The Only Real Moat for Generative AI: Trusted Data
speakers
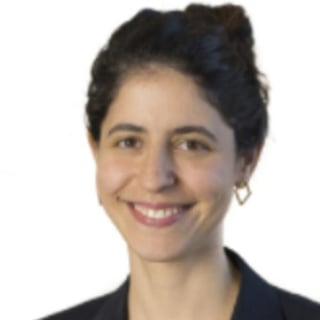
Barr Moses is CEO & Co-Founder of Monte Carlo, data reliability company backed by Accel and other top Silicon Valley investors. Previously, she was VP Customer Operations at Gainsight, a management consultant at Bain & Company and served in the Israeli Air Force as a commander of an intelligence data analyst unit. Barr graduated from Stanford with a B.Sc. in Mathematical and Computational Science.
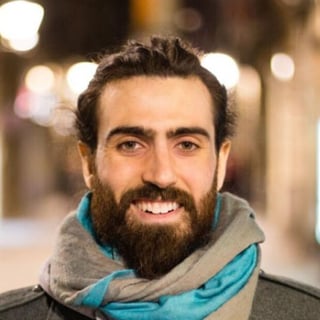
At the moment Demetrios is immersing himself in Machine Learning by interviewing experts from around the world in the weekly MLOps.community meetups. Demetrios is constantly learning and engaging in new activities to get uncomfortable and learn from his mistakes. He tries to bring creativity into every aspect of his life, whether that be analyzing the best paths forward, overcoming obstacles, or building lego houses with his daughter.
SUMMARY
Your leadership team is bullish about gen AI — great! So how do you get started? As open source AI models become more commoditized, the AI pecking order will quickly favor those companies that can lean into their first-party proprietary data the most effectively. And too often, teams rush headfirst into RAG, fine-tuning and LLM production while ignoring their biggest “moat”: the reliability of the data itself. Barr Moses, Co-Founder and CEO of Monte Carlo, will explain how data trust can be baked into your team’s gen AI strategy from day one with the right infrastructure, team structure, SLAs and KPIs, and more to successfully drive value and get your AI pipelines off the ground.
TRANSCRIPT
Slides: https://docs.google.com/presentation/d/1JPxdwsTopkEE0xSRetSWC-4Z9Z_sGVt2/edit?usp=sharing&ouid=108999209131560878693&rtpof=true&sd=true
Demetrios [00:00:00]: I'm excited to present bar. I had, I think, in podcast. What she's been doing since then has always fascinated me. The idea of data quality and just monitoring your data is super top of mind and I want to hear all about what you got for us. So I'm going to hand it over and we'll see you in like 15 minutes.
Barr Moses [00:00:25]: Great to be here. Here we are. Can you believe it? Da da da da. We made it. Awesome. No, but seriously, thank you so much for joining. I really appreciate many of you have come from afar and appreciate you all taking the time to spend just ten minutes today doing a lightning talk about the moat for generative AI trusted data. Just for introductions.
Barr Moses [00:00:51]: My name is Bar Moses. I'm the CEO and co founder of a company called Monte Carlo. I'll tell you just a little bit about Monte Carlo, but we'll spend most of the time on the content of this. Looking forward to chatting with some of you after. So, Monte Carlo is credited with creating and leading a category called data observability. It's one of the fastest growing technologies, is sort of trajectory over time, and maybe the thing that we're most proud of as a company and the thing that I'm sort of most fulfilled by is our amazing customers. Actually, several of you in the audience here, what you see here on the right hand side is sort of user reviews and sort of the love that customers give us is the thing that makes us the happiest. So, Monte Carlo, we really focus on making customers as happy as possible.
Barr Moses [00:01:37]: That's just a little bit about us. Let's turn to the meat of the talk. Monte Carlo is really famous with our memes. I'm not a funny person, so this is the company's memes, but I have to start with it because everyone loves it. So maybe we can do by show of hand here. Who resonates with this meme or just speak to them? Okay. You're laughing in like 70% of the audience, so that's good. This is the reality that many of us are dealing with today.
Barr Moses [00:02:06]: Right. And it's not a simple reality. Many of you actually are facing a lot of pressures, but what are we here for? Like, what are we actually. Why are we sort of struggling with this reality? I think, you know, there's a lot of talks today about sort of rag and fine tuning, a lot of different things. But, you know, kind of from the introductions that we did here in the audience, what are we all here about? We're trying to figure out how to build great generative AI products. Right. And what is the path for us to do that today? Today, the reality is we all have access to the best models built by 5000 PhDs and a billion dollars in GPU's. We all have access to that.
