Enhancing AI Quality for Content Understanding in Visual Data
speakers
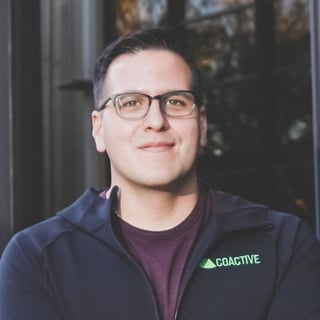
Will is currently the Co-Founder of Coactive AI, helping enterprises unlock the value from their visual data. A former Data Scientist at eBay, Will has previously held various roles as a visiting researcher. His most recent work focuses on the intersection of AI and data systems, including performance benchmarks for data-centric AI and computer vision (e.g., DataPerf @ ICML 2022, the Dollar Street dataset @ NeurIPS 2022). His previous work spans from IoT electronics to design and performance benchmarking of deep learning in neuromorphic systems. Will holds a PhD from Northwestern University and a BS from MIT.
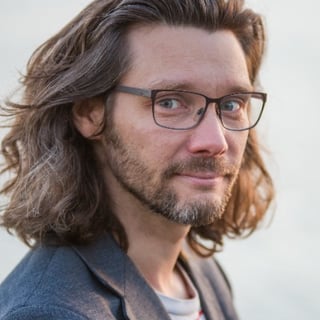
Florent Blachot is the VP of Data Science & Engineering at Fandom, the world's largest fan platform. In his role, Flo leads the data science and data engineering teams that define the global data vision for Fandom’s portfolio of businesses. He is tasked with building data products and services that enhance revenue and user experience and improving the advertising and engagement features across Fandom’s portfolio of businesses. Flo is based in San Francisco and reports to Adil Adjmal, Chief Technology Officer at Fandom.
Prior to joining Fandom, Flo worked as the Director of Data Science at Ubisoft, a video game company with several development studios across the world. Reporting to the VP of Audience Management and Acquisition, he managed and grew a data science department, delivered actionable insights to all marketing departments, and enabled direct uses of data for CRM, media, and digital marketing.
SUMMARY
In this presentation, we'll unpack how multimodal AI enhances content understanding of visual data, which is pivotal for ensuring quality and trust in digital communities. Through our work to date, we find that striking a balance between model sophistication and transparency is key to fostering trust, alongside the ability to swiftly adapt to evolving user behaviors and moderation standards. We'll discuss the overall importance of AI quality in this context, focusing on interpretability and feedback mechanisms needed to achieve these goals. More broadly, we'll also highlight how AI quality forms the foundation for the transformative impact of multimodal AI in creating safer digital environments.
TRANSCRIPT
Will Gaviria Rojas [00:00:10]: My name is Will Gaviria Rojas. I'm one of the co-founders of Coactive AI, and I'm joined here by Flo, who is the VP of data science and engineering at Fandom. We have a really awesome presentation for you today. We are going to talk about a really interesting use case around applying multimodal AIH for visual content moderation. So a trust and safety system. We're going to talk about everything from the business use case to, you know, what are some of the things in the weeds of what it took to actually bring that system to production. Without further ado, I'll pass the mic over to Flo, who will get us started.
Florent Blachot [00:00:43]: Thank you, Will. So first I will talk about the business case of Fandom, and then will we go into the more detail of the technical aspects. How do we solve it? So first, what is Fandom? Fandom is a very large wiki platform. I'm sure you know what Wikipedia is. So Fandom is a spin-off of Wikipedia. There is about 350 million users every month and a bit more than 250,000 wikis hosted there. So a lot of content, tens of millions of articles that are there. Like the biggest wiki of Star wars, for instance, is there.
Florent Blachot [00:01:20]: And we are regularly ranked in the top 25 sites worldwide and particularly in the US, and even more during COVID where everybody was watching Netflix, playing video games and so on. And so, as every wiki, everyone can come up and add content like text, but also images. And for images, we have about 2.2 million images every month. And unfortunately, we have some bad actors, like either knowingly, people that are putting something that is mild against policy, but so we have also some trolls that are going very hard and to bombard, for instance, my little pony, with porn images, which is exactly what we don't want for that. And so to do to that, we are going to do a lot of manual moderation. It's about 500 hours per week, which is extremely time intensive, but it's also a lot of mental health impact for the users that are going to see and even more for the staff that is watching all those images all time long. So even if it's a very small amount, we want to be extremely cautious, we want to be extremely good for our users. And most of the things that exist off the shelf don't work.
