Agents of Innovation: AI-Powered Product Ideation with Synthetic Consumer Testing
speakers
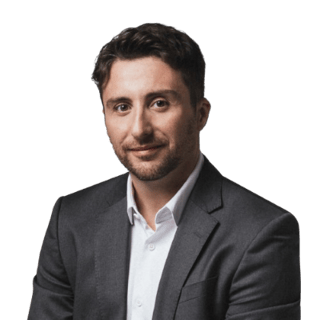
With over 15 years of leadership experience in AI, data science, and analytics, Luca has driven transformative growth in technology-first businesses. As Chief Data & AI Officer at Mistplay, he led the company’s revenue growth through AI-powered personalization and data-driven pricing. Prior to that, he held executive roles at global industry leaders such as HelloFresh ($8B), Stitch Fix ($1.2B) and Rocket Internet ($1B).
Luca's core competencies include machine learning, artificial intelligence, data mining, data engineering, and computer vision, which he has applied to various domains such as marketing, logistics, personalization, product, experimentation and pricing.
He is currently a partner at PyMC Labs, a leading data science consultancy, providing insights and guidance on applications of Bayesian and Causal Inference techniques and Generative AI to fortune 500 companies.
Luca holds a PhD in AI and Computer Vision from Heidelberg University and has more than 450 citations on his research work.
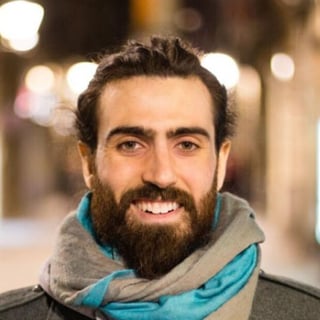
At the moment Demetrios is immersing himself in Machine Learning by interviewing experts from around the world in the weekly MLOps.community meetups. Demetrios is constantly learning and engaging in new activities to get uncomfortable and learn from his mistakes. He tries to bring creativity into every aspect of his life, whether that be analyzing the best paths forward, overcoming obstacles, or building lego houses with his daughter.
SUMMARY
Traditional product development cycles require extensive consumer research and market testing, resulting in lengthy development timelines and significant resource investment. We've transformed this process by building a distributed multi-agent system that enables parallel quantitative evaluation of hundreds of product concepts. Our system combines three key components: an Agentic innovation lab generating high-quality product concepts, synthetic consumer panels using fine-tuned foundational models validated against historical data, and an evaluation framework that correlates with real-world testing outcomes. We can talk about how this architecture enables rapid concept discovery and digital experimentation, delivering insights into product success probability before development begins. Through case studies and technical deep-dives, you'll learn how we built an AI powered innovation lab that compresses months of product development and testing into minutes - without sacrificing the accuracy of insights.
TRANSCRIPT
Luca Fiaschi [00:00:00]: Luca Fiaschi. I'm a partner at PyMC Labs. I take my coffee, I just drink espresso. I'm an Italian hardcore. Espresso is my way to go.
Demetrios [00:00:12]: Letters make words, words make sentences, and sentences make paragraphs. Welcome back to another mlops community podcast. We're getting into it today, talking about how GenAI can help the world of traditional ML. With Luca, we also go deep onto leading data teams at the end. Hope you enjoy.
Demetrios [00:02:01]: Hey, so this is like phone a friend. Basically I get to phone a friend. And man, you've been doing a lot of cool stuff in your career. We should probably just go over a bit of your journey at HelloFresh. HelloFresh, hello fresh and at Stick Stitch Fix. Both of those are not hard words to say unless you are me today. And so which one came first?
Luca Fiaschi [00:02:50]: Hello Fresh came first. And even before that I used to work with this large VC in Europe called Rocket Internet. It really moved me out of my academic track into the startup world. And Rocket was a lot of fun, built some of the largest E commerce in the world outside of the US and literally was empowering young people like me to solve big, big problem, putting a lot of money behind them and behind bold ideas. And there I got to work with companies like Zalando, Delivery Hero, lot of these, you know, like pretty huge names in Europe. Yeah. And I got to work with them when they were relatively small, like 20 people in the room and also when they became like these huge giants that they are today. So HelloFresh was one of the company of the RIP Rocket Internet Group and at that time the founder asked me to move to the US and build the data team for the US component of the business.
