Powering MLOps: The Story of Tecton's Rift
speakers
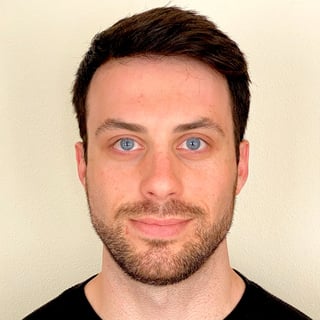
Matt Bleifer is a Group Product Manager and early employee at Tecton. He focuses on core product experiences such as building, testing, and productionizing feature pipelines at scale. Prior to joining Tecton, he was a Product Manager for Machine Learning at both Twitter and Workday, totaling nearly a decade of working on machine learning platforms. Matt has a Bachelor’s Degree in Computer Science from California Polytechnic State University, San Luis Obispo.
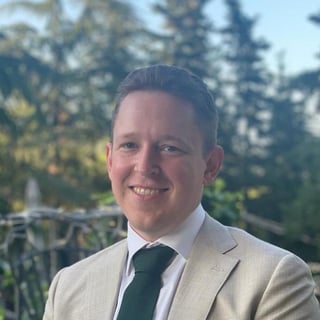
Michael Eastham is the Chief at Tecton. Previously, he was a software engineer at Google, working on Web Search.
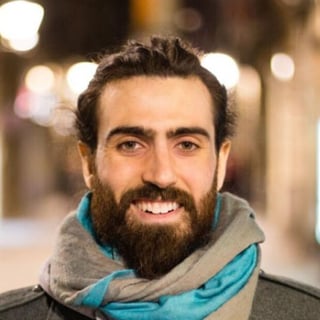
At the moment Demetrios is immersing himself in Machine Learning by interviewing experts from around the world in the weekly MLOps.community meetups. Demetrios is constantly learning and engaging in new activities to get uncomfortable and learn from his mistakes. He tries to bring creativity into every aspect of his life, whether that be analyzing the best paths forward, overcoming obstacles, or building lego houses with his daughter.
SUMMARY
Explore the intricacies of feature platforms and their integration in the data realm. Compare traditional predictive machine learning with the integration of Linguistic Model Systems into software applications. Get a glimpse of Rift, a product enhancing data processing with smooth compatibility with various technologies. Join in on the journey of developing Rift, and making Tecton user-friendly, and enjoy Matt's insights and contributions. Wrap it up with lighthearted talks on future collaborations, music, and a touch of nostalgia.
TRANSCRIPT
Demetrios [00:00:01]: Hold up. Before we get into this next episode, I want to tell you about our virtual conference that's coming up on February 15 and February 22. We did it two Thursdays in a row this year because we wanted to make sure that the maximum amount of people could come for each day since the lineup is just looking absolutely incredible. As you know, we do. Let me name a few of the guests that we've got coming because it is worth talking about. We've got Jason Louie. We've got Shreya Shankar. We've got Dhruv, who is product applied AI at Uber.
Demetrios [00:00:41]: We've got Cameron Rook Wolf, who's got an incredible podcast, and he's director of AI at Rebuy Engine. We've got Lauren Lockridge, who is working at Google, also doing some product stuff. Oh, why is there so many product people here? Funny you should ask that, because we've got a whole AI product owners track along with an engineering track. And then as we like to, we've got some hands on workshops, too. Let me just tell you some of these other names just for a moment, you know, because we've got them coming in. It is really cool. I haven't named any of the keynotes yet either, by the way. Go and check them out on your own if you want.
Demetrios [00:01:21]: Just go to home, dot mlops, dot community and you'll see. But we've got Tunji, who's the lead researcher on the Deepspeed project at Microsoft. We've got Holden, who is the open source engineer at Netflix. We've got Kai, who's leading the AI platform at Uber. You may have heard of it. It's called Michelangelo. Oh, my gosh. We've got Fazan, who's product manager at LinkedIn.
Demetrios [00:01:46]: Jerry Louie, who created good old llama index. Oh, he's coming. We've got Matt Sharp, friend of the pod, Shreya Rajpal, the creator and CEO of guardrails. Oh, my gosh. The list goes on. There's 70 plus people that will be with us at this conference, so I hope to see you there. And now let's get into this podcast.
Matt Bleifer [00:02:13]: Hey, I'm Matt. I'm a product manager at Tekton, and I like my coffee black, made with an aeropress and some fresh hand ground coffee beans.
Mike Eastham [00:02:24]: I'm Mike. I'm the chief architect of Tekton, and I usually make my coffee with a. In a pour over, and I just drink it black.
Demetrios [00:02:37]: Welcome back to the Mlops community podcast. I am your host for the day, Dimitri Ost. And we've got the Tekton team with us here, Mike and Matt. What a conversation. We went through their history where these guys worked before they jumped into Tekton. And both Mike and Matt have been at Tekton since pretty much the inception. They've been around for four or five years, and they've been working on a very challenging problem. For those of you that do not know, Tekton is a feature platform, and it helps you decouple your code from your feature engineering.
Demetrios [00:03:21]: It also does a whole lot more. And what I was excited to get into with these guys today was how they have evolved the product over time, and also how these days, because of technology advancements, they were able to make the product much more lightweight and not have it be dependent on Spark, which has been, as they noted, something that was a little bit of a hang up in the last couple years. So because Spark is such a beast, they noticed that not everyone wanted it and they wanted to see, can we go out there and can we build something for those people that don't necessarily need to be doing all kinds of big data? It goes in line with the ethos that we heard from the folks at DuckdB on. And actually, we got into duckdb a little bit because I think under the hood, Tekton is using a few different duck db tricks and trades. So I hope you enjoy this conversation with Matt and Mike. A huge shout out to Tekton for sponsoring the episode. And if you liked it, you know what to do. Share it with one friend.
Demetrios [00:04:41]: We'll be seeing you on the other side. I gotta call it out. Misim, you put the form that I asked you guys to fill out before this conversation for your job and your bio. It says Michael Eastman is the chief at Tekton.
Mike Eastham [00:05:05]: Oh, I missed a word.
Matt Bleifer [00:05:08]: That's actually his official title.
Mike Eastham [00:05:10]: Yeah.
Matt Bleifer [00:05:11]: It was recently announced that he would just be our chief.
Mike Eastham [00:05:15]: Everybody just calls me chief. No, that was. I accidentally worded there.
