LLM on K8s // Panel 4
speakers
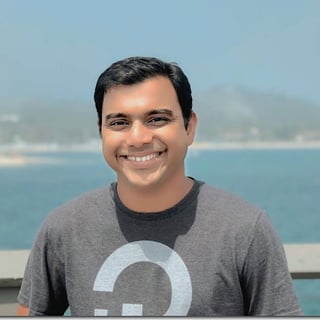
Shri Javadekar is currently an engineer at Outerbounds, focussed on building a fully managed, large-scale platform for running data-intensive ML/AI workloads. Earlier, he spent time trying to start an MLOps company for which he was a co-founder and head of engineering. He led the design, development, and operations of Kubernetes-based infrastructure at Intuit, running thousands of applications, built by hundreds of teams and transacting billions of $$. He has been a founding engineer of the Argo open-source project and also spent precious time at multiple startups that were acquired by large organizations like EMC/Dell and VMWare.
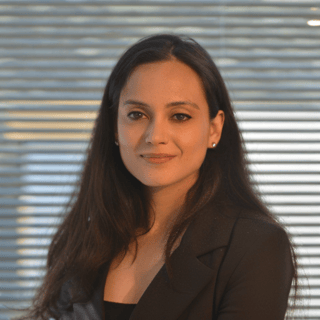
Manjot is an investor at Lightspeed India and focuses on SaaS and enterprise tech. She has had an operating career of over a decade within the space of fintech, SaaS and developer tools spanning various geos such as the US, Singapore and India.
Before joining Lightspeed, Manjot headed Stripe in India, successfully obtaining the payment aggregator license, growing the team from ~10 to 100+ and driving acquisitions in the region during that time.
She started her career as a software engineer at Google building products scaling to billions of users. She then headed product for Kubernetes, one of the fastest growing open source products in history. She moved back to India to pursue her entrepreneurial dreams and started up in the space of machine learning infrastructure in India.
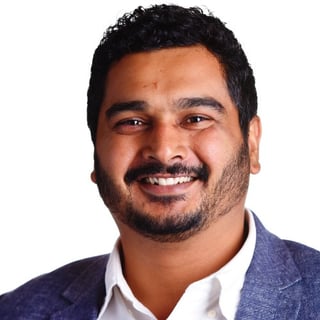
Rahul has 13+ years of experience building AI solutions and leading teams. He is passionate about building Artificial Intelligence (A.I.) solutions for improving the Human Experience. He is currently the founder of A.I. Hero - a platform that helps you train ML models and help improve data quality declaratively. As part of his work, he also helps companies bring LLM Models into production by working on an end-to-end LLM Ops Stack on top of Kubernetes that helps you keep your fine-tuning, data annotation, chat-bot deployment, and other LLM operations in your own VPC.
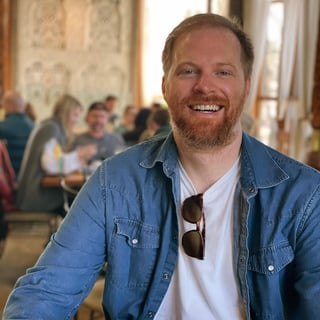
When Patrick is not occupied with building his AI company, he enjoys spending time with his wonderful kids or exploring the hills of Boulder.
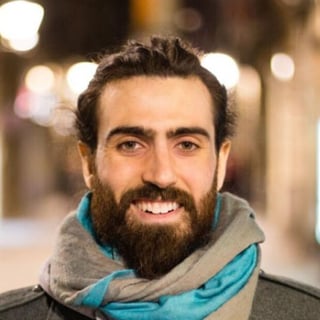
At the moment Demetrios is immersing himself in Machine Learning by interviewing experts from around the world in the weekly MLOps.community meetups. Demetrios is constantly learning and engaging in new activities to get uncomfortable and learn from his mistakes. He tries to bring creativity into every aspect of his life, whether that be analyzing the best paths forward, overcoming obstacles, or building lego houses with his daughter.
SUMMARY
Large Language Models require a new set of tools... or do they? K8s is a beast and we like it that way. How can we best leverage all the battle-hardened tech that k8s has to offer to make sure that our LLMs go brrrrrrr. Let's talk about it in this chat.
TRANSCRIPT
And now that is a perfect segue into, because it feels like that was just like the warmup. That was a little bit of, uh, the sound check before we bring on our next guest. What's up Sri? Doing well, Demetrius, this looks like a great event. Yeah, man. So I've got a shirt that I wanna show you all, but I'm gonna get everyone on stage.
