Partnering with Product for Effective, quality Data Ingestion & Training Data // Daniela Santisteban
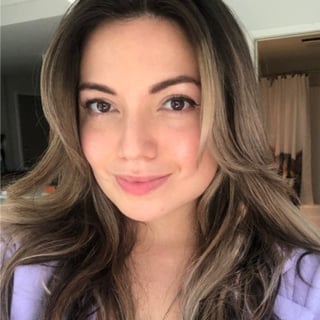
Analytics and data wrangling enthusiast. Starting with Nielsen, Daniela grew her career in analytics across multiple company sizes and technologies, growing love for product analytics and camaraderie and respect for my Data Science counterparts. In Daniela's most recent tenures, she led the user-event instrumentation of a major video streaming app in Hispanic markets (and tuning up my professional Spanish) to most recently creating external-facing data feeds and data products from a scrappy scraping-technology-based (pun intended) start-up focusing on retail pricing analytics.
The product organization at a company can vary vastly, but getting the right PMs on your side can give you certainty on what data can be collected, influencing the architecture to preemptively set up ML models for success, and prove out models' ROI to the business.
Skylar [00:00:02]: Very excited to learn from an expert here. So I'll go ahead and introduce our next speaker. Welcome, Daniella.
Daniela Santisteban [00:00:12]: Hi, everyone. Hi. Happy to be here. Getting used to the tech here.
Skylar [00:00:22]: Yeah.
Daniela Santisteban [00:00:23]: Yes.
Skylar [00:00:24]: We're all learning today.
Daniela Santisteban [00:00:26]: Yeah. Let me see if this works. Can you see my screen?
Skylar [00:00:31]: Perfect.
Daniela Santisteban [00:00:33]: Here we go. Okay, well, let me just get started. Thank you so much for mentioning my name. I'm Daniela. I'm just going to get right into it. Since this is a lightning talk. I'll be talking to you today about, as mentioned, partnering cross functionally, in this case, with non data product managers. And I believe that this partnership can help improve the value and optics of data engineering and make that more visible to the entire organization.
Daniela Santisteban [00:01:02]: So, a tiny bit about me my name is Daniela. I've been in tech for about ten years, seven of those I was a rank and file data analyst, and in more recent years, I have switched onto the product side, the dark side, and I have worked both with internal product management, enabling data products for internal teams, as well as external product management. So dealing with customers and delivering them data products, but always, always, always entrenched, embedded within data platform, data engineering, data analytics and other data teams. I'm New York City based, but as you can see in my picture, I'm always looking for reasons to escape onto the outdoors. But let's get started. Enough about me. I came onto this topic thinking about best data practices, and also about this kind of existential question about data engineering that's been ruminating in circles, proving the return on investment of the data engineering team. Once the lights are on and data pipelines initially are built, how do you continue to justify the headcount, the costs associated and projects to do with data engineering? And this is a question that execs just won't let go of.
Daniela Santisteban [00:02:20]: There's definitely schools of thought that say that this question shouldn't even be asked of data engineering teams, that it's an essential part of keeping the running. But there's a couple ways that you can kind of change the perspective, and one of them that is huge is being able to attach your data engineering talents to high value initiatives. And you're all here at this conference, likely because you're thinking about this already being the house of ML. Training data to create pipelines for ML models is definitely one way to get attention and recognition for being kind of this sticky part of the organization that is really entrenched in essential. My argument here is that while this is great, one flaw here is that we're not touching the end product that much. So there's an opportunity to also start to create partnerships to measure the success of these high value initiatives. And I'm going to focus on product today. But that can also be marketing initiatives or anything else, and it can be ML related, but it doesn't have to be.
Daniela Santisteban [00:03:38]: So why move closer to product? We're all here at an ML for data engineering conference, so we. Let me explain real quick. So when we're not, we're rarely creating ML models and using training data to release something out into the wild. For maybe a data scientist, that's the end user to download into an edge device and experiment with it. Most of revenue generation for ML right now is closer to just the overall software engineering process. You have to build a tool, build a wrapper, build a feature that ML is running behind. You have to make, put that out into the web, onto some marketplace for consumers to actually access it. So it's this end user that's really creating these streams of revenue for the organization.
