The Dollars and Cents Behind the AI VC Boom
speakers
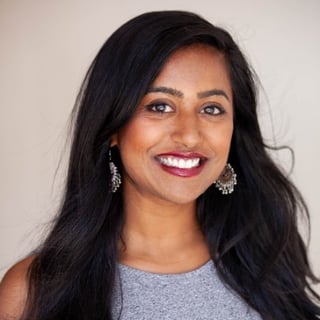
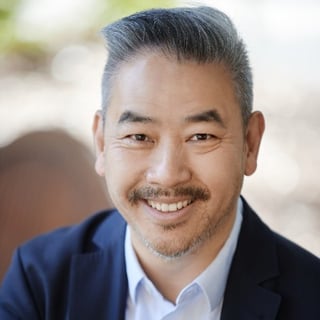
James Cham is a venture capital investor with Bloomberg Beta, an early-stage venture capital firm backed by Bloomberg L.P, focused on investing in the future of work. James invests in companies working on applying machine intelligence to businesses and society. He's currently invested in companies like Weights & Biases, Lambda Labs, Streamlit, and Kolena. James speaks and writes on the implications of AI for companies, including a landscape of machine intelligence companies [(https://t.co/jnBX6Wdr5R]](url) (https://t.co/jnBX6Wdr5R)). In the past, he's been involved in investments with companies like LifeLock, LinkedIn, Twilio, DropCam (now Nest), and Skybox Imaging. James was a software developer and management consultant before he started investing. James lives in Palo Alto. He was previously an investor at Bessemer Venture Partners and Trinity, a management consultant at The Boston Consulting Group, and a software developer. He went to Harvard and MIT.
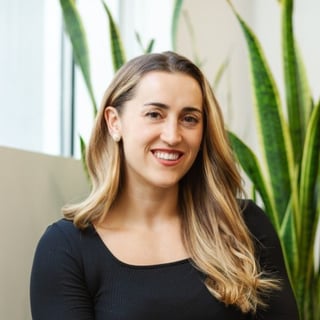
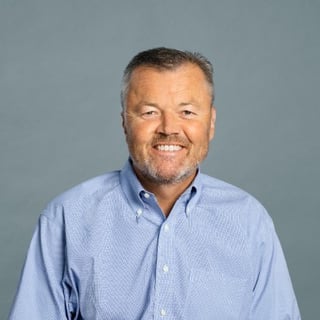
Prior to founding Lobby Capital, Eric was a partner at August Capital and Continental Investors. Eric's transactional experience includes serving as Co-Head of Technology Investment Banking at Merrill Lynch & Co. and as an Executive in Residence at Golden Gate Capital.
SUMMARY
Natasha moderates a panel of leading VCs who have backed the top AI companies and understand the correction within the boom, flight to quality and what happens when OpenAI eats your lunch, how founders should think about giving big tech a spot on their cap tables, & generally how to invest at the speed of innovation right now.
TRANSCRIPT
PANEL 6 Host [00:00:09]: All right, next up, we have the panel discussion with a team of investors, the dollars and cents behind the AI VC boom. Can you please help me? Welcome to the stage. I got my flashcards, so I get the names right. Here we go. Natasha Masqueranhas with the information, James Chan with Bloomberg Beta, Madison Faulkner with Nea and Eric Carlborg with lobby Capital. Give it out. Come on up here.
James Cham [00:00:36]: So is this what it's like to be on a journalist side?
Natasha Mascarenhas [00:00:38]: Welcome. You're on the right side. It's now debate style. Everyone prepare yourselves. Hey, everyone, I'm Natasha Mascarenhas. I'm a venture and startups reporter with the information. Today we're going to talk about a topic that is arguably talked about a little too much these days, the dollars and cents behind the AIVC boom. But of course, we're also going to cater the conversation to quality, due diligence, betting, all of the above.
Natasha Mascarenhas [00:01:06]: I would first love my panelists to introduce themselves.
James Cham [00:01:09]: Sure. My name is James Cham. I'm a seed stage investor at a firm called Bloomberg Beta, where we broadly invest in the future of work. And a lot of what I've done is invested around developer tools, from companies like Twilio to launchdarkly to weights and biases, Chroma, Fixie and Kalina. So I'm lucky to be here. Thanks.