We've got Raul with his new microphone and how you sounding man? Oh yeah, yeah, it's right here. Can, can you get the SMR fields? Yeah, maybe not that much. A little too intimate now, man. We've also got my nos. I think it is very late where you are, so I, but it looks light out. Is it already tomorrow?
Fortunately, I'm traveling to, uh, so I'm in Seattle right now, so it's, uh, it's the same thing going. All right, so that is awesome. And. Then where is my man? Patrick, where there he is. All right. I, why am I in big? I'm not the star of this show. I'm going to as I make myself even bigger now. Patrick. What's up dude?
I love the Martin guitar in the back. That is awesome. I love seeing you all. And sre, I'm gonna leave it off to you. This is kind of a, uh, What we would call a mix between a panel and a fireside chat. We've got so many incredible Kubernetes people in the same virtual space. And while you kick it off, I'm gonna get this shirt.
That is so good. It is not the, I hallucinate more than chat g p t shirt. It is. The, I got it just for everyone here. I don't know if you can see this. I gotta make myself bigger. Not because my ego needs it, but because everyone should be able to see that says Kubernetes is a gateway drug. And that is what we are talking about Kubernetes on, on running LLMs on Kubernetes.
I am going to get off the stage now and I'll drop a link, uh, in case anyone wants to buy that shirt and support the community. Sri, it's all yours, my man. Thank you so much. Thank you so much, Demetrius. Uh, hey. Hello and welcome everyone. Thank you so much for joining this, uh, this panel, panel discussion fireside chat about large language models and Kubernetes.
Uh, you know, one of the more well known instances of, you know, Kubernetes and LLMs coming together is open AI documenting a lot of how they've trained their models and actually serving Chad and Dali and. Other models on Kubernetes. So, uh, I asked to write like a small ous description about, about this session, and this is what it came up with.
In a world full of errors, may your code be bug free. May your containers be slim and efficient, and may they always sail smoothly like a rubber ducky in a giant data lake. Is this even possible or am I hallucinating? Well, well on that, maybe not. So funny note, it's safe to say that there is a lot of work to do, uh, you know, especially when it comes to LMS and humor, and maybe Kubernetes has a role to play in that.
Uh, and that's just the perfect segue for us to kind of get deep into these conversations about how LMS and Kubernetes, who coexist, what do they, what do they, how do they work, and so on. Uh, so before we get into the details, like it might be, you know, a good time to have quick introductions. Uh, man, do you wanna go next?
Introduce yourself. Um, hey, I'm, uh, I'm part of, uh, LFE right now and investing in, um, a lot of these new age NLM companies. Excited about that. But before that, uh, life actually started, uh, as, uh, as an infrastructure engineer where I was building internal infrastructure libraries. Um, at Google. And, uh, from there I became, I became a product manager in the Kubernetes team.
This was, uh, you know, far, far into the Kubernetes 1.0 ages when, uh, we were actually thinking about Mesosphere and other such things. Uh, so it was, uh, working primarily on networking and security, and, uh, eventually wanted to build something of my own. So left and started up in machine learning infrastructure.
So of course, and, uh, was lastly at Stripe. That's fantastic. That's fantastic. Welcome. Uh, Rahul, why don't you introduce yourself? Yeah. Uh, hello everybody. I'm Rahul. I'm the founder of AI Hero. Um, for me, Kubernetes is not just a GA gateway drug. It is a way to just stay high all the time. Right? So, um, I I actively think about, um, how do you, uh, deploy the l lm ops stack?
Apologies. And, uh, to, to use that phrase cuz it's not. Cool yet, but, um, what exactly does, um, training your own, uh, foundational models on Kubernetes look like? What does fine tuning a large language model on Kubernetes look like? Or even deploying applications like the q and as, um, or search based applications, agent applications on Kubernetes and how that can help.
Uh, increase the velocity of, um, what your company delivers or deploys. Is, is, is what I, uh, what I like to work on. Excited to talk to everybody. Awesome. Awesome. Thank you, Raul. Patrick, what about you? Hey, yeah, I'm, uh, I'm working as a architect for a startup. Right now we're focused on LM planning, which has been super fun to do historically.
