What is AI Quality?
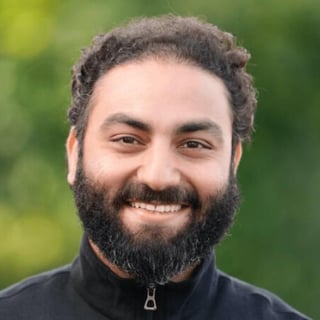
Mohamed is the Co-founder & CEO of Kolena and the author of the book “Deep Learning for Vision Systems”. Previously, he built and managed AI/ML organizations at Amazon, Twilio, Rakuten, and Synapse. Mohamed regularly speaks at AI conferences like Amazon's DevCon, O'Reilly's AI conference, and Google's I/O.
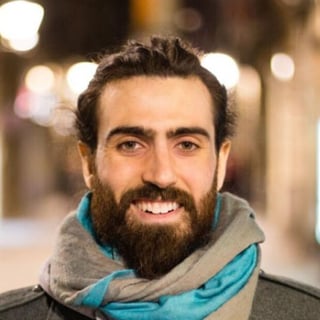
At the moment Demetrios is immersing himself in Machine Learning by interviewing experts from around the world in the weekly MLOps.community meetups. Demetrios is constantly learning and engaging in new activities to get uncomfortable and learn from his mistakes. He tries to bring creativity into every aspect of his life, whether that be analyzing the best paths forward, overcoming obstacles, or building lego houses with his daughter.
Delve into the multifaceted concept of AI Quality. Demetrios and Mo explore the idea that AI quality is dependent on the specific domain, equitable to the difference in desired qualities between a $1 pen and a $100 pen. Mo underscores the performance of a product being in sync with its intended functionality and the absence of unknown risks as the pillars of AI Quality. They emphasize the need for comprehensive quality checks and adaptability of standards to differing product traits. Issues affecting edge deployments like latency are also highlighted. A deep dive into the formation of gold standards for AI, the nuanced necessities for various use cases, and the paramount need for collaboration among AI builders, regulators, and infrastructure firms form the core of the discussion. Elgendy brings to light their ambitious AI Quality Conference, aiming to set tangible, effective, but innovation-friendly Quality standards for AI. The dialogue also accentuates the urgent need for diversification and representation in the tech industry, the variability of standards and regulations, and the pivotal role of testing in AI and machine learning. The episode concludes with an articulate portrayal of how enhanced testing can streamline the entire process of machine learning.
Join us at our first in-person conference on June 25 all about AI Quality: https://www.aiqualityconference.com
Mohamed Elgendy [00:00:00]: Hey, I'm Mohammed, CEO and founder of Kolena, and I'd like to drink my coffee black with two splenda.
Demetrios [00:00:07]: What is up, Mlobs community? We are back for another podcast. I am your host as usual, Demetri-os. And today we're talking to Mohammed, my man, my close friend, who I have been collaborating with a lot recently. We are doing this AI quality conference in San, France, Cisco, and he is the other co organizer, the other brain behind it. And I've got to say, I had to have him on here just because his vision for AI quality and what it means, what the industry is lacking right now when it comes to putting AI in production is so cool to see he breaks it down. I went deep on how you can look at testing, how you can look at quality, how you can look at frameworks. And one thing that I really appreciated from this conversation was how he broke down the gold standard that we're trying to create here with the conference. And after the conference has finished, we will continue to have working groups creating AI quality gold standards.
Demetrios [00:01:15]: And he breaks down what that means, what the working groups are going to look like, and we're launching it right now. If anybody is interested in joining one of these working groups, hit us up, because although there's still about two months until the conference happens, we're going to get cracking and the wheels are in motion. So last but not least, I mean, he dropped so many gems on me that I really want to say everything, but I don't want to spoil anything. So let me see if I can be vague enough to not kill it for you when you hear him. But his idea of making sure there are standards, that for each industry you have standards, and then inside of each industry you have, for each application you have these gold standards. And what these gold standards look like is what I really enjoyed because you can easily throw around the word standards. And I actually grilled him on this when it comes to how are we going to make sure if we create standards, it doesn't just become another meme like the, oh yeah, we had twelve standards yesterday and now we have 13 standards today. That comic that I'm sure everyone who has thought about creating standards has faced.
Demetrios [00:02:28]: And I thought his answer to that was insightful and brilliant. And so this idea around industry and applications inside of the industry and each of those having their own quality standards was so cool to see. I really enjoy the way that he thinks of things and how he's moving the ball forward in this domain. Hope to see you in San Francisco, June 25. If you can't make it, hit us up. We would love to chat with you all about these AI quality metric standards testing. You name it, we got it. And as always, if you like this, share it with a friend.
