The Impact of UX Research in the AI Space
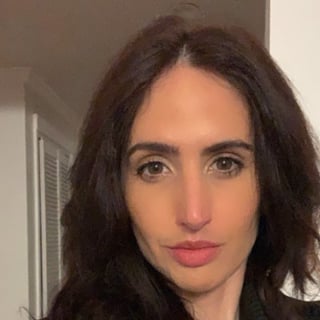
Lauren is a sociologist and writer. She earned her PhD in Sociology at Goethe University Frankfurt and worked as a researcher at the University of Oxford and UC Berkeley. Passionate about homelessness and Al, Lauren joined UCSF and later Meta. Lauren recently led UX research at a global Al chip startup and is currently seeking new opportunities to further her work in UX research and AI.
At Meta, Lauren led UX research for 1) Privacy Preserving ML and 2) PyTorch. Lauren has worked on NLP projects such as Word2Vec analysis of historical HIV/AIDS documents presented at TextXD, UC Berkeley 2019.
Lauren is passionate about understanding technology, and advocating for the people who create and consume Al. Lauren has published over 30 peer reviewed research articles in domains including psychology, medicine, sociology, and more.”
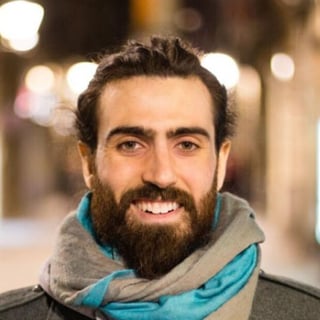
At the moment Demetrios is immersing himself in Machine Learning by interviewing experts from around the world in the weekly MLOps.community meetups. Demetrios is constantly learning and engaging in new activities to get uncomfortable and learn from his mistakes. He tries to bring creativity into every aspect of his life, whether that be analyzing the best paths forward, overcoming obstacles, or building lego houses with his daughter.
In this MLOps Community podcast episode, Demetrios and UX researcher Lauren Kaplan explore how UX research can transform AI and ML projects by aligning insights with business goals and enhancing user and developer experiences. Kaplan emphasizes the importance of stakeholder alignment, proactive communication, and interdisciplinary collaboration, especially in adapting company culture post-pandemic. They discuss UX’s growing relevance in AI, challenges like bias, and the use of AI in research, underscoring the strategic value of UX in driving innovation and user satisfaction in tech.
Lauren Kaplan [00:00:00]: My name is Laura Kaplan. I'm a mixed methods UX Researcher focused on AI.
Demetrios [00:00:07]: Welcome back to the MLOps community podcast. I am your host, Demetrios and today I'm happy as a lark because we confirmed Thomas Wolff, the co founder of Hugging Face, will be in person with me in Amsterdam for the AI Agents in Production virtual conference. Don't let that in person part that I just said confuse you. We're going to be streaming live from a really cool museum in Amsterdam. If you want to come, hit me up. But you don't have to be in Amsterdam to enjoy the fun. That's right, it's all going to be in the Internet, on the Internet, everywhere that you can find a webpage, we will be there. And the specific webpage to register for the AI Agents in Production Conference on November 13th will be in the description below.
Demetrios [00:01:02]: Highly encourage it. We've got Thomas Wolf, co founder of Hugging Face, as one of our keynotes and he is just one of 30 incredible speakers. I don't want to waste your time naming them all off, but they're good. And I'm trying really hard to just talk to you about the conversation I just had with Lauren. This conversation was all about UX research and she has a bit of experience with this because she worked on Pytorch as a UX researcher to know how to just make that framework and that open source project Excel. And I think they did a pretty good job. So we go through what it means to be a UX researcher, why it's important, why we should even be thinking about this, and how if you have a UX researcher at your disposal, should you interface with them? How can you be a good stakeholder in the research? Let's jump into it with Lauren. And as always, if you enjoy this podcast, just share it with one friend.
Demetrios [00:02:19]: That's all I ask. We have to set the scene. I want to know what user experience research is and the TLDR of the space and what you've been up to.
Lauren Kaplan [00:02:38]: All right, so I think starting with the first piece is generally the process of getting insights into how people are interacting with an experience, which that can be a product. We can just like start there and really just in their perspective in terms of like their needs, their pain points, their motivations with that secondly was like, what am I?
Demetrios [00:03:03]: What have you been up to?
Lauren Kaplan [00:03:04]: Well, yeah, I mean I've. I guess there's some background into like what I've worked on. Right. Like in the past worked at Facebook AI which turned into meta working on research for Pytorch and also on privacy preserving machine learning. And then most recently I've been at an AI chip startup and right now sort of exploring other opportunities.
Demetrios [00:03:30]: Nice. So there is something that I think about when it comes to user experience research and that is the typical way that I feel like it gets done, which is kind of, as you mentioned, folks sitting together trying to explore where there's pain points, where there's friction. Does that just mean that like the job is you watching people do their jobs?
Lauren Kaplan [00:03:59]: There's different methods, right? So I think like what you just mentioned is sort of like thinking of like what you call it kind of qualitative, right. Like you can do things called like a contextual inquiry where you're going where people are actually using a product and sitting down with them and observing them. But you can also do things like more quantitatively, right? Like you can collect data, even like analyzing it with some like machine learning tools now. Right? Like doing sentiment analysis or analyzing like behavioral data and looking that up with surveys and that sort of a thing. So there's like a big range of approaches you can use to get insight into what people need and what they're doing.
Demetrios [00:04:43]: Yeah, I can see that. So you have a lot of tools in your tool belt to try to ultimately make the experience as painless as possible. Do you feel like there are certain things that have worked better for you in your past that have given you or helped you gain those insights?
