Vision and Strategies for Attracting & Driving AI Talents in High Growth
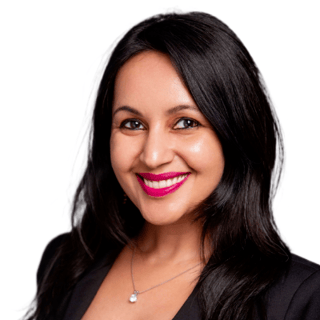
Shailvi is a seasoned Data Leader with over seventeen years of experience growing impactful teams and building technology products used by hundreds of millions of users. Her career includes notable technology roles at Salesforce, Fitbit, and as the Head of Data at Strava. As a fractional executive, she has consulted, advised, and invested with multiple high-growth startups. Shailvi has spoken at nearly 100 global conferences, coached more than 500 individuals, and authored the best-selling book "Self-Advocacy."

Olga has over 20 years of experience in Language Technology, NLP, Machine Learning, Global Content Transformation and AI Data development and is passionate about both growing businesses through driving change and innovation, and mentoring the next generation of the industry talent. Olga started her career in language technology working on Rule-based machine translation, gradually expanding her expertise into other broader applications of NLP and Machine Learning to enterprise localization workflows. Olga has also served as President of AMTA, currently serves as a Technology Program Sponsor for Women in Localization. Olga is a frequent presenter at industry events, such as TAUS, GALA, Localization World, and various podcasts and webinars, and an active contributor to technology publications. Olga received her MA, Linguistics/Germanic Studies at UC- Berkeley and BA/MA in Linguistics from St. Petersburg State University.
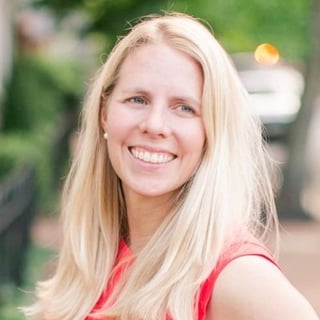
Dr. Ashley Antonides is the Associate Research Director for AI/ML at Two Six Technologies, where she spearheads research initiatives in AI security. With over two decades of experience leading R&D teams in machine learning and data science, her past roles include Research Scientist at the National Geospatial-Intelligence Agency, Principal Data Scientist at Maxar, and Chief AI Officer at Anno.ai. She earned her BS from Stanford University and her PhD from the University of California, Berkeley. Dr. Antonides has served on the board of the Data Ethics Consortium for Security (DECS), as a co-chair of the Open Geospatial Consortium GeoAI working group, and on the American Statistical Association's Committee on Data Science and Artificial Intelligence.
Attracting and retaining top AI talent is essential for staying competitive. This panel will explore how to craft and communicate a compelling vision that aligns with the organization's evolving needs, inspiring potential hires and keeping current employees motivated. The discussion will offer actionable strategies for sourcing top talent, adapting to changing needs, and maintaining company alignment. Attendees will learn best practices for attracting AI professionals, creating an attractive employer brand, and enhancing talent acquisition and retention strategies. Lastly, the panel will cover structuring and organizing the AI team as it grows to ensure alignment with business goals. This includes optimal team configurations, leadership roles, and processes that support collaboration and innovation, enabling sustained growth and success.
Shailvi Wakhlu [00:00:09]: Welcome, everyone, and thank you so much for joining this panel. We have some amazing people that we're going to talk to. So, yeah, would love to kick it off. Ashley, why don't we go ahead with some introductions?
Ashley Antonides [00:00:21]: Ok, sure. Is this on? Yes. Ok. Hey, everyone, I'm Ashley Antonides. Right now I'm the AIML research director at two six technologies. For most of my career, I've led mixed research, data science, ML and developer teams. Leaning a bit more toward the R and D side, I spent about ten years in the DoD and a research organization, have been involved with a couple of early stage startups that were very R and D focused. And then now the work that we do is very research focused, too.
Ashley Antonides [00:00:52]: We do a lot of work with DARPA and other government research sponsors and I will pass it to Olga. Okay.
