From MVP to Production Panel
speakers
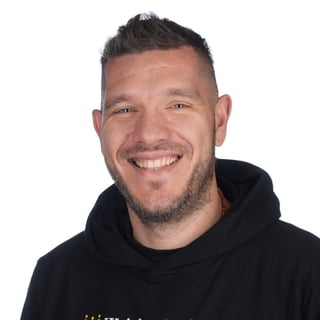
Alex Volkov is an AI Evangelist at Weights & Biases, celebrated for his expertise in clarifying the complexities of AI and advocating for its beneficial uses. He is the founder and host of ThursdAI, a weekly newsletter, and podcast that explores the latest in AI, its practical applications, open-source, and innovation. With a solid foundation as an AI startup founder and 20 years in full-stack software engineering, Alex offers a deep well of experience and insight into AI innovation.
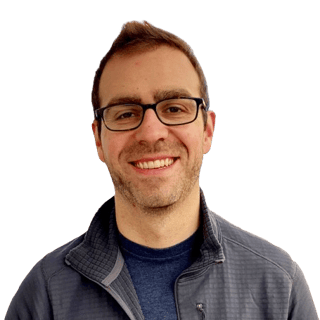
Product management leader and 2x founder with experience in enterprise products, data, and machine learning. Currently building tools for generative AI @ Databricks.
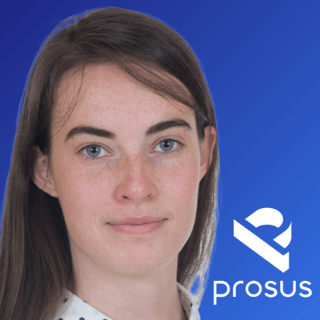
Focused in building AI powered products that give companies the tools and expertise needed to harness to power of AI in their respective fields.
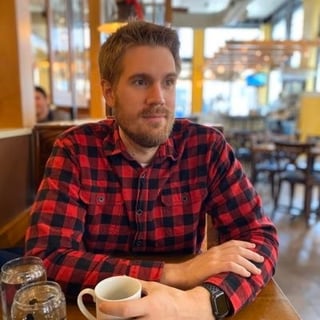
Phillip is on the product team at Honeycomb where he works on a bunch of different developer tooling things. He's an OpenTelemetry maintainer -- chances are if you've read the docs to learn how to use OTel, you've read his words. He's also Honeycomb's (accidental) prompt engineering expert by virtue of building and shipping products that use LLMs. In a past life, he worked on developer tools at Microsoft, helping bring the first cross-platform version of .NET into the world and grow to 5 million active developers. When not doing computer stuff, you'll find Phillip in the mountains riding a snowboard or backpacking in the Cascades.
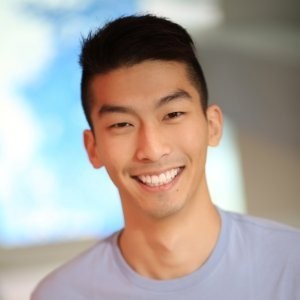
Andrew Hoh is the President and Co-Founder of LastMile AI. Previously, he was a Group PM Manager at Meta AI, driving product for their AI Platform. Previously, he was the Product Manager for the Machine Learning Infrastructure team at Airbnb and a founding team member of Azure Cosmos DB, Microsoft Azure's distributed NoSQL database. He graduated with a BA in Computer Science from Dartmouth College.
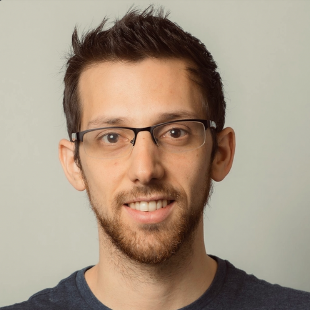
I'm a tech entrepreneur and I spent the last decade founding companies that drive societal change.
I am now building Deep Matter, a startup still in stealth mode...
I was most recently building Telepath, the world's most developer-friendly machine learning platform. Throughout my previous projects, I had learned that building machine learning powered applications is hard - especially hard when you don't have a background in data science. I believe that this is choking innovation, especially in industries that can't support large data teams.