Florent Blachot [00:02:42]: This is one example. What I can show, there is many that I cannot, but this one is extremely specific. Like we have Klingons, or we have many aliens. Spaces of images, and images of alien spaces on fandom. And nearly every, not every off-the-shelf trust and safety are going to label that as blood or anything that is around violence. So it's obviously something that we want to let passed other elements. And it's a specificity of fandom. We are hosting so many different communities.
Florent Blachot [00:03:20]: We can have Game of Thrones and malital pony, and for them you want threshold, you want different elements that are completely different. So you want also something that is different. You can accept some mild nudity on a Game of Thrones while on my little pony, you have zero tolerance over it. And so what we were wishing to do is have something that is fully automated, that will not like minimize the impact on human. But there's also those elements that are extremely domain specific to fandom, where we can have alliance, where we can have differentiation between different type of communities. And we want to be at this scale quite notice it's not Facebook scale or like this big social company, but it's still a big scale. And so we put five different evaluation points. So the first one is, the most important one is precision and in the sense of MLE, but more in the sense of we want to approve of, reject most of the images as much as we can.
Florent Blachot [00:04:29]: But more importantly, we want to not approve automatically every images that is likely bad. So this is like our first point for that. We have a couple of other elements that are around like SLA cost and so on that are extremely natural that you have when you want to settle ML system. The last piece is also evolution is we want to start with customer safety, making sure that we can erase images, but we want also to do more. So it's typically adding features that will label if an image is about a background, a landscape, a specific character and so on. And like this, we can build engagement feature from there. And so this is where we started to discuss with multiple companies, but more importantly with coactive, where we finally to work with. And so we went with three different phases with them.
Florent Blachot [00:05:25]: The first two proof of concept and then the integration with feedback. So the first proof of concept was just an in synchronous one. We give them access to 20 million images that were labeled between approved or rejected. And we let them train a model that was able to do that. We also look at their UI and UI tool and we were wishing to build concepts to check can we evolve beyond user safety? And I did one that was extremely cool at the time. That was can we recognize Star Trek crew member against Star Trek Star wars crew member? And it was extremely accurate to do it. Then we went into the second month of proof of concept. That was a live testing.
Florent Blachot [00:06:15]: So the flow of data images were going directly through coactive, but it was not filtering out or approving from scratch the images already. And when then we went to the integration where we started, when we had confidence, we went to integration, we built that and it continued to improve over time. Where now we have about 80% of 90% of images that are approved automatically. So when we are looking at the results, so 90% of the images are approved. We have no bad images that is approved automatically. So we have this about 10% buckets of images where we say we still need manual review for them. We are not sure SLA cost is grids cost particularly. We were just thinking about can we break even here we have divided by two, including the cost of steel manual labor that is there.
Florent Blachot [00:07:13]: It was extremely fast and we are also confident that it will be successful to scale up to other business cases. And with that in mind, I will ask will to come up and do the next.
Will Gaviria Rojas [00:07:26]: Awesome. Thank you, Flo, for the overview. This is an AI conference, after all. So we want to at least get somewhat into the weeds or to the degree that we can in a few minutes. And I want to actually cover some of the nuances of actually trying to solve this real use case that was very important. So the first thing is, let's define what AI quality meant in this context. Right. Again, we have a tremendous amount of data, but we're trying to find a very small fraction that is the problematic content, and we definitely cannot miss any of the bad stuff.
Will Gaviria Rojas [00:07:56]: So in this case, AI quality was really came down to what is the quality of the training on this specific task, and what's the adaptability as both the new categories emerge or the data changes? And so the journey from that zero to 90% that Flo was mentioning, there were three crucial design choices. These are somewhat obvious at a face value. You have to select the right model, you have to do some initial training, and then you have to have some sort of notion of ongoing feedback. But we dive a layer deeper. There's three lessons I want to pass on to the team that maybe anyone that's tackling a similar challenge. The first one is, what we found here is that the data was very nuanced. It covered a lot of different everything from cartoons to live action shows. And also, surprisingly, there were actually a lot of problematic images, that it wasn't the visual content, it would just be a Twitter snapshot in which the text was hugely racist or hugely just messed up.