I've, uh, worked a lot in Kubernetes. I worked for Joe and Craig at a couple Heptio. We get acquired by VMware. I did a lot of stuff. I put some features into Kubernetes and whatnot. Uh, over the last several years, gotten a, you know, traditional kinda machine learning 1.0 and then last, you know, year been focused on generative ai and now really kinda heavily focused on, uh, LM planning.
So. Awesome. Thank you so much. And just for, uh, for completeness, uh, hi everyone. My name is, I am currently an engineer at Outbound. Uh, at Auto Bonds, we are building a, you know, large scale machine learning platform for hand, a fully managed platform for handling, uh, data science and machine learning workloads.
Uh, so yeah, welcome everyone. And, um, maybe we can start off just a little bit about like, what are your impressions of kind of, you know, first impressions of large language models on Kubernetes. Uh, especially maybe with respect to training. Where does commodities come in? What does it offer? What is good?
What's not so good? What do you think?
Sure. Maybe I can kick it off. Um, yeah, go for it. Um, so when Kubernetes, uh, was, uh, I mean as you all might already be aware, Kubernetes is basically inspired by an internal, uh, Google, uh, product called Borg. And, uh, it basically tried to achieve the same objective, which is how do you make sure workloads, you know, are scalable, are reliable, uh, you have containers and how do you have an orchestrator?
So, so that you don't have to manage that infrastructure? And, uh, that's what Kubernetes was promising to do. You probably noticed in that last sentence, I never machine anything related to machine learning. So, so just a historical perspective here. Um, uh, Kubernetes did grow up without keeping ml, ml, uh, core ML in mind.
And it was more about general workloads, that, traditional workloads that you might be running, your APIs, your microservices, et cetera. So it was very microservice heavy, um, and focusing on that architecture. Uh, but for sure, uh, there are lots of, in the past couple of years, this is even before LLMs, um, became super popular.
Uh, in the last couple of years we've been seeing a lot of these, um, you know, um, uh, products, libraries, everything come up that help you, uh, basically run your machine learning workloads on top of, uh, Kubernetes because the principles remain the same. What are the principles? The principles are. Kubernetes gives you, uh, an abstraction layer, an orchestration, orchestration layer on top of your containers that abstract away hardware, right?
So effectively giving you more reliability, scalability, and, and um, um, you know, uh, efficiency out of the box. Yeah. Sounds great. Yeah, I think Raul, you had something to say. Yeah. Yeah. Um, and, and, and maybe I'll take a different, uh, perspective on this one. So, um, A few, like maybe a month ago, um, we were talking in, in this, uh, community called Tribe AI that I'm part of, super intelligent people.
Um, and one of the recurring topics that came up was enterprises are looking to deploy, uh, and train the models in-house cuz they really don't want data to leave, um, their vpc right. And I think that is a business problem for which Kubernetes provides a good solution. Obviously you have vendors, um, the cloud.
Service providers or even startups, um, who have, um, Kubernetes native solutions for you to deploy and train your machine learning models. Um, but this is what you know, um, hopefully what Kubernetes allows you to do is have a cloud agnostic, um, uh, platform for you to train and deploy your machine learning or large language models.
Interesting. One of the, one of the questions, and maybe Patrick, I'd love to hear your thoughts on this, like, one of the perspectives that gets talked about specifically with Kubernetes, uh, is that yes, it has options to run a lot of workloads in machine learning. Domain land up being batch workloads. And there is, yes, Kubernetes offers big support for running batch workloads, but oftentimes it seems like it's a little bit of an overlooked area, or at least something that hasn't yet gotten the attention.
It was always about microservices and orchestration of services that are long running services, running servers, whether it's web servers, database servers, whatnot. But if you are talking about running bad jobs, Yes, there is a Kubernetes job. Go do something about it. The community has come up with other things, things like Argo, um, that have actually enabled some of this, but still not kind of, sort of being the first class citizens for batch workloads.
What's your take on, kind of like, given that a lot of machine learning workloads are batch and Kubernetes offers some support, but not a lot. Is that, is that, is there an impotence mismatch there somewhere? Is there something better that can happen? I mean, I'd say a lot of machine learning 1.0 workloads were batched and I would say more the 2.0, like generative ai, most that's actually streaming.
So I see far, I mean there still is some batch use cases, but I think we moving away from dags primarily into kind of like chains and whatnot. I think that's changed significantly. I would say, you know, what Kubernetes is built for, which is microservices. You can almost think of lms, you know, as they grow as microservices, where you have, you know, these different parts of the models, these partitions that need to talk to each other.