Demetrios [00:05:22]: But it makes for such a better title, man. I'm expecting you to change that on LinkedIn. Now.
Mike Eastham [00:05:29]: My official title is Big Dog.
Demetrios [00:05:31]: That's Matt's official title. So we've got big Dog Matt here, the. Oh, man. Product manager. What is your official. So your big dog product, I guess Matt. And then.
Matt Bleifer [00:05:47]: Yeah, that's what it says on my, on my resume. I'm a product manager. I guess my official title is a group product manager. There's a group involved these days as we've expanded our team a bit. So that's been fun and, yeah, been pming at Tekton for almost four years. I got like two months till my four year anniversary. Pretty excited.
Demetrios [00:06:11]: Wow, that is crazy stock. Fully vested then, I guess.
Matt Bleifer [00:06:16]: Yeah, right. Four years.
Demetrios [00:06:21]: That's incredible.
Matt Bleifer [00:06:21]: That's the important metric. So, yeah, four years and almost. I realized I was introducing myself to some someone recently. I've almost hit a decade now of just working on ML platforms, which is wild. Might be even cooler than the four years at Tecton thing. So coming up on that, still in my final year, but it's exciting.
Demetrios [00:06:43]: Hence why you get the name big dog PM.
Matt Bleifer [00:06:47]: Yeah, that's how I got it.
Demetrios [00:06:49]: I know that before you were at Tekton, you did some work at Twitter, right? And this was early days, obviously, four years ago.
Matt Bleifer [00:06:56]: Yeah, similar situation just at Twitter. I came in in 2017 and was hired to be, like, the first ICPM on their ML platform team. And so was in the early days of, like, figuring out kind of what is an ML platform in the first place. I have a crazy story to tell about this, actually. So I joined. I joined in 2017. The team ends up going to an off site in Boulder, Colorado, to figure out what does our ML platform need to look like? How should we orient the teams? We had done some stuff, but we were really putting a stake in the ground there of this is what our ML platform is going to look like. And the day that we get to Boulder, Mike del Balso, our CEO, and Jeremy from Uber released the Meet Michelangelo blog post.
Matt Bleifer [00:07:55]: And in it, they're like, oh, yeah, we built this thing called a feature store. And we're all, we're in like diagram mode on the whiteboard and we're like, looking at it and we're like, wait, that's a great idea. We don't have that in here. And so then we resketch the whole thing. We put a feature store inside of it. We staff a team. It becomes one of my first projects. And so it was kind of crazy then to come full circle from, like, they, their blog post spawned all the work that I did at Twitter.
Matt Bleifer [00:08:23]: And then just a few years later, I came and joined them to work on it over at Tekton. So it was a quite full circle.
Demetrios [00:08:32]: I was expecting you to say. And then Elon showed up and I.
Matt Bleifer [00:08:40]: Was gone long before that. Although I'm sure that was an interesting thing to watch from the inside, or at least I've heard stories from friends and such. But yeah, I left in 2020, so long before the Elon phase.
Demetrios [00:08:57]: Now, are you a user of Twitter? And is there any sentimental value? Do you feel like he's butchered your featured platform? All that work you did?
Matt Bleifer [00:09:08]: I tried to remain kind of neutral or optimistic and see what would happen. I feel like the thing that finally upset me, though, was the rebrand. Like, the Twitter to X was like, this was a great brand. Like, I identified with it. It was like really weird because I was in San Francisco and I was walking down the street and like, you know, there's the famous, like, Twitter sign going down the corner of the building and it's just blank. And I think that one kind of hit hard. I was like, you know, I don't mind you, I don't mind you, like, changing things up, tweaking the product, all that stuff. But, like, the name had to go.
Matt Bleifer [00:09:42]: That one. That one hurt me emotionally.
Demetrios [00:09:47]: Classic, right? So back to the chief. I gotta ask you a few questions here. You've been in the software engineering game. So basically Matt comes to you with all kinds of requirements, or you get, you guys probably liaison quite a bit with what you're building and how you're building it. And before Tekton, you were at Google, right? Correct me if I'm wrong there, Misim.
Mike Eastham [00:10:12]: Yeah, that's right. I was at Google for about seven years before I came here.
Demetrios [00:10:17]: Wow. Wow. Seven years there. It's also incredible working on web search, right?
Mike Eastham [00:10:23]: Yeah. Started out on the indexing team, so was working on a project while we were trying to do kind of like what a web browser does when it's rendering a document, like getting layout and things like that, so that we could extract signals from it. Spent about half my time on that and then moved onto the web server team where I was working on a few different things, but experiments was one of the big ones.
Demetrios [00:10:51]: And now you are at Tekton, you've been there for quite a bit, too.
Mike Eastham [00:10:57]: Youre coming up on five years in a couple months.
Demetrios [00:11:01]: There we go. Hows your role changed over the last five years? What have you been working on? Whats the journey? Been there?
Mike Eastham [00:11:08]: Yeah, well, so my actual title now is chief architect. Its not just chief, so more of a kind of organizational focus, trying to figure out what direction we should evolve the architecture of the product in and trying to harmonize the work between the different engineering teams that we have now. But yeah, when I started, I was the first engineer here, so work was quite different. Just was trying to get something out the door up and running so he could try it out with customers. But, yeah, it's been a pretty fun journey so far.
Demetrios [00:11:47]: So now you're thinking more about the bigger picture, and I imagine things have gotten much more complex. And you've been there since the beginning, so you've seen it. You have a lot of context, and as the complexity grows, you're like, one of the key points where people, you're one of those people that understand how things work, I would imagine.
Mike Eastham [00:12:09]: Yeah, that's the idea.
Matt Bleifer [00:12:11]: You could say that, yeah.
Mike Eastham [00:12:13]: Yeah. But, yeah. Trying to kind of, I feel like we've done a lot of, as we've expanded into different markets and trying to support different customers, we've sort of grown out a lot of functionality in a sometimes kind of ad hoc or haphazard way. And so I've spent a lot of time trying to figure out how we can kind of actively reduce the amount of complexity we have in the product, figure out how to keep supporting those different use cases, but in a way that has a simpler implementation so that we can continue to build cool new stuff without being super bogged down by what we've done before.