Luca Fiaschi [00:03:56]: It's one of the few rocket companies operating in the US as well. And when I arrived in the US it was like four people working in data. And when I Left I was 35. So within four years tenure there. It was a tremendous ride. It was also the years of the pandemic, so the business was booming and we did a lot of crazy and interesting stuff by implementing great data platform Great analytics processes, recommendation engines, forecasting models and so on and so forth.
Demetrios [00:04:28]: Yeah, the interesting thing about this is that the ML aspect of the business is integral to whether or not that business succeeds or fails. Because it is such a hard thing to do when you have fresh produce. And you need to know how much of this am I going to need tomorrow, how much am I going to need next week? And if you get that wrong, that can really damage the business. And if you consistently get it wrong, you don't have a business.
Luca Fiaschi [00:04:57]: Yeah, I can tell you stories of missed forecast and people having to run all fours around the area with a company credit card trying to buy as much lime as possible to fulfill our clients demand. It is really interesting where small mistakes in your prediction can have such a big impact on the company bottom line. And definitely the forecasting aspects@hellofresh was extremely interesting and complex to actually solve because the product has so many in the box. You ship so many ingredients, but think about Amazon as a complex business model, but you ship mostly items that are not perishable and in quantities that are kind of like pretty defined in like HelloFresh. Every, every box I remember every recipe contains on average every box contains three recipes. And every recipe has like seven, eight perishable items that you need to package accurately and ship to the, to the users.
Demetrios [00:06:01]: And then you add the recommender systems in with that. And so I imagine the recommender system chops that you had from there translated nicely to Stitch Fix because that's another similar thing. Now you don't have to worry about perishables as much, I guess because clothes only go out of style, they don't go bad.
Luca Fiaschi [00:06:24]: Right.
Demetrios [00:06:24]: And some clothes never go out of style. So if you're lucky or if you're just oblivious to fashion like I am, then you're good.
Luca Fiaschi [00:06:33]: That's right. I think Stitch Fix is an interesting different set of problems. Actually. Stitch Fix has a very interesting business model where when you ask where is your inventory? 50% of your inventory is most of the time at FedEx. It's always in transit between the user or the final customers and the fulfillment centers. And you want to keep it like that because you want to be as efficient as possible with the fulfillment space. And so Stitch Fix had this very interesting business model where you need to be very accurate also on your forecast to always have available inventory and relevant inventory to present to your customers. Otherwise you can make the best recommendation, but if the inventory is not there, you can fulfill it.
Luca Fiaschi [00:07:29]: And so there is this very tight and nice Interrelationship between the two. Sometimes the forecast or the recommendation engine can be super accurate, but the problem is that the items you retrieve are out of stock. So the interplay between the two is extremely interesting and complex.
Demetrios [00:07:48]: And that business model, you have gone down the rabbit hole. And I know that you've talked a lot about Bayesian theory and working with Bayesian algorithms. Can you tell me a bit more about that?
Luca Fiaschi [00:08:01]: Yeah. So Bayesian algorithms is something that I came to from the sideways, meaning I have a background in ML engineering and I have a background in things like, you know, traditional ML and deep learning and so on. However, a lot of problems that I try to solve is actually to convince stakeholders of the reliability of the forecasts we can putting out for them. And it's super complicated because the stakeholders ask you what's the logic on the model? What is the prediction interval for the model? What features are you based this on? And you can do that with a traditional ML. And there are techniques for doing these explanatory variables and so on, but they're intrinsically hard to explain. And the confidence interval you get out sometimes they're not well calibrated. And so Bayesian models is a solution for that because it gives you two things out of the box, which is interpretability and confidence intervals, which is the ability to add easily constraints to your forecast. For example, if you know that the output needs to be positive, you can constrain that very easily in a Bayesian models.
Luca Fiaschi [00:09:22]: So for things like high stakes scenario where you need to do like if Invest HelloFresh had spent $800 million in marketing budgets across 40, 50 media channels every year, or at least when I was there. So that's a high stakes scenario. So you really want to understand how the model works, what are the causal relationship between the variables, not just statistical relationships between the variable. And the Bayesian model is perfect for doing something like that. When you have talked to the finance stakeholders and you see it CMO and cfo, you can really motivate why you actually put up a certain forecast. And yeah, and therefore we solved those with those tools because they were the right tools to solve the specific problem.