Natasha Mascarenhas [00:01:30]: Yeah, thank you.
Madison Faulkner [00:01:34]: Hey, I'm Madison, nice to meet you. I'm an investor in early stage data AI infrastructure, particularly more dev tools and especially those for data scientists and AI engineers. I am at NEA, which is a multistage fund, but I particularly focus on seed two around series Bjdehehehehehe. I am coming from a data science and machine learning background, so I actually used to work at Facebook AI research and led the AI evaluation platform building there years ago, but have led data science teams at a few other organizations and startups as well, and really excited to talk AI quality today.
Eric Carlborg [00:02:10]: Hey, I'm Eric Carlborg, general partner at Lobby Capital Work Classic Series, a relationship based venture firm. I've been an investor for about 20 years and have the privilege of leading the series A in Colette. And I think, you know, all of us are seeing either AI enabled or AI centric with about every new company that we see. So excited to meet a lot of you and discuss AI quality today.
Natasha Mascarenhas [00:02:42]: Amazing. Well, everyone, welcome to San Francisco. I really want to start with the why. And Madison, maybe I'll start with you. It feels like AI has to say the least. And as this room shows us, has swept us all up by storm, record amounts of venture capital going into the sector. Why do you think this was the moment that AI started having competing conferences and billions of dollars all in the same week?
Madison Faulkner [00:03:07]: Yeah. So I think a lot of us who have been working in machine learning and AI previous to a lot of the generative boom, have asked ourselves this question a lot. And a lot of the new newcomers of the space, I think, you know, similarly, this is one of the big questions. I like to think about it from the transition from machine learning to AI. I think in the last, you know, pre 2020, a lot of the work we were doing was in machine learning. And I define that as algorithms that we are using to estimate questions in the world, estimate answers in the world. And now the main focus of today is we are transitioning to really building mostly AI software and systems. And that's been the big switch.
Madison Faulkner [00:03:49]: And the opportunity for that has been with the capabilities of transformers and generative systems really catching up and enabling so many use cases that previously the accuracy wasn't there, the real time, the latency wasn't there. That switch is really key because the only AI software systems that existed in pre 2020 were really Facebook's ad auction or the search system at Google. So we're setting a precedent today for software systems and end to end solutions in AI that require quality, robustness, testing, deployment, experimentation in a way that we really were never able to build a market in traditional machine learning. So that's a big part of this opportunity is, I think, welcoming many more developers and engineers into building AI systems. And that expertise is desperately needed to make this a big market.
Natasha Mascarenhas [00:04:43]: James, what's your why right now?
James Cham [00:04:45]: I mean, I think it's important to realize a few things. On the one hand, if we were to think about 2021, which you've all tried to forget, there is a moment of desperation where people are really looking for something to work or something to even make sense. All the crypto guys had wasted a bunch of money on their gpu's, so they were terrified and they're looking for some use case and thus they also had it really cheap, so it's really available. Also, all the CEO's are really bored, right? You know, CEO's never pay attention to the IT guy. Cause the it guy's like, oh, we will run boxes and other boxes that run faster than other boxes, so we'll save you money. And so they never cared. And what was really interesting about 2022 and 2023 is for the first time, CEO's of Fortune 500 companies actually cared because their kids were able to cheat on their homework. And they're like, oh, I understand this in the same way that they might have understood mobile.
James Cham [00:05:37]: And so the terrible thing, though, is that it works really well on the one hand, but it's a little bit like the 14 year old who just hewed puberty really works. It's super gawky and it breaks in unexpected ways and they have bad moods. And I think that weird transition is what we're in the middle of right now. And both why it's so exciting and to be honest, a little terrifying, right? Because people are now not just doing experiments with things, they're betting their careers on things. And you're saying, you know what? If I make this bet now, either I will be famous and a VP or SVP of my big company, or I will be at McDonald's, right? And I think that's like, that's why the stakes are so high. And in some ways, that's why AI quality is so important and probably why Eric made this investment.
Eric Carlborg [00:06:26]: There you go.
Natasha Mascarenhas [00:06:29]: Let me follow up with you on that point. Do you think if we're imagining a spectrum of first movers and the real innovators to the people that are chasing hype, to then the correction of failures, and I think someone described to me the idiot phase of the hype cycle, where are we in the spectrum?
