How to tame your MLOps Mess with Run:ai
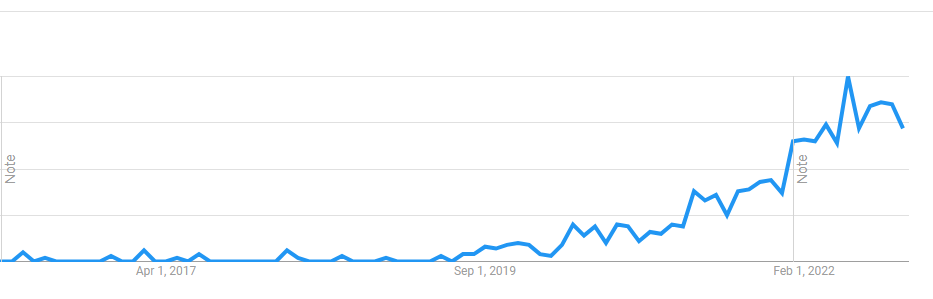
This blog was written in partnership with Run:ai
January 13, 2023

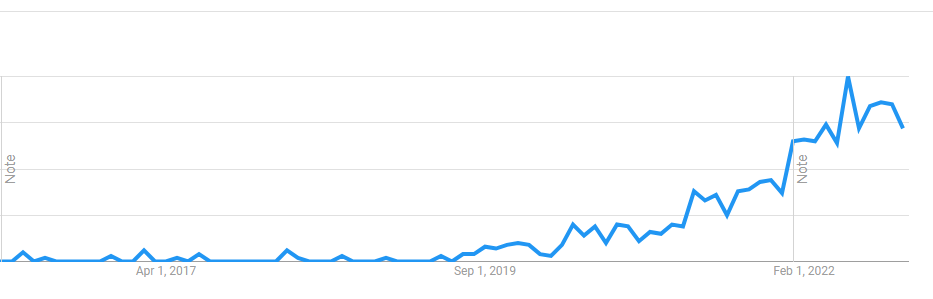
This blog was written in partnership with Run:ai.
MLOps – a term that only started to gain steam in 2019 – is big, and only getting bigger.
MLOps searches, source: Google Trends.
It feels like a new AI / ML marketscape diagram pops up every day, with more and more tools in it – now in the thousands. Here’s one example – the MAD landscape (Machine learning, Artificial intelligence, and Data):
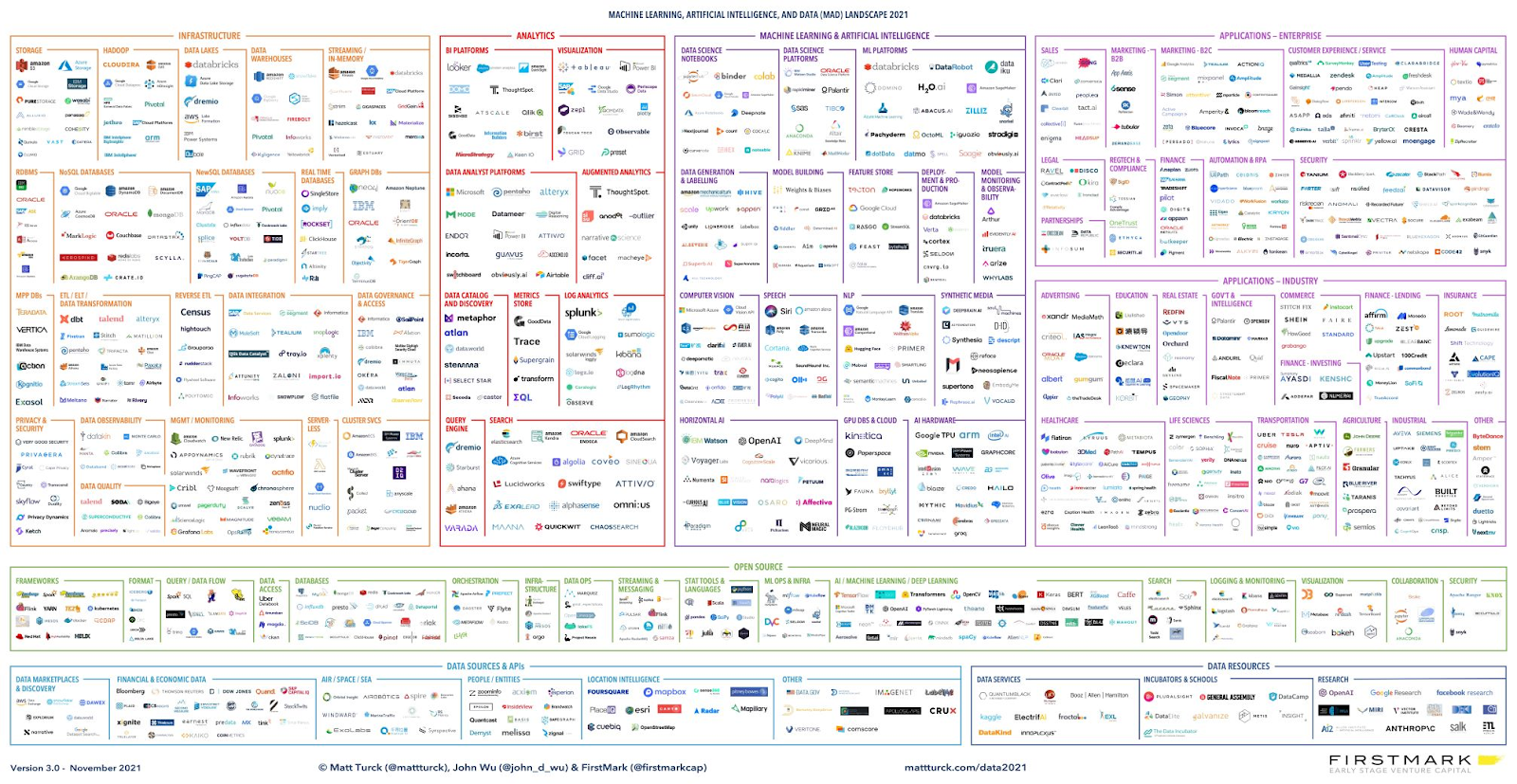
Have we reached “peak tooling” yet? Not at all. This trend is not stopping any time soon: globally and across industries, more organizations are starting to engage in AI/ML as part of their digital transformation efforts, and more startups are founded to serve their needs.