Demetrios [00:10:17]: And you've kind of taken that and run with it because now you're still doing stuff with it. Right, Right.
Luca Fiaschi [00:10:24]: So that actually solved like what I want to solve right now. So we with the models, we build very sophisticated models for marketing allocations that through the years, you know, they've been published. Then companies uses PMC Labs has companies that has been developing the open Source library or supporting the open source library pmc, which is a statistical library that's very much used in the industry and is perfect for building these type of models. Now the key idea there is that in my career I always had these problems that you're over short of very, very smart people to hire. And the other problem is, especially when you're running analytics teams, what really kills you is the delivery of the insights. But it's all the follow up that comes from stakeholders. So how do you solve that specific problem? Augmenting your data analytics workflow and data scientist workflow with AI so the idea is that you can take these models and talk to them, chat to them using LLMs and basically you can do two things. One is to simplify the process of building these models by having a swarm of agents.
Luca Fiaschi [00:11:48]: Some are modelers that actually put together the relationship between the variable that you need and write the code that you need. Others are quality control agents that control the quality of your incoming data and these accelerate the production of these models and coming out with them. And the other one is once the model has been built, well, you can ask questions of make a what if scenario analysis. So if you make the specific forecast, what could be a certain scenario that you haven't thought about? Please right now answer to the questions. Hey, what happen if I drop my marketing budget? A hundred million dollars in Google Next day the CMO and individual process scope, it wasn't really there. This question, you thought about this afterwards? No problem, I can give you the answer right away without having to involve my analyst because I know how to talk to the model and I don't need specialized expertise to rerun that code again. So I think that to me it's a way of solving this very hard problem I always had in my careers that it's so complicated to scale up data teams effectively and really augment the workflow of your data scientist and analyst using these agentic AI technology.
Demetrios [00:13:14]: Yeah, I love this because you are combining the new world gen AI capabilities with the traditional ML capabilities and each one has their purpose and has their value. And so there's going to be times where you need to use traditional ML and so being able to augment the capabilities and augment your understanding of how this works and your benefits from them are, are such an incredible superpower to have. So if I'm understanding you correctly, you're saying something like on the front end when you're building the model, you're getting help from LLMs, correct. And are you also getting features that are being suggested to use. You mentioned like making sure that there is clean data or the data that comes in is good data. How are you doing that? Because that seems like a bit of an impossible job.
Luca Fiaschi [00:14:18]: It is a very hard job. But these LLMs are surprisingly good. Maybe I should also prefix this. So Bayesian models are particularly tailored towards this problem because the first things that you would do with the Bayesian models, you don't work in a scenario of very large data sets with millions of features. So Bayesian models are really, really good. Where you have relatively small data sets, you have several features, but maybe in the area of 30, 40 features themselves, it's a small data application, very high tailored problem in a very high stakes scenario, but relying on relatively small datasets. And the reason is why when you have small datasets, the prior of these Bayesian models help you with completing the missing gaps in the fact that you don't have enough data to draw full inference. So they're pretty particularly tailored to that.
Luca Fiaschi [00:15:16]: So that allows you to give this data in the context of the LLMs often. And so in the LLMs by having access to this data in the context of the LLMs will realize, for example things like hey, there are missing values here, hey, there is a trend in the data that's a little bit strange. And then when you actually prompt the LLM appropriately by telling the LLMs hey, probably these are type of analysis that you need to actually do for quality control and so on and so forth, it often comes out with some interesting insights about the data that that allows you to then catch early specific problems. It's not perfect, certainly still developing the technology, but there is a series of quality control checks that you can prime the LLM to and they're going to come up even with follow ups and further checks and they can execute that pretty quickly on this type of data sets and the next LLM to the next agent for the next steps of the analysis.
Demetrios [00:16:25]: Yeah, and how are you doing? How are you operationalizing this? Is it some DAG that you have the LLM cleaning the data as one of the steps of the dag.