Demetrios [00:03:06]: Because you know, the world needs more AI quality. And especially those big businesses that are losing money every time they put out one of those shitty chatbots. Alright, see ya, dude. Bo, we gotta start with the question that I know everyone wants to hear. In your eyes, what does AI quality mean?
Mohamed Elgendy [00:03:35]: What is AI quality? So that's the question of the hour. Everybody's asking this question. We talk to hundreds of customers all the time. And then the question is, and even when we talk about more details about let's build quality standards, people are asking, what does that mean? Let me actually tell you a good story here. And then we jump into it. So if you give context for everybody as well, we at Colin, we're building AI testing and quality validation tools. So we've been working with enterprises, enterprise customers and leading startups, building on top of gene AI or even robotics. If we're talking about what became old AI now, we always think about, ok, what is this? What can we do more? We are a testing solution.
Mohamed Elgendy [00:04:21]: And we see our customers testing rigorously testing their models, and finding exactly where the failure points look like. But we still feel, we have felt that something is missing. Something in their testing process, or their validation, or they seek for high quality product. Something is missing there. So we were having one of our internal product design sessions, and then our CTO and founder, Andrew, she shared a really good insight. Like he asked a question or threw a statement on the table, and they just kind, kind of change our perspective. He mentioned, he said, we are providing testing to our customers, we're not providing quality. And that had us start thinking about the question that you just asked, what is quality? So we started thinking about, okay, what is quality? And how we can define that and actually help our customers achieve, because that's the end goal.
Mohamed Elgendy [00:05:15]: Testing is a step in the quality process towards that quality goal. So we started zooming out and thinking about, what is AI quality in our case? And then the quality here is dependent on the application and the domain that this application is being used, or the problem that's being solved. To give you an example here, is just outside the AI site and we come back to it. If we're thinking about like this pen here, it's a $1 pen. And is this $1 pen better quality, or would this pass the quality bar more than a $100 pen. Now this is a quality question. Not has nothing to do with AI, but it's a manufacturing quality question. And they had this question asked the same question, what is quality 100 years ago when they are building these standards? So the answer here is this $1 pen can pass the quality bar and $100 pen not pass its own quality bar.
Mohamed Elgendy [00:06:12]: And this is, to answer your question, there are different quality party standards based on the application that you are using it for. So the $1 pen here is not expected to perform under severe weather conditions, for example, it's not expected to live for years. It's expected just to be disposable, use it for a couple of months and then throw it away. Now, having that context defined, let's think about Genai. For example, if you're building a chatbot that will be used in twitch for gaming industry. Now, the quality standards for that is completely different than the quality standards from a chatbot used in a fintech company or in a bank where you are prohibited by law to give a financial advice. And even if you're allowed to give a financial advice, you have to be very precise. You can't say three ish.
Mohamed Elgendy [00:07:02]: The price value is going to be three ish. It's always going to go higher. You have to be very precise in timing. And actually, what is that change that expected. So with that, the gold standards or quality standards for the AI product. Now, in our definition, it means it's built for specs, for the specifications that is meant for and deployed safely. So that's the whole thing, like hallucinations and all the issues and risks that come with it. These are all called risks, like NIST, the National Institute of Standards and Technology in the US, they define that as risk management framework.
Mohamed Elgendy [00:07:39]: So, if I am a data scientist and I'm thinking about what is AI quality for my product, it is. There is two main sections. One is what are the specifications, what is it intended to do, and what are the risks that are associated in your industry. Then you're able to benchmark for testing models or your products based on that. So we ask a lot of, then I will give you the short answer. What is AI quality? We ask a lot of leaders in the data science and AI space, and then they tell us in name and terms, which actually makes a lot of sense, the quality means that my product performs as intended, safely, that no risks associated with it that we don't know about. That is the thought that happened to us to start thinking for us to deliver quality to our customers and to the industry. We need to define these gold standards for each domain.
Demetrios [00:08:36]: Brilliant. There's so much to unpack there, man. Especially, I really like this idea of the pen. And you have two separate pens. One maybe has diamonds on it, the other doesn't. And you have two separate quality standards that when each one of these comes off of the assembly line, they're going to be inspected and they're going to pass the quality check. If they, or if they can pass the quality check, that doesn't mean that the $1 pen is going to be able to pass the quality check of the diamond laced pen, right?
Mohamed Elgendy [00:09:11]: Absolutely, yes. So, and then if it's diamond laced, you have your, your quality requirements will include the diamond quality requirements too, because it's the quality requirements for your product.
Demetrios [00:09:20]: Yeah, exactly. So, so there are many different layers of that quality to make sure that it's not only the actual ML, but then you have the software and there's that tried and true practice of quality assurance and then you've got the actual product. Yeah.