James Cham [00:06:49]: I mean, remember, there are multiple things happening at the same time, right? Computer vision. And AV is at a very different position now than, let's say, the average enterprise application or the consumer thing where someone's about to get sued for stealing content, right? And so, like, I think we are like, so it all depends. I'd say that at the enterprise, we are just at the point where some of these projects are starting to work and people are getting promoted, right? Which I think matters a lot for the rest of the industry and economy, because it's going to be those folks that are going to be the reliable customers that are going to drive a lot of value over time. And so we're there for that. I mean, for Av, I don't know who's from out of town, like, hit up a friend who's, like, taking a self driving car, whether it's cruiser, Waymo. I feel like it's so interesting because it's so boring, because it works so well and so reliably. And there's a way in which we're going to be in that world for the rest of the industry in two to ten years.
Natasha Mascarenhas [00:07:50]: I mean, even in 2019, when I first moved to San Francisco, I remember going to Sandhill Road and asking about a firm's AI strategy. And they said that there's no such thing as an AI strategy. And so things have definitely changed. Eric, I want to bring you into the conversation. I saw you nodding along with James's perspective. Please, please add on.
Eric Carlborg [00:08:09]: Yeah, I agree with James. I would also pick up on Madison's point point the transition between machine learning, or maybe not the transition, but the addition of generative AI. For us, the companies that have been quickest and get the most impact out of generative AI are usually those machine learning companies. And I'll give you an example, because they have clean data and well labeled data. We made an investment in a little company and VC's can't help but talk about their own portfolio. So sorry about that. But we made an investment in a little company called Docket, which is real time accounting. So they do real time accounting for small businesses and they have about 1000 companies, and they use machine learning to reconcile revenue and purchase orders on a daily basis.
Eric Carlborg [00:09:00]: So as opposed to just getting your revenue number or your cash balance, you can close your books every day. Well, if you think about that, for a thousand companies, they've been getting the same twelve pieces of information from every invoice. So they have all this data. And then on top of that, you can layer on Genai, which can ask questions of really clean data and make inferences on really clean data. So I think we'll see that happen first or happen fast, and that'll lead a lot of other people to start experimenting in all the ways that James talked about.
Natasha Mascarenhas [00:09:35]: I want to talk a little bit about everyone's investment process right now and how specifically they are doing due diligence for the theme of the conference. The actual quality of the AI in each of these companies. I'll just go down the stage right now. But yeah, how are you? You know, what are the first few questions you're asking companies?
James Cham [00:09:55]: I mean, I think there's, well, there's the quality question, which I would just emphasize to everyone. Nobody really knows what quality means for you, that there is no objective for SQLs ma. That process of defining quality is as detailed and specific to you as defining your business requirements.
Natasha Mascarenhas [00:10:17]: How do you define it?
James Cham [00:10:18]: Well, it depends on the use case. It depends on what people actually want. And I think part of what's exciting about Kalina or a bunch of folks who are thinking about this world is that they're saying quality is not like some platonic ideal quality is what you need to build right now. And there's a way in which like a bunch of the really interesting philosophy of the last ten years around like ideas around alignment, because alignment and quality are actually very close, right? Those sort of idealistic ideas are kind of just helpful frameworks for accurately figuring out the thing that you want to do. And so in that way, on my side, like we are right now, we invest in a bunch of developer tools, right? And so like that's, that's in some ways easier to assess because you just look at the person and you say, would I use their framework? Would I buy their thing or do I believe in them? But the fringe, which is say like the cutting edge of the applications and the use cases, that, to be honest, is slightly kookier, right? That that's not like, oh, is the market big enough? That is much more like, oh, does this person taste little bit of the future? And then we'll find out in ten years whether they were crazy or whether they were brilliant.
Natasha Mascarenhas [00:11:25]: Is your investment appetite in one more than the other right now?
James Cham [00:11:28]: I mean, I keep on saying that I'm going to invest in more applications and I keep on investing in developer.
Natasha Mascarenhas [00:11:33]: Tools because I like, I appreciate the honesty, right.
James Cham [00:11:35]: I mean, like, to be honest, right. But I will say that I am desperately looking for more variation in applications that I am constantly surprised at how little variation there is in either applications, interfaces or especially in business models. And it feels like that's the place to play because the amazing thing is, for better or worse, a bunch of VC's have invested in a bunch of applications and a bunch of infrastructure to make this stuff cheap enough to do. And so why not try the slightly on the edge thing?