Luca Fiaschi [00:16:35]: Right. So right now we are setting this up as a Lang graph application and there is, there is a full graph that is behind this and one step as a quality control agents, another step, it's an insights agents that taking the data can actually draw some interesting plots of it just to explain what is in the data in the main trends and the main insights, the relationship within the variables. Then there is a modeling agent downstream that actually builds the model on top of it. And there is forecasting agents, allows you to make inference with the model about the future and the scenario planning agents that allows you to create scenario plans, optimized configurations for your variables for your forecasts and so on and so forth. And even a some which is a big problem for analytics teams and time consuming things which is creating PDF and decks out of the insights of the models of your analysis. We are also building right now a PowerPoint agent that actually creates a deck with the final recommendations for the stakeholders so that they can have it at end and readymade.
Demetrios [00:17:49]: And how are you confident that the data you're getting after it's gone through all of these different steps is high quality data.
Luca Fiaschi [00:17:59]: So at this stage of course there is some parts of automated checks you can make. For example, you can check for null values, you can check for things that are out of scale. For example, if you see that you're spending in a marketing channel, in the MMM example, if you spend in a marketing channel, hundred, two hundred million dollars in a week, that's probably a little bit too much. So there is something that's certainly out of scale and LLM will note that down. Otherwise you still rely on a workflow where the human is in the loop. It doesn't need to be a human which has specialized technical expertise in terms of coding because the LLM does the coding for you, but it needs to be a human that has business context good enough so that can understand whether the data is the right data and the output of the entire workflow is something that's sensible and you can make a decision on reliably.
Demetrios [00:19:05]: That's fascinating to think about. The most important human to be in this loop is less of a data scientist and it's more of the one who understands the business context so they can flag something when it looks a little off.
Luca Fiaschi [00:19:22]: Right. So in fact who's the target customer of an application like this is like busy analytics and data science tips that want to augment the workflow of their analysts and data scientists without having them to write the code necessarily from scratch for some of these models or come up this semi automated analysis. And the other one is really business stakeholders that are technical enough to understand what's right and what's wrong, but have also like a deep business context that they can help them guide the analysis and guide the results of the models.
Demetrios [00:20:01]: Yeah, it reminds me of the SQL analyst agent that process put into production recently and they were talking about how they have this bell curve in a way where you have the very advanced SQL queries that you need to ask and you need to spend a lot of time digging into the data. And then on the other side of that you have the do it yourself and everything is going to be written by an LLM. So there's like a spectrum of how much LLM you're using and on one side it is only LLM self serve and on the other side it is no LLM because it's far too complex. And what they were mentioning was the majority of the lift is in the middle where it is something like you said, it's augmenting those who already understand or have enough experience to be able to get the value from it, but they aren't on either side of this spectrum where it's highly complex and you can't use an LLM or you don't know anything about it and you're relying 100% on an LLM.
Luca Fiaschi [00:21:14]: That's exactly right. And it's a great example that you make because the idea comes a little bit from that and it's a development of the idea of SQL agents. Because when I look at that idea I thought, oh wow, that's great. But it stops at descriptive statistics, which is the 101 of analytics. What if instead of just doing descriptive statistics and get SQL out and nice plots, you can actually do predictive analytics and mix forecasts and close the gaps between those advanced statistical models and the delivery that you need to give to the business in a very fast way. So it was inspirational. The work of what companies like DataBricks are doing, for example, like agents like GENIE that does X to SQL completely integrated in the databricks ecosystem and data lake. However, our idea was like you shouldn't stop there, you should actually go the last mile now, help automating or like supporting and augmenting the entire analytics and data science process.
Demetrios [00:22:27]: And so now there's a lot of agents that you have working and you also have the human in the loop being able to oversee the agents. How are you doing evals, if at all? And that feels like it could get very sticky very quickly or it could just become a ton of work. If you have all of these agents and you're trying to make sure that they are producing high quality data.