So you need to schedule containers and network them together, right? So it's really the same principles. It's just kinda being thought of different way. So I think Kubernetes actually fits that use case really well. Interesting. Interesting. That's, that's a good use case. Uh, that's a good point. What about things like as we go deeper into kind of LLMs and, you know, one of the backbones almost so to speak, of LLMs has been use of GPOs pretty much every L L M that, at least from things that have, you know, generally been talked about, always talked about how they've been trained on LL m or sorry on GPUs, uh, and then all these other conversations about GPU shortages and whatnot.
You know, does, do you think Kubernetes helps there? Are there things that are better because of Kubernetes for using GPUs and being able to kind of train models there? Or are there, there are lots of other companies nowadays which don't run on, uh, Kubernetes, but still provide you with GPU access. What, what does that happen?
What do you guys think?
Um, oh, go ahead. Yeah, go ahead, go ahead. Yeah, I mean, I think the biggest thing is cost, right? So I've worked a lot with startups, you know, who are trying to build generative AI applications and, you know, some of 'em don't wanna use open AI for, you know, the privacy reasons or whatnot. Uh, and yeah, the cost that you used is, is horrible, right?
I mean, to run these models, it's super expensive to keep these things running. The start of time is super long, right? So it's like you shut it off. You could have, you know, several minutes to try and boot back up and load the model weights into the gpu. Like that could, that can all take a significant amount of time.
So helps with this where, you know, obviously we can, you know, skill notes up and down, uh, you know, try to make that. Start of time as, as fast as possible. Um, but I think there's a lot more work that can be done here. Right now I'm kind of looking at, you know, can we like Hot Swap Laura's or Cue Laura's in the models, right?
So could we, instead of like having to wait to pull up a whole no, could we just run these base models and then hot swap Laura's for special, like fine tuning capabilities. So it's kinda where, where I'm heading right now. Yeah. Yeah.
Uh, yeah, just adding, uh, on top of what Patrick said, right, uh, spot on about cost being one of the bigger constraints and problems that needs to be solved with GPU access, right? So I think, um, um, in the first sort of wave, um, in the first set of set of changes and, and, and these changes are all, you know, weeks apart, but.
Still, uh, just support for gpu, uh, was added, uh, so that you can run, uh, you can enable, uh, gpu, no pools instead of your cpu, no pools, uh, which enables at least some GPUs to be used, but obviously that's not enough. Mm-hmm. Then, you know, you have these newer things like. Spot instances being available, um mm-hmm.
In, in the various cloud that can really help with, uh, you know, making your infrastructure run cheaper. But even that's not enough because, um, just like with everything in infrastructure, the develop is in the details. So if you look at, you know, components of Kubernetes, uh, and this is where, going back to what I said previously, right, it was designed for traditional workloads, not necessarily machine learning workloads.
So going back. Details of how Kubernetes works, something like scheduler, which I think Patrick briefly touched upon how, uh, Kubernetes actually decide to schedule, uh, and provision nodes, pods and everything else, um, is uh, can, can, there can be a lot of optimization over there, uh, because obviously there's.
Cost. And there's also things like, you know, networking, uh, how, uh, how much data is being transferred between different nodes and different pods and, and where they are actually located. Um, um, so secondly, I mean, uh, if you look at autoscaler again, uh, in fact even funnily enough for traditional workloads as well, we used to say auto skater is a, you know, easiest way where you can shoot yourself in the foot.
Uh, I think it's even harder. To, uh, make autoscale work with machine learning, uh, and l l m workloads. Uh, so I think there's a lot of work where templates can be provided, you know, defaults can be configured well. Um, um, and the right sort of parameters can be optimized when auto scaling and L l M based workloads.
Yeah. Uh, just, uh, carrying that, that thread, um, of auto scaling. Um, a couple of weeks ago, um, we participated in a hackathon, uh, where we trained or fine tuned a last language model on top of Kubernetes with R lsf using the DP library. Right. And originally our, our aim was like, Hey, we are gonna do this auto scaling thing and enable, you know, uh, make, make sure that that works.
The, the real challenge right now is GPUs are super, uh, Rare to get hands on. So even if you want to auto sales, you're not gonna get it. So, um, uh, a smart way to think about this is negotiate with your cloud provider to get, uh, to reserve those nodes that you need in advance so that your workloads at least are, are deploying.
Um, and then on the, uh, yeah, basically that's, that's, that's the point with the auto scaling, right? I think the other side where, Uh, the costing issue that Patrick mentioned and, um, we, we, we talked about, I think, and this is speculative on my part, so, um, who we'll know in a couple of months whether this is true or not.