Natasha Mascarenhas [00:12:06]: Madison, coming from a technical background, entering VC, you're three months into the gig at NEA. And obviously I've been investing for years prior. What are your first impressions? And also, yeah, same question to you. How are you due diligencing these kinds of companies?
Madison Faulkner [00:12:21]: Yeah. So obviously I am coming from a very similar background as a lot of founders and will often make a lot of the same challenging mistakes as founders, which is really focusing on the AI research and the innovative approach. And I think that is a fundamental piece of finding great innovations in this space. Really deeply understanding the research. I spend a lot of every day reading the latest papers, deeply understanding what's happening in the industry and forming my own theses, so that when I meet a company, I have a very good sense of where this fits in my own thesis, of where I think the world is moving and I think it's very important for me to build a relationship with a founder and feel aligned as to where we think that's going to move in the future. So that's just fundamental. Now, the other side of that, and I think this is where a lot of technical founders often struggle and where I've also had to learn in my own venture journey, is building go to market expertise. And that is such a massive abstract concept, but I really think more technical founders should spend the time to think through.
Madison Faulkner [00:13:23]: Is this top down? Is this bottoms up? Do I really want to run after a developer, go to market motion? How is this going to look in two years, in five years, in ten years? Because that is just fundamentally, I think, where most infrastructure and developer tools fail. So having that really strong, clear thesis is just non negotiable when you come in to look for fundraising and also just to set yourself up for success. So I look for that thesis and it's something I love to work with founders on too, and very important. And then maybe the third piece that I would mention is I have fundamentally believed, and I think many of you also share that belief, that data is the most important piece, piece of building a long term moat and owning a problem. So I really look for an innovation, whether it's on the app side or the infrastructure side, where you're building a better product through more uses and really understanding and owning the user problem and workflow. So having that data flywheel is critical. And a key part of the diligence process is understanding that thesis.
Eric Carlborg [00:14:28]: Yeah, I think I, from our perspective, it's really the same process that we've been doing for years, which is try to talk to customers, try to talk to technical people at the bigger firms and see, you know, we see a lot of things where an entrepreneur is looking to solve a specific problem. And in the diligence process, we're trying to understand, at least on the enterprise side, is the global 2000 really trying to solve that problem? It may be a problem, but is it a problem that anybody cares about? And you kind of have to read between the lines when you ask those questions. And what I mean by that is when you talk to a global 2000 company, they'll say, yeah, I want to solve that problem, but I'm not willing to pay for it. And then you kind of got, you got to push your way through and figure out how much pain and what the ROI is. And a lot of times it's, you know, it's not super clearly defined and that's the inference you're making. And then we talked to a lot of startup technical people, and the response that you often get because entrepreneurs have such a can do attitude is, oh, I could build that with two people in a week and a little bit of money. And again, you kind of got to work your way through that and figure out whether or not there's just some bravado in that or whether or not there's a real problem being solved.
James Cham [00:15:58]: I did say that to vc friend of mine when I was a developer, because I was like, this is so dumb, forms on top of a database. And that company was salesforce.
Eric Carlborg [00:16:09]: Yeah, you got to be, don't trust the developers. That's right. And so, you know, I think we're, we, you know, we're always diligent people because it's always about the people. So we try to, you know, understand their connections and where our connections are and, you know, get, get a read on that person's ability to recruit and to hire, and then we try to assess the size of the problem. But then, Madison, you hit on a giant point is go to market. You know, how do you get there? And, you know, I'll be honest with you, when we invested in Colena, I wouldn't have suspected that you would host a thousand person conferences that's go to market. So well done.
Natasha Mascarenhas [00:16:53]: I really do want to double click on this idea of how early quality is coming up during those initial pitches. I think going back to what you said about the idea of alignment and quality being closer than we think and touching on the same tension, if we look into the stories coming out of OpenAI and the broader AI space, clearly engineers disagree on how to prioritize quality and how to prioritize alignment. And so I am curious how each of that, how that tension is coming up in those initial conversations. Do people have a P Doom score that they're bringing up?