Olga Beregovaya [00:00:59]: Hi everyone, I'm Olga Beregovaya. I'm a vice president of AI at Smartling. We are an AI powered translation platform. My entire career has been in natural language processing from day on. One early days of machine translation, summarization, sentiment analysis and so forth, and slowly working my way into more diverse AI.
Shailvi Wakhlu [00:01:20]: Awesome. And my name is Shailvi Wakhlu. I run my company called Shelby Ventures, working as a fractional executive with early stage startups. Formerly I was the head of data at a company called Strava, and now I'm also a best selling author on a book called Self Advocacy. So with that, let's kick off our topic for today, because we have a lot of accomplished people here with us, and I think AI in general is a very hot topic. Everybody's focused on it, everybody's talking about it. But the thing that people tend to focus most on is the technology aspect. And what I'm really curious to learn from both of you is what about the people aspect? How do you find the exciting talent in this field? How do you sort of set them up to build the solutions that we want to build? And Olga, I'd love to start with you.
Olga Beregovaya [00:02:11]: Yes, I already hold the microphone again as well. Yeah, I mean, people could not be more important. And we all know, I mean, we all from the same space here, we all know how hard it is to find talented in our space, how hard it is to retain talent. So maybe I'll go into how do you keep people? I think it's all about an interesting job in this modern time and day. It is so exciting to be in the area, in the field that actually just keeping people intellectually stimulated. I mean, all the other perks aside, basically, I think that's key. And I know we'll go into more detail later.
Ashley Antonides [00:02:41]: Yeah, I think in addition to thinking about sort of technical skills when hiring to, I'm sure a lot of us think about just overall team composition, how the team is going to work together. I think that a learning culture is really important, especially now. There's so much new coming out every day. So how to build that team culture where everybody is like reading papers and, you know, okay to ask questions and really collaborating and building off of each other's energy. I think we'll talk about kind of diversity later, but I think that's also an important component. Just thinking about the AI systems that we're building are interacting with people and how people can bring different aspects to thinking about how what we're building is going to affect them and different people across different walks of life.
Shailvi Wakhlu [00:03:30]: Whatever we build, it affects people. Companies always try to have that perfect alignment with company goals, product roadmap, and that talent that is internal. So how do you sort of think about that structure that helps you support that perfect alignment or that perfect teams divided up into those functions that actually support those goals?
Ashley Antonides [00:03:55]: So one thing that comes to mind is actually just areas where I have seen maybe friction between teams, or as we're trying to alliance, say, toward the business goal of delivering a product. I think maybe one kind of common division is between the developer team and the data science team. Maybe that will change over time as there's more blending between the roles, but it can. I've seen a lot of teams that are structured as like the dev team versus the ML team. And so, I mean, I think one way to address that is if you have sort of an overall leadership role, whether it's the chief product officer or the CTO who really is coming, has empathy and background in both, both perspectives, and is helping to build that infrastructure between the teams for model delivery and the different sort of approaches and workflows that those different roles have. One other area where I have kind of seen some friction is as more and more companies or organizations are realizing that it's important to have a chief AI ethics officer to be addressing ESG, having maybe more data governance. Sometimes the technical team can see that role as almost like an adversary or like an auditor who's coming in. So what I've seen worked well is where you can bring that person actually be part of the technical team, and there's really a dialogue about what are the true requirements from that perspective, so that the technical team understands that.
Ashley Antonides [00:05:18]: And then that is incorporated into the overall kind of delivery lifecycle.
Olga Beregovaya [00:05:22]: Okay, I completely agree on a couple of things I wanted to add. We all see that at the end of the day, it's not that difficult to hit an LLM API and deploy an application internally. And what I see also, and I don't know whether it's friction or opportunity, you often see, especially in large enterprises, this accessibility that can drive in equal parts collaboration and friction. So what I'm seeing a lot is on the product team, they just, I mean, I'm in the translation space. Hey, let's just plug in, chat, GPT, and let's translate everything with GPT. At the same time, you discover that in digital marketing there is a separate initiative because everything is so accessible. So I think it's super essential to have again, same thing, centralized governing office, and while again fostering creativity, making sure that there is internal alignment as opposed to billion scattered individual initiatives. I also think what's essential now, again, there are so many aspects of equally developing and deploying AI.