So as you know, that H one hundreds are going to be released and generally available or starting to get adopted, and we are gonna see the hold of. People start migrating to that. And guess what that means? A 100 s are going to be more available, cheaper and so on. Right? And so maybe the market will self correct with availability, but, you know, remains to be seen.
I think for now, um, either you can wait and watch for two months or, you know, plan out a strategy where you, where you reserve your instances and instead of thinking about. Like over optimizing on auto scaling. Um, try to get a repeatable, uh, platform in play, which, uh, in place on which you can rapidly de deploy and iterate on.
I think the iteration part of your, um, model is why, where should you, you should be focused and not on the iterative, like the how correct or how awesome your, uh, Kubernetes platform looks like. Interesting. So thinking about large language models, so Raul brought up this point, uh, a little earlier, that if you think about large language models, kind of, sort of, there are two or three kind of big domains of work.
One is companies or teams starting to train or doing. Training a large language model completely from the ground up, like literally everything from the data to training the whole thing. It can sometimes take months open AI, for example, has documented how it took, I think about three and a half months or something for them to, you know, get it completely right.
And there is fine tuning of the models. And then there is also prompt engineering. So two or three different domains of work. So Raul, how does Kubernetes play a role in this? Does it make it better? Does it, is anyone. Type of work better suited for Kubernetes versus the other. Does that make a difference?
And maybe even with GPUs and, and I, I think as, as architects and people who are, you know, designing these platform level solutions, there's no one right answer. There's always tradeoffs. So the tradeoff, you are mm-hmm. You know, Kubernetes is, is a great platform for you to be vendor agnostic, move fast and, and, and, and, you know, be able to automate a lot of your workloads.
But it's not, It, it comes with its own kind of challenges, right? Number one being if you are a foundational model company, your data is in the petabytes. If you are, um, if you are trying to fine tune your models, you know, obviously you can use, um, P F T and um, lower to kind of, you know, fine tune only a small part, but then you still have to have the whole model in memory.
Guess what? These models are really large. Um, the containers that these would run on, go in the tens of gigabytes. And so, you know, big data, bigger models and everything on Kubernetes just becomes a, you know, uh, heavy, heavy lifting kind of effort. And so, mm-hmm. It, it comes with its own problems. Just yesterday, um, I had to like ssh into the node.
To look at the journal cuttle to see what's going on. Like, Hey, why is this pod not pulling fast enough? Cuz some nodes it is five, like 15 seconds, some node, it's like taking a minute and a half. And so, you know, these kind of, especially when you're talking about like 15 gb uh, container sizes Yeah, things start getting really, really, uh, hard.
And kind of sort of everything then, you know, follows that larger containers can mean bigger startup times. They also mean that it is poten, potentially possible that, you know, pods may not come up because that much disc space is not available. And then you know many, many things that the cost can go up because you're pulling down, especially if you're, every pod that starts is pulling down 15 G of data for every time, like you crash back off.
Just like adding onto cost complexity and everything. What. Is it, can you maybe quickly talk a little bit about what do you think is a, is a good, you know, data scientist experience if they have to use Kubernetes? Like what would be a good, I'm, I'm, I'm guessing data scientists are probably not very, very enthusiastic about wanting to deal with, you know, deployments and demon and, uh, DNS resolution and DNS and whatnot.
I think I can maybe answer it in, in one uh, sentence on, the best experience for data scientists to deal with Kubernetes is not dealing with Kubernetes, so, um, And, and even organizationally, right? If you look at this, if you, uh, and, and Raul and Patrick, feel free to chime in here. I think organizationally what I've seen typically is there's a different deployment team versus a data science team that actually deploys these models and deals with all these complexities.
Uh, in fact, funnily enough, some, some organizations were telling me that in the past, at least with. Traditional machine learning workloads, they would write their models in Python. There would be a team converting that into like running C code and then finally deploy. Like, this just sounds, you know, a hundred steps for me, uh, that are not optimized.
Um, curious to hear your thoughts. Yeah, I, you know, I think obviously traditionally it was used Python right from Jupyter and try to make that work as good as possible. I think what's interesting at generative AI is we're seeing it really stretch. Like we don't really need data scientists as much anymore, right?