Mohamed Elgendy [00:09:36]: If it's sitting, let's say if you're deploying on edge, then latency is part of your quad requirement. I mean, if not. But in terms of latency, it's in fact much more if you're deploying on edge on some hardware. So you're thinking about the specs of the hardware should be that high or that good. So it gives you a response within half a second or 500 milliseconds versus a couple seconds if you're doing on cloud, for example. So that could be part of your quality requirements in the on edge deployment or on time sensitive applications. Depends on how we start thinking about breaking down these standards.
Demetrios [00:10:10]: Well, you mentioned gold standards and knowing that each one of these specific use cases feels like it has a new set of gold standards. How do you go about creating that? Because if I'm thinking about it, I'm like, okay, cool, the financial advisor chat bot. It's never going to say that it's giving financial advice, but that's one lane of gold standards. I feel like you have to really curate and create gold standards for that use case. Is it like you need gold standards for each specific use case or can you generalize the gold standards, like break down what exactly these gold standards look like?
Mohamed Elgendy [00:10:55]: Yeah. So it will not be too detailed to the use case level. You can generalize that more. That provides the same details, but it doesn't have to include the overwhelming work of defining each specific use case. So it would be for the domain like, let's say fintech or banking. And then within the domain you have to fork out for what kind of application it's been used in. It could be in banking, but still it's a customer support kind of banking application or in banking as well in financial services. So if you are seeking, let's say fast forward many months from now, a few months from now, and we have like a rigorous gold standard developed by the industry, you need to get into, okay, Genai standards and then double click into that and then get inside the financial sector or the banking industry and then get into that.
Mohamed Elgendy [00:11:48]: And then if you're building a customer support bot, you will see it there. If you get to see what are the functional requirements that you should be thinking about and the acceptable limit. So we talk about latest, you just talked about it now then what is the acceptable limit here for a bot that is in customer support? Maybe it's fine to give you an answer in five or six or 7 seconds, and then you can add a spinner or something that for UX and then that's one thing. And then you go back to the financial sector again, up and then go down into financial advice or financial advisor. Now you have a different set of requirements here that you need to think about. And they always would be thinking about this for months. And then they are almost always fall under one of two categories. One, the functional requirements, which is what you're tempting your users to use, which is the use cases that he talked about.
Mohamed Elgendy [00:12:42]: And the other ones are the risks associated with the technology that you're using. The technology that you're using here in this case is Genii. Now, what are the risks? We all say hallucinations. What are these hallucinations? Right now if you have hallucinations that could be here, like we said, if you're asking a question and it's not part of your reg system, you don't have the answers for it. It can confidently give you a wrong answer. That is the known type of hallucination. But another type of hallucination is jailbreaking. So it gets outside the guardrails that was built around that.
Mohamed Elgendy [00:13:14]: It was built around like actual builders of the application. Like don't give financial advice, or you can give financial advice, preempting it with some kind of a caveat. Based on my understanding of the industry and analysis, here is what I can tell you as an automated bot. Like there are some regulations here. This is when we want to work with regulatory bodies as well to tell us how we can bridge this gap. These standards have to be defined for the industry and for the type of application. And then now you get to say, okay, the acceptable score, because now with everything you said, you need to figure out the metric for it and then how to test it. And what's the acceptable score? Because you get some scores at the end and you don't know what is that score for? You don't know if that's good or not.
Mohamed Elgendy [00:14:00]: So based on the acceptable, here are the acceptable limits, from 70% to 90% accuracy in these things, based, again, on the application that is being developed.
Demetrios [00:14:10]: So it feels to me like, and I appreciate the idea of industry and application because it feels to me like you have a certain baseline that you're probably going to see across the majority of the applications and industries. So if you have a chatbot and a financial use case, it's probably going to need a lot of the same stuff that a chatbot in the insurance use case needs. Or if it's a customer support in finance, it's probably very useful to have customer support for like a telephone company, right?
Mohamed Elgendy [00:14:46]: Absolutely.
Demetrios [00:14:47]: Then you, depending on each one of these specific verticals and each one of these industries, you're bolting on specificity, I would imagine. So what is wild to me is how you need to fan out and think about all the different use cases and then all the specific risks that are inherent with these use cases and in these industries.
Mohamed Elgendy [00:15:13]: Yes. So it is a huge undertaking here, and it doesn't take one person or one company or one institute to go ahead and build this whole thing. And that's why that we are working together, Golena and MLOps community, in hosting the AI quality conference and the AI quality conference. We're thinking about it here as a. We've tried to find another word other than conference, but this is the kickoff of this AI quality movement. We are putting together a nine months program to build these quality standards and to be able, from our understanding, and we believe that the best way to build a applicable and safe gold standard for quality, you need three main players to be in the room. The builders, the AI builders, we understand that. And regulatory bodies obviously there, and the Mlops, or the tooling and infrastructure layers.
Mohamed Elgendy [00:16:11]: I seem like it's a quality, you know, triangle that these are the three diagonals.