For example, I previously co-founded Call Time AI, where we used Artificial Intelligence to assemble and study the largest database of political contributions. The company powered progressive campaigns from school board to the Presidency. As of October, 2020, we helped Democrats raise tens of millions of dollars. In April of 2021, we sold Call Time to Political Data Inc.. Our success, in large part, is due to our ability to productionize machine learning.
I believe that knowledge is unbounded, and that everything that is not forbidden by laws of nature is achievable, given the right knowledge. This holds immense promise for the future of intelligence and therefore for the future of well-being. I believe that the process of mining knowledge should be done honestly and responsibly, and that wielding it should be done with care. I co-founded Telepath to give more tools to more people to access more knowledge.
I'm fascinated by the relationship between technology, science and history. I graduated from UC Berkeley with degrees in Astrophysics and Classics and have published several papers on those topics. I was previously a researcher at the Getty Villa where I wrote about Ancient Greek math and at the Weizmann Institute, where I researched supernovae.
I currently live in New York City. I enjoy advising startups, thinking about how they can make for an excellent vehicle for addressing the Israeli-Palestinian conflict, and hearing from random folks who stumble on my LinkedIn profile. Reach out, friend!
SUMMARY
Dive into the challenges of scaling AI models from Minimum Viable Product (MVP) to full production. The panel emphasizes the importance of continually updating knowledge and data, citing examples like teaching AI systems nuanced concepts and handling brand name translations. User feedback's role in model training, alongside evaluation steps like human annotation and heuristic-based assessment, was highlighted. The speakers stressed the necessity of tooling for user evaluation, version control, and regular performance updates. Insights on in-house and external tools for annotation and evaluation were shared, providing a comprehensive view of the complexities involved in scaling AI models.
TRANSCRIPT
From MVP to Production Panel
AI in Production
Alex Volkov [00:00:05]: Everyone, my name is Alex Volkov. I'm the host for this panel. Our title of our panel is going to be from MVP to production. And it looks like almost all of us here, I think we're missing one more person. And while she joins, I'm just going to introduce myself briefly and going to ask you guys to introduce yourself as well. As I said, my name is Alex Volkov. I'm an AI of interest with weights and biases. And I'm also the host of the Thursday Eye podcast, a recording of which I just came 30 minutes ago.
Alex Volkov [00:00:32]: So I'm still kind of hyped up about everything that happened in the eye for this week. And I'm here to host a panel on everything from MVP to production. I have an amazing panel here. So we're going to start with, let's say I'm going to ask you guys to just introduce yourself. I guess we'll start with Eric, and then I'm going to call out some names. Introduce yourself, and then we're going to get started.
Eric Peter [00:00:55]: Awesome. Thanks for having me here today. I'm Eric Peter. I'm one of the PM leads on the AI platform databricks. I look after kind of two primary areas. First are our model training capabilities. So what are the tools that we provide to help customers build AI models? That's everything from a simple scikitlearn model through fine tuning large language models, all the way up through kind of pre training, completely from scratch, large language models. Second, I look after retrieval augmented generation.
Eric Peter [00:01:26]: So how do we help customers with that? Rag is the fun buzword. I'd really call it more like AI systems. It's a lot more than retrieval, is what we found out. And we deliver tools there to help folks really build high quality applications. I think that's one of the biggest challenges that we've heard from our customer base. And in both of these efforts, I work really closely with our academic research team, who are kind of working on everything from building their own foundational models to depriving new information retrieval techniques to new programming paradigms for large language models. So excited to be here and chat with everyone.
Alex Volkov [00:02:01]: Awesome. Thank you for joining, Eric. And then I want to ask Don to introduce and say what you're working on.
Donné Stevenson [00:02:09]: All right. Hi, I'm Danae. No worries. I work as a machine learning engineer with process. So yeah, we're working on using llms to create assistance that kind of help portfolio companies. And now we're going public with a product that acts as an AI assistance. And I'm currently focusing on doing that for very specific use cases within the companies that we work with.