Barr Moses [00:02:42]: However, in order to really make generative AI useful for the enterprise, we have to introduce proprietary data. That could be internal data or third party data. It has to be the data that a company or an organization has in order to make generative AI powerful, in order to either create personalized experiences for your users, or in order to automate your own business processes. Without that internal data, it's very hard to actually make generative AI useful. And so really, sort of the competitive advantage is with that data now. It could be used with rag or fine tune, whatever method you like. But the key is, in order to make generative AI compelling and powerful in the enterprise, we really need to think about our proprietary data. Now, the problem is, of course, everybody assumes that proprietary data is in great shape and in awesome shape.
Barr Moses [00:03:30]: But who here has confidence that their proprietary data is 100% right and everything is good with it? No, no. Show of hands. So we actually did a full survey with hundreds of people, and let's see what the data shows us. The first thing that we learned is that 100% of data leaders feel pressure from their leadership to build generative AI. Literally every single person surveyed. We also learned that 90% of them have succumbed to the pressure and are actually building generative AI, which is, I guess, is a good thing. However, 90% of them don't think their leadership actually sort of has realistic expectations. What is the root cause of that? What is the source of that? And here is probably the sort of stat that's most mind boggling to me.
Barr Moses [00:04:19]: A staggering, almost 70% of data leaders do not think their data is ready for AI. That means that literally only two or three of us in every ten people actually think their data is ready. To be honest, this is higher. This is sort of higher than what I thought it would be. Now, the interesting question is why? Data is new. We've been here for a while. We've all been in this space for a fairly long time. Why is this the case? Here's what I'm going to propose, or sort of the theory that I have.
Barr Moses [00:04:52]: I think the data state has changed significantly in the last five to ten years or so. However, our approach to data management has not changed at all. And I'll explain what I mean by that. But if you really reflect on sort of the last decade or so everything around how we. Everything, how sort of our data state, our data infrastructure has changed radically. Like it's. It's very different the way that we work today, ten years ago, but how we manage data that hasn't changed at all. And that's quite interesting to think about.
Barr Moses [00:05:22]: Let me explain what I mean by that. Ten years ago, the most important thing for a business was actually the applications in infrastructure and websites or sort of products that a business was using. Today, all of that is based on data. So data is powering your dashboards, but also your products and also your AI and ML. And so we need to have a more sophisticated understanding of what our data state looks like. And I think if you break down our data state, there's three core components to it that are most important. The first core component is the data sources themselves. So this is data that you're ingesting.
Barr Moses [00:06:01]: Again, it can be third party or first party. Oftentimes we don't have control of that data. The second component is the code. So this is code written by a variety of people, engineers, machine learning engineers, data scientists, analysts, lots of people, actually writing code that transforms this data. And then the third component of this, of our data state, is actually the systems. It's the infrastructure and applications that run all of these jobs. Now, this has actually become quite a complex web of dependencies, if you will. And the thing is, problems with data can happen as a result of each of one of those three things.
Barr Moses [00:06:44]: So it could be as a result of bad data that got corrupted or just didn't get didn't arrive. It could be a result of mistake in the code, and it could be as a result of an issue with the system itself. When we actually think about our data state holistically, we need to think about each one of those three components. We cannot focus only on one of them. However, in our day and age of today, despite the complexity of our state, of our data state, more than half of those surveyed still use manual rules to actually make sure that the data is accurate. And so they will state things like a field needs to be between the values of 23 and 45, or a particular field needs to be some level of some percentage of null value, et cetera. And so it's really mind baffling that our data state is so complex and there's such an interweb of dependencies, and we're still using manual rules in order to actually ensure that the system is reliable and holistic. We're clearly doing something wrong.
Barr Moses [00:07:47]: Here's a different way to think about how we should do it. I don't think manual rules are going anywhere, and I think there's a lot of things that are staying, but I think we have to change the way that we think about actually managing the reliability of our data state overall by double clicking into the code, the data and the systems. So let me actually walk you through kind of an example of what that would look like. If we have an issue with the system, if we have a problem with the data, how we trace back through what that may look like and how we can work through it in this new paradigm. The first thing I want to say is we have to start by having this sort of overview of the entire data state. So we have to have one unified view over the data, the systems and the code in one place. And let's say we take some sort of imaginary incident that we have. In this case, what you see here, there's a field metric anomaly with a value that has a high percentage of null values.