Olga Beregovaya [00:06:19]: You really need to have a champion in every function. Dev, product marketing, compliance, HR, every function now plays a role. So again, it's all about identifying the needs and the role of every function and making sure that there is alignment.
Shailvi Wakhlu [00:06:34]: I love that idea. Like have a data or AI liaison in every department.
Olga Beregovaya [00:06:39]: And actually, to add to this, I was on a panel with a lady from IQ here, and they indeed have the digital ethicist that actually looks, and I'm pretty sure it's not unique, she was speaking specifically about this role, AI digital ethicist that's really looking after ethical deployment and distribution of roles within the company.
Shailvi Wakhlu [00:06:57]: That's really cool. I also think often when we talk about ethics, one of the topic that comes up is that if we have very homogenous teams, we end up having products that have a little bit of a blind spot on what is possible. And the common answer that a lot of people say can help solve for that is to have more diversity in the talent profiles that you have in AI. What about that aspect? How do you prioritize it in your teams? What do you think about it? And most importantly, what happens if we don't prioritize it? What happens if we don't prioritize having diversity in AI?
Olga Beregovaya [00:07:32]: Okay, maybe I'll go first. I'm pretty sure we've all seen similar research that if you don't ensure diversity equally on model data collection, model development and model implementation team, you actually do get this one sided view. And we have empirical evidence, especially when it comes to different kind of cultural and all sorts of biases. Actually, one of the techniques, known techniques of mitigating data biases and algorithmic biases is actually ensure there's diversity on teams. So where I work, for instance, what we see is we have very, very diverse representation. Again, I come from the language AI space, one of our chief data scientists. She's not only she a chinese lady, she's also a young mom who is raising a toddler. And it is pretty fascinating things that she notices from just her language perspective.
Olga Beregovaya [00:08:20]: She comes from a completely different language group. So she catches hallucinations and thinks about things that I would not even think about with my language background. But on top of that, she's a young mom. And what she says, which I absolutely love, is she says, look, I do two things at home and at work. I'm talking to a toddler. So my prompt engineering actually is very identical to what I'm telling my toddler give very specific instructions to somebody who doesn't quite understand but wants to learn.
Ashley Antonides [00:08:51]: So just thinking about. So acknowledging, I think diversity on AI teams is really important, how to build that. I mean, there's so many different aspects of diversity, but how to build that can sometimes be hard. You can often you look at teams and they may be homogenous. So I personally feel pretty strongly that the hiring manager plays a really important role. And one thing I like to ask when we're interviewing is just some basic question about kind of your question, like how do you think about diversity? How do you think it's going to be? How will it play in your role? And just even that simple question can actually, you can tell if the person has even like, thought about this at all. But I have seen where if you have someone who maybe hasn't thought about that, their team as they grow it, especially if you're in a high growth area where somebody is going to be hiring a lot, you can kind of see how that composition is a certain way over time versus someone who is really intentional and really wants to have that be an objective as they're building their team. I'll just leave it at that for now.
Ashley Antonides [00:09:58]: Yeah.
Shailvi Wakhlu [00:09:59]: I think another aspect there is also sort of, you know, we don't talk about educational diversity a whole lot. Like a lot of job descriptions in AI and data in general, they still sort of require certain types of education. They're not as open to people who've come from sort of a boot camp or self learned background. And I really think, like, you know, if you've excelled in a different field and then you choose to come to AI. Like that's a huge, huge benefit for AI that you get to learn from an expert in a different field who's also now applying, applying to that area. So, yeah, so I think, I think.
Ashley Antonides [00:10:38]: That'S a really good point too. I think, yeah. The role of also just the domain expertise and just the lived expertise of the folks on your team and how to leverage that I think is really important.
Olga Beregovaya [00:10:51]: I really like what you said about the outside perspective, fresh perspective, right. Because a lot of people, we've been in the same field for years, and then somebody comes in from different industry, somewhat adjacent skillset, they will have all the capabilities of learning on the job, right. But they will bring the perspective that we would not have.
