of pattern where you use entity resolution to pull the pieces together.
Paco Nathan [00:17:14]: And you might use some sort of algorithm like Louvain to do partitioning into subgraphs. And once you've got that, then you can use other algorithms like centrality. I mean, think of like PageRank basically centrality, where you can rank the real crucial players within a subgraph and then take all that and run it through case management tools. That's typically what would happen inside of a bank. And here I'm showing some data from open sanctions and open ownership, which are two excellent sources for open data in this field. Without using entity resolution, they're already connected, but they're not completely connected. Once you pull it all together, you find out there's this one guy, Avacyn Badshah, and he's kind of the, the center. It's like, you know, Avacyne Batshaw owns some shell companies.
Paco Nathan [00:18:03]: His spouse Rihanna Badshah owns some other shell companies. They're, they're doing a lot of bogus invoices in between. And as it turns out, he was, he was convicted of really large scale or fairly large scale money laundering. He can no longer be a corporate officer. He's on a watch list. So it's this idea of we can use graph algorithms once we can pull the data data together to really focus a light on what we think might be the most important points to investigate. And the criminal activity is trying to hide behind a network, but we can leverage algorithms and AI applications on the networks to really Surface the parts and make it much more human scale. There's also great auditing tools if you want to drill down and find out why and how these decisions were made.
Paco Nathan [00:18:49]: In particular here about Abyssin Batra and his different ownerships. I I do want to stress if you're listening to different talks a day in natural language, you'll hear a lot about NER named entity recognition. And that's different. That's where you. You take noun phrases that are parsed from raw text and you apply some kind of semantic label to them. And Gleiner is a really excellent state of the art zero shot model for doing nervous. Entity resolution is where you're looking at entities across multiple structured data sources and you're using PII features, typically name, birthday, tax code affiliations, et cetera. So NER and ER are different and then in between.
Paco Nathan [00:19:34]: Entity linking is a way to pull these together so you can build graphs that have a backbone from structured data and then layer in elements from unstructured data and then do better AI applications downstream. This is a typical kind of application, a generalized approach where we're leveraging entity resolution to help augment the graph at the top. We're also generating essentially a thesaurus of your specific data, your domain specific entities, definitions, linkages. We can customize an entity linker that way when you go in and do your text chunking, you can also parse the text and build a graph using any R, but then run any linker to pull all the graph elements and the vector elements together as best as possible. I'll show a demo of that later on, but that's for downstream cases where you would bring in a prompt and go in a couple of different directions. In the regulated environments, they really want to see this unbundled. There's a lot of reasons, but the main reason is you do not want to go to a judge and say, your honor, we kicked down the door and shot five people because the LLM told us to do so. I mean, that's just not going to cut it.
Paco Nathan [00:20:47]: You want to make sure you're making the right decisions at every step. And our kind of stuff gets used all day every day by law enforcement and intelligence and military to make those kind of decisions. So you want to do that as best as possible. I wrote an article at O'Reilly recently about understanding the graph in Graph Rag that goes in more detail about this and just for demos real quick. I know we're coming up on time, but if you want to work with entity resolution, we've got A Docker Quick start. There's a lot that's up on Docker Hub that you can use. And we have a lot of different products, different repos up on GitHub too to show illustrations. But this is one of the best ones to get started.
Paco Nathan [00:21:28]: And then there's a series of articles about entity resolution and knowledge graphs and downstream AI apps. I did one based off of PPP loan fraud in Vegas during the pandemic. And then my colleague Claire Sullivan took that same data and code and extended with LangChain to create a chatbot where you can explore fraud. And she shows some really interesting insights about what was going on in Vegas during the pandemic. Different kinds of fraud. We have some other demos that talk about how do you bring in unstructured data if you've already got this backbone from entity resolution with structured data in your knowledge graph and there's also code for that too. Then my colleague who's here today, he did a really fantastic tutorial open source project taking data from ICIJ, Panama Papers, etc. Running through sensing to do entity resolution and then working with Lance DB to build a customized entity linker inside a spacing pipeline.
Paco Nathan [00:22:30]: So take a look at this. If you've run entity resolution on say open sanctions and open ownership, you have these watch lists, you know about who some of the bad guys are. You've built a graph. Now when you're starting to look at. For instance, here are is an article about the Azerbaijani Laundromat. If you parse the raw text about this, you look in the pink rectangles, those are identifiers. These extracted entities from raw text are being automatically linked into the backbone from the results of entity resolution. It's a nice embedding trick by using the results out of ER.
Paco Nathan [00:23:09]: So definitely check out Louis work there up on GitHub. More recently, Claire Sullivan and I have been looking at temporal graphs. And so this is a temporal graph of the Azerbaijani Laundromat. We did some forensic accounting and then flow analysis of how are they, how are they actually orchestrating the money transfers to launder $3 billion. And so they dump money into like about 420 different companies, shell companies, they scatter it around and then they dump all of that into four drains, which are companies in the UK that they took the $3 billion out of to go off and pay bribes and buy condos. Long story short, we're building up simulations to do synthetic data for money laundering so that we can hide it like a needle in a haystack within Legit data and then do red team, blue team analysis. So more to come on that if you're interested in this area, and I apologize, my voice is kind of going, but if you're interested in this area, we've got some great resources. And my larger deck has more.