It's like these models are so smart that now just regular old engineers. Can pick them up and start building AI apps. Right. So I think there's this like shift to where, you know, I, I think python's still ideal, but I think a lot of times it's gonna be more actually like taking those models and like meeting engineers, they.
And one good point I think about all of this is that, you know, uh, this is almost kind of the other side of it, which is on the one side there is complexity, but the other side of that Kubernetes gives you is, is, is composibility. Like, there are so many different tools and services that we talked about literally in these, what, eight minutes that we've had this conversation about, uh, different libraries, different tools, and the, the amount of innovation happening there.
Deep speed. You know, Laura, Theft. So many of them, um, coming up. It's uh, it's easier to get these up and running. It's easier to containerize this and kind of sort of deal with them in within containers because with the isolation boundaries. Compared to, let's say, saying that here is, here is one GPU instance, four of you go and SSH to that box and run whatever you want.
I'm sure everyone will step on each other's doors saying, I want Python three nine. Someone else wants deep seed version four seven, and then it's just like little bit chaos. So there are benefits of Kubernetes. There is also the complexity of it, but the composability and the extensibility that you get with it to some extent, maybe even isolation.
That can be, that is very, that's very appealing, but it probably comes a little later. Do you think we, we are there yet? Do you think there are teams who actually can benefit from all this, you know, large scale platforms that can be built on Kubernetes? Yeah. AI platforms. Yeah, so, so one quick point that I think it's, um, obviously as switching into nodes kind of makes, makes sense in, in, in ways.
But if you look at, um, the non foundational model, and we've talked about most of the, these foundational models and fine tuning kind of approaches here. Uh, so far, the others, which I think Patrick was alluding to was like, let's say you wanted to spin up a q and a, um, bot that you've created with some Vector DB and some.
Um, uh, store, you know, data going in there answers, maybe users, um, Azure's, uh, open AI bot or anthropics new SOC two compliant. Uh, but like, Creating these, uh, services that can help support these kind of applications, can also also work with Kubernetes. Right. I think that's, that's like the flexibility at the end of the day, Kubernetes is not the silver bullet.
Like, like mm-hmm. We, we, we, you know, everybody here agrees it's, it's not, but you know, there are these challenges that if you were doing this challenge on a regular note that you have set up, The community support is just not there. You try to Google search, you try to ask Chad G PD to help you out. It's not there.
I think Kubernetes is just way more adoption and um, you know, the support that, um, can allow you to debug. And I think that is part of the developer experience as well. And not just having a dev box and aging, but also if you get stuck, how do you unblock yourself? Yeah. Sorry. Yeah, go ahead. Go ahead. I think it's still best in the business of, uh, you know, having multiple services.
And like Rahul just said, you know, you might be running a vector database, you might have a web app, you know, and then you might have your lab and you need those all to coordinate to build your applications. So there's still nothing as good as Kubernetes. Like run those all in one place, orchestrate 'em together and have like one coherent API to that.
Yeah. Go ahead. Go ahead, go ahead. Sorry, Manjot. No, no, go ahead. I was just adding, uh, uh, I think you mentioned these three, four areas as well, right? In the, um, in the previous, uh, uh, question on, um, prompt engineering versus finetuning versus training, and then these service already ended architectures. I think the last bucket I add there is, uh, inferencing, right?
Mm-hmm. Um, out of all these sort of workloads for sure, uh, um, um, Kubernetes right now is far more, um, uh, you know, scale, um, battle tested, I would say for the training and the fine tuning parts versus the inferencing part. But coming down to the inferencing part as well, when you have, and I think, um, at least the applications I seen are still sort of figuring out exactly what that architecture would look like on having an external victory, not just for training, but also for.
Um, actual inferencing, uh, that sort of also fits into the service oriented architecture for which Kubernetes was basically developed. Interesting. So, so having said all of this, I think like, you know, is there, I'm sure there are lots of people who are maybe just starting out or who've always kind of, sort of felt like, yes, we've been able to train models either locally or using some service, but we want to kind of standardize on, on something.
And Kubernetes seems like, uh, you know, an industry standard almost. What's a good place to start? Like what would be your recommendation for where to begin and how would they kind of, you know, how would they go about doing this?
I, I'm almost, I'm, I'm almost hesitant to say this, but you know, a lot of companies have come up with simpler, um, you know, one line kind of commands that, um, you know, data scientists can run to get started if they don't have a, uh, team that can help you set up the platform, right? So you just write your Python code, Python code, or whatever you want for your training, the large language model, and then just have a one line command.