Demetrios [00:16:15]: Trifecta.
Mohamed Elgendy [00:16:16]: The trifecta. There you go. Because these are the ML ops toolings or the infrastructure companies. These are the people who are going to make it feasible, automatable, because the builders cannot be stalled down or slowed down by a lot of governance work or providing a trace to their data and their models, everything that's required. But for that to be implementable, and actually the AI builders adopt this technology, it needs to be, or these regulations, it needs to be automated in a very supporting fashion to the data scientists. They don't need to think about what's happening on the backend. We're bringing these three players together in what we call the AI quality conference, AI Q Con. It's happening in San Francisco on June 25.
Mohamed Elgendy [00:17:06]: And in there we are. This is the kickoff. And then we bring in industry leaders, regulatory bodies, and obviously infrastructure companies and teams. And we are putting together a nine months program. This nine months program has three main phases. The first phase is the initial phase, like you're getting to understand, okay, discovery. What are the domains that we're thinking about? And we initially thought about three main domains, the robotics, robotics, we talked about. We now it's called old AI, but the robotics domain, think about all the robots, including autonomous vehicles that are out there being deployed in front of us that we started seeing them.
Mohamed Elgendy [00:17:50]: So that's that number one domain that we're focusing on. And for Genai specifically, we're focusing on e commerce and the financial sectors, both of them are. This is where we see the highest adoption for Genaii, and we're working on defining goal standards for those. So that's phase number one, the discovery phase, and we will have three months for that. And then the second phase is we want to define the risks and the application guidelines, the specification guidelines. So, for each of these domains to be able to allow the provide some kind of portal where people go ahead and select their domains, like we mentioned, and then zoom into really quickly what is required from them, what are the risks expected? And this has to be something that is always updated with the updates. And now this is still in recent stage now. So this is growing with every other releases that we see every day.
Mohamed Elgendy [00:18:41]: So, second stage is defining these risks and the specification guidelines and the applicable or the acceptable scores or guidelines for these. Now, last thing is the third stage, which is the last three months, we want to define the processes, the tools, the infrastructure, make something that is applicable. We want to see industry leaders being able to just take this and give them to their teams and say, okay, start building against that. That will be a great stepping stone for regulatory bodies to start thinking of a practical way to enforce these regulations without stifling innovation for the builders.
Demetrios [00:19:20]: Yeah, this is cool because it is something tangible that comes out of a fun party that we're having.
Mohamed Elgendy [00:19:28]: Exactly. Exactly.
Demetrios [00:19:30]: We're going to have a great time. And nine months later, there's going to be a baby that's born. It's not the baby that you would think is being born.
Mohamed Elgendy [00:19:39]: I just noticed the analogy here. I didn't think about it, that it's actually. Yeah, it's exactly nine months and a new baby was born. That's a great analogy here.
Demetrios [00:19:47]: There we go. And I really like that because the ability for us to be able to say, all right, we're going to understand deeply from the subject matter experts, from the builders, from the people that are creating the tooling for this to all try and work together and make this whole trajectory a whole hell of a lot easier, then that tangible thing that comes out in nine months is going to be so valuable for the community. Yes.
Mohamed Elgendy [00:20:18]: And so that's the goal. Right? We have been. We see regulatory bodies are trying to protect the people, us. Right. We are the builders, but we are the people to be protected. So they are rightfully doing what they are trying to do there. But again, they are the AI leaders or industry, the builders. They have the right to think about.
Mohamed Elgendy [00:20:40]: Okay, there's next to this tension. I see it as a healthy tension. Right. That's trick that's needed in these kind of conversations. And that's why I see that the infrastructure teams are within organizations or companies. They are. I believe this is the magic stick here. Not necessarily like they're going to magically make it happen, but more importantly, okay, how can we use engineering to make sure that this is done safely without stifling innovation? And this way we are able to unlock this bottleneck where everybody's standing in.
Mohamed Elgendy [00:21:13]: Okay, we want to regulate you and don't regulate us. So in the AI quality conference, we focus on bringing for when we're thinking about the leaders, the builders, we focus on bringing the actual people who are going, influencing their teams. You can always get speakers, which they are great conferences to bring speakers. But in this specific conference, we brought the heads of AI's or the ctos of these companies that are coming part of a mission, coming to kick off this mission with all of us. And everybody has 30 minutes, 45 minutes. That's not enough to share how they think about quality, but it's great to start here is as a kickoff, here's me as a company, how we're thinking about quasi standards. Here's so on. Regulatory bodies are coming to us.