Lauren Kaplan [00:05:10]: I think it's very context specific because you have to start on and that's what I think was maybe the workflow for a researcher really defining what is the problem that you're tackling, like identifying all of your stakeholders and like that's all of the context that goes into it. Like who are you actually including in the research and why? And like what would people even feel comfortable with? Like in the past some of the topics I focused on people were pretty private about, right? So some methods like hey, let me sit and follow you at Google while you're working on some confidential projects. Like no one's going to do that. Right? But will they maybe do. Not saying anyone was necessarily from Google in my research, but maybe they'd feel more comfortable doing an interview right? Where they are clear on like, hey, like these are your rights as a researcher. You don't have to talk about this or that. Like this is going to be anonymized. There's a lot of like privacy concerns for people sometimes or generally.
Lauren Kaplan [00:06:13]: And also like best practices as a researcher that you have to follow that will also kind of shape what you can do.
Demetrios [00:06:22]: The other thing that as you were talking about, hey, I would love to have someone get to the end of an interview and say, wow, that was amazing, I want to do more with you. What are you thinking about as you're trying to prep questions or you're trying to like go deeper? Is it just trying to ask someone to tell you about their pains when using a product or when like where does the product not hold up? Is it feature requests?
Lauren Kaplan [00:06:53]: So I think we're getting kind of like into like what the goals of like what your, the insights are trying to get, right? So before you even get to the point where you're doing, let's say I'm just going to anchor in an interview, you've already ideally like met with all your stakeholders internally. You're very clear on like what the impacts, like everything should be very solution oriented. What is the impact of doing this research? Why are we doing it, who do we want to talk to and why? This is what we're going to focus on, on this work. And then when we sit down, you start to create what's called like an interview guide, right? And ideally in like a successful collaboration, right, with you know, you'll work with engineers, designers, product, depending on the team, the company you're with. And ideally like a really good collaboration between an engineer and a researcher would be like, they're giving you feedback in that doc, right? They're being very engaged, like, hey, we want to know about this. Or you draft out a question like, yeah, we want to get like drilled down into, I don't know, like what percents, you know, what's, what does it mean to have like a good experience with like their inferencing or like they just give like very specific feedback. Because if you don't give specific feedback and you don't engage with a researcher, you're gonna get like really high level results and you're not going to be happy. Well, why do we do all this work? It's just all high level.
Lauren Kaplan [00:08:17]: And so in order to have like really targeted insights, you need a lot of engagement and collaboration. And I think that's what I saw at Meta that was very positive was the engineers are just like super engaged. Like if you left to dock with them, you'd see like hundreds have people like can go like commenting. So that's a really positive sign, like and positive way to work when you're a researcher. If you don't have that, it's going to Be tough.
Demetrios [00:08:48]: And do you think at Meta you saw that because folks could see the outcomes of the research, they would see a product or a experience that they were having get better. And so it's almost like, you know, it's easier for me to pay taxes in Germany because the roads are really smooth. And so I see my taxes at work in a way.
Lauren Kaplan [00:09:14]: I think so. And I think also the engineering teams like had experience working with researchers, so even if they hadn't worked with you, they might have worked with someone else. I also think the culture was just really strong. It was very open, like, no stupid questions, right? Because as from my background being a sociologist, and then remember like one of my first projects there, like really going deep into AI and I was a little intimidated, right? But I like, I had to like dive in and like learn really more deeply about the topic. And something that was really positive was like, I remember like drafting out an analysis and like sitting down with a products and engineering lead and like, I'd have like a couple questions and they'd be like, yeah, that's a total reasonable question because that's an internal tool you would have never even known about. Right? Like, hey, you're asking good questions. And I think having that culture of like encouraging people to learn is really important as well, because especially now that AI has grown so much, I feel like more and more researchers are going to have to onboard onto AI and so not everyone like has that experience yet. So that was of a ramble.
Lauren Kaplan [00:10:30]: But.
Demetrios [00:10:32]: And one thing that you told me was that UX or user experience can be a much broader thing than just like product. And what exactly does that mean?
Lauren Kaplan [00:10:46]: Yeah, so the reason I started like this like working definition with product is because I feel like it's the more traditional and more concrete thing that people think of. But in reality I think you're working much more broad than that. Like, for example, you might be as a researcher working on understanding people's experiences like, of like, for example with AI, like how do we even communicate about AI to consumers? Or how do we meet the needs of like, what's the developer community and what do they need? Or like what are the ways that like an AI framework can be competitive with other AI frameworks? And how can we, what are people really working on when it comes to different model architectures? Or what language support do we need? Like, it becomes like very broad in that sense. And then I also feel like researchers will cover not only product research, but also things like market research. So like, you might not necessarily have someone who's just like a dedicated market researcher because maybe your, your marketing team is like, you know, tied up with other things. So, like, in my work, I've had, I've done work that it's impacting on both the engineering side, but also for marketing as well. Right. So there's like a pretty broad set of stakeholders that you need to make happy.
Lauren Kaplan [00:12:06]: I think that there might be, you know, some folks that are more focused on like the design component, but my work has been a bit more broad than that.
Demetrios [00:12:18]: And when you are doing this market research, are you pulling from sources that you yourself did not create? Like, are you just trying to get information from anywhere? So going back to the interview or surveys, that is what I would. You probably have a technical term for it, but I would consider that's like something that you've created, data that you created while doing the research. But then you can go out and you can look into communities like the mlops community and kind of see what's the sentiment around this. And so that's not necessarily data that you've created, but you're still researching it. And you're almost like letting your tentacles go out into the world and then bring back things that are of relevance to this research.
Lauren Kaplan [00:13:07]: Yeah, it's like primary. I think the right term would be like primary and secondary research. So primary is like minor collecting your own data. And then secondary, you're kind of leveraging existing data sets in a way. So I guess you could say the discourse on like the MLOps community, like channels could be an existing data source, which I guarantee you, many researchers are super interested in your community. Right. I think that either way you need to be systematic. And this is the thing, is that you have to always anchor again and like, what is the impact? Why are we doing this? You have a plan.