Shailvi Wakhlu [00:11:06]: Absolutely, absolutely. So sort of shifting gears from, you know, we've all had a tough time hiring and identifying the right talented, but for the talent itself. So there are so many people who are excellent AI innovators and everybody's pitching to them. Everybody's pitching to them. Like, come work with me, my problems are great or my company is great. So what are your strategies from separating yourself from the noise? How do you make sure to set a really clear vision and a clear pitch so that AI experts choose you over the next company?
Olga Beregovaya [00:11:43]: Maybe I'll start here. I think you make the message of impact. What we're doing is real and it's going to be impactful and it's going to drive outcomes. So I think it's much easier to attract the talent if you say, hey, this is where we're going. This is the impact that we're going to have on our customers, society. And, I mean, you can take it to any degree and any scale. And if they see that they're working towards a specific tangible goal that contributes, I think at least that's been something that's been working for me. It's got to be interesting and you know where you're going with this.
Olga Beregovaya [00:12:14]: So that's one of the techniques. And obviously, again, if you're inviting somebody into a diverse team with great culture, that's something usually what I would do, I would do a round or what we would do a round of interviews. So management interviews, senior management peer. So the person really gets to meet everybody and be motivated?
Ashley Antonides [00:12:31]: Yeah, I think so. For a lot of the teams that I work with, again, are very research focused. So there is a lot of interest and motivation or questions around what conferences am I gonna get to attend. Is there an opportunity to publish? Is there an opportunity to at least do maybe like blog posts. So having that record of like, hey, our team is published here, you will have an opportunity that's prioritized and also just people talk about what the training budget is or what the training availability is. So folks know like, hey, I'm gonna be able to go and keep my skills up and go to conferences is really important. And then I like to talk to the learning culture too, about kind of what cadence we have for internal reading groups, for workshops, for cross training across maybe other groups within the organization. I think that's really key, just that folks know there's gonna be opportunity for them to keep upskilling and keep learning new things.
Shailvi Wakhlu [00:13:21]: And actually, I'm very curious, but in your team, do you just suggest that people in the team go for conferences or do you almost require it? Is industry thought leadership part of their criteria for career laddering?
Ashley Antonides [00:13:37]: We don't require it, but we actually, as a team, we try to identify maybe three or four kind of key conferences throughout the year. I find that there's value if you can send maybe like a few folks to the conference, especially if there's concurrent tracks, they have an opportunity to come back and kind of talk with each other. So we try to do that kind of collectively of like what are going to be like the CDPR nurrips that we're going to send the team to through the course of the year.
Olga Beregovaya [00:14:01]: Yeah, maybe I could add to this, and there is a person in the room way more qualified to speak on the subject because Jen there manages all of our conference submissions. But no, in reality, I think we're almost crossing over to please publish. So people know that they will be given the budget to travel to conferences, but also know that they will be encouraged, greatly encouraged to publish. One thing is, because we are in practical application of AI, we actually have something that, and those of you from academia would probably agree, we have the data, we have the field data that we can share. So quite often when we go even more so to research conferences, we bring something that quite often folks from academia might not have as much exposure to. So we're usually a very welcome addition because we come with practical, real life case studies. So, yeah, encouraged and welcome to publish.
Shailvi Wakhlu [00:14:49]: So, yeah, awesome. Awesome. That sounds lovely. All right, so the next topic. I know Olga, this is a favorite of yours, and I know Ashley, you're passionate about it too, but I, we know that AI implementation still requires a lot of contributions from the human in the loop. So how do you sort of think about that? Is there specific strategies that you have for, again, identifying the right people with the right us.
Olga Beregovaya [00:15:15]: Yeah, I mean, this conference is about quality in AI. And we still agree that despite all the mitigation techniques, models do hallucinate, data is biased. So you do need, and even more so again, in our industry and language industry, you do need human in the loop, and you'll probably need human in the loop in the foreseeable future. Equally for direct assessment, for human ranking, for validation, for post editing, for fact checking. Not an issue, but one of the key factors there is quite often that would be a smaller gig. Quite often that would be. Sometimes it can feel like a repetitive job, like if you need to click x number of images and decide whether it's, I mean, right now we're beyond a cat or a dog, but there are other criteria, or if you need to validate an output. So it's very important to keep people motivated, those human in the loops, to actually be there and contribute.