Demetrios [00:12:52]: So give everyone that's listening a bit of a refresher on what exactly Tekton does. I know in 2020, especially when the community was first starting, it was hard to go a week without hearing someone talk about a feature store. And so there was a lot of questions about it, because I think of that blog post that you were talking about, Matt, that had come out, and when Michelangelo was being examined by everyone else, they realized, okay, there's some here. When it comes to decoupling features from code and models and all that. So Tekton has also evolved, though. It's not just a feature store, I don't think. Now can you break down what it does for us these days?
Matt Bleifer [00:13:46]: Yeah. So to kind of tell the story a bit here, it's interesting because, like, back in 2020, I think if you were kind of in the, like, niche, mlops type of community, you are aware of what this was. But when I joined, I. So I joke that, like, when I joined, I, you know, did everything except product management and then kind of just moonlighted as a PM on the side. And such is the nature of, like, a super small company. And so I was doing early on, like, a bunch of our demos and sales calls, and there was a fair number of them that would come in and be like, I have no idea what you guys do. I just heard that the Michelangelo guys were starting something, so tell me what it is. And so I'm like, okay, let me tell you about this product that you didnt even know existed in the first place in a category that youve never heard of.
Matt Bleifer [00:14:34]: And so id have to really start from scratch. In the early days of the feature store. Its a very different world at this point. And so really the way that I pitched it was like, hey, you have all of this raw data that youre trying to use to make automated decisions in your business, whether its detecting a fraudulent transaction or making recommendations or, you know, close to home use cases. For me, like ranking someone's Twitter home feed. And in order to get that data to that model, it has to be turned into features, and then it has to be delivered in two contexts, which is at training time, offline, people need to be able to get the values of the features as they were at any given training event. So like, hey, this credit card transaction, we determined it's fraudulent. At the time at which someone was making the transaction, what were their feature values? And that's a tricky problem to get right.
Matt Bleifer [00:15:26]: But then also hard is getting those same feature values to your model at inference time, all at low latency. And what makes this really hard is that it's kind of this place where data science and software engineering suddenly meet, which have traditionally been a bit of different worlds. Like, data science is very scientific, it's very experimental in nature. It's kind of the Wild West. Pip. Install whatever you want and figure it out. That's totally fine. But the world of software engineering had decades of best practices built up with DevOps and CI CD and people thinking about scaling and latency, et cetera.
Matt Bleifer [00:16:03]: And it turned out that when you finally merged the two of those, it didn't go quite as well as everybody had hoped. There was a lot of room there for people to figure out the right technologies that were going to merge data science into the software engineering realm. That's like key part where the feature store comes in. And so we were building this out. But what was weird is at the time, we didn't know what it needed to be. It was almost like the people on the calls were like, we don't totally know what this is going to turn into. We're solving some problems, we're working with customers. But as time went on, we realized that it really had to do with more than just that online offline storage and serving component, which was what it was kind of like, evolving to mean canonically was like, oh, you have an online store, you have an offline store, you serve values at training and inference time.
Matt Bleifer [00:16:55]: But what we found when we talked to a bunch of customers who were just having problems is they also had a ton of problems with actually building and orchestrating and running the feature pipelines themselves. So the streaming infrastructure to calculate these values in near real time, or the orchestration to backfill them, or even we started to find customers that were like, all of my logic needs to be executed at request time and I need to be able to manage that. And so there was a whole category of how do I build features and engineer them? There was the storage and serving component. Then obviously monitoring became a natural progression to this. Okay, now I need to monitor for SKU and data quality. And then as we helped more and more big organizations, there's also this huge collaboration component of like, hey, how do I share features that another team uses? How do I have access controls on this whole system? And so I feel like for a while we kept trying to call it a feature store, and internally we were like, this isn't like checking out anymore, because then other people would say feature store, and they meant something different. We're like, is it a feature store? Is it something else? And so we finally bit the bullet a few years ago and we're like, all right, we're going to put a stake in the ground. It's a feature platform, and the feature store is like the core storage and serving component, which there's several different solutions for.
Matt Bleifer [00:18:13]: But really what we find is the feature platform is the thing that ends up really unlocking a lot of the real time AI at these organizations.
Demetrios [00:18:22]: Yeah. Because the feature store really just makes people think like, oh, that's like a glorified database.
Matt Bleifer [00:18:30]: Yeah, exactly.
Demetrios [00:18:32]: I can just do that in my database. Why do I need a whole new product for that? But really what I'm hearing you say is there's a lot of other hard parts to this, whether it's orchestrating these features in their pipelines, or it's monitoring that the features are actually doing what they're supposed to be doing, or it's serving them at very low latency, which I know was always something that became problematic when you wanted to get to, yeah, real, real time. As somebody, some people call it, you know, like not just that, every 5 seconds real time, or like when you're talking about real time, I guess you guys know best, like, what is real time to you? What does that even mean for you, you know?
Matt Bleifer [00:19:20]: Yeah, and really, like that's, it's funny you said like, there's other hard parts, but it's almost that like the hardest parts actually lie, you know, in the rest of the system, at least in, in what I found, I sometimes say even just internally, like the storage is almost an implementation detail. It's there so that we can cache values effectively and serve them faster. But if I didn't need an intermediate storage layer, then I wouldn't use one. And even an offline case sometimes we actually don't, which is interesting, but really I'm a pm. I think in jobs to be done, it's like you have some feature that you're trying to express or what's the user's average transaction total in the last one year, and you have some requirements, like I need to know that value as of, to your point, like ten milliseconds ago, and I need it served to my model that's running in my production environment. You just want to express, here's semantically what the feature means, here's what my requirements are. And then you want the system to worry about like, oh, okay, there's an orchestrator to figure, to schedule these and to backfill them, and there's a storage layer that's going to help cache these. And you know, there's real time layer to finish computing them, but you don't want to worry about that stuff.
Matt Bleifer [00:20:31]: You don't want to think in terms of the components and the architecture. You're like, I'm just trying to express a thing and when and where I need it and how, and then the system takes care of kind of getting that all done. So I almost think like feature store is just like increasingly kind of like a misnomer. Like it feels very like you said, like it's indexing on a component or the underlying architecture instead of like the problem that is really what people have.
Demetrios [00:20:55]: Yeah, you don't want to worry about the architecture. Let the chief deal with it.
Matt Bleifer [00:20:58]: The chief will figure it out for you. That's what we have him for.
Demetrios [00:21:04]: Oh, classic. And I know you all have been working hard to update things and there was a big announcement. I feel like that came out when I did the apply conference a few months back. But since you've also had newer announcements, can you update me on what is like the newest stuff you've been doing?