Alex Volkov [00:02:41]: Incredible. Andrew. Hey, how are you, man?
Andrew Hoh [00:02:45]: Good, how are you doing? Great, Mike, sounds great. I'm Andrew. I am co founder and CPO of last Mile AI. I used to be the GPM for AI platform at Facebook AI, then did some AI at other companies for that start last mile AI. Last mile AI is a company building generative AI platform. Basically. Been working a lot on ML platforms before now. What is a generative AI platform and how do we help solve that? So, actively thinking about MVP to production and what does production mean for generative AI, and how does it differ from traditional ML? Cool, nice to meet you.
Alex Volkov [00:03:23]: Awesome. Nice to meet you. Andrew and Philip, last but not least, definitely tell us about what you guys are doing at honeycomb.
Phillip Carter [00:03:31]: Hello, my name is Philip. I'm on the product team at Honeycomb. We are not an AI company. Primarily not doing AI stuff. But what I think is quite exemplary of where we're at in this industry right now is you don't have to be an AI company to actually do useful shit with this tech. And some of the things that I do there is I'm kind of sort of the main person who builds and prototypes things and sort of gets them into production in some way, shape, form, or fashion. Started that last year and started driving a little bit into AI observability or large language model observability in particular, and really focusing on how you can use just information from production to directly impact. Well, sorry, I shouldn't say directly impact, directly improve the features that you're actually building and base that not on speculation, but on like, hey, this is what people are actually doing, and this is how we can use that to understand patterns and inputs and outputs and meaningfully improve what we have in production because our users are going to want stuff to get better over time instead of worse.
Alex Volkov [00:04:40]: Awesome. So I wanted to maybe use this as kind of the first talking point, getting feedback from production. So it's been fairly clear that once different APIs were released for JGPT, a lot of excitement, folks. Boards are talking, pushing their ceos like, hey, you have to add AI. What's your AI strategy? Everybody started building mvps, a lot of glowing videos on X and elsewhere, and then putting those production to production in the hands of your users. Suddenly you get things like, well, this doesn't actually work, or this says something that a company is not really aligned with, I guess. Philip, we'll start with you because you mentioned this first. What are some things you've seen that changed since.
Alex Volkov [00:05:23]: Something that you've kind of played internally and kind of presented to stakeholders and then went to production, and then the users kind of showed that there's something there we have to fix. Do you have a moment like this?
Phillip Carter [00:05:35]: Oh, absolutely. I think somebody is quite arrogant if they think that they're going to be able to predict what their users are going to try to do with what they throw into production. It's rather easy, actually, I would say in many cases, not necessarily all cases, but it's rather easy to get something to the point where it's probably good enough to go to production, but that's where the hard stuff actually starts. You're going to find that, at least in my experience, that when you present sort of not even just a blank canvas, but just like a way for someone to input what they actually want to input, like what's closer to their mental model, rather than them having to learn the particular gestures of your particular UI or something like that, you find that they're going to approach your product just differently than they would have if you didn't have that user interface in there in the first place. And so that almost kind of resets expectations around what users are going to want to do and what they're going to try to do. And that gets you to the point where, like, okay, if we want to actually keep this thing in production, we have to take what they're actually doing and what they're getting back. And if there's a way to get it, what they think about that information, like what they're getting back and feed that back into development. And the software industry always talks about, like, oh, yeah, we should be shipping code faster and we should be learning from what we're shipping and all that.
Phillip Carter [00:06:52]: Most teams don't really do that very well, but this cranks it up. You have to do that very well here because otherwise you're just going to ship shit. And nobody wants bad software. That's all janky and doesn't actually do what it's supposed to do. And I think Gen AI in particular makes that a lot harder because you're dealing with things that are, in some cases, sometimes by design, non deterministic, but then also dealing with practically unbounded inputs that anybody could put in that you have kind of no hope of predicting upfront.
Alex Volkov [00:07:25]: I guess I see quite a few folks agree. So I'm going to ask Danae, I saw you kind of go like this for a few items here. Talk to us about how you guys are dealing with the problem of users doing unexpected things or even following back and seeing what users actually did to improve the continued kind of deployment, the next deployment, especially as these models are nondeterministic. I think. I cannot hear you.
