How Distributed LightGBM Works

This article discusses a talk by James Lamb
January 31, 2022
This article discusses a talk by James Lamb. James is a Machine Learning Engineer at SpotHero, based in Chicago, IL. He is a LigthGBM maintainer and has led several large efforts to expand access to LightGBM, including publishing that project’s R package on CRAN and integrating ‘dask-lightgbm’ into the main ‘lightgbm’ Python package.
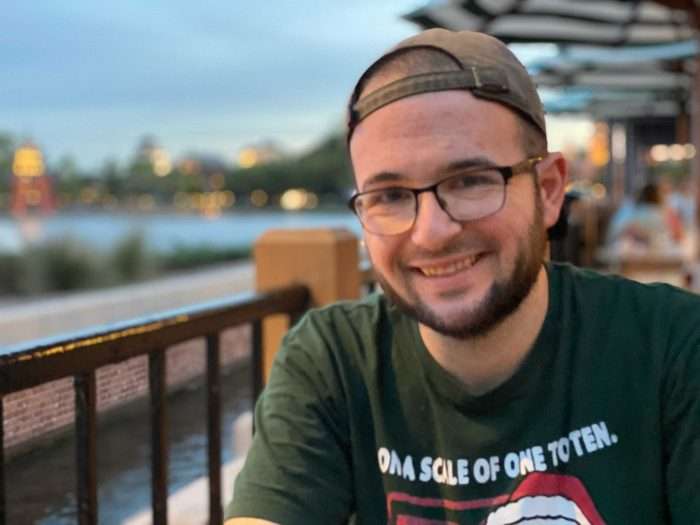
LightGBM is a framework ( documentation ) for supervised learning tasks (regression, classification, and ranking) on tabular data. People use it for tasks as varied as building search engines, detecting fraud, deciding whether or not to offer loans, predicting failures in industrial machinery, and forecasting demand.
James gave a great talk about LightGBM. In this talk, attendees will learn about LightGBM, a popular gradient boosting library from Microsoft. After a high-level overview of the LightGBM algorithm, the talk will describe strategies for distributed training of gradient boosted decision tree (GBDT) models generally, and distributed training of LightGBM models specifically. With this base established, the bulk of the talk will cover the current state of LightGBM’s Dask integration.
Attendees will learn the division of responsibilities between Dask and LightGBM’s existing distributed training framework, which is written in C++. The talk will also cover the specific components of the Dask ecosystem that LightGBM relies on. The talk offers details on distributed LightGBM training, and describes the main implementation of it using Dask. Attendees will learn which pieces of the Dask ecosystem LightGBM relies on, and what challenges LightGBM faces in using Dask to wrap existing distributed training code written in C++.
Links to talk: talks
Notebooks in talk: https://github.com/jameslamb/lightgbm-dask-testing/tree/main/notebooks
James’ previous MLops coffee talk on Building for Small Data Science Teams : https://www.youtube.com/watch?v=yAsPfhI5Jd8