Matt Bleifer [00:21:25]: Yeah, totally. Yeah, I'll kick it off here. Mike can chime in. So historically, Tekton has been a fairly spark centric platform and that kind of evolved as a consequence. I think of the types of customers that we worked with, like a lot of that like early adopter category was made up of like these larger organizations that had super real time requirements. A lot of them ended up being like spark shops and were very proficient in Spark. And so we indexed on Spark as like, a key technology to start inside of Tekton. And then we started exploring, like, the data warehouse space and how we would integrate there.
Matt Bleifer [00:22:07]: And, like, quickly, quickly started realizing a couple of things. Like, one, when we went out and talked to a bunch of the market, Spark only really works for maybe about half of them, and it's kind of either just overkill or unfamiliar for the other half. But we also realized that we didn't want to build all these versions of Tekton. That was going to be, there's Tekton on Spark, and then there's going to be Tekton on Snowflake. And which version of Tekton do you want to get? We wanted to design something that was like, okay, what's the one solution that's going to work for all of these organizations? And so something that we built and released recently that we were super excited about is a built in compute engine, Detecton, that we call rift. And it's entirely Python native, so you can run Python and pandas transformations. Whether you're building a batch feature, a streaming feature, a real time feature, it's super performant. We can do cost efficient aggregations over millions of events in a very large time range, which is typically super hard.
Matt Bleifer [00:23:11]: It requires no external infrastructure. You can literally hop into a hex notebook or a deep note notebook, or even a local Jupyter notebook. Pip, install tecton and do all of your feature development there with Python as your only dependency there. And then it plugs in the data warehouses, too. So you can plug into your snowflake. It'll actually push down compute to your warehouse to do initial stages of transformations and then follow that up with additional logic. What's cool is it dramatically simplifies for a lot of customers, like what it takes to get started with a feature platform. It's a lot more in their wheelhouse.
Matt Bleifer [00:23:46]: The iteration speed is like a lot faster for them. It's snappier when you don't need to start a spark job on some attached cluster. If you have a whole company designed around making spark, not a giant pain to manage, then it can be all right. Or if you're a spark shop and you're really deep in spark and we know how to manage it, then great. But if those things aren't true, you don't want to be diving into it unnecessarily. You're like, look, I'm a, you know, I'm a python shop. I'm a data warehouse shop. Like, I just want to pip install something and be off to the races.
Matt Bleifer [00:24:22]: And so that's what we've been working on. That's what our chief here has been helping design out for us. And, yeah, we finally launched it into private preview in November. Racing towards a public preview. Already got a few customers on board and go into production, and we're super excited.
Demetrios [00:24:40]: And there's one thing I want to say before Mesam jumps in, which is, you mentioned before, I feel like it was the understatement of the year, of when data scientists had to do software engineering stuff, or even data engineering stuff back in the day. That is a recipe for disaster almost nine times out of ten. And what you're talking about here, if I'm understanding it correctly, is you're saying, look, we know it can be painful to use Spark if you're not used to using Spark, so we want to just get that out of the way and we want to come to where you are instead of you having to come to where we are.
Matt Bleifer [00:25:24]: Yep, that's exactly right.
Mike Eastham [00:25:26]: And to add to that, I think it wasn't even just a matter of, like, people not having prior experience with Spark. We were also kind of asking them to use Spark for use cases that didn't make a whole lot of sense. So in a lot of cases, some of our customers would be dealing with datasets that were like a few tens of gigabytes, and we spinning up these giant spark clusters in order for them to process those datasets just didn't make a whole lot of sense, and it wasn't worth the extra complexity that spark comes with. So one of the big design principles of, like, this new piece of the product we have is just like, kind of choosing the appropriate tool for the job and not going overboard.
Demetrios [00:26:12]: So I'm very close to Amsterdam. I live right outside of Frankfurt, and Amsterdam is like 4 hours away. And I noticed that there was going to be duckdbcon, and I was looking at who was speaking, and I feel like somebody's name on here on this call popped up. Was it you? What? Is it true? Is it the same person?
Mike Eastham [00:26:32]: It's. That's. Yeah, it's the same person. Even though it says chief architect instead of chief. That's. That's still.
Demetrios [00:26:38]: Yeah, that is you.
Matt Bleifer [00:26:39]: We'll get that fixed.
Demetrios [00:26:41]: And so is that what's going on under the hood? Are you running duckdb? And is that how you're able to do things? Still fast, but not having to be reliant on Spark yeah, that's a big piece of it.
Mike Eastham [00:26:55]: So part of what Tekton provides is sort of this kind of built in library of queries that we combined with the user query in order to prepare their feature data to be materialized for online or offline serving. We've used duckdb for all of that. It's been a really great experience so far. We've really enjoyed using it. It was really quick to get going and we've been impressed with the results that it can get with limited resources. I am going to be giving a talk about [email protected]. i'm looking forward to meeting more users library because it's been really great so far.
Demetrios [00:27:36]: Are you going to be in Amsterdam?
Mike Eastham [00:27:38]: Yeah, coming to Amsterdam for that.
Demetrios [00:27:41]: All right, looks like my choice has been made. I wasn't sure if I was going to go, but if you're going to be there, I guess I have to go now and also be able to say hi in person. That's super cool. So tell me more about what's going on under the hood with rift.
Mike Eastham [00:27:58]: Yeah, so like I mentioned, part of it is duckdb. And then I would say really the most underlying piece of everything is that we sort of shifted everything so that everything is built around aero. And the reason we chose to do it this way is because we had, part of the design goal was that we really wanted to be able to easily integrate with a bunch of different warehouses and different data engineering libraries, pandas or polars or even duckdb if our customers end up wanting to do their feature engineering in SQL. So we've built this thing out in a modular way where we use arrow to exchange data between the different stages. A typical query would look like maybe the customer sets up a data source which is a snowflake table. Then they give us a transformation that they want to run in order to produce feature data from that table, and then they want to serve those features through our online serving infrastructure. What we would do is we would first run the query in Snowflake, potentially applying filters or projections in the snowflake query based on what the users ask us for. If they're only interested in a subset of the data or something like that, that gets streamed back to our job.