So, They will take care of containerizing that code and deploying it on, on, on their Kubernetes thing. Right? And so they are kind of taking care of the orchestration for you. I think that's a good place to start if you um mm-hmm. If you really wanna get started and you don't have a team supporting you.
But I would almost be hesitant to say that that's the best way in which you'd. Do a repeatable kind of interac. So once you deploy like manor's, good point about inferences, right? Once, once that large language model is an inference, um, then you have this human feedback loop that you need to think about, right?
So, oh, people are uploading or downloading the replies that the agent is giving. Okay, what do we need to iteratively improve on? And now suddenly your data is again leaving your B PC to go into some other cloud. How do you connect all of that? Eventually, um, you're gonna have to have a robust platform, uh, on top of Kubernetes to support all of that.
And maybe it's not a bad idea to, you know, just, um, have some help from, from, from, um, somebody who's, you know, helping deploy this on top of your Kubernetes platform or, or, um, you know, learn it yourself. I think there's enough, uh, resources available in upcoming that, that, that would be super helpful. So, so fair point.
I think what you're saying is there is, there is a layer that can be built on top that to some extent hides some of these complexities that people are talking about, and it can provide that experience that people directly, you know, that you write your code and like whatever, run it with. Some special command or decorators or whatnot, and it eventually goes to the backend and runs it on Kubernetes.
As time goes by, you might want to be interested in what is actually happening behind the scenes, and it'll, that'll be a good introduction to kinda start with something basic and then do this. I, uh, given that we, like Demetrius is gonna tell us that in a few minutes, I also wanna touch up, A little bit of these non-technical aspects, especially Mjo, like your experience.
I'm sure there are company people who have been thinking about, you know, building companies and products that kind of, sort of aid and assist data science and ML applications. Like what do you see on the non-technical side like Kubernetes for LLMs? Does that, does that seem like things that have, you know, that where products can be built that can, you know, be good, viable businesses going forward?
Yeah. So, uh, I, um, actually this is, uh, this is a great question and a great segue from the previous one as well. But there was utter silence when you asked us best practices. I think the main reason for that, utter silence, ity. Was, you know, sweet. There's no real answer right now. I think the space is moving so fast and everything is so recent that, uh, people are still figuring out, okay, what are the, you know, best tech stacks for hosting an l LM application in production?
Uh, what is the best way to use Kubernetes or not? Like what are the alternatives? Right? Uh, so, so every day you see like new things coming up. Like there's covid, the hosted model, there's replicate, which is like completely, like you don't have to care about anything, which Raul touched upon, right? There's so much happening.
Uh, and to answer your question on, uh, are there, you know, businesses possible yes. Uh, I think for sure there is, if there were ever a white space to create like, you know, a cloud offering from scratch, uh, this is an, this is a very interesting white space. Uh, there's such a do of GPUs right now. Uh mm-hmm.
There is also lack of knowledge on what is the best way to host, manage, uh, and serve your applications, right? So there is a real white space right now in terms of. A being the thought leader that, hey, you know what, like you, you care about, what are the big problems like in serving application? Mm-hmm.
Like broad, right? Forget Kubernetes, forget everything. Broad problems are hallucinations. That's one of the biggest core challenges for enterprises. Second big problem is cost, which Patrick also mentioned. Uh, and last can be just reliability and latency. So when someone thinks about creating the next, you know, uh, infrastructure platform, uh, to serve l l M applications, these are the broad three, four problems that they have to tackle.
And there is a good chance that the answer under the hood will be Kubernetes. But the, I think the I idea is to abstract these things away versus, uh, display all the moving parts. Interesting. Well, what do you think about the case? Sorry, go ahead. Go ahead Patrick. Go ahead. I was gonna gonna say LMS will probably be the abstraction on top, right, which we're already kind starting to say we're probably, I'd also say it really depends on the size of the organization, right?
Startups are gonna really wrestle with the cost of Kubernetes and the cost ELs is already really high, and if you add Kubernetes on top of that, it gets even higher. Yeah. So, you know, if you compare that to running something like a CloudFlare worker using OpenAI api, that gets a lot cheaper. Right. So I think you have to kind of weigh like the size of the organization and you know the capital they have available.
Mm-hmm. Interesting. Uh, I'll take one question from the audience here. There is a question about, can you describe the pros and cons of Kubernetes for element training versus the pros and cons for Kuber for, uh, uh, using Kubernetes for element inferencing. So Kubernetes for training versus Kubernetes for LLM inferencing, what are the tradeoffs?