Daniela Santisteban [00:04:30]: And staying attached to kind of the left side of this equation is super valuable, but it's not as visible as finding ways to attach yourself to the end goal. That's actually bringing in the dollar bills. So enter the data PM. That's usually the bridge that allows data engineers to kind of build their way to touch the product as closely as possible. So I've been this guy before, and if you're in a well resourced data engineering or data organization, you might have one of these people or may have worked with them before, and they have the data chops. They understand data best practices, can gather requirements from business needs, and they do have stakeholders that are less attached to the pipelines and more attached to the business. But the great thing about them is that they have this existential need that's very similar to data engineering. They're trying to prove out and build things that show that these internal needs are super relevant for new and ongoing developments, and essentially proving out that data Eng isn't just a cost center, but they're not that common.
Daniela Santisteban [00:05:46]: Not every organization is equipped to hire a data engineering PM, to hire PM's to embed into data teams. So what if this is you or you're working for a team, a new team that doesn't have this role? Well, you have two options here. The first one is that you can continue to work away from the product. And honestly, this will work okay. You will have, your major stakeholders will be these kind of core data users, data scientists, the analysts that are building dashboards, and you will still have some contact with product management when they need your work, but it'll be more removed and it'll likely be more reactive. So in my experience with this lead to is bad surprises. They come to data engineering because analytics doesn't have the data or can't find something to show the success or the tracking of this new big thing that's been launched out in the wild. And a lot of times if they can't find it is because it doesn't exist and hasn't been planned for or built out.
Daniela Santisteban [00:06:50]: So this can throw sprints into this array brings urgency and chaos into your so my suggestion, if you don't have a data PM to take on this role and manage, you can still partner. Go for option two and partner with these traditional product managers that are building out these products to launch out into the wild and put on this hat or mask of a data PM yourself. And I'm going to teach you how you can be that in about five minutes or less, I think. So why do this? Because you're going to create a symbiotic relationship with this traditional product manager. You're going to prepare them for success by giving them the tools and data that they need to prove out that this is indeed a successful launch. And in return, you're going to be closer to the end product, have more visibility as a team, and become stickier in the organization that they need you to really show how something might be leading to success and money. So I created this datapm hat trick. So the hat trick has three goals, and these are it.
Daniela Santisteban [00:08:00]: The first one is understanding who is your customer, in this case, the traditional product manager. But it can be another internal customer too. The second one being where you can help improve their life and reduce their pain. And lastly, how once you figure out what is the solution in order to do that, bring it to production. And the best part of this short framework is that it's very product oriented, is how many product managers do their work and you can reuse it, as I mentioned, not just with a PM, but other internal stakeholders and revenue generation organizations such as marketing. So first things first, we'll go you. I'll did the homework for you on who is this traditional PM. So you still need to get to know them as a person and understand their particular struggles.
Daniela Santisteban [00:08:49]: But generally their Persona is this person that's much closer to the business and still is able to lead a development team. But a lot of their days are spent with customer research, modeling out, modeling out financials and business cases, and communicating the business needs to their development team. And as such, they're super close to the revenue generation branches of the organization, marketing, sales, partnerships, and honestly, top executives. And another thing about them is that they are pretty much everywhere. They're the OG product manager and product manager role. So if you're in software development, you likely have a product manager. They're just customer facing product managers. The big difference between them and data PM's is that their existential threat is totally different because they're thinking about the end consumer.
Daniela Santisteban [00:09:45]: So they're thinking about not missing release timelines that consumers expect, and also just having a flat out failure where the end consumer does not accept the product that they built out and worked really hard to do. So I created this quick allotment chart of how a product manager might spend their day. I'm not sure if my little window here is blocking some, but this line here just shows that the left hand side is more execution tasks and the right hand side is more planning tasks. So white planning, pink execution. And while I kind of made this up, a lot of these numbers actually are quantifiably correct. On average, product managers spend more than half of their day firefighting urgent things that need to get moved along. That includes unblocking their team or other teams from doing work that's needed to keep on track and meet deadlines. And all these execution tasks take up about 70% of the activities that product managers really spend time in.
Daniela Santisteban [00:10:57]: So that leaves 30% for the actual planning and strategy, which is not ideal. And as you can see here, I have the saddest little line, which is planning for success, measurement of their product. So once it's out in the wild, they need to know if it's working or nothing, how it's performing, and while that's super needed and very important, and they will ask for this 80% plus of product managers are tracking the success of what's out. It's usually in a chaotic way, and it's at the end of the day when something is out. And as I mentioned before, it leads to bad surprises for both the PM and the data engineering team that now has to take on this work last minute. So this is where step two comes in, where data Eng can come in to improve their lives. And that's helped them plan the thing that they don't have time for, data requirements or new pipelines in order to make their measurement plans that they also probably haven't made up. Be ready and be ready for launch or shortly after launch.