James Cham [00:17:27]: I have a little bit more of. From a software development point of view, the quality alignment question comes up differently than when I was a mediocre software developer. When I would build software, I kind of cared about what was in the database in the sense that I wanted the reports to add up. And I kind of like, I was like, well, you know, like that thing aggregated the right way and maybe it'll be sent off to some accountant at some point, they'll look at it. But I didn't really care, to be honest. I wanted it to work. My tests were like, does this flow? Go to this flow and cover this weird business case? Right. And it was never really about like, oh, is the underlying data even vaguely right? And then are the conclusions driven out of that data interesting or vaguely right along the lines that I care about? And so in that way, I feel like the nature of software development is fundamentally changed because the part of the cycle that the developer now needs to care about is so different and so much worse.
James Cham [00:18:24]: Right? And like, God bless the developer who suddenly has to figure out statistics for the first time, right? And I think that like that shift, that's actually the big shift. And in that way, like that question around sort of quality of the model, that's sort of like an underlying assumption around like sort of my guess when I'm diligenting someone to say, oh, well, will they have access to good data? Are they competent? Are they thinking about the business problem in pragmatic ways? Are they making the right trade offs? And that's how I talk about quality. But that's also because I'm super early, right? I'm investing like it's like three people and maybe they have a logo, right? So it's a slightly different situation than I expect. Madison has all sorts of friends who are doing sophisticated analysis that she might be willing to share.
Madison Faulkner [00:19:08]: These are good and proprietary questions.
Natasha Mascarenhas [00:19:11]: I'm going to start using that.
Madison Faulkner [00:19:13]: But no, we are definitely working on a data internal data project. So it's important for VC's to think about as well. But I would just say I think AI quality is hands down fundamentally the biggest gap in the AI market today. And obviously that's a nebulous concept. That's a lot of different underlying umbrella points. That is robustness, reliability, trust evaluation, red teaming, risk security. There's so many pieces that fall into it. And going back to what I said earlier, this is how we build big AI businesses is building quality around it.
Madison Faulkner [00:19:47]: That is something that I think is still missing from most machine learning and AI systems is the trust and it is because we do not have software, AI, software, AI meeting, software expertise right now. So fundamentally, my thesis in this space I think is very aligned with Colette. It's super important for us to take the lessons of what has worked well in software development 2030 years ago when they were just establishing it and develop unit testing protocols where we can build pipelines in process and actually see alerts when they come up, when this unit case or this eval goes out of lines, set guardrails, be able to evaluate jailbreaks and do this red teaming in process. There's so many great tools that are just starting to come up and really answer these solutions in a best in class way. Like Hayd's labs, I think is a great one. Kolena Distributional, so many other amazing companies and definitely a space. I'm meeting everyone there. I'm very curious about it, and I think there's huge opportunities to establish AI as a software.
Eric Carlborg [00:20:48]: Yeah, I guess I'd chime in again on the problem you're trying to solve, because sometimes what AI does for you is does 20% of the work, but gets 80% of the answer. And that's plenty. Move fast, learn fast. And like you brought up Twilio, right. When we first saw, I mean, it was just so clever and so fast and so viral, but you weren't focused on perfect communication or perfect connection. So there are certain things, you know, fintech things where you're dealing with people's money and that sort of thing. You gotta be 100% right. And there can't be, but then there are other things where, you know, it's just gonna allow, and does allow people to move fast and break things and that's okay.
Eric Carlborg [00:21:37]: I feel like there's tons and tons of focus around hallucination and there should be and testing, but then you have to kind of back up and say, how much am I willing to pay for? And that could be in speed, right. By continuing to test things before you roll it out or in money before I roll something out that I think is sort of good enough. And I think that depends on the, the problem. And that's going to be the real, you know, fun part about this is what goes fastest.
Natasha Mascarenhas [00:22:07]: Yeah, you mentioned clever, fast, viral. I feel like these are all things VC's look for and just to speak maybe for the founders in the room. Oftentimes the type of capital you raise can dictate the type of product you build and team you hire and what you prioritize when you have to pick, you know.
Madison Faulkner [00:22:25]: Yeah.
Natasha Mascarenhas [00:22:25]: What the next move is. What is your advice for AI founders that are feeling the pressure of the venture capital on within their company to kind of move fast and not get eaten up? By OpenAI?