Lauren Kaplan [00:13:43]: Like your workflow would be, you have your like, research plan. You're working through that with your stakeholders. And if there's going to be a marketing component, then your marketing stakeholders are there and they give their voice and their opinion of what they want. And then also the engineering team is going to be there giving their voice and opinion of what they need. And then you'll have to come to an alignment from the beginning before you even start, because that's going to shape the type of data you're trying to get and it'll go deeper into also how you're going to design things, if that makes sense.
Demetrios [00:14:15]: Yeah. And in that plan are you mapping out different hypotheses?
Lauren Kaplan [00:14:20]: You can do that or you can like, generally, like every plan should have, like, their questions or research questions. Right. And so there's a difference between your research questions, which are like the broader goals of what you want to learn, and then, like the questions you actually, let's say, ask people in a survey or an interview. Those, like, separate. I notice people kind of mix those together.
Demetrios [00:14:41]: Yeah. And so this is free going out there and starting the research. Then afterwards, once you've gathered all this data and you are working through it. One thing that I found, especially when we did just the surveys within the mlops community, which I would say is very, very adolescent compared to the stuff that you've done, I had the hardest time synthesizing the data and going through and drawing conclusions. And so I don't know if you have advice on how to go about. Okay, I've gone through and I've done this. I have this plan, and here's all the different stakeholders and what they're looking for and their needs. And I've gathered all this data and now how do I connect all those dots?
Lauren Kaplan [00:15:38]: That's a really important skill for a researcher to have. I think that's something that helps, is you could have something like an analysis plan from so that, like, when you're actually going through the data, you at the outset sort of know like, how you're going to be using it. So I'm trying to think of how to describe it. Like, for example, let's say you're like, don't have a lot of budget and you're like using even a Google form, right? Like, basic questions, regardless of what you're using, let's say you're using that and you're. You can only ask a few questions, right? Because you want to have people spending 20 minutes answering questions. Every single thing you ask needs to be strategic and mapped to what you're looking for so that when you have your data collected, you have a plan. Let's say you're looking at mlops community, like, hey, I want to know people's backgrounds in terms of what roles they are. Are they looking for work? What's their biggest challenges in finding that? How do they feel about the content of my podcast? You would plan all of that in advance so that once you get the data, you already have a plan of how you're going to analyze that data and the patterns that you're generally looking for.
Lauren Kaplan [00:16:52]: And sometimes the data might surprise you and you'll get something you weren't anticipating, but at least you're going in in a systematic way. And I think also the types of questions, the way you frame them, you can have structured, let's say you're looking for, hey, I want to get a sense of the overall, I don't know, satisfaction with my community. Right. You can ask a question that you like, you know, calculate a mean or something like that, but then you can also design a question that's more open ended and you could either like manually like open code that to see what's going on, but you could also like couple it with like a pre trained model to analyze it and see how it, how it works. Yeah, I've done that as well.
Demetrios [00:17:33]: I'm always paranoid that the people that fill out these surveys, they're the ones, if they're taking the time to fill it out, they're going to give me more or less good reviews on it of how they feel about the community because they, they spend the time to do it. And so it's like how can I get those folks that aren't taking the time and the diverse opinions, right? Like how do you cover the robustness of the data that you're collecting?
Lauren Kaplan [00:18:02]: Yeah, like that's definitely a good point because you're kind of getting into like bias, right? There's like a social desirability bias there where people are going to be more engaged saying more positive things. I mean some ways you can do that is try to incentivize people by like giving them, you know, gift cards or something that maybe someone who maybe even they're not as engaged, like hey cool, I can get a $25 gift card. I guess I'll do it. I, you know, could help kind of get more people to respond but it can be challenging. And like even now there was a study that came out, it's like it's not peer reviewed yet I don't think. But they actually like analyzed one of these commercial platforms where people are doing research and like 35% of the responses were they found that people were actually using AI to help them just fill out the surveys to get their like mind. So like that. Yeah, so that's like a whole nother thing because that's going to affect methods because like I think something that researchers have in common or like UX researchers have in common with like AI researchers.
Lauren Kaplan [00:19:08]: And also like that kind of community is like we have to think about things like missing data, like is there a bias to that and like accounting for all these technical components and so for having people use AI to write their questions, use AI to respond to the questions, like how do you handle that data? And I don't think there an answer right now and there kind of needs to be an answer. We need to know how to like data.
Demetrios [00:19:34]: So let's go over some scenarios and like why, why we should care about this. Right. And maybe you have a few examples of things that we could look at from the ML and AI lens.
Lauren Kaplan [00:19:50]: Okay. Um, I think like one example would be if you are like one obvious one for like an ML engineer is like if you are deploying models and you have a like a user researcher who's like focused on understanding like developer tools, like that person is going to be able to like bring a voice to the pain points you've been having and you can point to that and say yes, see we do need support for this to make this easier for people. So like I think that's one very clear place where it can be a strong partnership and benefit to people.
Demetrios [00:20:29]: And that's always fascinating because the what I'm hearing you say, especially in that pre data gathering phase when you have to have your plan in place, is you really want to know what the impact is going to be. Have you been able to measure impact in like monetary ways? Is that the most impactful way of putting it for someone to say look, if we do X, Y, Z, it's going to save us or make us millions and millions of dollars or billions. Right. And. Or it's going to. Are you doing it more in ways that are a few steps down from money that you can kind of extrapolate out? Like hey, if we can save time on the deployment process or have our ML engineers dealing with deployment processes less, that is going to save money in the form of their salary and also in the iteration speed that we can get models out, et cetera.
Lauren Kaplan [00:21:38]: Yeah, I mean I think that organizations are generally driven by efficiency. So I do think that the efficiency argument definitely is useful for organizations. Of course. Yeah. Like the monetary component can be there and is definitely convincing. If you have like a billion dollar problem that you can show like that you've made like a 4% difference on that is pretty big for companies as well. Yeah, I mean I think a lot of like the work that I have done is like really focusing on like actually understanding like the ML engineering workflow. So and I think a big part of the reason of that is I'm trying to be mindful of like what I can say, but it's like it's really, really important.