Donné Stevenson [00:07:52]: Sorry, I muted myself. Yeah, it's interesting that this was the first thing that came up because that's definitely been for us. This mvp process to production is definitely the place where you're finding, oh, it looked fine. It looked fine. While we tested and we had done due process, we had test sets. We had checked and we tested. But once you give these things to users, they just don't behave in the way that you expect them to. I think that comes from a place of, we're designing with the people who have defined the problem, but not necessarily the people that use it.
Donné Stevenson [00:08:31]: And then we're also giving people blank canvases, and they don't think about these things the same way that we think about them. And they don't always understand kind of what's happening under the hood. And so they treat it as a black box, and black boxes are magic. Right. So it is a challenge, and I think what we've done or what we're working on is kind of what we think of as an evaluation period. So it doesn't have to go from zero to 100. You can release in sort of phases. So stepped releases, give it to.
Donné Stevenson [00:09:07]: If you have an end goal user who is like a very general user, you don't have to go straight to them. You can go to an in between person who has some expectation of what it's going to do, but also some understanding of how it's being built, and they can test it in a way that's a bit closer to real life and get feedback, and you can kind of iterate on that process and gradually work up to your final end user. And this kind of evaluation period is because it's also smaller. You can evaluate on a much deeper level than you could if you're getting hundreds and hundreds of new users at the same time. And I think for us, that's been really useful to get an understanding of how real users are going to use it without risking releasing a product that we don't always understand what's going to happen afterwards.
Alex Volkov [00:09:56]: Yeah, I want to send it to Eric. I say, eric, you're in constant agreement here, and getting that evaluation. Evaluation is a big thing that I would like to ask to stay on for a minute. Getting that evaluation back into your model training and continuous updating, whether if you're serving your own models, whether you're fine tuning some prompts as well. Definitely a big piece of all of this. And in the MVP, at least for some folks who are just building a quick demo, that's not something folks think about, they don't think about. They're like, okay, I wrote my prompts. I put it up.
Alex Volkov [00:10:27]: How have you found this kind of transition, both from the folks who are building kind of maybe agents and retrieval systems where evaluation is hard, but also from putting it out there in the actual users hands and then seeing what they actually do to this and then bring it back and iteratively improve the product?
Eric Peter [00:10:44]: Yeah, I mean, I think the process, now that you kind of decide, hey, giving it to a small group of users who are somewhat close, but not necessarily the full breadth of users, that's what we see a lot of our customers do. We actually call it like the expert stakeholders or the internal stakeholders, where you have four or five experts who are kind of like, maybe they're not the first person who defined the requirements, but they're close enough to actually understand the content. Because a lot of the times for our developers, the challenge they run into is they're like, I actually don't know if this answer is right. I'm building a bot for the HR team or I'm building a bot for the customer support team. And this answer looks good, but I don't actually know. This isn't my day job. I'm a data scientist. I'm not an expert in this.
Eric Peter [00:11:25]: And so having that loop and being able to get really fast cycles from that small group is really important. I think the thing there is having the right tooling to do that is incredibly important because the most brute force way to do that is, hey, here, you can come chat with the thing, write it down on an excel spreadsheet and give me notes. Or that's maybe the second most brute force. The first one is just go play with it and tell me if it's working or not. And what we've heard from a lot of folks will get feedback like, yeah, it doesn't work. I hate it. They're like, well, which ones weren't working? They're like, oh, was that question I asked about? And I was like, well, that's not helpful for me. And so a lot of what we help our customers with is how do we make it really easy to put tooling in place such that every time someone uses it, no matter what, there's a log, there's a full capture of everything that happens.
Eric Peter [00:12:14]: And there's the ability for the end user to quickly send like a thumbs up, thumbs down, along with rationale, and even go as far as edit and look at what was retrieved, along with think, you know. Alex, your point? You said, well, logging and looking at the feedback is one part, but how do you actually measure, like, it's great if I can go look qualitatively through a bunch of things and see that maybe people are happy or unhappy, but what are the metrics I can use? And so I think that's a really important part. And we see both emerging kind of the standard information retrieval metrics that people use, but also this emerging kind of area of LLM judge oriented metrics, where you can actually get pretty detailed feedback from having a model judge these things. And that's not perfect, right. It's not quite the same as a human, but it can give you additional signals to work with and kind of have a suite of metrics to look at.