Daniela Santisteban [00:12:08]: So how to do this? You? I have two. One big step. That's two part you have a little homework and you have a meeting that you should probably hold. You have to remember top of mind that their existential threat of product managers is missing these timelines and that the product is not successful. So in order to kind of find those timelines, instead of asking them, one quick thing you can do is find the product roadmap and I assure you this thing is out somewhere in the organization. You'll find these delivery timelines. Likely you'll also find a lot more detail. This might be in Jira and product board, but you'll be able to find what are the teams that are involved.
Daniela Santisteban [00:12:49]: Maybe your team is actually on here already at the very beginning of the gantt that are created for road mapping. Find the places where it makes the most sense for data engineering to create measurement plans. What are the highest impact solutions that are coming out and touching end consumers? Where is ML being used that you are team is already a part of? And once you identify some of these where you can insert yourself before launch, talk in faces so it doesn't have to be in person, it can be zoom, but it should be a meeting where you are talking to the product individual contributor, you are talking to their analytics team and you are representing data engineering. And in this meeting you're going to use a discovery tooling that product managers usually use to understand the true needs here and from those create data requirements and a measurement plan. We don't have time in this lightning talk to get into everything that you should be asking or can ask there. But overall you are trying to figure out what does success actually mean for this product, for this product manager, for this feature and what, when, how do they plan to show out and expose this data that will put them in a good place. And as I mentioned, we don't have time for all these amazing questions that you can use. But I do have a free resource at the end that you can access so you can download these guidelines and really pointed questions to bring out how to get to these answers.
Daniela Santisteban [00:14:25]: On the core of the problem with a traditional product manager or another internal customer that might not be as technically adapt as your normal stakeholders are. So lastly comes productizing it. And you're doing this already, right? You have all the data requirements. You are probably making some schema changes, creating a couple new tables, ensuring that the tracking plans are updating, bringing everything to production. But the piece that might be missing is are you becoming part of the at large product development plan and part of that production cycle and the way to do this and really inject yourself and entrench yourself, there is to keep on remembering their Persona, the product manager. They don't have time for planning, they are focusing on execution. So you kind of have to build a plan out for them and explain to them where you really fit in. So you need to sequence and identify the work for your team and also dependencies.
Daniela Santisteban [00:15:30]: Is there an API that, an API team team that needs to expose some new variables and calls for you to gather the right data for to measure a specific metric? Is there work that the PM's development team needs to do in order to unblock you? All this needs to be laid out. You need to map out the work and create some jira tickets probably, or whatever tool you use and kind of also find a delivery timeline for yourself. And it's okay if this timeline falls outside of the actual product launch. Analytics is not mission critical for execution, is just mission critical for planning. So it can come afterwards. But this is kind of the plan that you need to bring to the table when you talk in faces again with this product manager and align on the timing of this work. And this is a place where I definitely recommend the meeting just with the product manager to establish this partnership. Data engineering is bringing better pointed measurement from the beginning, so there's no bets or prices.
Daniela Santisteban [00:16:36]: And the symbiotic part is that the PM is going to provide execution on those dependencies. They're already herding kittens everywhere. They should be able to not just include your work and squeeze it in where needed in their teams, but also talk to other teams and build those dependencies out in their schedules as well. And lastly, they also need to be observing your work as part of their project. So if there is a product board or a Jira epic, something that is tracking the finalization of this product being released out into the wild, you should be one of those checkboxes. This measurement plan that your team is working on and you releasing it into production for analytics to form analyses, that's something that needs to be included in there. So a golden question to help you get there is ask the product manager how they can help you visualize in their roadmap where the building blocks for measurement are really falling in. So take these guidelines as take these as guidelines and not rules.
Daniela Santisteban [00:17:46]: The key part of product development is iteration. Keep asking for feedback of your product counterparts, reinvent the process and also try to make a cadence of this talk about we ask them and invite them to bring new products and ideas to you and how you would start to measure that and always, always continue to ask yourself, you're already entrenched in the beginning of the equation. How can you tie yourself to where the data, or your data to where the money flows in at the end of the equation? As I mentioned, I have some brieftain free resources for you to touch base on and I forgot to put my contact information in, but you can find me on LinkedIn and happy to connect if you're New York based.
Skylar [00:18:30]: Awesome. Thank you so much. Daniella. We're running a little bit over so we don't have time for questions, but thank you so much.
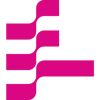