Lauren Kaplan [00:22:28]: I hope. Like, like community members, like no, like if you're not having Like a good experience. People aren't going to use like your products and they're going to switch over to something else. Yeah. And so the, you have to find as a researcher the synergy between what's good for a company and what's good for the people who are using their products. And you have to advocate for that and find that balance.
Demetrios [00:22:52]: Okay. So you're, you're gathering this information. Ideally you're tying it to a monetary number, but efficiency is also a great number that is going to get you far and workplaces tend to understand that gain and that trade off. Are there other. I'm not, I'm not going to say buzzwords, but just like words or things that you could anchor on that is going to help folks understand the value of. If we do this research and we are able to create an impact in X way, whatever it is, efficiency gain or monetary gain or blank, then it's worth, worth it for us to do that research.
Lauren Kaplan [00:23:42]: Yeah, I mean, I think that, I mean it's funny because this is actually like a big topic among researchers is like how do you measure impacts, how do you track effective research? Yeah, I think that another component could be more anchoring and just, I don't know if I'm answering your question, but things that show that you have a very intuitive product that people are enjoying using and understanding what are the strengths of what you're offering and where are the gaps so that you can maintain like being competitive, that's very valuable. And I think in AI people know like if you're in a very AI driven organization how valuable that is. I don't know if that's getting into tracking the impact, but I think that might just be more into the value of understanding people's needs. I think also just like companies really struggle, I think to understand who their audience even is. So I think if you're able to get insight into people at a really like a deeper level, it's super valuable for teams. Right. To know like who is, who are, who are users. Like a lot of companies actually don't know that or who are customers.
Lauren Kaplan [00:25:08]: They're trying to struggle and figure that out. As AI is changing, which it changes very quickly. And so you have to cover like, if you're focused on like a products like for example Pytorch, you have to be focused on like the entire, for me it felt like like all, like all domains. Are you doing video, are you doing speech, what languages are you using? Like it's super broad and so you have to be able to like really prioritize and like go both broad and deep, to I think really give a signal that's like accurate and useful for people. That's gonna be good for like potentially even like a couple years. Like you'll be good, you'll know who your audience is for a while. But now things are moving even faster so you might need to do it more often.
Demetrios [00:25:52]: But how did you prioritize?
Lauren Kaplan [00:25:57]: I think by having really strong partners is super useful to help me to prioritize, right? Like what is important to them and why. And then taking that as a whole and putting that together, it's almost like you're doing internal synthesis or analysis of your stakeholders in a way to be able to figure out what you really need. And it can be really hard. Actually you ask good questions because, well, of course you ask a question, you're good, have a good podcast. But I think that that's something that people really struggle with too is like, especially when you're a researcher that comes I think from like a back, like my background, like having a PhD, like you're used to doing these like really broad, deep, comprehensive studies. But in industry you have to move a lot faster. You're going to have to make trade offs, you're going to have to streamline it so you can't cover as much as you want and you might walk away feeling, even if everyone thinks you did an awesome job, feeling like, eh, I guess it was good enough. So it is a struggle that researchers have.
Lauren Kaplan [00:27:02]: Like sometimes you just have to like cut certain things out. And I like don't like to say like ruthlessly prioritize but like sometimes you have to do that, right? And some ways you do that, it's like maybe you pilot what you're doing and you realize like, you know what, like this just doesn't, or you learn, sometimes you don't learn until you're done and you share insights and you're like, yeah, I thought that people would really care about X topic, but actually, yeah, no, that was a waste of time. Next time, lesson learned.
Demetrios [00:27:32]: It feels like potentially some of that could be combated by having almost like a three phase rollout or hey, we're going to go out there and phase one is we're going to try and validate this assumption and then when we have a little bit of data, we're going to go into phase two which is playing or building off of that assumption. Do you tend to set up projects in that way at all or not?
Lauren Kaplan [00:28:02]: Yes, actually a lot of my work has Been like multi phased work. Right. So you say, hey, you know, first phase, we're going to do like a pilot based on like whatever your situation. Let's say you start off with like a survey. Right. Okay. And then after we get that, we're going to go and dive deeper into, I don't know, let's say like ML, like model monitoring, let's say.
Demetrios [00:28:22]: Yeah.
Lauren Kaplan [00:28:22]: And we'll do a deep dive with that. But first we want to get a sense of like what models people are using or like getting more context into who they are are. And so you sort of plan things out that way and that helps you to cover more ground as well. And it can also help for you to see what's working versus not like, hey, yeah, we asked some of these things in the first phase, but what actually we can refine those questions even more. So you're actually spot on with that.
Demetrios [00:28:51]: Yeah. Because you don't want to spend six months only to realize, oh, we're not asking the right questions. And now we recognize that, which probably still happens even with these phases, that you gain this insight. I really like how you say that, is that you gain an insight after everything's all done. But that insight is probably so valuable, even if it is the insight that you were completely wrong.
Lauren Kaplan [00:29:22]: Yeah. And I think that you don't really even have that amount of time realistically. I think in the past you might be doing more foundational research, which is work that you do when there's not really any research in your problem space and there's just a really broad scope. So with those type of projects in the past, I feel like researchers were given resources to do those and they'd maybe have a quarter, three months to get them done, and that would be considered a long time frame. Now I feel like researchers are doing things, so maybe now I'm getting to a different topic. But then there's also like more iterative research where you have like some understanding, but you're iterating on like a certain experience. Right. So you might be doing like, have a couple weeks.
Lauren Kaplan [00:30:08]: And then I see it going down to like, I've had things where it's like, can I have it in a couple days? And so I actually think that kind of going on a tangent, but like, I think AI moves really quickly. I think everyone's workflows have been like, organizations are just really making things very efficient. And so I think that the efficiency pressures that engineering teams have been facing have also like trickled into other people's workflows. Where we're all just working super, super quickly and have less time to do our work. And so it's gonna. You have to be able to learn and iterate very, very quickly in your work. And that was always important, but I think it's like even more important now. And that's why I think the collaboration piece between different people and different functions is so important.