Alex Volkov [00:13:03]: And I want to ask Andrew as well. Andrew, any of what we're saying resonates with how you approach figuring out what doesn't really quite work for users in production.
Andrew Hoh [00:13:14]: Yeah, so you might have to dig me out if I get too into the weeds, because I've been thinking about this for so long, and we've been working with some customers on this, that I might get a little bit too deep into it. But for evaluation, it really breaks into three possible steps. The first one is you have human annotation, human and loop style, right, which is like audit logs or manual experimentation, where you're able to manually annotate whether the results are right or wrong. Then you have your heuristic based ones, which are like the classic NLP ngram algorithms for figuring out whether the results produce are correct. And then the last one that Eric talked about, which is LLM based or feeded into a large language model. Each one has its pros and cons. Generally, people are thinking more about the annotation style or the LLM one. The problem with the annotation style is pretty expensive.
Andrew Hoh [00:14:01]: You're looking at going to a company and trying to spin that up. But the biggest difference is it's no longer the annotation that people are familiar with, which is like, you can get a lot of people to help annotate now. It's like specialized roles. I was talking to a company, and they mentioned that they have a summarization task. And so part of the annotation process is who can distill 100 pages of material into a single paragraph and annotate that, which is like, it's no longer just someone you find now it's like we need an expert who can process 100 pages of data and really annotate whether the summary is correct. The other approach of LLM is great, but it's quite costly, right? And you're kind of feeding GPT four results, or any LLM back into another LLM, and you're doing some system prompting to try to get it the right results. We found the most progress with actually not using llms, and we use more encoder based models, which are much cheaper. When you think about evaluators, they're just classification problems.
Andrew Hoh [00:14:57]: And so if you can have a really robust classification problem, that's like one, five hundreds of the cost, why not go with that approach? The hardest thing though, is it's so diverse. I think Philip and Donnie mentioned it, which was like, so many people have so many different requirements for it. And so you find evaluation is more less of single metric driven, more of like a composite metric, or many different functions people are optimizing for. And so there's some assertions that go on, which is like, is this JSon or Markdown? There's some content quality, which is, is this faithful to what's retrieved in the original data set? Is this relevant to the answer? And so some composite goes on, and it's almost like industry based. We've been working with different industries, and each one is so nuanced, it's so difficult to do it broadly for everyone. It almost feels like you need a custom one per industry. Hopefully not every company needs a custom one, because that's unscalable.
Alex Volkov [00:15:52]: That's true. And I wanted to dig in into the custom industry one because it's going to be impossible to cover all the possible industries. And the very generic answer may not be the super most helpful one for our listeners here. And maybe I'll go around and ask one industry specific thing that you can think of that maybe in evaluations, like an example that you had to work through in how to actually build an evaluation for. Right. It's fairly difficult. So whoever wants to go first in the industry specific one. Andrew, if you mentioned a few, feel free, but also let's go around the table, just see how we're differing.
Alex Volkov [00:16:27]: There's difference between a lawyer summarization task, for example, and translation for another example.
Andrew Hoh [00:16:34]: I can start off with one of the most classic ones, which is for sales. NASA actually means North America, South America regions, not the NASA that will most likely come up from any LLM, which is our space agency having that context of where evaluation to understand that it's a sales specific system and it should actually use the terminology and vernacular similar for sales and whatever the acronyms are used. And I think all of us who've come from those companies that have a thousand acronyms, Facebook used to have a dedicated internal site for acronyms. You're going to find yourself needing to do that translation and make sure that the answers are correct as opposed to the original training data that is most likely most embedded into the LM. So that's the first example that comes to mind for me.