Luca Fiaschi [00:22:56]: Yeah, we're still learning about what's the appropriate way of doing evils. We have some reference workflows, so we have some reference data and workflows and we do know that the agent's applications needs to come to certain conclusions and we have way of verifying that the agent applications, you know, doesn't get stuck or gets results and parameters for the models that are aligned with reference parameters for the models because you can verify that even generating synthetic data, for example. And so we have a set of those that are in place. We have Langsmith and other telemetry that allows us to check what the queries of the users are and see whether the user gets stuck. But finding the precise way and the more systematic way of evaluating this type of applications is something that I don't think we have solved yet. And I don't know if the industry has entirely solved yet. There's still kind of an open, an open debate.
Demetrios [00:24:03]: Yep, a hundred percent. And you mentioned to me before too that you're leveraging this for product development and almost getting like user research done. Can you talk to me about that use case?
Luca Fiaschi [00:24:16]: Yeah, that's, that's a, it's a little different use case of what I thought, thought about. But it's kind of the same idea and in, in a bit of a, in a bit of a different way. So let's. The idea here is that you have an entity like a Bayesian model, a machine learning model. You want to talk to it like it was your friend and ask how you've been built, building a forecast and so on and so forth. Now there is another problem that often is company have is that they don't know that much. Their customers and product managers, business stakeholders, marketing people, designers, they wish they could talk to their customers and user every day to learn and get more insights about what they are doing, how they're using their product, why they're using the product. And so another applications we are building is some virtual representation of your consumer that we call synthetic consumers which you can ask any type of question to.
Luca Fiaschi [00:25:18]: And the typical applications would be, hey, you are, I don't know, a CPG company for example, and you are developing a new type of, I don't know, like let's say a new type of toothpaste. And you want to know whether this resonates with the user base and the consumer base. Well, the only way you'd have to do it right now you need to do very expensive panel interviews with tons of consumer research and it's very time consuming and expensive. While you could have a swarm of LLMs that are primed and prompted to behave like real people and you can just ask, show them pictures and ask them questions of would you buy these products for a certain price point? What are the features of this product that you like and there is research that have shown that within certain constraints this can be actually representative of the real population. Especially if you build this synthetic consumer in the right way, using the right type of data and so on and so forth. And we are still in the early stages of building this application for a Fortune 500 client, but that's the next type of things. And I think in the longer term this could become really an interesting technology. So imagine that you're a product manager and you're building a new website.
Luca Fiaschi [00:26:42]: Well, you probably want an operator, synthetic consumer that browse through your website, try to do specific actions and then you want to ask questions, oh, how did you like the button up there? Or did you find this confusing? This kind of like user workflow and why. And I think this could be extremely powerful to really close the loop between and design better UX and close the loop between, you know, product development and real users.
Demetrios [00:27:15]: Yeah, you can take that a step further too. And anyone that's to developing software tools or infrastructure tools, you can get synthetic data or just have an LLM use your software tool and tell you what they think about the API and how your specs where it's confusing. And so that is a huge promise. I wonder how in line it is with real humans and how many insights. I guess at the end of the day, even if it's not totally in line with humans, like what real humans would be doing, if you can gather insights, that's what you need and you can at least get a few iterations before you bring something to market or before you come out of stealth or whatever. And so this is a fascinating way of doing it. Now the, the main question that I have is how are you making sure that the LLMs are properly set up to be these consumer profiles? Are you prompting them? Are they fine tuned to a certain person? What does that look like? And also what kind of models are you using?
Luca Fiaschi [00:28:37]: Yeah, that's a very good question. I wanna answer first. Your first insult. This can be fascinating. So the idea comes from a very personal actually experience. I'm a little bit of a timid person. I kind of like, I wish I could speak freely with and get connections with a lot of different people. And sometimes the applications I have and the discussions I have with LLMs, it's extremely deep.
Luca Fiaschi [00:29:03]: So that's why I wish I could talk to anything. I could talk to a scientist or I could talk to famous scientists like Richard Feynman or I could talk to a user of my final applications that have no problem asking deep questions to them. That's why I'm so excited about building stuff like this. Now the second part of your question is are you actually building it and making sure that they're aligned with the entity you want to represent, meaning the user that are the user of this application. So at the moment we're exploring variations of prompting and that brings you or like let's say 70, 60% there. And the typical way you would do this is by really prompting the LLM with things like hey, you are a black woman who's living in Brooklyn, you have a tech job, your confidence about this, you bought items of this specific product category recently and that brings you there up to a certain level. What you can also do, and we are experimenting with that, is that you can take this company's past consumers research and surveys that were answered in the past, do supervised fine tuning and basically they had already like massive data sets in the past of product. They've shown to consumers research users where they collected demographics of those users and specific behavioral traits.