Lauren Kaplan [00:30:55]: Because without that, it can take longer to even figure out you're not in going in the right direction.
Demetrios [00:31:02]: God. But the value prop there is so nice when you talk about, hey, can we have some kind of a question and get some early signals in a few days that is really powerful to be able to go back and say there's worth us spending time on this problem or no, we weren't able to do it. So we can double down and spend more time, like spend another week on it trying to see if there are signals or we can just leave it and have it be something that we tried and then we'll table it for now. There is something else that I think is worth talking about and that's like you've been working at gigantic companies and then startups. And the thing that I have been wondering as we're having this conversation that's been going on in my head is I see the value of having a researcher. I would love to have a researcher that I could have on the team because I have so many questions and it would be great to be able to say like, can we go and find signal? Is there anything there? Or am I just hallucinating this? Right. But it feels like the user experience researchers that I know are all at big companies. Have you seen that trend where it is more of a big company type of role?
Lauren Kaplan [00:32:28]: It's hard because actually like speaking of data, like, I just feel like I see like these like anecdotal data of like what's going on in terms of the user experience, like research, like what's the market and what's going on? Because people like struggling to really land roles right now and like they want to kind of know like what's going on. So it's kind of hard to like say concrete things.
Demetrios [00:32:56]: Do you feel like there is a. When it comes to this user research, it is really interesting to look at this idea of your researching a product versus you are researching a framework per se. When we're in the developer sphere, right? There's like the end product that a user is using that uses AI maybe or there is some developer tool be that a framework that a developer is Using and do those two worlds differ that much or where do they have their overlap? Like, what does that Venn diagram look like?
Lauren Kaplan [00:33:45]: I mean, I could start with, like, the framework piece. I think I kind of touched on it before, where it's like, you have to cover a lot of ground in terms of what's going on in AI and understanding people's workflows and really deeply understanding what they need. I think, like, the more like B2C, like, let's like, build something on top of chat GPT and then we'll have, I don't know, like a recruiting tool, a tool for recruiters to automate their, like, thing. Like that might be a bit different where, like, you might be doing, like, shorter, like, design sprints and to have some iteration of the product and maybe your scope might be a little bit more narrow and, like, focused in on, like, a very specific niche. Niche area, which can be useful in a way because it could help you to prioritize. But then there can also be a lot of challenges in terms of, like, alignment. Like, I was talking to a company, an enterprise company, and they were working to, like, integrate AI right into their products. And, like, it was, like, really surprised to hear.
Lauren Kaplan [00:34:51]: It's like, kind of sad to hear. It's like, yeah, you know, we have. Sometimes we do research here and like, the product's already launched and nothing gets integrated. And actually we're using this AI model. And when people ask a question to this model, it just says, I don't know. It's like, okay. And like, you know, so I. I'm kind of going in circles in a way, I feel.
Lauren Kaplan [00:35:13]: But I feel like the challenge, what I was trying to get to is like, this alignment. Because I was like, oh, yeah, well, you know, are you talking to engineering? They're like, no, we don't talk to engineering. Like, okay, so you're creating a product that's built with AI, but no one's talking to engineering. It sounds like an issue. Right? Big issue. So I feel like, I don't know, it also will be very organizational dependent and like, how advanced, like, people are in terms of, like, both research and AI and just, like, their processes at the company. And like, I think a lot of companies are actually really struggling unless they're in the lead of, like, how do we integrate AI into our products? Because they feel like they need to do that to stay competitive, but they don't actually know enough about.
Demetrios [00:36:02]: You don't even have to finish that sentence. I know exactly what you mean. That is. And that's how I've been feeling. And funny enough, I wrote this whole talk because I was going to a conference last week and I thought I had a talk. Turns out I didn't have a talk, I had a fireside chat. So I didn't need to spend the six hours on the plane to the conference frantically trying to write this talk and being like, is this good enough? I don't know, there needs to be more substance here. And the talk was all about how in my eyes, the best strategy right now for companies that are not on the full cutting edge, like not your Google meta type companies, but companies that you were just mentioning that feel pressured to implement AI in any way, shape or form, but they don't have the understanding or maybe they don't have the culture and they think they can just skip over all of the data foundations.
Demetrios [00:37:03]: In my eyes, the best strategy for them is to play the patience game because in one or two years it's going to be a lot easier and it's going to be a lot more clear for what use cases are really effective and what ways you can leverage AI. So that's like from a high level, but then from a, from the engineering level, all of these frameworks we'll see will kind of shake out and you'll have one or two or three that are very used and there's a lot of support for. And the community has kind of rallied around. So that's going to be easier for the engineers because right now you have this sprawl that's happening and so maybe you choose this one and then in a year or two it turns out that company got bought by another company and they got discontinued. And so you have to do a whole lift and shift or whatever. And so those were a few of my, my arguments we could say as to why it might be better just to, I don't want to use the word do a fast follow, but really be patient and almost like a cat that's waiting for that mouse, like pounce when the time is right, but don't just pounce because you can.
Lauren Kaplan [00:38:28]: Yeah, I mean, I feel like they're doing like the latter and like tying it back, I think to research, like that's why research is valuable. Right. Like companies like tech leading companies have already like done research to know the direction that these things are going to go to a certain extent. And I can't say too much about that. But I mean, if you take the time to invest and to understand, okay, we want to implement again our AI for recruiting or our AI for hr. I've seen a lot of company like few companies doing that with enterprise, right? Like how do we, our talent pipeline and people who are working at the company. It's like you have to really sit down and understand like what are the use cases at this specific company? I might be using use cases in a different way than you right now. But you know, like what are the like top like four use cases we want to prioritize for example.
Demetrios [00:39:23]: Exactly.