Alex Volkov [00:17:23]: One example from us is that we've built a bot for translations and then it started translating the links as well. And for example, weights and biases. You don't want brand names to be translated because this in Spanish does not sound like what it needs. Eric, I see, also, having fun with this. Do you have any industry specific examples of what you had to work through in a specific domain? Or, I mean, I can actually just.
Eric Peter [00:17:46]: Give an example from some things we've been doing on our own products for our coding assistant. And so one of the things, obviously we want the coding assistant to be helpful, generically helpful. Right. Well, what does helpful mean? Well, helpful, what we kind of discovered was that if you just ask GPT four to evaluate if an answer was helpful or not, it essentially says close to 100% of the time that what the LLM generated was helpful. It's like, okay, well, they asked a question, here's some response. And so not until you kind of prompted the LLM with kind of very specific guidelines of what does helpful mean. And kind of, you almost set it up as a few shot problem rather than a zero shot type problem. But that's kind of one specific example where you do have to give the model some context.
Eric Peter [00:18:32]: And once you did that, you actually started to see pretty good results in terms of, yeah, there are some helpful and not helpful answers, but I think that pattern probably applies across other, like one, I think one bank told me they're know, Eric, for safety, for us, it means something different than everyone who talks about swearing. It means specific, incorrect infactual information because that's a safety issue for us because we might make a bad trade on it. And so kind of what these definitions actually mean to each person, I see where the LLM judges can be going, and I think where we're going to take them is to make them so that they can start to be tuned with that small number of human labels you get. And that's a lot of the research that we're know, Andrew kind of made the point of they're expensive. Well, the best way to reduce cost is to distill something down to a smaller model. And if you can, while doing that, you can also get something that's like a little, I essentially tune the classifier head on the end of it. You can get a lot better outcome there.
Alex Volkov [00:19:30]: Awesome. Daniel, I want to hear from you as well. I saw that some of this resonates.
Donné Stevenson [00:19:34]: Yeah. So just like this idea of context is really important for the models to do. The right thing is the context, I think, for what we're working on right now is around SQL, but SQL with the actual user's data. And there the issue becomes distilling the context in a way that it can use enough of it without losing itself. And then I think also, again, the challenge of working with consumers directly is a lot of the implied knowledge, a lot of what they know is not always available to the questions they're going to ask. And how do we then map that? Or I think the biggest challenge is get the model to recognize that it doesn't know that and ask for clarification. The abbreviations and the terminology that's so specific, if it can make an inference, it will. And that's getting it to understand that you don't know everything.
Donné Stevenson [00:20:35]: So please check. That's kind of one of the things that's interesting about working with a model that can know so much.
Alex Volkov [00:20:46]: That's true. And I think for many people, definitely the assurance with which, the seriousness with which the model is like, yeah, this is the right answer, and it's not. It confuses users on the other side because users are used to something that it's clear as an answer. Philip, have you ran into these issues as well? What's your take on how to even know when a failure mode fails and how to even grab those information and use them to actually improve some of these evaluation methods? The.
Phillip Carter [00:21:19]: So, yeah, that's a really difficult one. I've primarily been working, when I think of my day to day work, not like user interviews and stuff, primarily with data querying. The problem is data querying is a very generic term. There's like a million different databases out there that all have different ways that they're really good at some stuff and really bad at other stuff. And your LLM does not know the difference between these things at all. It has a passing knowledge of various query languages, but it has no understanding of. Is this actually a good query for the task that I have at hand. I found in my case that the thing that really differentiates someone is, it feels a little bit obvious, but actually being able to run something that the LLM generates and find a way to evaluate how correct or useful it is in tandem with presenting something to someone in some, like, it's a little bit generic, but for example, with honeycomb, we're effectively a data querying platform for your observability data.
Phillip Carter [00:22:29]: A query has a particular shape and there are rules for that. So there's levels of validation and evaluation even before we go and run the query that we can sort of say, okay, here's some hard criteria, if this actually works or not. That can be a surprisingly deep thing to go and solve for because I guarantee you you're going to have your product in some thing that some users kind of want to do. That's kind of awkward to do in your product. And like in Honeycomb's case, saying the words what is my error rate? Is a surprisingly challenging, difficult question to answer. We're really, really good at other questions, but that one maybe not so much. And what is a useful query for that? Trick question, there is no useful query for that, but it still outputs something. Now we're getting into, okay, well, we're giving you something.