Olga Beregovaya [00:16:08]: And I mean, first of all, I mean, it's a combination of, it's fairly easy money to be made on the side. But also I think what we are learning is essential to give the right tooling, if you give the right tooling to those people who perform the human in the loop tasks, if it's easy to rank prompts, if it's easy to assess the output, if it's easy work surface, that's usually what keeps people motivated, because they feel that they are productive, they feel that they're doing something interesting, and they feel that they're given the right tooling to do their job.
Ashley Antonides [00:16:38]: So I guess for us, depending on the project, we usually go through kind of three different options for data curation. One being do we hire somebody into the company? That's usually not the course that we go, because then it sort of questions around what is the growth path for this person. Maybe it's something where they come in as a data curator or labeler, and then there's an opportunity to grow into more of an ML engineer role. We've talked about, not in my current company, but I've been at companies before where we actually have almost like an internal crowdsourcing system, which maybe isn't totally scalable, but it is kind of. I do like when the ML team has an opportunity to label, just to actually go through some of those edge cases to put together the guidelines and then think about, okay, is this now at a point where we need to scale this up and pass it out to an external labeling company or partner? And I know there are challenges with that, too. There was a great panel this morning on data quality and labeling and all those challenges, but those are typically like the three courses of action that we, or kind of courses that we look at.
Olga Beregovaya [00:17:42]: Just on the subject of tooling, I wanted to comment, I don't know if we have any Kalina folks here. I'm a huge fan of the product, and I swear I'm not, like, I'm not affiliated with a company in any form or shape, but when it comes to model evaluation, I think that highly recommended. Okay.
Shailvi Wakhlu [00:17:59]: Yeah. And I think back to the earlier point, like exposure, the more exposure that people on your team have at conferences to other tools or to new, newer technologies that people are using. Like, it's really great when people come back to the company and say, hey, there's all this other stuff that we have out there that can not just make our life better, but it'll be more accurate. It's better for our product, it's better for our customers. So it's great to be able to identify those pieces. Yeah. I guess one thing that's on everybody's mind, whoever came to this session, where do you actually source talent from?
Olga Beregovaya [00:18:38]: Well, I mean, we might be saying the same thing.
Ashley Antonides [00:18:42]: I mean, so I think we're laughing because it is a challenge. So, I mean, I think we'll see. There's always the sort of first tier of, you know, who's in my network or the network of the people, like within the group or within the company. I think that second to that is like, we develop a lot of partnerships with universities, go to conferences, that sort of thing. So kind of building over time that broader network of partners who may also have students or folks who are, who want to come in and then sort of scaling from that, too. Like, we have worked with both with our sort of HR departments and also with maybe recruiters or head hunters, too, which is maybe a little bit more hit or miss, too. But that is one way of scaling the approach. And I think there has just been, for me, most effective working with them to very clearly articulate what the requirements are because maybe they may not understand or kind of know, like, what the use case is.
Ashley Antonides [00:19:43]: So it takes maybe some iteration in that case. Yeah. But, yeah, I just want to acknowledge, like, it can be challenging, especially going back to sort of, like, diversity, too. So how do you, how do you recruit for that and find those pockets as well?
Olga Beregovaya [00:19:58]: Yeah, I agree. And also I think being present at conferences indeed matters, because if you, if your presentation, your company presentation is super interesting, and you are delivering some, like, you're sharing some interesting insights and you're sharing whatever the latest, be it's research or be it a product feature. Usually those in the room who are looking for the next most interesting thing would react. And quite often we would be approached actually, like, hey, what you presented really resonated with me. I really want to. I really want to be a part of this project. Do you guys have anything interesting happening? Same usually applies actually to LinkedIn activity. If somebody, if the company is posting interesting content and the company leadership is posting interesting content, again, you get followers.
Olga Beregovaya [00:20:39]: Eventually those followers will graduate or will be looking for the next opportunity and they will come. So quite a bit of inbound. If you do enough outbound, I think that's what works. And internships always work magic. If somebody comes and works, looks for an internship. Actually, we just hired a couple of interns as ftes, as full time employees after the internship, so. But it is hard. I'm not going to say that, you know, you wake up in the morning and, hey, here is your senior data scientist with natural language processing background.