Lauren Kaplan [00:39:24]: Like what support are we going to need to actually implement it? Like on the engineering side and all that is stuff that like you can do with research, right? Like oh, do we want to prioritize support for Pytorch? I feel like this answer is going to be yes for that generally. But there's other like pieces too. Like what cloud service providers are people using and like I feel a lot of empathy for engineering community because it's like I, I get targeted with these things all the time. It's like be a cloud engineer because then I. Okay, okay, your like syllabus, like what are you offering to teach me? It's like well you're gonna learn, you know, Google Cloud. And it's like well okay, well what if you want to use different cloud? It's like oh never mind, you have to also learn this. Like well what are the commonalities like across those experiences that would like make it easier for people to like switch between the different, these different tools. Right.
Lauren Kaplan [00:40:15]: It's like going on topic but I think it's really like a lot of companies don't have an understanding of like AI infrastructure and how to build that and what's going to be in house versus not. And so they just, I don't know what's going on.
Demetrios [00:40:31]: Yeah, yeah. And that's where you get my favorite job title ever, which is like center of AI excellence. And I feel like that's their job is to go and figure out all right, how can we implement AI, what are the top use cases here? And so despite having the funny name it, there is some value in that. But the other piece that I was wondering about when you were talking about how there's these research projects that you have and sometimes they're going to be very broad and then other times it's going to be like super pointed and very short lived. How would you kind of juxtapose the two? How much weight is it going to take us to actually put this research project out there or gather all the data and then go through all the motions, et cetera? Et cetera.
Lauren Kaplan [00:41:26]: Yeah, I feel like you're asking questions about prioritizing and balancing research. And it's funny because that's often an interview question. It's like, tell me how. Oh yeah, tell me how you like prioritize, you know, these different things. I mean I think ultimately it, it also has like, it's hard to answer it without context. But like let's say you're in a company where it's, they haven't done research before. I feel like you'll find yourself maybe saying yes more often to some of these like shorter, like iterative, narrow, focused things. Because you're trying to build trust with folks and show do things and that you can, that you're not going to be a burden, you're not going to be moving too slow.
Lauren Kaplan [00:42:10]: But at the same time you have to also like be meeting with leadership and across the company to understand like yes, we're doing these like iterative, smaller things but bigger picture. What we really need to know is this. We don't know, let's say who our audience is or like what our market actually is or like we don't know what tools people are actually using. We don't know like how, like how can we meet, I don't know with model survey. It's a big pain point. How can we make that easier for folks? Like you have to be able to execute and advocate at the same time as a researcher and I think that's pretty challenging. And then also layered on top of that you have what we mentioned before, which is like tracking the impact. So you have sort of like executing then landing your research which is like really like sharing it out to different stakeholders.
Lauren Kaplan [00:43:01]: Like, hey, these are the insights we found. Here's the supporting data, these are the recommendations, these are the solutions. And then you have to, after you've landing it, it's almost like monitoring like what's happened since then while keeping the next things moving.
Demetrios [00:43:15]: And it sounds like it's a bit of education that you have to do like from your side to leadership to help them understand what the impact is. Have you seen that? Or is it a two way street where sometimes it's like leadership is telling you what the impact is. And so it's very clear.
Lauren Kaplan [00:43:40]: I think it's like a bit of a mix, but I feel like it's almost more like you actually as a researcher sometimes have to really explain what you even do in the first place.
Demetrios [00:43:50]: Like I feel like this call, just send them this podcast, that's all.
Lauren Kaplan [00:43:55]: I don't know I mean, it depends how this, I want to see it after. I wonder how it's coming out. But I mean, part of the reason because when you invited me I was like, hey, is this going to be valuable to people? What do we want as outcomes as it could be helpful to have some clarity of to what do we actually do? How can we collaborate for the ultimate goal of improving like our work for one another in a context where everyone's struggling and like taking on sometimes like the job of three different people across different functions?
Demetrios [00:44:28]: Yes. Yeah. Speaking of which, you mentioned how engineering, especially when you were at Meta, they were great partners. Are there certain things that you look for or that, that are like green flags with a partner when you're going and you're trying to get this research done, what are things besides them being very enthusiastic on a doc?
Lauren Kaplan [00:44:53]: Well, I think when I, when I see things like in terms of like, are they active and like getting feedback, are they community, like, are they engaged like with external communities? Right. Like, are they like parts of like, are they contributing to open source communities? Like that's also like a signal that they really want to stay close and connected. Right. With users. So that's definitely a, like a very important piece and I feel like engineers are actually like very, can be very good at that. Right. Like when you're, when you're doing well with a, you know, with a team and the engineer goes out and gets feedback, what you're going to hear is going to be really close to what you're finding with your research. Because there's just such a strong connection between the engineering team and like the external audience.
Lauren Kaplan [00:45:49]: And so like that's powerful to have because then they, the engineers also will see the value of like, yeah, like, of course we need to stay close to what people are doing and we need partners and like resources to do that. So like they see you as a resource and they have that hunger and appetite to like mutually succeed together. Right.
Demetrios [00:46:11]: And you create the tangible assets from these research projects. But then how do you make sure that something is actioned on them or does. Is it kind of out of your hands once, once you've created that, it's like, yeah, I've done my part because I, I know you mentioned before where there was that disconnect, there was the user research team, but then nobody was doing anything with any of the stuff that they were creating.
Lauren Kaplan [00:46:42]: Yeah, I mean, I didn't work there, so I don't know what's going on exactly.
Demetrios [00:46:45]: At that sounds dysfunctional. Yeah, yeah.
Lauren Kaplan [00:46:49]: Well, Something's, you know, they're just facing challenges and there were some really great people that I talked to. So you know, I don't want to like say anything negative, but I think a lot of it's just organizational and like, that's why like, I think part of what we had planned to talk about too was like, when does research succeed versus not? And it's like organizational. And then there's also like components of like as a researcher, like are you going into a situation where there's like what we would say if there's like a high maturity and awareness of what you actually do, Are you going into a situation where there's a very low awareness and understanding of what you do and having to like build all of that up? Because if you're going into a situation where the research maturity is high, I feel like you have a lot more potential to have impact because you've already established this function. Right. Among the other like organizational factors that have to have alignment. Right. And also it depends too because it can be a mix of like top down thinking where it's like, you know, executives, there's only so much you're going to be able to change and you're just kind of going with what they need versus like bottom up. And so there's I think also dynamics with that within organizations to navigate as well.