Luca Fiaschi [00:30:49]: And you can use those to do supervised fine tuning and then create LLMs that are specifically for that. Of course you base these on open source models to do supervised fine tuning like the llama models for example. Now it's really complex and there's a lot of nuances of it. For example, you know that an Most of these LLMs they have specific political biases. For example, has been proven that American LLMs they are a little bit like left leaning in general. And what you can do, and we are also exploring is techniques to remove these biases to make sure that the base LLM you start with is actually impartial. And you can do that with also technique like ablation for example, which is a technique to remove specific biases from LLMs by removing and killing specific neuron within the network. So we are still at the beginning of it.
Luca Fiaschi [00:31:59]: The basic applications bring you 60, 70% there, especially when you go in fine grained subsegments of users. It gets the average and the bulk of the American populations right. But when you look and slice your data in subsegment of the users can be very off. So we are looking into these techniques like supervised fine tuning, ablation to refine the model outputs, especially on subsegments.
Demetrios [00:32:26]: Well, the idea of having someone go through your website too and looking at heat maps and all of that, one of the first things that I did when I was at a startup that kind of gave me a bit more freedom to play around with the product was we had a tool installed on our product called fullstory and that would record everyone's sessions in the product. And I would just sit there. I was mesmerized by how people were using the product because you can really see where folks get caught up and where their snags and full story, I remember had this feature, I don't know if it was a feature. It had an alert when someone would do what they called rage clicks and that was where if it wouldn't get, if someone wouldn't get what they wanted on the first click and then they would click like four or five more times because they wanted that. But for some reason the button wasn't working or the page wasn't loading, whatever it may be. And so it's, it's incredible to have that almost before you do anything or before you actually have real users and you don't have to have real users go through the pain and that rage and you gather the insights and then you can Talk with the LLMs to gather those insights and say what are some of the key things that I'm missing? Here's my write up maybe or here's some things that I'm seeing. What am I missing?
Luca Fiaschi [00:34:01]: That's. That's right. You need to do it. If you gather that just from data you did do it need to do a lot of guesswork and that's the reason why UX research exists and qualitative surveys exist is because quantitative data brings you like probably 80% there but you still want to understand human behavior also from a qualitative background. And these techniques I hope in the long term will be a way of like bringing UX research to the next level.
Demetrios [00:34:36]: Yeah. And so are you trying to operationalize this or is it something that is much more handcrafted for each use case that you encounter with the companies?
Luca Fiaschi [00:34:48]: Yeah. So at the moment we are basically consulting companies. So we have clients interested in building specific applications which is for product innovation and in the CPG space. So we are developing this with that angle in mind. I do think that unlike other consulting companies we are a little bit different at PMC Labs because we have a strong belief in innovation and open source. And that's the reason why we called Labs because we are recognized internally as a group of researchers that it's wants to solve interesting problems and everybody has fantastic backgrounds both in academia and in the industry. And so we think that some of these applications we are developing right now as using concrete problems that comes from our clients in the industry in the Longer term can be strategic for us to develop. And they're going to be released as open source where we can of course, um, and you know, they're gonna constitute a body of work that God's gonna remain for everyone.
Demetrios [00:36:02]: Now when you look at other use cases and ways that you can merge the Bayesian world with the LLM or just language model world, have you seen other stuff that you want to attack? Maybe you have put time into trying to make it work. Maybe it's an idea that's floating around in your head.
Luca Fiaschi [00:36:23]: Yeah, I think this area of probabilistic deep learning and probabilistic neural network is really interesting and it's another area you want to attack. So we talked about the idea of augmenting the Bayesian work through LLMs. We talked about the idea of running even simulations of agents that behave like users. And then what you can do, and I mentioned later on, is to add to these simulations and Bayesian prior later on. But intrinsically there is also another angle which is your fundamental deep learning model can become a probabilistic model if you add probability distributions on the weights, for example, and you sample from the neural networks. That's the reason why the technique you use in Bayesian modeling like building computational graph sampling from GPUs, they're actually the same technique, computational technique fundamentally that you use in deep learning. There is Even libraries like TensorFlow probability, which is an adaptation of TensorFlow to the problem of building Bayesian models. Now probabilistic deep learning, it's extremely interesting, it's very hard to solve computationally and there is still a lot of research to be done on whether the probability distribution you get from these deep learning models is really calibrated and the correct probability distributions you can rely on.