James Cham [00:22:38]: You mean the ones who've already raised money or the ones who are trying to raise money?
Natasha Mascarenhas [00:22:41]: The ones who have already raised money.
James Cham [00:22:43]: I mean, I think there's like a funny dynamic with VC's who seem like, I don't know, Madison and Eric are super smart. They're like really wise. They say lots of good things. At the same time, we don't mind if we're wrong and if like the founder does something that we, like, we're not your manager, right. You know, like, we don't really care whether you listen to what we say or not. We just care if you're right, you know? And so I think, like, that core dynamic is, I think, really hard for first time founders to internalize, right? There's a little bit of this dynamic where you're like, oh, but my VC forcing me to do this. No, the VC's just saying, and like, you know, like, you still get to run the company until the VC firm suit, right. You know, but like, but like, just.
James Cham [00:23:22]: Exactly, but I mean, but that is just, but honestly, that's the only lever they have, right. Other than moral suasion. And I think it's really important right now when you're reading, like, hopefully everyone's subscribing to the information, but, you know, but don't get too distracted when you read about every other competitor or thing that's hot or interesting. Like, there's a little bit of, like, all of that is just noise, right? There's like, you have like one manager. That manager is the market, right? And the market is very harsh. But also, to be honest, the market doesn't really often care what's written in Techcrunch. They care about what's written in information, right. But like, you know, like, and I think there's a dynamic there for these early stage founders to say, you know what? Like, I just need to make sure that I have conviction in the thing I'm working on right now.
James Cham [00:24:06]: And I just like, you know, you could go on Twitter or you could call another potential customer or an existing customer asking them, why are you even paying me money? What's working here? And I feel like that orientation and that psychology for founders, whether it's someone building nameframes or someone building AI, that's, I think, still one of the big challenges and to be honest, one of the secret powers of the best founders.
Natasha Mascarenhas [00:24:33]: Eric, I see you nodding. I also would love to ask you if you're starting to see founders start to push back more and also if they're viewing other types of capital beyond venture when you speak to them.
Eric Carlborg [00:24:45]: I guess I would say that most of the time, just the general nature of a founder is they're willing to move fast and break things and reach out. What I love about this community in general, not just the VC community, but the founder community, is how much sharing there is. We see the questions will get if we were to say something like that, like, hey, you should be using AI more. The response back is, well, show me who's doing it well and we'll connect them to somebody else in the portfolio. So there's really fast information exchange and I think it's sort of bigger companies that are going to get massive, massive benefit from this AI boom, but it'll take them longer to get there. And the founders will be super fast to adopt and pivot and change. So, you know, they're usually, at least, they're usually reaching out to us as opposed to us coming up with an idea like, hey, you should use more AI. You know, they're reaching out to us and pushing us to connect them to places where things are going well.
Eric Carlborg [00:25:52]: You asked about other sources of capital. You know, we went through a big dislocation. I'll call it 1231 2021, sort of a big, big crack in the public markets. But I felt like the private markets completely stayed intact. And, you know, it's sort of been a little bit of an investors environment. And what I mean is we get to see more proof points than maybe we did in, in 2020 and had to invest more in a story. But, you know, when we do see those proof points, we're all piling in and we do, we do have a reputation being a little bit of, a little bit of, a little bit of lemmings and followers into things that are working. I'd say that the lending market with SBB really got dislocated, but that feels like coming back together and seeing a variety of people trying to fill that void and provide capital that maybe isn't capital, permanent capital that you can use to grow your business for a long period of time, but capital that helps you sort of bridge from one equity financing to another.
Eric Carlborg [00:27:01]: It's nice to see that liquidity coming back into the market.
Madison Faulkner [00:27:05]: I'll build on a point that Eric said earlier. I think you should be very thoughtful in how you choose to work with the VC that you choose to work with. I think a lot of people very much just slide into a relationship without thinking about the fact that this is someone who's hopefully going to be along with you for ten plus years. You should feel comfortable pushing back. That should be an expectation is that the two of you are challenging each other and that you feel comfortable pushing back. So, you know, vet and diligence. Your VC's feel really excited that there's alignment in that thesis. I think so many people maybe focus on valuation or the round size above even the relationship, and the relationship is really going to set you up for success long term.
Madison Faulkner [00:27:45]: So treat it as like, as a teammate. That would be my best advice. I love when founders push back.