Barr Moses [00:08:42]: Now, this issue can be detected either with AI in an automatic way with no human intervention, or it can be detected with a manual rule that says, you know, the percentage of null values cannot be higher than x. Regardless, we start with the level of understanding that there's an issue, we start with detection. And so actually understanding that there's a problem is the first step, again, can be either AI or manual driven. However, most approaches stop here. So most data teams, data organizations will say, oh, there's an issue, and then everything else sort of collapses from there. What would the next step look like? What's required from us in order to build more resiliency with these systems, ultimately building generative AI products? I think the next step is to actually rely on AI and lineage to help paint the picture. So can we actually use some of the things that we've advanced in AI and lineage to zero in on the root cause? And I'll give an example here. The first thing sort of the first incident is sort of the systems that I mentioned.
Barr Moses [00:09:46]: And so it could be, when I say systems, it can be really any ELT, general system, it could be airflow, DBT, Freumatica, whatever it is, to sort of transform the data. And in this case, this is actually a DBT instance. And you can see that there's a specific DBT job that failed that is correlated with this particular null value issue. There's also cascading jobs that might as a result have be problematic too. And so can we actually correlate a particular job at the same time that the null value issue happened in order to help us identify the root cause. So this is a fairly straightforward sort of like, systems detection issue. The second example here, what we're actually doing is doing a query comparison between different sort of code snippets. And so what do I mean here we talked about the second sort of component is code.
Barr Moses [00:10:42]: And so when an engineers sort of, um, transform data could be to create aggregates, for example, mistakes happen. And what kind of mistakes happen in writing code, for example, it could be a bad join. It could be a schema that changed schema error. And what people do today is actually manually comb through blocks of SQL code to figure out where the issue is that's correlated with the null values. What if instead of that, we could take, you know, actually like, comparing different queries to pinpoint the exact specific issue of what happened with the code and correlate that with the null value issue that we saw before? The thing is, oftentimes it's not just one of these things that happens, it's multiple of them at the same time. And so we talked about problem with the systems, problem with code. And then the last thing is tracing this back to an issue with the data. And so using lineage and AI, again, can we trace the null value issue that we saw downstream to an upstream source of data, let's say a transactional database, like Oracle, for example.
Barr Moses [00:11:48]: And in this case, it can trace it back all the way here and say, hey, there's a particular field, a particular segment that has a higher than usual percentage of null values. Maybe that's data that arrived from a particular geography, like California. Maybe it arrived from a particular source, like LinkedIn or meta, or maybe it's a particular partner that you relied on to share data at a particular time that hasn't arrived. And so actually being able to sort of trace back is the data, is the data issue that we've experienced downstream. A code, a systems, or a data issue is what really helps us take this to the next level. So we're moving beyond simply detection of issue to sophisticated resolution and triage and remediation of problems. Now, when you're managing hundreds of thousands of data products in the wild, whether they be generative AI products or otherwise, you cannot do this manually. We have to find the way to build this at scale and do this across data systems and code.
Barr Moses [00:12:48]: The last part is, if you can imagine having all these alerts, just practically thinking about alerts flying between ten different teams, 20 different domains, you could be running around sort of with fire drills, all day long. What can you actually do about it? Again, using similar techniques, we can actually route the relevant alert to the relevant person. So in this example, we can let Wendy, our engineer, know that there's a particular anomaly and a particular issue that's traced back to these DBT jobs or this query change or this oracle table, for example, and that this needs to be investigated. On the other hand, we can let our CMO know that the analytics dashboard that they're looking at is under construction at the moment and hold their horses before they actually use this. And so actually with this way we're able to take all of sort of the data, the metadata and the context that we have and put it to use in a real application for folks. I have to wrap up with a meme, I promise. Another one. This is my favorite meme, actually.
Barr Moses [00:13:59]: I think as long as we continue with the traditional paradigm of how we're thinking about data quality, data observability, we're going to stop at detection, we're going to stay with everything that's sort of manual. We're not going to be able to deliver generative AI products. Everyone here in this room and online has a big challenge ahead of us. We need to figure out, you know, I was talking about this with one of our customers. This is a historical moment, right? We're all sort of fortunate to be part of this, you know, very important moment in history and it's up to us to chart the path forward. And I think if we don't move this, don't make this jump from the traditional way of managing the data state, we're going to fail ourselves. And so we actually need to be able to make this leap not only in how we think about our data state and how we deliver generative AI products, but also in how we manage the reliability, the observability and the scalability of these products. And hopefully this does not happen to you all if we do that.
Barr Moses [00:14:57]: My name is bar. Thank you so much. Really enjoyed chatting with you all.
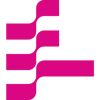