Paco Nathan [00:24:19]: But follow the money is from open sanctions. And they need data experts, open ownership. It has the BODS standard, working with GLEIF and others. They need people to get involved, too. ICIJ and occpr, they take leaks from banks, law firms, et cetera, from whistleblowers, and they convert it into data, which then gets used to Fight Club Autocracy. They need help. And if you're interested in human trafficking certification, there's some great resources as well. Amy Hodler has been here and gave a talk earlier.
Paco Nathan [00:24:53]: And so shout out to Amy. We've got a wonderful study group at Graph Geeks about investigative graphs. And I know a number of people from the group are here, Franique and others. And for what it's worth, I, I, I do a webinar every month with experts. We got one coming up next week with Thibaut Galam from Linkurious. We'll be talking about new integrations there. And please link up with me on LinkedIn and I'll jump over to questions. Thank you very much.
Demetrios [00:25:27]: Excellent, man. Thanks so much for this. I think it's absolutely amazing that you get to do this as a job. I just gotta say that right now it is very cool.
Paco Nathan [00:25:40]: Thank you kindly. It's kind of scary, though, when you start to see, like, what these people are up to and, and then you're like, oh, wait, wait, we could do that. Wait a minute. I mean, yeah, it really plays with your mind.
Demetrios [00:25:54]: So there's a question coming through from Nico. Can you discuss limitations on accessing data?
Paco Nathan [00:26:03]: Okay, great. Okay. So that's an excellent question, certainly, when it comes to the risk side of the data. And I'm going back to Friedrich Lindenberg's model, the risk data and the link data, those are open like we now. I live in California. I have a corporation, my former consulting firm, and as of this year, it's a felony if I don't disclose who owns what percentage of that company. And so around the world, we're getting more and more of this kind of like, ultimate beneficial ownership data being disclosed and, you know, shout out to like, Stephen Abbott Pugh and others who are really pushing the charge on this. But around the world we're finding out, like, uncovering what's going on with shell companies.
Paco Nathan [00:26:49]: And then the watch lists are public, but the event data, log data, suspicious activity reports, money transfers, all that, it's super confidential, you just almost never see it. And so that's why we really, you know, if you are working in the bank, you can work with their data or maybe you've got some whistleblower data you can work with. That's why we're interested in synthetic data in this area. But the thing is it really has a lot of applications in Graph Rag and Agentix. I can't name names, but I know very large agencies that are doing some really fantastic work in Graph Rag and using a lot of open source tools because there's so much information floating around the world in like little weird news reports. But they also knew who the people in the companies are. So if you can start to piece it all together, you can use AI as a tool to really empower going after the bad guys.
Demetrios [00:27:38]: Oh yeah, that's fascinating. Okay, so I also had a question because it feels like you're going to be at constant battle with PII and folks don't want to give you this information in a way because it's like, hey, that's leaking pii. Or is that, you know, is that not so?
Paco Nathan [00:28:05]: No, that's a, that's a fantastic question. The reality is that we work environments where there is pii. In fact, it's based on pii. Yeah, so I mean, if you're talking about, let's say hypothetically a very large tax agency which may or may not be on one continent or another, they, they have some of your pii, believe me. And if you're talking about a bank, what's interesting is yes, they also have PII for you've applied to have a credit card. They know who you are and where and they know a lot of different addresses. They have your credit history and they're buying data to juice that up. If you're a marketing firm and you're trying to like clean up your customer leads, maybe not pii, but you might buy data from, I don't know, maybe like SafeGraph or somebody, some commercial provider to try to clean up your data.
Paco Nathan [00:28:54]: So yeah, actually we really rely on PII and understanding like the do's and don'ts because we are totally under all kinds of compliance and I mean, you should. I'm filling out an expense report for Florida last, last week and it's just crazy how we have to be super squeaky clean. Just even filling out an expense report is crazy here because we just have to do Everything the right way.
Demetrios [00:29:19]: Yeah. So the last thing that I wanted to ask you was around, I think I've seen a company that does something similar to this, but on the blockchain. It's like chainalysis or something. Have you heard of them?
Paco Nathan [00:29:32]: Well, I. I was on the phone with a group of people who were. Yes. Working on blockchain. And I. I shouldn't say exactly who because there's another. There are two other companies that are helping provide them and. And they may or may not be customers of ours.
Paco Nathan [00:29:47]: So yeah, we're kind of a weird company because we're like 35 people, been together for a long time and we only do. We do this one C SDK and it's got like Java bindings and Python bindings. We don't do any other source code other than just like, you know, here's a demo on GitHub. We just do that one library and it can run standalone because it has to run in air gap and we don't do any professional services. And like all of our customer support is free, our prices are published. But the reason is that we partner far and wide. And so like I say, this gets used by other organizations. You know, the majority of voter registration in the U.S.
Paco Nathan [00:30:32]: tax agencies in a lot of the English speaking world, a lot of police departments, a lot of law enforcement, counterterrorism, that kind of stuff.
Demetrios [00:30:42]: Yeah, there's so cool. So, all right, we're gonna keep it moving. But man, I love this and I love talking to you. I'm excited we're gonna have you back on the podcast. I know we already that. So if you're intrigued by this topic, come and listen to the ML Ops community podcast and you can hear Paco and I chat more at length about this. And yeah, man, thanks as always.
Paco Nathan [00:31:11]: Thank you so much. Demetrius, this is fantastic conference.
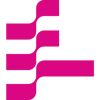