As Matt Turk of FirstMark (prominent AI/ML investor) eloquently said: “the fundamental trend is that every company is becoming not just a software company, but also a data company… This is the beginning of the era of the intelligent, automated enterprise – where company metrics are available in real time, mortgage applications get automatically processed, AI chatbots provide customer support 24/7, churn is predicted, cyber threats are detected in real time, supply chains automatically adjust to demand fluctuations, etc.”
More tools = more flexibility… and more headaches
The availability of so many tools, each catering to specific verticals, use cases, and even developer preferences, lead to individual data science and AI/ML teams choosing and running different tech stacks.
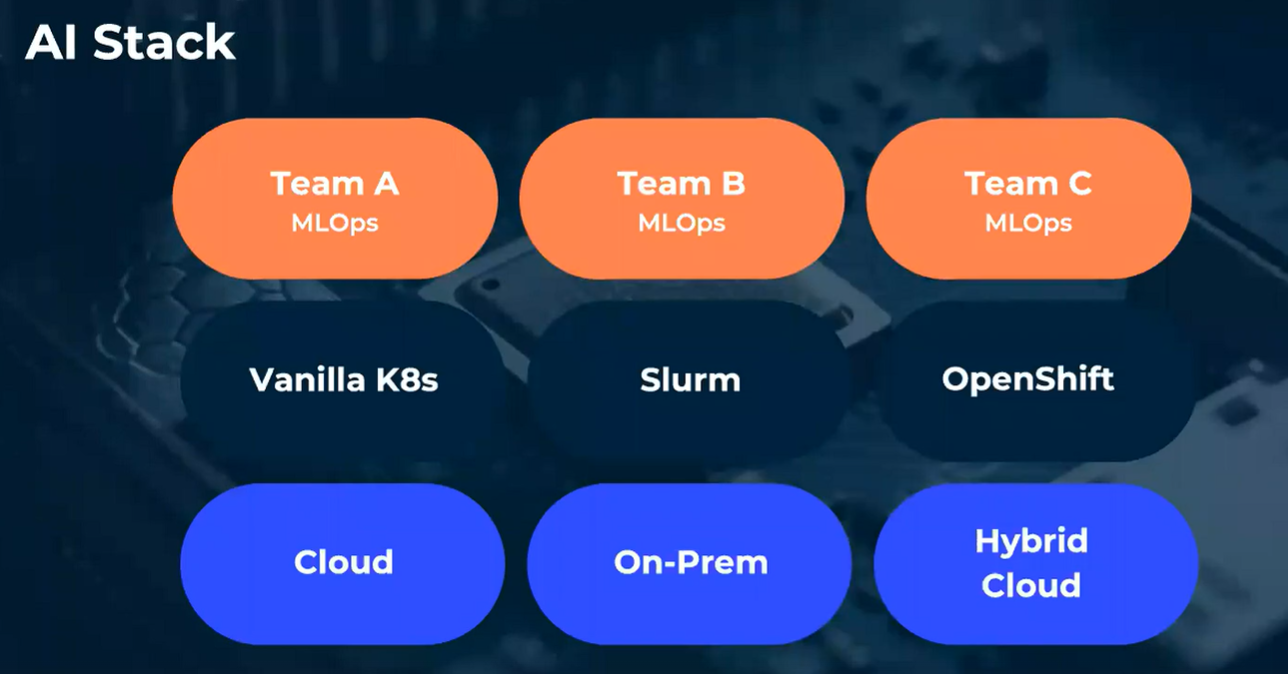
This phenomenon is known as Shadow AI – building AI infrastructure and tooling without IT’s input. While the individual teams are proud of their configuration in the short-term, Shadow AI presents significant long-term and strategic drawbacks