Demetrios [00:48:03]: When you've seen low maturity in this field, are there things that you feel like can help bring the maturity up? Is it just a matter of time and more experiences with the user experience, researchers, or are there other things that we can do?
Lauren Kaplan [00:48:26]: Yeah, I mean I think one piece is like as a researcher, if you're in that context, you almost have like a second job of like doing a lot of extra work to really make sure that you're like meeting with people throughout the company, like aligning on like what they need and what's important to them, explaining what you do at a level that you might never have had to do before. You might even like be building up the operations for the company as well and trying to convince your stakeholders of like the value of investing in having like platforms to even be able to do your work. And you're going to have to be able to really explain things in ways that you like repeating myself you haven't had to do before. And so I think when it comes down to it, it's also the same thing with products and with AI. Like people don't engage unless there's trust. And so I think you need to be in the right fit for a company and they have to be the right fit for you ultimately like you can do all of that work but if people don't have that trust through collaborating with you over time, then it's like a good signal to move on. But there's definitely, you need to be very patient, self reflective and empathetic of like hey, not everyone's going to know what I think is this very simple thing and I need to explain it and why it's important and just be very patient. I think is advice that I would give to a researcher but I realize like this audience is not necessarily researchers, but I think the collaboration piece and working closely is important and I think it's been really challenging because like some organizations just had a lot of infrastructure like with like you know, the pandemic when they went remote that they just had like strong culture and collaboration whereas other companies didn't.
Lauren Kaplan [00:50:18]: So like any work is going to suffer if you don't have the culture. Right. And you don't have that opportunities for people to work together, whether it's remote or in person. And some cultures are more in person and you don't really know that until you join. Right.
Demetrios [00:50:35]: So yeah, it's, it's almost like you have to evangelize for yourself as you are explaining the role in, in a way and help see the other person's. I imagine with time you will get to see what each stakeholder is looking for and how they are working or what, what's really impactful for them. But in the beginning, if it's all on you and you're the one who has to do this across the organization, that can be a bit daunting and overwhelming and it will take time for you to understand each stakeholder's needs and wants. Now let's talk about the career paths into being a user experience researcher. I think there are some fascinating for me, one thing that stands out or that feels like there's like this bi directional, probably road or superhighway is someone in the product position that would go to a UX researcher position and then vice versa. Have you seen that? And what are some other ways that you've seen people come into this position?
Lauren Kaplan [00:51:54]: Yeah, I mean product management and user experience researchers are very similar in that they're very collaborative and need to stay really close to the users of a product and like drive the direction in terms of like the vision and strategy for products. But I think there's so some like, you know, rough pass I would say is like I could start with mine Right. It's like, is like, let's say you're a PhD and either like a social scientist or you could be like a neuroscientist, like, or you could be an anthropologist, you know, from a range of different backgrounds. Right. And you've just been maybe you've been working on like policy research that has impact, that's changed something. Right. Like, so I got to work on homelessness and got to do work that impacted on that. And so that sort of helped me to transition into like industry.
Lauren Kaplan [00:52:43]: Right. And then in terms of like another path I would see is like people who are very focused on like human computer interaction. So like very like human factors focused, like, makes me think of like kind of like a Stanford person. They go into industry and then another. And then a third path I would say is like you could even be a designer. So because designers do like a lot of work, especially UX design of like understanding like user flows and like, you know, the product. And so they're like, you know, you see a lot of roles, like design researcher roles. And I've also noticed a lot of roles want you to be both a designer and a researcher.
Lauren Kaplan [00:53:22]: So that's interesting. And then yeah, you could be in marketing as well because again that gets into like using some of the methods to understand audience. And I think maybe for this, you know, for engineers. I've definitely met people who used to be engineers that like switched into ux. So there's definitely like a range of different paths.
Demetrios [00:53:48]: I could see the value in a marketer wanting to do this or coming into this. Because if you are able in. And it's almost like the way that I look at it is, you know how there's sometimes politicians that will go and work for the big companies that are paying them when they're a politician and then all of a sudden they retire from being a politician and then they go and sit on the board of one of these big companies. It I see that with like the value of being a marketer and then going and being in being a UX researcher and then going back to being a marketer type thing would be huge because you go and you're trying to speak to the audience, you're trying to understand what hits. And if you ideally are learning different marketing chops and the nuts and bolts of just doing marketing, that's great. But it doesn't matter if you don't truly understand the customer. And so that's why I could see the value in being a user experience researcher, understanding the customer. And then Going back and like leveling up.
Demetrios [00:55:04]: If you ever wanted to go back into marketing, assuming you're in the same field or the same space as you go through these different positions.
Lauren Kaplan [00:55:13]: Yeah, and something with the engineers too. Like I noticed there's a few that I've met that are like having their own consultancies, like with AI, like trying like helping companies to figure out how they're gonna implement AI. And I've noticed that some of them are like, oh, wow. I'm realizing that like this project's. I actually could really use someone on the UX side as I'm figuring out and implementing what this client wants. And so that could also be a potential point of collaboration if you have your own company as a consultant. And you might notice that people are asking you questions that are very ux.
Demetrios [00:55:50]: Like there is a little bit tangentially related to that. There's so much more than the tech when it comes to the user experience. And we kind of touched on that before. But the user experience, maybe you can break down all the different areas that aren't tech that still are part of the user experience.
Lauren Kaplan [00:56:20]: So not the intro, but what to would make a good experience beyond.
Demetrios [00:56:25]: Yeah, exactly. Not, not the exact, like, oh yeah, you can deploy this and then it creates low latency. But how does the, how does that actually look when you're. I. I'm just thinking about, for example in this consultancy issue where it would be nice to have somebody that is. Is a user experience part. And there's a whole lot that goes into. I get.