Mike Eastham [00:29:22]: And then we pass that as an arrow dataset into DuckDB where we can do aggregations on it depending on what the user's configured. And then that gets passed out of duckdb as another dataset. And then that's where we upload it to our online database. Then later on when they come to query that, then we can serve it out of that online database with reliable low latency. So that's the batch workflow. We also have a separate interface where people have a stream of data that they want to create features from. We have an HTTP interface where they can just send us a row of data at a time and we can apply similar types of transformations to what I was just describing for the batch workflow.
Demetrios [00:30:10]: I know that when I've looked at other engineering blogs, a lot of times you'll see like the Kafka flink kind of pair come up. And I imagine that you guys thought long and hard about the architecture of this and the design. What are some design decisions that you were a little bit skeptical of making? But now that you've done it and you've seen the fruits of the labor, you are like, oh, I am going to do this nine times out of ten for the next, whatever, 100 times that I do this, right?
Mike Eastham [00:30:50]: Yeah, I think one big one is that rather than using a purpose built stream processing engine like Flink, we've gone for this kind of simplified approach where our stream ingestion, it's actually just stateless. In contrast to Flake, where it has to, depending on what types of aggregations you're trying to do, you have to potentially maintain these very large query states, which can lead to a lot of operational issues. Hey, this is Mike Delvalso, co founder and CEO of Tecton.
Matt Bleifer [00:31:25]: Mlops Community is the best way to stay in the loop on the latest.
Mike Eastham [00:31:28]: Mlops news and best practices.
Matt Bleifer [00:31:30]: It's also a great way to connect with experts and get support from an amazingly helpful community subscribed and stay in.
Mike Eastham [00:31:36]: The loop ingestion time. We, all of our processing is totally stateless. So you can filter things out or you can do projections, but no, like aggregations at materialization time. And then what we do is we just actually do the aggregation observing time. And so the benefit of that is that it's very simple. There are a lot fewer things to go wrong. You can't run out of disk space to hold your streaming checkpoints. You don't have to worry about the latency of the stream query committing a checkpoint.
Mike Eastham [00:32:19]: Basically, the critical path from data going into being available at serving time is just dead simple. And I think that served us super well and it's made it not just possible, but actually relatively easy for us to operate this managed service in a way that meets our customers reliability goals, which are pretty high. I mean, we have customers that are using this for things like fraud detection for credit card transactions and things like that, use cases where they want it to be online nearly all of the time. Super important design goal for us. The downside of that, of course, is that if you get super large aggregations, it can start causing performance issues. And so we're currently actually working on a project to do those, what we call compactions. So if you're aggregating over a large time series, we don't do the aggregation at materialization time, but will sort of ingest the data later on, we'll come back and do the aggregation and replace it in the database. And so you can kind of get the best of both worlds that way.
Demetrios [00:33:36]: You talk about how, if you have larger aggregations, what, I guess, what is large in your eyes? Define large and what that looks like. And I don't want to be the guy that says, because I know in every talk that has ever been given at a conference, you always have one person that will raise their hand and be like, so, uh, how does this scale? And I don't want to be that guy right now, but I'm gonna be that guy. What is large and what is not large?
Mike Eastham [00:34:09]: Yeah, so, I mean, I guess the typical, like, engineering answer to this question is always, it depends. And it does depend in this case in terms of. So there's a couple of different issues where you might imagine there would be a couple different dimensions where you might have trouble scaling. One is the amount of data that has to be aggregated for a single feature. I think with our current architecture, without the compaction, we're doing pretty well aggregating a single series of maybe a few tens of thousands of data points, potentially even into the low hundreds of thousands. Past that, it can start to be a bit of an issue. I mean, of course, it depends on the latency budget that that particular use case has. So 100,000 is like plenty for a lot of use cases, but for use cases where people have more than that, we're working on this compaction feature to address that.
Mike Eastham [00:35:08]: And then another dimension that you might be concerned about is like the total size of the dataset that you're trying to transform into feature data for materialization. In the batch use case, it's a little bit early days for us with this. We're still in private preview. We don't have a whole lot of experience, but what we found so far is that DuckDB and arrow do surprisingly well with large datasets. They are much more memory efficient than pandas would be, for instance. So I think a lot of people probably have the experience of trying to load up a five gigabyte data set into pandas, and then they find out it's actually exceeded the 32gb of memory they have on their laptop. Arrow and Duckdb are both more efficient about the memory they use, and then they also have ways of processing larger the memory data sets, where you just go through a chunk at a time. And so the combination of those means that we're able to handle pretty large datasets better than what you might think if you're used mostly napandas.
Matt Bleifer [00:36:21]: We also make it pretty easy for people to vertically scale. So at materialization time, it's choose an EC two instance that you want, you can scale it up really big, it can process a fair amount there. We find it works for, honestly, the majority of use cases. I don't know if you saw d there was a really good blog post from, I want to say he was a product manager at Bigquery. It was called big data is dead. That came out somewhat recently, basically arguing that big data has been the whole wave and that's where everything's going, etcetera. But really, it's a very small percentage of companies that have truly large data, and instead the vast majority of people, even on a large single node instance, can actually process most of what they need to. And there's other clever ways of being able to optimize this, like Mike's been talking about, and we split up backfill ranges intelligently, we do optimizations like he's talking about with compaction to make things simpler along the way.
Matt Bleifer [00:37:22]: And so I think what we find is it actually turns out for the vast majority of use cases, you can get super far scaling up on a single node. And so it's not worth all of the additional complexity that needs to be introduced by large scale distributed processing.
Mike Eastham [00:37:37]: Yeah, I think if you go and look at that blog post, he kind of identifies 1 tb as the maximum size of a data warehouse that you would expect to find in most companies. And if you look, you can actually, from Amazon, you can buy EC two instances that have 24 terabytes of memory in them. Now, the size limit for vertical scaling these days is pretty large compared to what lots of people actually have in their data warehouses.
Demetrios [00:38:08]: Yeah, I think that was the, speaking about DuckDB, that was the creator of Motherduck, like the managed. He went from Bigquery and he started to do motherduck and he was talking about that how because we just had him on recently, like whatever, a month ago to this podcast and he was mentioning how it felt like for a while, if you weren't overengineering things for all this big data, then were you actually an engineer, you know? And so it was almost like you were getting peer pressured into doing more engineering than you needed to because you were expecting to get this influx of data, or you were expecting to be working with gigantic amounts of data. And then when push comes to shove, the data scientists and the people that are actually using and consuming this data, maybe they don't even want that much of the data. They just want like the last week's worth of data because of the freshness constraints that they have.