Mohamed Elgendy [00:22:02]: So on the industry side, we're bringing Mol Shinawi, for example. Right? Mol Shinawi here is the CTO and president of cruise. He's the man in charge of bringing this technology out to the streets for us. So he will be sharing Cruise's end to end AI philosophy and how they are thinking about building new quality standards for autonomous vehicles. On the other. That's for the autonomous. On the other end, on the LM side, we have Richard Socher. He's a Stanford researcher and CEO of you.com, and he's sharing with us how they are building quality standards to build trust in lm based applications.
Mohamed Elgendy [00:22:41]: Now, speaking of regulatory bodies and the government, we have a government panel that's moderated by the Washington Post. They are hosting NIST, they are hosting the DoD, the representatives from the White House who wrote the executive order. We also are bringing on a VC panel that is moderated by the information reporter. They are bringing in VC's who are investing in tons of AI companies and applications to think about, okay, where. How we can put our, and deploy our resources and our funds to promote this AI quality movement. So many industry leaders are coming. Like we talked about Kodiak robotics, torque robotics. That's an autonomous track.
Mohamed Elgendy [00:23:28]: On the LN track origin, AI, there's OpenAI, coherent topic and many more. So there's tons of conversations that we. The goal of these conversations, every leader is coming to say, here's how we think about AI quality and here's we can work together to define these gold standards.
Demetrios [00:23:43]: I just gotta say, I am so excited to wear my I hallucinate more than chat GPT shirt with a bunch of these government folks that are making regulations. We're gonna see how that goes over and maybe some of them. We're gonna. We'll bring some and I'll give some to the government. I see that.
Mohamed Elgendy [00:24:01]: Yeah, I'm excited for this one. I'll be ready by the end of it.
Demetrios [00:24:05]: Everybody's gonna be wearing that shirt and it'll be a good time. It's a big step up for us, man. Like, let's be honest, having a panel moderated by the Washington Post, for me, I never would have thought four years ago that would be happening with the mlops community stuff. So it's brilliant to see that. I also kind of wanted to dig into this idea of, we talked four years ago, and you, you were one of the first guys on the podcast back in the day, and you were talking about this. It feels like you've learned so much in the past four years because you've been out there, you've been talking with people, you've been recognizing like what people are saying as far as the quality standards, how they need to go about it. And the first thing that comes to my mind is that with this nine month project, and we're really going to try and create these gold standards, right. How do we not become the meme of, oh, there's 13 standards but none of them work.
Demetrios [00:25:15]: We should make a standard that encompasses all of these. And then next thing you know, there's now 14 standards.
Mohamed Elgendy [00:25:23]: Yeah, I mean, that's a very good question. We started thinking about this, given that everybody's thinking about gold standards differently and it's a challenge that we will go through and to say upfront, it's an evolving process. So there will be, let's define standards first. So if standards are list of like the way we envision what the standards would look like, it will be the list of functional specs, what's intended to do and potential risks for this domain now comes with it, the methodology of testing. So for each one, it has different logic to test. If you're testing PII, data leak is different than testing for correctness, correctness or fractionless of the results, right. So it has different methodology to test. So we talked about the functional requirements and risks, how, what's the methodology to test? What are the metrics that you're going to use and what are the acceptable guidelines? Now having these four, that is the layout.
Mohamed Elgendy [00:26:25]: That is the gold standard of the gold standard, if you will. Now people start thinking about, okay, I'm going to create different gold standards using this layout, this framework of thinking, right? So that is the framework. So that's one point we get there. We're already winning as a community because we're talking the same language. So now we're just agreeing or disagreeing on probably the acceptable ranges. This is where things are going to be left to that provider. Or maybe what are the metrics? This is evolving. But as long as we agree on, okay, do you have to define the risks, the methodology to test? And that's what so we have seen when we started, like you said, three, four years ago, when we started talking, we had the conversation, you and I, we believe when we started, Genai wasn't out there and we were looking, obviously we believe in AI testing and quality for AI in general.
Mohamed Elgendy [00:27:14]: But when we looked at who is doing this to, we were the first ones to start thinking about it and it felt like, okay, this could be something wrong, right? Like, okay, maybe we're working on something that is not important or is not feasible. You know, you understand this, when you're working on a new startup or thinking about something. But that was that concern in our hearts when we started launching a company. Just went away in the first couple of weeks talking to customers and before Genei, the amount of traction and that we got from customers like, yes, we want testing. And the biggest thing we heard, that's an old AI. Oh, Mohammed, I thought we were doing it right now. I'm rethinking. And that has been a challenge in early stages when we are in our sales or go to market motion, that when you message Demetrius, say, man, you're a great data scientist or ML engineer.