Um, I think Raul, you've done both of these to some extent. Yeah. And, and, and this is, this is a long question, but, uh, long answer, which I'll try to keep short. I think when I'm, IM, uh, advising my clients, um, I, I'm telling them about like I'm, I'm. Uh, optimizing for velocity, um, and, uh, you know, having a platform that is easily like, make, that's helping you deploy models faster.
And I think that is one thing that I think Kubernetes is great on for training. Um, for the inference side, I, I, I defer to majority. She, she had, uh, raised that point. Yeah, no, I think the infant side, right? I, um, uh, we've already discussed some of the challenges present, uh, in managing a model as well as challenges in Kubernetes.
Specific components on how they are designed today versus how they're supposed to work. I think one more, uh, challenge I mentioned is, um, a lot of these, uh, Uh, you know, a lot of the l l M workloads might be using these, you know, specific libraries and divide, uh, uh, drivers that, uh, work with accelerator or some specific hardware component, right?
And the whole point of Kubernetes, uh, and containers is to abstract all of that away. So I think the world is still sort of also, uh, um, struggling right now. Finding that sweet spot of, okay, I need to abstract away hardware, but like, this world does not quite abstract it away a hundred percent. Um, so, uh, inferencing, I think there's a lot of work to be done.
Uh, if someone were to start building an application today, uh, you are better off picking up some of these open source solutions, uh, present that at least abstract some of that complexity away. But I think we still see a lot of development, potentially new products and services being built here. Got it.
And there is also a little bit of this, uh, referencing. At times can, can be, you know, real time, uh, online inferences versus training being kind of bad jobs. Yeah. So it goes back to one of the original earlier questions we talked about that Kubernetes for bad jobs is, I mean, it's of course it's there.
It's useful. Versus Kubernetes for inferencing can be like, you know, server-based processes and whatnot. So there'll be some of that that comes out. Uh, you mentioned about the abstractions, like one of the abstractions that available that's available today is, uh, an open, multiple open source projects out there.
One of them that at least I'm involved in is called Meta Flow, that people check that out. Um, uh, in, in case people are interested in kind of, you know, learning about abstractions that can deal with Kubernetes or hide the complexity of Kubernetes. But, uh, but yeah, I think this was, this was great and you know, We write on cue.
We have, we have someone, we have a person with a Red hat here, pirates Pirate. We have a pirate. It's uh, Demetrius all man. I've rated the ship and I have come to steal the show back. That was absolutely incredible. Thank you all and I will remind everyone. Well, Raul, I may need to make new shirts now. Like Kubernetes is a gateway drug or I guess Kubernetes is the way to stay high.
Uh, that is, you can't really see it cuz of the lighting there. Maybe you might be able to see it. I'll throw up, you know, you know, I, I, I wanted to make a joke about kind of, sort of wearing your heart on your sleeve. Oh. But you know, your, you know, Kubernetes is a gateway drug. Trumps me there. Yeah. It's hard to follow that one man.
That is so true. I know. I did a little bit of a, I did a, a wardrobe change and I busted out the, I hallucinate more than chat G p T shirt that you can get here in case anyone wants to grab a copy of that. We've got it here, folks on the panel, I must say. Awesome. And Raul, I'm gonna give you a shout out right now because you are running the after party in San Francisco.
You got like a bus or what do you got? That's right. So if you're in San Francisco, um, you'd probably know Mission Bay has this, uh, awesome outdoor space called. Uh, spark social. There's sun outside. It's a nice day for us to, you know, mingle. Um, we have a bus, a double decker bus that we'll be meeting up at.
There's 120 people already signed up, so I, I, I think we can get a couple of. Uh, dozen more. So just join us. Uh, spark Social is a big place. This has been an awesome panel. Uh, thank you everybody for your valuable, uh, thoughts. Yes, thank you. Thank you so much everyone. This was a lot of fun, uh, and a lot of interesting conversations over to you, Demetrius.
Yep. And any more questions for these Kubernetes experts? The Kates, as they like to call it. I think that's what the cool kids call it. So I'm gonna. Pick that up. Go ahead and throw it in the chat, throw it into Slack, wherever it may be. I'll see you all later and hopefully thank you. Like bye, uh, see you in a few weeks because most of you, except for Manoj, were in San Francisco.
Ah, yes, Patrick, not, not really.
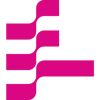