Mike Eastham [00:39:14]: Yeah. Personally, I'm super happy that that blog post is around and it's getting a lot of traction because I think like, you know, it's kind of one thing to be able to look at the performance of these systems and come to the conclusion yourself like, well, this will be adequate for what our customers need, but you also have to be able to convince people that it will actually be adequate. And so the fact that there's sort of this growing consensus around kind of simpler single node systems led by people like Motherduck, I think has been super helpful for this new product to be successful for us.
Demetrios [00:39:53]: That's so true.
Matt Bleifer [00:39:54]: I think combining that with this kind of stream architecture that Mike talks about has been the biggest factor in us simplifying the product. And it's a similar thing of you could easily over engineer the problem and be like, yeah, we're going to run a flink cluster under the hood and you're going to worry about managing and provisioning streaming infrastructure, et cetera. And it turns out that the majority of what people need is a dozen or so aggregations. And really what they want is they want very limited operational overhead. They want to be able to just use Python and pandas to run transformations, and then they need it to be performant to their latency or scale, which generally means, hey, I have 100 millisecond budget to be able to get features back, but I need to be able to have an aggregation that spans the entire lifetime of an account, maybe indexing much more. Well, just make that as simple as humanly possible. I think has been retrospectively looking at it one of the better design decisions along the way. I think the mixture of that with rift, with simplifying away from the large scale distributed processing when it's not needed, that's been dramatically helping us lower the complexity of the product.
Demetrios [00:41:14]: And Matt, you know how every engineering blog post, when you talk about creating some kind of a product. There's like, here's our fundamental principles or the guiding lights that we were looking at and our constraints and that kind of thing. Did you have principles as you were putting this together and you were going through the product creation phase?
Matt Bleifer [00:41:40]: Yeah, definitely. One was the simplicity in getting started. Like I said, it can't take more than a pip and solve tecton for a data scientist or an ML engineer to start to be productive with this. If suddenly you're like, ah, no, you're going to need to have some sort of spark provider external compute that we plug into and you're going to need to know how to use XYZ in order to do this. That immediately would be kind of a no go.
Demetrios [00:42:07]: It's like, how are your gamble skills?
Matt Bleifer [00:42:10]: Yeah, exactly right. Like it needed to be like, look, if you know python, like we're, we're good to go, you know, you can start using the product. So that was one for sure. Like testing an iteration speed is another thing that we really wanted to optimize for. So kind of in that same vein, like, you should be able to work in whatever environment makes sense to you. And that goes to like the principle Mike was talking about. Like, let's meet people where they are and let them choose the right tool that makes sense for them as opposed to saying like, oh, you know, I know that you work in deep note or hex, but now you're going to need to learn how to use databricks notebooks as your core development environment and you're going to have to use Spark, et cetera, it's like, hey, if you work in hex and you like to use pandas, sick. If you guys are a data warehouse die hard shop and you want to be using Snowflake SQL for all of your future engineering, that's awesome too.
Matt Bleifer [00:43:00]: Like we can make that work. And so like meeting people where they are and working inside of their environment and simplifying that testing and iteration loop, that was also a big element of, I think what we were trying to go for as we built out this solution. And then like I said, kind of in that same vein, we really wanted this to just be like one product. We didn't want to have all these different versions of Tecton built on different underlying compute technologies. And so we needed to find something that was modular and flexible enough that whether you're using spark or pandas or snowflake sequel or whatever else that fits nicely into the platform. And that kind of goes to some of the architectural decisions that Mike was talking about of standardizing on the arrow format, et cetera, and also allowing us to evolve. Because today it might be data warehouses or everyone's in on pandas, but the industry is going to keep evolving. This isn't going to be the last time that customers come to us and want something else baked into their feature platform to be made really simple and to fit with the tools that they're using.
Matt Bleifer [00:44:01]: And so we wanted it to be able to kind of grow with that. And so I think that's where we hit, like, a good, best of all worlds. And we talk about a lot of this in contrast to Spark, but actually, how we designed the system was like, we wanted them to both kind of live harmoniously under one roof. So we still have a lot of customers that, for very good reason, use Spark. Like, we have customers that are generating 90 terabyte training data sets. And what's interesting is that even within an organization, there's teams that use Spark, and then there's teams that don't, and there's teams that use the data warehouse, and then there's teams that don't. And so it's not even just like, oh, some customers will use one or the other, but it's like, in a single organization, you might have different data scientists or ML engineers choosing different technologies, and we want all of those to work together to go into a training data set. If you need to scale up and use Spark, then cool.
Matt Bleifer [00:44:52]: If you don't, then use rift. We designed it all to just live really nicely under one roof at the get go. So it's one product, and under that product, you just reach for the tool that you need, and they all work together, and that's what really lets your data science team collaborate effectively together.
Mike Eastham [00:45:09]: Yeah. To add to that, I would say that, kind of ironically, I think the existence of the spark version of the products really enabled us to make a lot of product decisions to simplify rift, because there was always an option to say, there's a trade off here where we could make the product easier to use, but maybe somewhat limit the maximum scale that it can get to in this circumstance or that circumstance. And by having spark available, it made it a lot easier for us to say, okay, if someone hits that, there's this kind of escape hatch to go to spark. And so we can kind of focus more on the, like, 90 or 95% use cases without worrying about the last 5%.
Demetrios [00:45:53]: I love that, because in my mind, it seems like a lot of times there's the battle between simplicity and flexibility. And it almost is like you were able to get the best of both worlds there and say we're going to go for simplicity, but were also going to make it very flexible. And if you hit that certain scale, then we can bring in the big guns and we can go and get even more firepower behind you.
Matt Bleifer [00:46:21]: Something we talk about internally. Kevin, our CTO and head of product Im and I talk about this a lot, though. I think as a product its really important to make the simple things simple, but the hard things possible. What you're doing is like, you know, working with small to medium sized data sets, then it should not be hard at all for you to get started and to, uh, be able to, you know, engineer your features, scale up, uh, appropriately, et cetera. And but if you're really going to the most extremes where, like I said, we have some of our customers there, like, that's got to be doable and like, there might be incremental complexity that you accept as you do that necessarily. But like, that complexity can't then, like, spoil the easy cases in your product journey. And so I think part of that is like giving that optionality and knowing that, like, you know, you can kind of progress with people through that complexity by allowing them to, like, choose the tool that makes sense and like, voluntarily take on that complexity as their use case requires it.