Phillip Carter [00:23:18]: Is that something actually helpful? And does that set you on the right path or does that lead you astray somewhere? And that's, I think, a point that a lot of people are going to be heading down towards eventually once they get past the initial hump of like, okay, we're generally doing the right thing. If you want to be really useful for people, especially the people sort of thinking like from a pareto distribution standpoint, like that smaller percentage of things that are a little bit like wonky inputs, but tend to be really important for a lot of people, that's where you're really going to make or break, having a good product experience using this stuff. And that's where I've not found any best practices, really. And it ends up being so domain specific that there are ways that you can quantify if something is good or not, but it ends up being so custom almost that you have to instrument this thing for yourself and you have to bake in some of that domain knowledge about what is generally a useful thing versus what is generally a not useful thing. And if you don't have that domain knowledge from people on your engineering team, your product team and so on, who deeply understands generally what is helpful for customers, even if it's not actually what they're asking for, then that's where you're going to end up struggling a lot because I guarantee you that people with the title of VP and executive staff or whatever are going to expect this stuff to be able to do that. And if it doesn't do that then you're kind of in trouble.
Alex Volkov [00:24:41]: So I want to talk about you mentioned knowledge as well. And I want to talk about specifically if you're building a model or you're using model in production, just a straight up model, you're kind of at the whim of the cut off date when Openei decided to kind of stop the knowledge cut off. October, December 23, whatever. They keep updating this. And if you have a rack system on top of this information knowledge system like Eric said, you're still at a risk of providing some amount of information. Let's say it's not like a live API. Everything sits in the vector store and then that also could go stale. So how are you guys thinking about this additional problem of data ingestion and updates pipeline? How are you keeping the knowledge up to date? I see Andrew, you're agreeing with this and then maybe Eric, let's talk about how we, before we even get this to the users, how do we even make sure that the latest version of our SDK appears there or the latest version of documentation also gets in there and the model actually knows about this.
Alex Volkov [00:25:40]: Andrew, go for it if you want.
Andrew Hoh [00:25:42]: Yeah, big smile. So I'll go first. I mean it sucks. Long story short, it's like a massive version control problem. We abstracted as much out as possible to try to version control everything because there's two things, which is it sucks when it comes updated underneath the hood because then you see some type of performance or distribution change in terms of the performance it was delivering. And it also sucks when the other side, when you want to keep it up to date. How do you refresh it and make sure that you understand when to reevaluate things? The best that we can do is just try to version control everything. Actually, I don't know how many folks here have been part of the recommendation systems for some of these companies.
Andrew Hoh [00:26:29]: It feels very familiar, like you're trying to version control all the infrastructure for retrieval, the data sources, the processing pipelines and then the under LLM. You're trying to fix that version and say this is pin version. If we a b test it, do it with a b, but you've versioned everything and it's like this is getting so gnarly there's no way to roll back because it's going to be so painful. It feels the same. And that's the funny thing, maybe I think I listened a little bit into the talk before and they're talking about how many of the problems seem so familiar to just classic MLS problems. It's funny because it's under the generative AI umbrella, but for all of us, we're just like, yeah, it's the same problem under a different name and it hurts maybe even more.
Eric Peter [00:27:14]: Now, I was going to say, philip, you alluded at the idea of evaluation sets and actually curating for you, kind of like what's a good bad answer? And it's funny because I think maybe a year ago, a lot of people, jennifer, a guy's magic, it's going to change it. We can just put this thing out in production. It's going to be awesome. And I think it's kind of funny to see now the world shifting back to what ML practitioners have been doing for not hundreds of years, whatever, some very large number of years, which is okay. When we start our task, we're going to go curate ground truth evaluation set. We're going to decide what metrics we're going to hill climb on. And now the rest of the world, everyone who's doing generative AI is like, oh wow, actually now I get why that's necessary. And so it's interesting to see, Andrew, to your point, these paradigms that have been around for many years coming back, and it's a much harder problem now because Hill climbing on a regression model with a ground truth set, that ground truth set is relatively straightforward to collect, but hill climbing on non deterministic english language input output is a much harder, you know, Alex, to answer your original question, like keeping things up to, you know, there's obviously you could say, I'm going to retrain my LLM every day or every night.