Mohamed Elgendy [00:28:06]: We are a rigorous testing platform. Always the answer is, oh, we have something, right? Because, yes, everybody has something. Nobody's pulling out a product. And we realized that, okay, the industry needs testing, needs rigorous testing to be automated, too, right, but needs education on what is that testing look like and why testing will save your effort. If you're thinking about effort on upstream processes like data labeling and training, you can just label only the data that you want. Saves 90% of your effort on labeling instead of turning the model, staying in the experimentation stage and trial error or, okay, am I improving the model in this or not? Now we exactly know where your models are failing exact scenarios, and then you go ahead and fix them. So it turns the experimentational nature of machine learning into an engineering discipline where you find bug and test it and so on, and you improve it and test it. So that was for what neural network is.
Mohamed Elgendy [00:29:05]: And predictive AI now came in Genai probably a year and a half ago or a year ago now they have been. Now the evaluation, it's called LM evaluations now, which is we like to think about as testing and quality more has become the, everybody's thinking about it, right? Which we're happy with this. Like, it's not about thinking about the competition. Business is big and the industry is, is evolving very fast, but it's more important, like, okay, there's awareness here. The awareness part is gone, but we found, okay, still some awareness is needed, at least the awareness in terms of testing is needed. Quality is needed. Now, the second level of awareness is, okay, what's the methodology? What are the, what's the quality framework? NIST likes to call it risk management framework. Europe called it the EU trustworthiness, I guess, act or something.
Mohamed Elgendy [00:29:56]: They use a term around trustworthiness, reliable or safe AI, something like this. So it is all, it all falls under quality. And things have been developing very fast since you and I talked three years but in the last twelve months, every month or so, like you said, we see a new development and new evolution in the technology, in the standards, in the regulations, and we're working on this, we're working on with everybody, trying to just make sure that everybody's working together and defining these for the better for the entire industry.
Demetrios [00:30:27]: You said something before that. I also wanted to dig in too, because you mentioned how testing is just one step along the way to quality.
Mohamed Elgendy [00:30:38]: Yeah, I mean, there are two main sections for this. One is test coverage and one is what's acceptable, right? So let's break it down a little bit. One is test coverage, right? When you are testing. When you're like software, right? When you're testing in software, you have to assert a lot of functions, which is, this is what unit testing would look like in software. But you know the logic, right? You know what you built it for. Testing in machine learning, or AI, is different. You're testing based on datasets. So when you are testing your gen model, here's a good example, actually outside lms there, let's say generative AI images.
Mohamed Elgendy [00:31:14]: Gemini. Gemini is a great example for here. And then exactly like you mentioned, things are good until they are not going to push.
Demetrios [00:31:23]: You, give it to the world.
Mohamed Elgendy [00:31:25]: So that is exactly, that's a great example of. Okay, how do we push out our first product with no embarrassing risks or even worse, no impactful risks, right? So that's the first. The first piece, and then obviously after that, how do you, that's the first product. How do you keep pushing out new releases and giving your customers the better product? You want to see regressions and you keep capturing detail.
Demetrios [00:31:51]: Yeah, because that's things too, right? Like you update and then all of a sudden it's way worse than the last version. I know that has happened plenty of times with GPT-3 and GPT four. It's like, wait a minute, are things worse now?
Mohamed Elgendy [00:32:04]: Yeah, it was good. So that's exactly the nature of testing today in AI jar, whether it's old or new AI, if we were to use these terms. So if you're thinking about Gemini now as a case, right, and then you're writing, usually the testing is done. The old school testing is you have some benchmark datasets of prompts or even data, and then you're just, let's say the benchmark is like a million data points, and then you're running your emphasis on your chain model, and then you come out with, okay, my model is 90% metric, right? F one score, recall, accuracy, hallucination, however it is.
Demetrios [00:32:44]: Right.
Mohamed Elgendy [00:32:45]: So now this 90% doesn't tell you what's in the coverage. In the test, you have a bunch of data. Usually you have some specs, you keep adding to it, but it's just a bucket of data that doesn't say exactly what slides are. And then the second point is the 90% metric. What does that mean? Is it better than 85%? We don't know. When you're setting up your benchmarks, it has to be. Or the way we think about it here at Polanda is the test case based evaluation, testing scenarios. You create your data, stratify your data, or into, or prompts into specific scenarios.
Mohamed Elgendy [00:33:22]: These are the reflect your functional requirements. So if we're talking about, let's say, the Gemini case, they had a great, noble goal, if you will. Right like the goal was, right. Like, hey, we want to diversify. We don't want this to have any bias in race or bias in gender. That is great. So that's the requirement. Now you need to the way to construct the test case, you have to add the test examples.
Mohamed Elgendy [00:33:44]: And we add that in our tool exam, saying, infrastructure teams are very crucial in this process because they can just build in these checks, data checks inside the platform. So if that goes on to Colonna, then the test case is to verify diversity and no bias. So the data checks should look into negative examples, too. So when you say, who are the founders of the United States? The quality check here should show that, okay, you need, for factualness, you need to deliver the right ethnicity. That's the critical part because it's historical event. So in that here, that hasn't been the test coverage. Hasn't tested that. And then I don't know, obviously, what's happening inside, how they are evaluating Gemini.