Natasha Mascarenhas [00:27:51]: I want to end with liquidity. We recently wrote that Cerebras had filed confidentially for an IPO while at the same time cutting some of its share prices around half. And to me it was a really interesting signal on how one of the companies in this race is viewing the public markets. But maybe I can speak with you first, Madison, about the M and a environment and really like coming from a big tech background, how you see big tech kind of looking at the startups in your portfolio.
Madison Faulkner [00:28:24]: Yes. So this is an interesting question for me because like I said, I'm very early stage, not as much on the growth side, but no, the M and a markets are starting to pick up. We're obviously seeing OpenAI start to get pretty active with looking at acquisitions. I think we're at a perfect moment for a lot of the smaller startups that have been built and funded in the especially early, I think Infra dev tools space really start to find interesting homes because they've been able to focus on a niche problem or feature functionality that is really necessary for these big tech companies. I think a great example too is if you think about run AI being acquired by Nvidia Mosaic Databricks, there's a number of great smaller companies there that have blown up in a year plus time with massive valuation. So there's a lot of need in that space and I think it's an exciting part of being an early stage investor is sometimes it's not always a big outcome, but it's great to try and always, you know, think about the long term best strategic outcome for every company. And also something that we think a lot about even at the earliest phases, is partnerships and relationships with strategics. So right now I'm making a seed check in a company and I'm thinking very carefully about the strategics.
Madison Faulkner [00:29:35]: I'm working with the founder to construct that round and we believe that setting up good relationships early with strategics will help that founder be set up for the long term in really building a good horizontal business, but also having those partnerships, the go to market and the possible acquisition targets down the line would.
Natasha Mascarenhas [00:29:55]: Love for either of you to also add on.
Eric Carlborg [00:29:57]: I'll just chime just one thing. I think in the last, certainly this year it feels like the big financial sponsors who have lots and lots of money, and I don't mean the big venture financial sponsors, but the more traditional private equity players are beginning to get in this market and sort of saying, okay, if there isn't, if I don't have to compete with an IPo price, I'm really willing to pay for growth, for growth. And, you know, we recently saw audit board get sold for, you know, rumor has it 1617 times current run rate from a traditional financial sponsor. And I think that'll be a.
James Cham [00:30:37]: I.
Eric Carlborg [00:30:37]: Think we'll see a number of the big private equity players look to acquire some of the best later stage companies in our portfolios and own them privately for a handful of years and take them public when the market opens.
Natasha Mascarenhas [00:30:53]: James, any parting words for founders who are considering exiting this way, maybe early, earlier than their vc's want?
James Cham [00:31:02]: I mean, I think that it is true that the person who has the best nos is going to be the founder because they're the ones stuck all day talking to customers and talking to potential strategics now. So that's on the one hand. On the other hand, I remember Rob Stavis, who was a senior guy at Bessemer once told me, oh, companies are bought, not sold. And I was like, that's amazing. And of course, it's a cliche. Everyone says it, but he said it. I also have to give him credit for saying it to me first. And I was like, oh, that's true.
James Cham [00:31:31]: The reality right now is that at the big company level, there are a number of very big companies that are all fiercely fighting with each other, and they are looking for ways to get an edge. And you look at how well mosaics work for these guys, like, transformative, not just a great team, but it's really worked out. And I think that sort of ability to operationalize, not just to bolt on revenue, but actually to change the trajectory of a business, I feel like the best big company founders are thinking that through right now. And to a certain extent, to be honest, the founders at the smaller companies are just making sure that they're visible and legible to them. Right. And so I think, like, that in some ways is like the, like you'd be glad to have, like, someone poke you and then figure out if you want to get sold or not. Right. You know, so, and then, but one last note on the public markets.
James Cham [00:32:23]: I think, like, there's this, like, illusion that subscription software is all the same. And I think what's really happening is that the public markets are getting sophisticated about, like, what's a good subscription software business and what's a bad one. And then that creates lots of confusion for, like, Normie investors, and that's gonna continue to bifurcate like a lot over the next few years is like normal. Investors understand this market, but they'll get sophisticated and they'll recognize the good ones.
Natasha Mascarenhas [00:32:49]: Well, startups, that is your moment. Thank you everyone, for joining me today and enjoy the rest of the conference.