Olga Beregovaya [00:21:05]: No, not quite. But there are ways.
Shailvi Wakhlu [00:21:09]: Yeah, you know, so my last company, like, it was a consumer tech company, very popular. So I think that was the one time in my career that it felt very easy to hire because if people love the product, they will come to you. And I worked in other, like, most of the other companies I worked at were b, two b, and nobody wakes up thinking like, oh, I'm going to see if they have a job for me. So I like the idea of putting yourself out there. Maybe it's projects that even your consumers can work on or even somebody else can collaborate with. Those can become spaces where you identify the talent then comes. So once you find, you know, once you find that talent, this is an area that is facing very rapid changes. Like, there's a lot of stuff changing every day.
Shailvi Wakhlu [00:21:57]: How do you encourage your team to stay sharp on their skills? How do you sort of, you know, keep rejigging the team as needed with a changing environment? And how do you actually grow that talent?
Olga Beregovaya [00:22:08]: Maybe I'll start here. I really agree with Ashley, what you said about learning culture. I think it's super important to do internal webinars, internal knowledge sharing. Like, you know, I don't know if you guys are familiar. There's archive.org comma, which is like a big source of NLP research. Like, you would just divide articles and just say, like, hey, such and such person will report on this, and such and such person will report on this and let's brainstorm. And then always, since we're all in the field of dynamic research, I think just making sure that people follow, you don't follow the next shiny object, but you definitely want to be appraised of the state of the art. And I think that again, if somebody's research topic is pretty cutting edge, the rest of the team will learn from them.
Olga Beregovaya [00:22:47]: So I think, again, it's almost impossible. I think many of us probably have been in the situation. In the previous conference I was speaking at, the submission deadline was a week, a month before the conference. I showed up with a flash stick five minutes before my presentation because everything I was producing was becoming obsolete every morning. So I think it's, I mean, that's just the fact of the matter. So you don't chase, but I think you put just the tracks of these are the topics we're going to be. We're going to be researching and making sure that somebody really goes in depth and can coach the rest of the team.
Ashley Antonides [00:23:21]: I mean, so, yeah, I definitely agree on kind of internal brown bags workshops, having internal reading group. One thing that has worked, or I found has worked pretty well across a couple different teams is we call it like a micro sabbatical where folks can say, here's like a topic that I really want to learn about. And I think it's going to be most effective for me to take a week or two to go research that and maybe the end product is a white paper or a prototype. At the very least, they're going to present back to the group and share what they learned. Sometimes that's more effective than trying to find a training class, especially for some of these newer topics. And it also gives them the actual hands on experience. So that's something that I found to be pretty effective across our teams.
Olga Beregovaya [00:24:08]: And maybe I could add to this. And also I think it's very important that the company is willing to invest and invest. I really mean dollars. I really mean dollars in terms of allocating certain budget for people to take courses, for people to get certification, to be able to expense their books if they decide to buy books. And I think also people stay motivated when they see that the company is really investing time and effort and actual money into their growth. I think that also helps.
Shailvi Wakhlu [00:24:35]: Yeah. And to your earlier point, like not just invest this for the data team, but like maybe across the company just to improve the education and culture related to the topic. So that, you know, even if it's like, hey, product managers, how do you engage with this topic. Designers, how do you engage with this topic?
Olga Beregovaya [00:24:52]: And nobody canceled the good old hackathons, so there is always that.
Shailvi Wakhlu [00:24:57]: Yeah, so I think you brought up the example of, as you submit new stuff, it goes out of fashion or something like that. So I think that's one of the components of how do you actually keep your team motivated? This landscape changes very fast. Companies are in almost sort of an AI arms war of sorts, where maybe you're working on something cool in your company and another company gets to it first. Like how do you actually keep talent motivated and excited about what they're building and the solutions that they're bringing forth outside?