Demetrios [00:56:57]: I guess it could be solved with tech, but I'm trying not to be like technocentric on it. But there's a whole lot of just like the human aspect of, hey, we are keeping the client updated on how we're moving and where we're going and what we're doing. And we're also making sure that these things are checked off, like the visibility and the communication. And those are. Those are some things that come to mind. The transparency of the project.
Lauren Kaplan [00:57:31]: Yeah, I mean getting into like staying in communication with folks and the reporting and like having it reminds me like having like dashboards and like showing things like visually to folks of like what's going on is like definitely something you could collaborate on. I think in terms of like getting back to the question of like, what's a good experience? I think like some like measures that people use are things like getting back to again, like it's pretty like kind of like core things are like satisfaction, ease of use, what are the pain Points I feel like. And how can we improve? I think if you have those like four pieces that's like a good place to start if you haven't done it before. And I think what you said also of like making things people centric, like in the case of being like a consultant in AI, yes you have to be keep both your client as a person, human centric, but also what they're actually making themselves. And you have to have a knowledge of like all right, my client wants to implement this AI for again I'll just go back to the HR thing but they don't really like have a like clear understanding of like their use cases or people's needs. And that's like exactly. What UX research does is like our role is to advocate for people so that things are built around them and that AI is people centric. Like that's sort of the core of it, right? Because without that ultimately these like long term things will fail.
Lauren Kaplan [00:58:59]: Right? You'll implement, you'll think short term things are working but then you might have backlash, right? And a lot of companies and that are more well known know how the damage to their brand that that can happen, right? Like if you roll something out and people have a bad experience with like let's say Gemini or something, it's going to be all over the news, right?
Demetrios [00:59:21]: Shit.
Lauren Kaplan [00:59:23]: So being able to like have the foresight and like have a long term view is also super important there.
Demetrios [00:59:31]: The thing that you said, four kind of pillars there that I wanted to write down but I didn't catch em all. It was satisfaction, ease of use, what.
Lauren Kaplan [00:59:42]: Are the pain points and like what are the areas to improve?
Demetrios [00:59:47]: Have you seen folks that are not user experience researchers still go out there and do this type of research because it's almost like they're. It's not necessarily that their position is calling upon them to do it, but they see the need and before they go and they invest or they make a decision to invest all this time and energy into building out an ML platform or building out or hiring teams and then choosing a centralized way of using a platform, et cetera, et cetera. I'm just trying to like bring it back to what engineers probably will do. The it feels like this can be done by engineering teams and this is almost like a new tool in their tool belt as they're trying to think through some of these hard decisions and recognizing oh yeah, like you said it's not the most comfortable but we could get some signal in a week and maybe we can really dive into knowing that we make the right decision here.
Lauren Kaplan [01:01:13]: Yeah, I mean, engineers definitely can and do do that. And also I think like when you were talking, founders came to mind, right? Because if you're starting your own company, right, you're going to be really, I mean, I might be generalizing, but what I've seen is like, I might be really, really focused on like having the best possible products. And a big part of that is talking to people. So I've had people who are, you know, founded a company and they want like feedback, right. And they're reaching out and they want to talk. And so you see a lot of that as well because they know their, their companies aren't going to be successful if they haven't like gotten a sense of like their market and how they could be positioning themselves.
Demetrios [01:01:57]: Yes. Awesome. Well, I am so glad that we did this.
Lauren Kaplan [01:02:03]: This.
Demetrios [01:02:03]: And the whole reason that I, you know, like, I find this fascinating is for that very fact is that I've heard too many people come on here and say, we built a great product internally and we launched it to crickets. And really it feels like some of these tips and tricks on becoming a better researcher and understanding the pain points and the ease of use can be ways that you will avoid these problems. Because if people are not using your tool, there's a few reasons that that could be for. Right? Like it could be that you just didn't do a good enough job letting them know that the tool is there so you have an awareness problem. Or it could be because the tool sucks and so nobody wants to use it because when they use it it's like, eh, this is a lot harder than when I do it on my own. And so for that problem, the tool sucks. This is a great way to counterbalance that.
Lauren Kaplan [01:03:15]: Yeah, I agree.
Demetrios [01:03:17]: Is there anything else? Like I know that we had thought about talking about developer experience and how we apply everything to developer experience. Is that like, do you feel like there's things that you want to touch on there?
Lauren Kaplan [01:03:36]: I think what I had planned to talk about was really like, you're just taking all of what we talked about and applying that specifically to developer focused tools. And also like their experiences, for example, like both in just their careers or at the organization they're working at and really understanding people's needs. And I think that Google does a pretty good job of that with Dora. They have a team that's like, they publish their annual report and they're really focused on that developer experience. And I think it's a good resource. I linked it into the doc I shared with you. Because that's another area too of like if, let's say you're, I don't know, you're an engineering manager and you're trying to think of ways of like mitigating pain points for your team. Like you could do like an internal, like you could actually do that internally, right? And do like a developer experience research and do like a survey throughout your company and understand like, hey, okay, my team, most of my team uses this.
Lauren Kaplan [01:04:40]: So a gap here is that we need to have more support for learning like this other tool if we want to switch or like, you know, people aren't feeling that great about this thing. This is a solution that I can do to support my team. So I think that's another important piece that could support developer teams as well.
Demetrios [01:05:01]: God. As you say that it's so true that just the. Hopefully every engineering manager is doing this, but taking the time to survey, especially if you have big teams and or you have a big engineering organization to recognize where pain points are for the teams and that's where you can spend your energy and focus. It's. If there's signals coming out of a survey saying, oh yeah, we're having, we're spending a lot of time because we're always having to go back and fix the data quality. It's like, maybe we should start figuring out how we can get better data governance, right? Or if we are spending a lot of time or there's, there's a lot of pain because the open source tools that we're using on our ML platform are not reliable or doing something. Oh, should we maybe look at how to fix that? And so yeah, doing, doing this research from a managerial perspective or a leadership perspective, it just feels like something that should and hopefully is constantly happening.
Lauren Kaplan [01:06:16]: Exactly.