Mohamed Elgendy [00:34:35]: But after that, then you define, this is the test coverage. Okay, what am I testing? Guests? We said diversity. Then what does that diversity test case look like? Are we actually validating diversity in both ends? Right? Are we? Diversity and factualness should come together. So that's the test coverage. Breaking your data into test cases. These are test scenarios. And then.
Demetrios [00:34:55]: Wait, right, just hold on a sec. Because diversity and factualness come together. Those are two different data points, right? Correct. What are other data points that would need to come together? Like there you gave two data points, but potentially, what's another scenario where you have two different data points that you want to play nicely together that cover the test case?
Mohamed Elgendy [00:35:17]: So it. The factualness usually comes with a lot of stuff because you're trying to mitigate the risk, but you don't want to compromise factualness or correctness in your answers. So it comes in different applications. Like I'm trying to think something off the top of my head. Yeah, if you get twitch example, right, okay, who is the top scorer in this game? Right. This is a rack system. Just go post that from there. Now you don't need to up to this is factualist.
Mohamed Elgendy [00:35:46]: It has to be correct. You can't optimize for, okay, I'm going to say the top scorers, there are four. I have to put two male, two female, for example, right now in factualness. And this is how you design your test, that factualness should proceed in most cases, until I know something else, it should proceed other risks, like in this one, but should proceed the risk that PI daily proceeds factually. So now you start thinking about, okay, how they come together and that tooling can make this sense of data. Scientists should not be thinking about what are the gold standards out there? What are the evaluation criteria? I just need to share my problem, configure my functional requirements, and I see something populated based on my data. What, how are you going to test for the risks and how are we going to verify that my test for the functional requirements are correct. And then after that we have the metrics and then the acceptable score.
Mohamed Elgendy [00:36:41]: Because the acceptable score, if Gemini, for example, was not a public thing, maybe it's a fun tool that is just meant for to model how initially started the X Twitter tool, it just meant to be azure, then that could be unacceptable. And you have high tolerance for this. It's fine. You put the founding fibers with different ethnicity. So again, the acceptable standards here is the last thing. So once you have the quality, the test coverage, now you will start having conversation inside the organization. And this is where the quality centers should apply. Ask to mention that you have to put quality test coverage.
Mohamed Elgendy [00:37:18]: How are you testing your, your application on your data? Now these centers or these test cases are left for the teams to define them.
Demetrios [00:37:28]: And I've heard a lot of talk about model cards. What's your opinion on those? Is it kind of in the same vein as that?
Mohamed Elgendy [00:37:38]: Yeah, it is. It is an attempt from the industry to cover this gap, to close this gap with every model provider, whether we're not even talking about jihad neural networks, all providers, they share, whether it's a marketing effort or like a technical effort or academic, they share how my product is performing and that's the point of it. And that proves the point, that proves that they need it as providers and their customers or the other data scientists building applications. Now what they needed. So we need to know from vitris is building a model and building a new company, right. That's going to compete with OpenAI. Then I want to know, how do you think it's performing against OpenAI? Now, the missing part that we saw from the industry here, that it doesn't have the enough trust like that, the sufficient trust to start working on it. From the data sets that are being used, it's not clear what they are from the methodology that's being used, what it is from the metrics and that what's the acceptable.
Mohamed Elgendy [00:38:37]: So all these detail and obviously from the risk, everybody's thinking about risks from their own angle and they can put any, any results in their model cards, others. So we want to standardize that too, which is the same pillars that we mentioned before the test cases are the coverage, the metrics, the methodology and acceptable guidelines or acceptable thresholds. Now, once we set these as standards, then everybody should come in. I'm a provider, I'm OpenAI. I have GPT five coming out. I will benchmark it and I will create a model card that follows these gold standard principles.
Demetrios [00:39:12]: What I really like here is that once you have the test coverage out there and you understand how it's performing, you don't need only technical people in the room, you can have other people. And that's the beauty of it, right? That's where the subject matter experts can come through, or a diverse range of selected people can come through and look at the results and say, okay, have we thought about this as a test coverage? Kate?
Mohamed Elgendy [00:39:41]: Yes, that's music. Where, and then this is where you collectively start chasing that long tail of edge cases and in a systematic way like you, the long tail of edge cases. And not saying it's now just exponentially growing in risk with the genei capabilities, then the chasing that long tail is not something that you do in a notebook or something, or usually it's in the data scientist's head. Okay, we've seen these cases before, but now you have a systematic query and we've seen customers start with 1020 test cases. That's our understanding of our domain today. And then few months into testing with this approach, they have hundreds of these test cases. And now it becomes, okay, now I have more understanding of my own domain as a company. I collectively, and I love what you said here about everybody.