to the organization. These include insufficient and inefficient use of GPU resources, lack of visibility, control, and access to compute resources, inability to align AI infrastructure to business goals, and added IT overhead.
Ultimately, stakeholders are unhappy across the board. IT has lots of overhead to manage, and unnecessary waste, and data scientists don’t want to manage this cacophony of tools, and deal with artificial resource limitations.
Interestingly, the major analyst firms and other industry pundits all struggle to define exactly what MLOps is and what tools belong to which layers in the MLOps stack. The conversation around MLOps focus on the categories above the water level in this iceberg analogy:
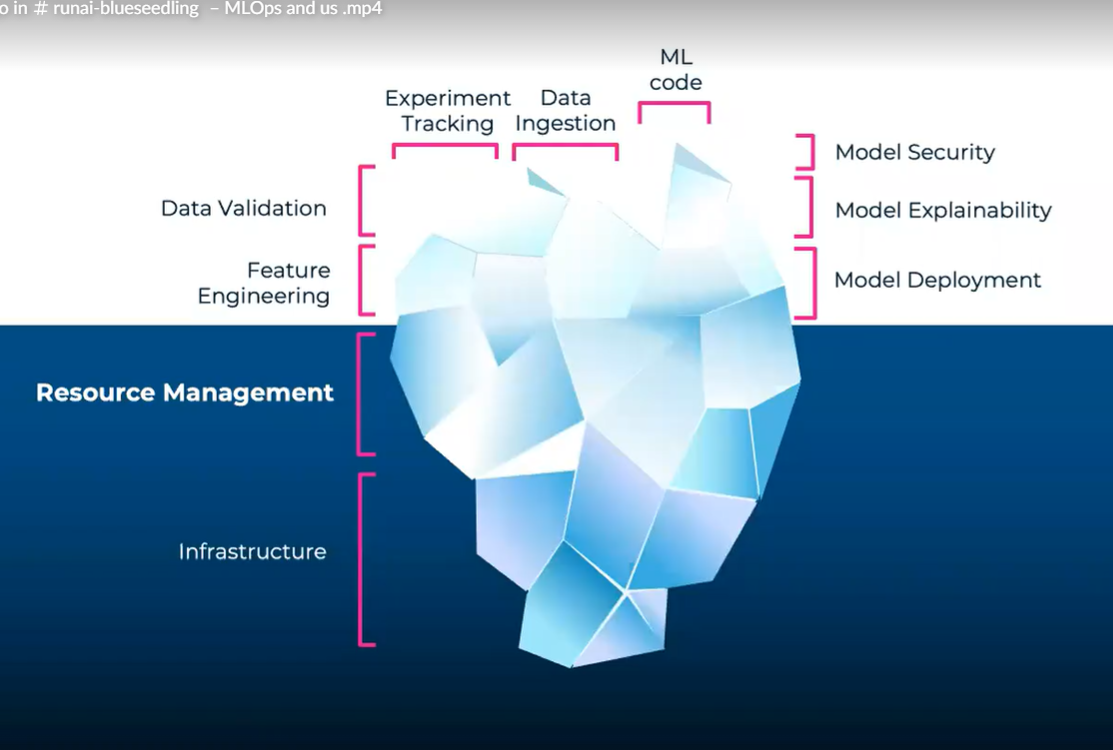
Infrastructure and Resource Management are taken as a given, but they’re actually a critical piece of the puzzle, even more so in the case of shadow AI and the proliferation of different stacks in the same organization.