Demetrios [00:47:25]: So you ready for the part of the show? This is a new segment that I'm gonna call is he serious right now? And or even better, I'm gonna call it is this guy on drugs? And the questions that I am going to ask you now may seem like they come from a drug induced stupor, but I've heard murmurs, and I want to know if you all have been encountering this out in the wild, because it's your day and day out. One of these questions comes from features. And looking at features and seeing that in the data world, features could maybe they're not that far removed from what data analysts use as KPI's. Have you ever seen features and KPI's being shared or used in almost like the same way? What does that look like? Explain that.
Matt Bleifer [00:48:28]: I was expecting an even crazier question, so I'm fully.
Demetrios [00:48:31]: Wait till the next one.
Matt Bleifer [00:48:35]: Yes, definitely. We see some kind of cross pollinating in that world. And so this kind of comes in two different forms. One, sometimes we'll see like, hey, I want my data analysts to be able to create features, or I want the stuff that they've already created to be able to be used as features, then the other way is, hey, I've created all of these features inside of my feature store, but these could be really important business metrics for us. How do I get that back available inside of my analytics systems? Something we've done for the latter that we published recently is actually the ability to take any of your offline feature store data and publish it back into your warehouse. It shows up just as standard. You know, snowflake tables, for example. You can find all of your features there.
Matt Bleifer [00:49:24]: And Tekton takes care of all of the orchestration and publishing of all of that feature data. And so that's one way that we kind of like, get the features back in the analytical world so that they're available in both systems. And then analysts using a feature platform, that one's interesting. Like, one that's important is like, or like, one element that's important is definitely allowing them to engineer with things like Snowflake, SQL, and what they're familiar with. But I think there's also room for feature platforms like lower the barrier of entry and make it even easier to work inside of the platform, et cetera. Some things that we do is make it really easy to take an existing snowflake table and just be like, this is my features, so we can get those really in really easily like that. But I would say it's in the realm of things that we think about and things that we get asked about quite a lot. I do think that there's like a, there's good overlap in that you want features and business metrics available in both systems, but I think that there's also enough divergence that you don't want it to be the exact same system.
Matt Bleifer [00:50:27]: There's a good reason why analytics teams have their tools, and data science and data engineering teams have their tools. They hit on different requirements and different interfaces that those teams are familiar with. I don't think you actually want them to converge to one tool. I just think that you need that data to be really easily shared between them.
Demetrios [00:50:48]: Yeah, it's like one hop away as opposed to being the same thing.
Matt Bleifer [00:50:52]: Yeah.
Demetrios [00:50:54]: So I gotta think of a better name for this. This one is gonna be the part of the show. This is the segment that we call can you get any more hypie? And you know, it was coming. But how are people, how are you seeing people use LLMsdev when it comes to feature stores? And are they creating features with LLMs? I know there is actually one guy, Sumat, I think, that I follow on LinkedIn, who's always talking about LLMs and recommender systems, and so it feels like there's a lot of potential there. But again, you're in the wild, day in, day out. Is anyone actually doing stuff in production with this?
Matt Bleifer [00:51:35]: That's so. Good question. Definitely saw that one coming, because I think if you're in the data space at all, you're getting hounded with this, regardless of what team you're on or organization. So, yeah, for a while we ourselves had to figure out what does the story actually look like? Where do feature platforms fit into this space? Do we use LLMs to be creating features? Maybe people are giving human readable descriptions of features and were then in the same vein as what I talked about before. We worry about all the data engineering under the hood, and you worry about just telling us what your feature is. So we explored that path a bit, and I do think there's actually some.
Demetrios [00:52:18]: Real fruit there that's English to feature basically, instead of, or text to feature.
Matt Bleifer [00:52:24]: Yeah, pretty much. Because we have a giant repository of feature definitions and associated descriptions and things like that, it's not unreasonable that you could go from a human readable description of a feature to all the data engineering pipelines needed to make it happen. But I think the most interesting thing for me of how they fit together is if you zoom out and you look at what these systems are doing in traditional predictive ML, you have data that is getting turned into relevant information that then goes into some model which is making a prediction which is then being used to affects the application in some way. And as we kind of integrate LLMs into software applications, it's kind of fundamentally the same thing. Like you have relevant information, that relevant information needs to be turned into a format that can be digested by an LLM. You take that, you put it into an LLM, it gives you back some results. In most cases, in traditional ML, it's like some prediction here, it might be like some generative text, and then that goes into your application and changes the behavior. And so there's a ton of parallels.
Matt Bleifer [00:53:36]: It's like the LLM systems, and I'm sure you've talked all about the rag design with retrieval augmented generation. So you have some data instead of turning it into features, like you're turning it into embeddings and turn it, instead of loading it into a key value store, you're loading it into some vector database. Instead of writing some rule on some prediction that you get back, you're taking back all of that text and you're inserting it back into your application to show to a user a lot of the core components of this are all the same. At the end of the day, you have data. That's information it needs to go in a model. The results of that model need to go back into your application. I think there's a ton of overlap in the fundamental problems of how do you productionize this, how do you integrate it into an application, how do you get that relevant information? Think feature store is getting features for a model. It's really not that different to get relevant business data to an LLM.
Matt Bleifer [00:54:35]: So that if you say, if you're a travel website and the person's like, hey, I need to figure out where I'm going to go next summer, it's like you want that LLM to have relevant information about that person, what they've been doing, like where they've been before. And that's honestly the same role that feature stores play today. I think they'll play that same role in kind of these rag systems in getting that information there. And then I think also there's another interesting part that I've been looking at where we do a lot of these real time feature pipelines, where at request time someone might need to run some logic to compare an existing transaction amount to a historical one. And I actually think there's some really cool things we can do right in that space with like kind of like managed prompts where like you're storing your prompts as code, but then at retrieval time you're kind of hydrating in relevant information from your feature platform. So you're like, oh, like tell me all this useful information about the user, then take those values, inject them into these prompts, then take that prompt and call out to an LLM, then get that LLM response, parse it, send it back. And so you then just have one API where you're like, I'm putting in some user query here, and the feature platform is taking care of all of that. And where you used to have hard coded features, you might actually have dynamic prompts that you're then feeding into Olms.