Mohamed Elgendy [00:40:30]: The product manager, who is voice of the customer comes in, even the customers collaborate and leadership, because when we start thinking about testing. That is the name we chose for our company, Kolina. It's an egyptian slang for all of us. And that's the point of it's like, okay. All of us are collectively working together, collaborating into chasing that long tail, dude.
Demetrios [00:40:51]: So you want to know? Speaking of egyptian slang, my favorite thing about the whole conference is that it made people always say that Mohammed is the most common name on earth. I was like, I don't know that many Muhammads at this conference. We've got three different mos. Talking true to form. We are perfect distribution.
Mohamed Elgendy [00:41:21]: This reflects, actually, the real sample here of the realization of the world, the population in the world. Yeah, it's obviously, it's just a coincidence that happened, but, yeah, this is something that we. Especially if you look at the. The name is very common. Right. And then we started seeing here, like, oh, okay. We need a representative from this organization. We need the leader of this organization.
Mohamed Elgendy [00:41:44]: Then it starts happening. My name is Mohamed, too, but it's fun. It's fun to have everybody there.
Demetrios [00:41:50]: Yeah, I know I've been kind of a pain in the ass the last couple months on trying to make sure that we have representation from diverse fields. And it is so cool to see this coming together right now. We've got so many incredible speakers, and I'm so excited because we're. I think we're almost hitting the goal that I set out with where I wanted to have 50% male, 50% female. I don't know if we fully hit it, but we're close, man, and that's really cool.
Mohamed Elgendy [00:42:26]: Yeah.
Demetrios [00:42:27]: For me to see, because I know that's really important. And having two daughters, you also have two daughters, too, right?
Mohamed Elgendy [00:42:33]: So, like a daughter and a son, but. Yes, exactly.
Demetrios [00:42:37]: You have a daughter. Having two daughters. You have a daughter. It makes. We're, like, trying to create the world so that hopefully.
Mohamed Elgendy [00:42:46]: Absolutely.
Demetrios [00:42:46]: If they ever want to get into this field, they don't feel that stigmatism where they can see others in the field doing what they're doing.
Mohamed Elgendy [00:42:55]: Yeah. And we talk about representation matters. Right? Like, representation matters. Obviously, it matters even more when you're trying to standardize the most important technology.
Demetrios [00:43:06]: You just sit around and it's a bunch of men between 25 and 45 talking about white males, talking about how we need more diversity. A new gold standard. Yeah, no, we're not going to do that.
Mohamed Elgendy [00:43:20]: Diversity here comes not just in the gender, gender, race, location, domain. We focused a lot, you know, that's very well. We focus on domain being represented. We focus on everybody coming as a leader and influencer in their own organization so that we can take actual actions with this. So everybody's coming, coming representing their own organization into this AI quality movement. It's going to be very exciting. I'm very excited about it.
Demetrios [00:43:46]: And of course, we're going to throw in a few of these curveballs from my side. We are going to have a little jam room. I think Joe Reese is going to be there and he's going to be able to dj. And we've got my buddy Michael Eric, who did a stand up set at the last virtual conference. He's going to be making fun of me and do a little stand up set here, too. So, yeah, I'm super excited, man. It's coming together. It is going to be a blast.
Demetrios [00:44:15]: Yeah. Excited for this?
Mohamed Elgendy [00:44:16]: Yeah. Get your tickets early, then. We're about to sold out probably by the next few weeks.
Demetrios [00:44:21]: Yep. And of course, like you said, there are tangible outcomes that we're shooting for. We've got working groups coming out of this, which is awesome to see, like these special interest groups, the working groups that anyone can join. So even if you are not able to make it in person, hit us up about the working groups, because that's how we're going to move the ball forward in the industry.
Mohamed Elgendy [00:44:47]: Yes, sir. Let's do it. All right, man.
Demetrios [00:44:50]: Well, it was great talking to you, and I'll see you soon enough. We. We sync like every other day, considering or. Yeah, we're getting speakers. We're doing all kinds of stuff for the conference, so, uh, yeah. But it's been good to have you here.
Mohamed Elgendy [00:45:05]: Scottish poet. Yeah. Let's put this together and want to hear from everybody who's watching this episode as we built for it. We have two months until the conference. Let us know your thoughts. Let us know if you're thinking about how we can collaborate. If you can't make it in person, let us know. Or if you can make it in person, great.
Mohamed Elgendy [00:45:21]: Let Demetrius and I know we can meet now and we start thinking this is a huge effort that requires a lot of people in the industry so that we can add to the EU act and the NIST regulations. We can provide something that is applicable and is actually something that provides safe AI. So let's work together as an industry for this.
Demetrios [00:45:42]: Love it, man. It's inspirational. Right onto it.
Mohamed Elgendy [00:45:45]: There we go.