Eric Peter [00:28:33]: I don't think that's practical. And what we've seen is that is why people start using these systems that are for rag or augmenting the prompt with information. But in our view, what it comes back down to is it's data. Where's your data? How good is your data? Is your data available? Do you have a robust infrastructure to version control it? Do you have a robust infrastructure to deploy it? And at least for us, what we see is this is why a lot of our customers like, yeah, it makes sense to build our generative AI stuff right on top of our data platform because guess what? That's what we need. That's where we have to start. We have to get our vector database in sync with our source systems. And so I think just having those pipelines, having that becomes even more critical these days. Whether you build it on spark or you build it on choose your favorite data pipeline builder and poison.
Adam Becker [00:29:24]: Guys, this has been full of insight. One of the takeaways that I have from this is just, okay, this new slogan, LLM ops. Same problem, new name, but it hurts a lot. My, this is my takeaway. Thank you, guys. I think we have a couple of questions from the chat. One of them is from Jeffrey. What would be the right tooling to capture and annotate user feedback? We've been looking at Argilla, but interested in what else is out there.
Adam Becker [00:29:54]: Any ideas?
Eric Peter [00:29:56]: I think we can all do our shameless plugs.
Adam Becker [00:30:00]: Go for it.
Eric Peter [00:30:01]: We have great tools for that at databricks. That'd be my biased answer.
Adam Becker [00:30:09]: Have you guys mostly just built these things in house? Do you use just like off the shelf?
Phillip Carter [00:30:13]: Yeah, at Honeycomb it's all in house. Granted, since we're an observability company, we have really good observability for our product. And user feedback is basically like a column on an event because we have that sort of rich instrumentation. We can just slice and dice based off of user feedback and splay out like, okay, thumbs down. Result. What was unique about inputs and outputs? Or we know this to be a bad output given an input. What's unique about user feedback related to that? There's probably some more tools that can do that out there. But frankly, if you have pretty robust application observability, it's quite straightforward to add that and then use it within another tool.
Phillip Carter [00:30:58]: Just don't do it. Maybe with one that doesn't handle high cardinality data very well, like datadog, otherwise, or at least be careful about doing it. They have a great product, but that's a way to get your bill to explode. So watch out for that one.
Eric Peter [00:31:12]: It always comes down to the shameless plug.
Andrew Hoh [00:31:15]: It's like the anti plug.
Adam Becker [00:31:21]: Any other answers?
Alex Volkov [00:31:24]: I mean a quick plug. If we're doing shameless plug, everything in width and biases is stored and evaluated within width and biases. We have reports we have an internal bot called wantbot. You guys check it out. It's on slack. I think it's now on part of the custom gpts as well. We can ask and get like a precise answer of how to set up everything with biases end to end. We've open sourced onebot and its evaluations and auto evaluations and also reports how we built it and how we're doing the data pipeline ingestion and auto evaluations as well.
Alex Volkov [00:31:54]: And we mentioned some automatic evaluations like GPT led evaluations and all that data and all of the ability for us to know whether a small change in the vector database or embedding dimensionality or even GPT four and different model. All of this kind of happens and is tracked obviously with weight and my assist. So you can take it from the model training all the way to production and then kind of see end to end, what changed and how it affected your users eventually.
Adam Becker [00:32:24]: Nice. Thank you very much, guys. By the way, in New York City, we ran a workshop the ML community in New York did of how to build the one bot. So that was pretty fun. Awesome. Thank you very much, everybody. Please stick around in the chat in case people have more questions. And it's been a pleasure having you.
Andrew Hoh [00:32:44]: Thank you.
Alex Volkov [00:32:45]: Thank you, everyone. That was a great panel.
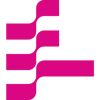