Taming the MLOps beast: A unified layer
As we established, there’s not going to be one MLOps tool or stack, there’s no “one size fits all.” The solution is different: a unified layer that gives IT the control and ability to deliver a managed stack, while allowing individual teams the flexibility they crave, and the ability to use innovative new tools.
No matter which tools are in a company’s AI/ML tech stack, there’s a need for a solid resource management layer. This is where Run:ai fits in.
Run:ai Atlas: Unlock enterprise-grade MLOps workflows across clouds and squeeze up to 10x more from your GPU infrastructure
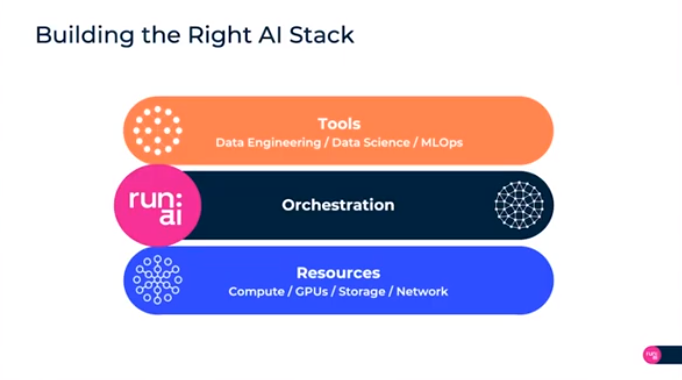
Run:ai Atlas is an MLOps infrastructure platform that helps organizations simplify and deliver faster on their AI journey from beginning to end. Using a multi- and hybrid cloud platform powered by a cloud native operating system, Atlas supports running your AI initiatives anywhere (on-premises, on the edge, in the cloud).
Run:ai Atlas provides tools that let AI/ML teams build, train, and deploy their models, while maintaining the flexibility to integrate any other open-source or 3rd party MLOps tool that runs on Kubernetes.
How Run:ai Atlas thrives in the MLOps jungle
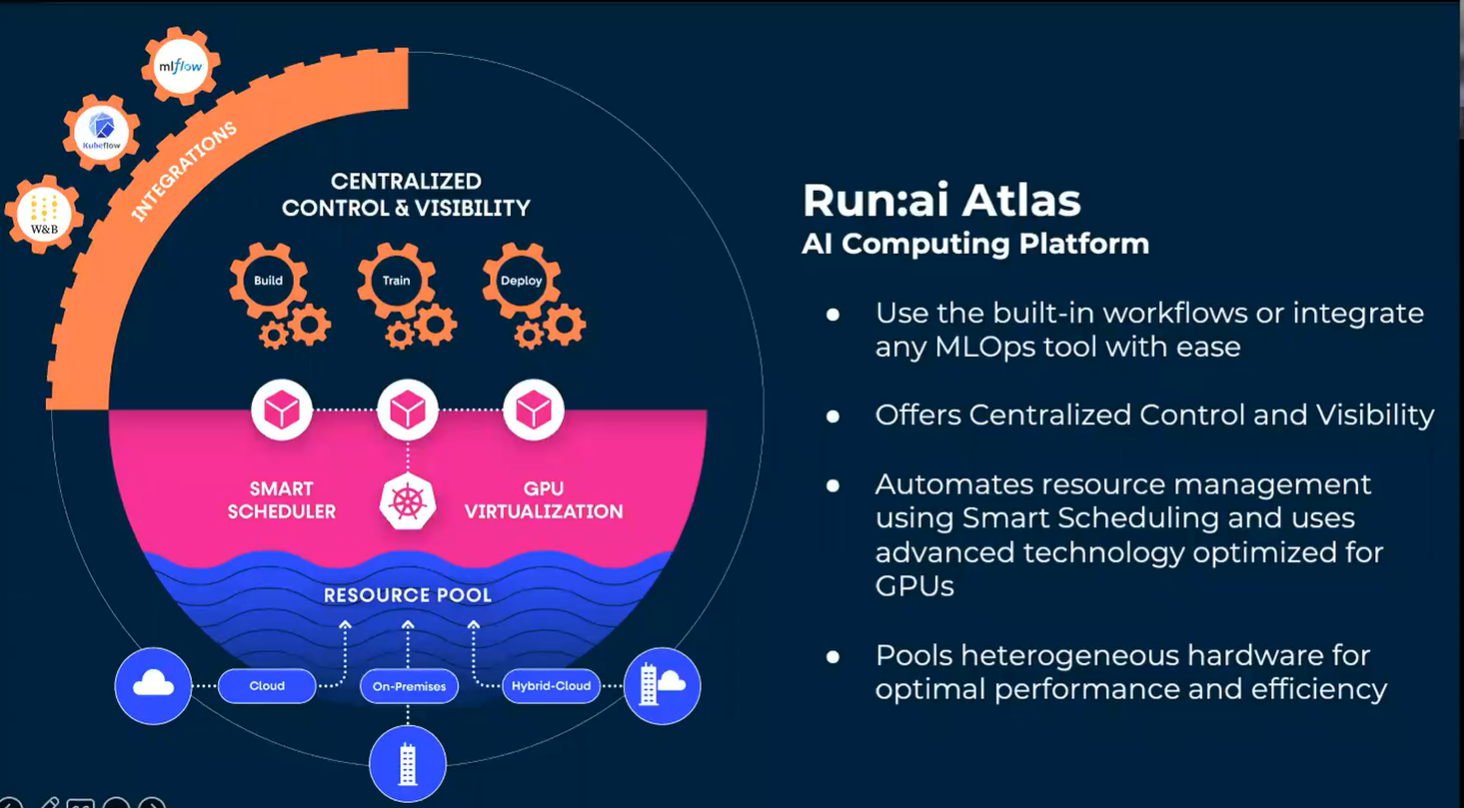
Run:AI Atlas helps you align your needs to the specific workload needs.Each AI/ML team can use its own MLOps tools of choice, while Run:ai functions as the orchestration layer. It pulls resources from different locations (cloud, on-premises, hybrid) into one resource pool. On top of it, Run:ai’s Scheduler automatically shares infrastructure resources between the tools, and allocates resources according to business policies and priorities. The scheduler is workload-aware, and takes into account the different needs of a Train vs Inference load, for example. And most importantly, it optimizes GPU usage and eliminates any idle GPUs.
The benefits of using Run:ai as your MLOps Orchestration Layer
Accelerate time-to-market for different AI models. Run:ai helps MLOps and AI Engineering teams to quickly operationalize AI pipelines at scale. It also allows them to run production machine learning models anywhere while using the built-in ML toolset or simply integrating their existing third-party toolset (MLflow, KubeFlow etc).
Unify visibility and unlock insights for AI infrastructure. Run:ai visualizes every aspect of the AI journey, from infrastructure to model performance, giving every user insights into the health and performance of AI workloads. These dashboards allow IT to have the visibility needed to set policies around which AI teams, users, stages, and projects get access to GPUs. This ensures that organizations remain focused on projects with higher business impact.
Increase efficiency and performance of AI infrastructure resources. Run:ai pools all compute resources and optimizes GPU allocation and performance. With Run:ai’s unique GPU abstraction capabilities, organizations can “virtualize” all available GPU resources and ensure that users can easily access GPU fractions, multiple GPUs, or clusters of GPUs which drastically reduces the percentage of idle GPUs and enables a wider range of access to compute resources.If you’re an organization struggling with shadow AI and MLOps, learn how to centralize your AI infrastructure with Run:ai today.