Olga Beregovaya [00:25:31]: Well, again, I think, I mean, from my perspective, again, it's all about the outcomes. Like if the person developed a prototype and suddenly your automated scores are through the roof, I mean, that's very inspiring. Right? When you did something and you really see, hey, I really did something awesome, and you really see that the metrics, like you met all the metrics. That's one place where people really see the outcomes of what they do. And then I would also say, I mean, in my industry, a lot of it is about quality, language, quality. It's super arbitrary. But when the person sees that or the team sees that, their work really turn the dial, move the needle, turn whatever that word on the customer side and we see that our customer CSAT goes up customer satisfaction and the customer is able to meet their KPI's. That also keeps the team motivated because they did something good for the customer.
Olga Beregovaya [00:26:22]: It really has practical implications.
Ashley Antonides [00:26:27]: I agree. I think you mentioned earlier kind of the impact in addition to just the continuous learning, I think really seeing what the end value is, is really motivating. I know for us, again, coming from more of a research perspective, so often that means getting to the end of a project and then its transition to that customer and really seeing what the operational or the end use is for sure.
Shailvi Wakhlu [00:26:52]: Awesome. So I think this next topic, maybe it resonates with a lot of people, but how do you keep your team focused on the current projects? Because there's a lot of distractions, there's a lot of shiny objects in the horizon that people may chase after. How do you actually keep people focused?
Ashley Antonides [00:27:13]: So it is challenging, I guess maybe in some ways it's sort of redundant with what I've already said is like having those outlets for exploring new papers, being able to do some prototyping, some sabbatical, going to conferences so that there is space for that, and then also thinking about how to bring that back to the current work as well. So it's not so much, you know, someone's 100% on the current project and can't spend any time thinking about newer things. It's sort of a high level answer, but yeah. What do you think?
Olga Beregovaya [00:27:47]: I actually agree, I think to a great extent is I think it's Google, right. That has this one day for your personal project thing. I mean, you don't necessarily have the luxury to do it every day, but you can like somebody on our team. He loves to write like long esoteric treatise on different topics of natural language. Sure, do it if you want to do it one day a week. Right. Every dissertation you want. We'll listen, we'll talk, but then let's just make sure that the other four days of the week we actually are working towards teams goals.
Olga Beregovaya [00:28:14]: But it's extremely difficult. It's super hard to stay focused for us as leaders or for the team when there is a new model released every day or a new method released every day. So what do you do with this? People do get distracted. So I think it's really, again, setting the KPI's. Let's meet the KPI's and let's have fun outside of it.
Shailvi Wakhlu [00:28:30]: Keep the threshold for the KPI's and then you earn yourself some time off for the shiny projects. All right, so just to close this out, there's a lot of competition for niche skills. What are your go to strategies for retaining AI talent?
Olga Beregovaya [00:28:48]: Okay, I think I'll just repeat myself. I think it's really just, I mean, first of all, there, I mean, we're all humans, right? So there are all the perks, the compensation. Right. And all the things that are just native to. You have a job, you have a job. But I really think you retain the talent by keeping researchers and product managers and developers lives interesting. If there is enough to satisfy their intellectual curiosity, I think people stay that and culture. We all know that people don't leave companies, people leave their bosses quite often, right.
Olga Beregovaya [00:29:24]: And leave their teams. So it's essential to keep that there cannot be any us and them. There cannot be any competition. But that's on us as leaders to make sure that that's fostered.
Ashley Antonides [00:29:35]: I agree. And I think at this point, just reiterating what we've already discussed, I'm trying to think in terms of, yeah, really building that culture where everybody's supporting each other. I think just for folks to be able to see that they can continue to grow throughout their career. One thing I guess we haven't talked about is also, if folks do want to take some time to go get a degree or something like that, having support for that in the company, whether they need to take time off to get a full time degree or do something that's also part time, and they're also working at the same time. So I think it's just identifying those opportunities for continuous growth.
Shailvi Wakhlu [00:30:13]: As they say, culture eats strategy for breakfast. Awesome. Well, thank you both so much. This was wonderful. And I appreciate you both sharing your thoughts. And thank you to the audience for just sitting with us. Thank you.
Ashley Antonides [00:30:26]: Thank you.
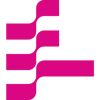