Will Gaviria Rojas [00:08:52]: And so it actually involved having some notion of a model that could understand and pick up text. Right. Because visually, it just looked like a Twitter picture, but if you read it, it was horrific stuff that shouldn't be on the Internet. So, with that, we actually found that multimodal foundation models were the best choice to start us off, and that got us to about 65% of the way there. Right. But that wasn't enough. Right. The next piece that we had to do is then, well, how do we then leverage? How do we do training? Right, but how do we do it in this setting with these very few data samples? And for that, we had to do something that is essentially active learning that's augmented by a retriever.
Will Gaviria Rojas [00:09:26]: And I'll go over that in a second. And the last piece is, how do we keep going and give the feedback over time, as human reviewers give you more and more input and as you start seeing new and probably worse edge cases. And that's where a foundation model and adapter architecture were really critical in being able to decouple the expensive piece of the foundation model from that final fine tuned layer for the specific tasks that were at hand. I've gone to a lot of talks, and I've seen a lot of people talk about number one and three. So for this talk, I wanted to talk about two. If you are curious about one and three, feel free to come up to me. I'm happy to answer any questions. I have a lot of thoughts.
Will Gaviria Rojas [00:10:03]: I think I only have three minutes, so I'll go over this second one. And so what's the challenge? If you're doing active learning in a traditional active learning setting, you have some sort of training set. You have some sort of data set, and you have some sort of model, and you're trying to essentially figure out, hey, what are the best data points to add to my training set for this model? The challenge here is that you have, again, 2.2 million images, but less than 0.1% of the images are of the target class of what you're trying to do. Not just that, but these break out into about 25 different categories that have nuance. Right? Like, actually, I'll remain from giving you guys the specifics of, like, the difference between gore or others or some of the other categories, but there was a lot of nuance that wasn't just visually obvious. And so you have this combination, and what it equals is you have rare classes that are very hard to pick out and very hard to build a training set out of. And so this is where you have to shift your focus from the traditional approach of focusing on data quantity. Right.
Will Gaviria Rojas [00:11:00]: Because if you do that, it's just extremely computationally prohibitive to absolutely do right, just running a single uncertainty sampling round on the entire data set, which is cost prohibitive. So you really have to actually have a very data centric approach here and actually focus on the quality as opposed to the quantity. And if you do that, you can actually get to a point where it's actually efficient and feasible to be able to train this final adapter layer to do this specific task, to actually give you a natural implementation of how to do this. How we approached it for this one is to actually not just do active learning on the entire set space, but to limit it to a specific neighbors. And so this is kind of what we're calling here is this retrieve augmented active learning. In the paper we call that seals. Feel free to check out our position paper overall, on the advantages of using this kind of data-centric AI approaches for data selection. And feel free to check out the specific algorithm.
Will Gaviria Rojas [00:11:51]: But just to give you at a glance, what we did is we had only a few samples of the target class. We then randomly selected samples from the rest of the space, which actually gave us a really good training set. Overall, this was a starting training set. Then, rather than doing, we then trained a model. But then, rather than doing uncertainty sampling over the entire space, we actually limited to just the K nearest neighbors. To give you an example, it goes from having to do uncertainty sampling on 2.2 million data points, versus if you have a training set of 100, and you do, the k equals tens of the nearest neighbors. Now you only have to do it over a thousand, so it's much more computationally efficient. And it turns out it actually performs extremely well.
Will Gaviria Rojas [00:12:32]: It's a relatively simple algorithm, because then you do it over the space, you rank them by uncertainty, you label the most certain ones, and then you just repeat the steps. Right. And so by repeating these steps, it makes it really simple for a human that's actually labeling the edge cases to prevent provide that feedback. And number two, we made it really simple to go from only a few label data points to a full fledged system that was actually able to capture all the nuances of the categorization of what fandom was looking for. And so with that said, again, come up to me if you'd like to chat some more about kind of what we did. But overall, if you're interested in kind of just how to apply multimodal AI at scale, this is what we do at Coactiv, we're actively hiring. We're also actively in the market. If you're looking for a solution, we really helped power, essentially search, tagging, and analytics on your visual content, anything that's images and video.
Will Gaviria Rojas [00:13:17]: And, you know, one of the big approaches is we have a solution that's ready. It's on the marketplace. And you, you know, we went in production in just under 30 days just to give you a sense of the power of the platform as it exists today. With that said, a huge thank you all for for joining us here. I think now is the official end of AI QCon. So thank you for coming. And, you know, if you're interested in getting in touch with us, please scan the QR code or come talk to us after the talk. Thank you all for your time.
Florent Blachot [00:13:46]: Thank you.
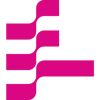