Demetrios [00:56:02]: I like that. Yeah, I've definitely seen that. As far as a pattern goes with prompts, you need to give that context. The more context you can give the prompt, the more that you're going to get a valid answer or a useful answer. Right? And so if you can give context around how many times did this person look at this flight in the past 24, 48 hours? Or where else have they traveled to? And they've given these stars on Google, all of that context is going to be really useful. And that is features right there. Like everything that I just said, those are all right.
Matt Bleifer [00:56:39]: So I, and it's really the same thing. Like, you're taking that data, you're putting it in a model, you're taking those results, you're doing something with it. Like, it was almost funny. Like looking back that you're like, oh, that's actually so obvious. If you look at the system at first, you're like, oh, how do these fit together? And you're like, wait, it's like kind of the same thing that we're doing. Like, you know, maybe the individual components are now a little bit different along the way. There's no fundamental difference in taking data, giving it to a model, taking the results and changing the behavior of your application.
Demetrios [00:57:09]: Incredible. Well, fellas, I guess I've got one last question for you before we go. If I'm out there and I'm thinking about what you're going to be doing next, what's on the roadmap and what do you want to sink your teeth into?
Matt Bleifer [00:57:29]: I guess as a pm I can kick this off. So certainly a lot of this LLM stuff that we're talking about here, we're actively making investments into this area, them. So stay tuned with more to come. Um, that's definitely a big area for us. Um, I think continuing to make this rif product successful and lower the barrier to entry, like, I think, you know, we're still in the early days of a feature platform. There's a lot of people out there that are trying to figure out how to infuse their products with real time decisioning, whether it's with an LLM or whether it's with, uh, you know, traditional predictive ML types of applications. And I think we want to make it really easy, make it possible, allow these teams to make their applications way smarter. And Rift is going to be a big part of us doing that.
Matt Bleifer [00:58:18]: And then I think it'll be a lot of fun for us to expand beyond just managing features to managing really those entire real time decisioning pipelines, everything from submitting prompts to LLMs to running multistage ranking for recommendation systems. I think that's where Tecton is going to continue to go in the future. Curious, Mike, if you have any thoughts around this.
Mike Eastham [00:58:41]: Yeah, I mean, definitely. I have a lot of focus on Rift right now. I mean, that's kind of one thing to be developing something within the company. But of course, when you get users onto it, they find all sorts of things. You might never have thought of that break. So focusing a lot on making sure that we make that successful. Another one I'm excited about, and Rift is part of this, but we also have some other things in the works, is making it easier for people to actually try out. So historically, it's, you know, we've had a fairly involved, like, people have to, you know, talk to sales folks in order to get their hands on the product, and we're.
Mike Eastham [00:59:21]: We're focusing on making it a little bit easier for people to kind of test drive things. So I'm excited for that in the next year.
Demetrios [00:59:28]: Yeah, it feels like Rif lends itself nicely to that. Just the pip install tecton is a much different story then. Yeah. So talk to me about your spark cluster.
Mike Eastham [00:59:38]: Yeah, exactly.
Matt Bleifer [00:59:40]: How about you, Demetrios? What are you excited about in the next year?
Demetrios [00:59:43]: Oof, man. What am I excited about? The main things that I think my focus are on right now are virtual conferences and having awesome experiences, as you all know, whether that is Tekton apply that we're going to be doing pretty soon here, or it's the AI in production conference that I'm putting together, or it is any other slew of conferences that I can con people into letting me be a part of that is what I'm excited about. But then I'm thinking about doing an in person conference. It's just a little bit scary because I could totally go bankrupt if I do it wrong. You know, like, that's the big part on it. It's not like a virtual conference where, all right, nobody shows up, no big deal. If nobody shows up, I've got a whole warehouse rented or whatever, and I do want to do it differently. If I do a conference, I don't want to do a conference.
Demetrios [01:00:50]: I want to do, like, a hullabaloo or something like that. I want to do an actual festival type vibe as opposed to a conference type vibe. So people in costumes and all that.
Mike Eastham [01:01:03]: Stuff, but the Coachella of data conferences.
Demetrios [01:01:08]: Yeah, yeah, that's kind of it. It's just, yeah, it's really like, I gotta work up the courage for that one. So we'll see if in Q three or Q four, that actually happens, that materializes or not. And in the meantime, the other thing, like, my main focus is the virtual conference. But the other thing that I've been focusing on that has been an absolute blast because I learn a ton about what people are doing in the wild is the surveys that we do. So we do surveys and then I kind of rack my head against the wall, or I hit my head against the wall for a few months, try and gather data and understand the data and really parse it out, and then I write a report on it. So that's something new that I did last year with the LLMs in production survey and report that came off the back of it, and we're doing it with the evaluation survey right now. So how people are evaluating their systems.
Demetrios [01:02:03]: And so there's a painful process, but it is exciting because I learn, and those are the two things that I've got on my mind.
Matt Bleifer [01:02:14]: That's cool. If you pull the trigger on your conference festival, send me an invite. I'll be there.
Demetrios [01:02:20]: There we go. In costume. Yeah, chief, you got to come with a headdress and all of that. I'm expecting big costume from you that might be seen as it might not be politically correct, it might be creating.
Mike Eastham [01:02:38]: Think about that one a little bit. Yeah.
Demetrios [01:02:41]: Well, yeah, we'll figure out a different costume.
Mike Eastham [01:02:43]: It's.
Matt Bleifer [01:02:44]: Right.
Demetrios [01:02:44]: Well, guys, this has been awesome. I really appreciate you coming on here and getting to chat with you again. And, of course, like, hopefully when we meet in person again, we will get to make some music, because I have fond memories of the last time that we were hanging out in person making music. People probably don't know this that are listening, but Matt is an expert drummer, and you give him a tabletop, he'll make it into a drum. Even so, pairing that with my poor guitar skills is a recipe for disaster. But that doesn't mean we don't do it.
Matt Bleifer [01:03:19]: You are underselling yourself. I think some good jam sessions are for sure in our future.
Demetrios [01:03:26]: Excellent. Well, fellas, I'll talk to you later. This was awesome.
Matt Bleifer [01:03:30]: Good. Catch it up, Dean. Yeah.
Mike Eastham [01:03:31]: Thanks for having us.
Demetrios [01:03:36]: Ops.
Matt Bleifer [01:03:37]: You have to immerse the yourself in the Mlops content.
Demetrios [01:03:41]: The best way to do it is.
Mike Eastham [01:03:43]: To subscribe to the Mlops Community podcast.
Demetrios [01:03:47]: So good luck and keep learning.