Luca Fiaschi [00:37:56]: So lots of research to be done. There is a body of research that comes from ATH Zurich that came a couple of weeks ago out in a very nice summary paper. So I think that's where I want to go there next. It's because it actually addresses very key problems, business problems, especially in the area of you know, high stakes predictions like for self driving cars for example, where you need to know the confidence of your like predictions for is there a pedestrian there? Or things like financial forecasts for I stick scenario like for example hedge trading and hedge fund trading or things like medical predictions for knowing whether a person has cancer, for example. So these are extremely interesting topics that we probably are going to get also like you know, some work from our side in the future.
Demetrios [00:39:07]: And I missed how Exactly. That corresponds. Or did you say that that's just somewhere where you want to start focusing your attention?
Luca Fiaschi [00:39:18]: Yeah. So I think it's the bleeding edge of research today. It's still, I would always divide, let's say the world into categories. The bleeding edge of research, it's not yet ready for industrial applications, then things that are ripe for building applications on top. And then there is the state of the art that everybody uses and keeps building up. I classify, and this is of course just my opinion, but I would classify probabilistic deep learning as the bleeding edge of research. Almost at the brink of becoming something that you can implement in industrial applications. And of course, you know, people is going to shoot me and they're going to say, oh, there is already some applications built on top of probabilistic deep learning.
Luca Fiaschi [00:40:04]: I'm super deep expert in that topic. But that's kind of my, my high level assessment of the field at the moment.
Demetrios [00:40:12]: It's so cool, man, that's. It's really nice that you are so deep on this stuff and you're thinking about it and then thinking back to how it can be tied into the companies that you're working with and how can we bring business value with this. Right. And so one thing that I, speaking of asking users for feedback, it's so funny that you mentioned this because I literally this morning before we hopped on this podcast, I spent probably an hour and a half emailing folks that sign up for the mlops community and that have written me, because one thing that I ask for, just to find out if they're human or not, is what's your favorite song. And so on that first email, when you join the MLOps community, it says, hey, this is the ML Ops community. I'm Dimitri is here just so that I know what's your favorite song. And so people write me back with like favorite artists and all that. And by the way, it is an incredible way of finding new music.
Demetrios [00:41:09]: I have such cool playlists now that I never had heard of. But what I was doing for an hour and a half today was writing everyone back saying, great suggestions, finding new music, this is awesome. And also asking for what's going to be the most valuable thing that this community can offer you. Because I always want to know what else can we be doing in the community to make it more valuable to people. And so that type of stuff, I need, I need the LLM, I want to talk to the LLM also. And then also, just the reason I was bringing this up is at the End of last year, I sent out a bunch of emails and messages to close friends asking them what's something that we can do more of in the community in 2025 that will be valuable for you? Right. And one that has stuck with me. I can't remember who told me this, but somebody said, I really appreciate it when you talk about bringing the ML&AI field to the business side and tying it to business metrics.
Demetrios [00:42:29]: I think I'm paraphrasing obviously, but tying it to business metrics. And so I feel like you're someone who sits in a unique position because of the area that you're in right now, being able to work with a lot of these Fortune 500 companies, but also all the stuff you've done in the past with these hyperscalers. Do you have ways that you can sniff out high value use cases or high value or. Or basically bridge that gap between the machine learning and AI side of the house and the business metrics and tying it back to it that isn't just an LLM creating a PowerPoint for you?
Luca Fiaschi [00:43:11]: That's a very, very, very good question. I think that I don't have the magic answer to that, but there are two principles that I use that are really guided me in my career. So the first principle is try to understand very deeply the business model and what moves the needle percentage wise, meaning is in these specific business models, you can always model as a graph and then if you change x percent in this variable, let's say marketing spend, how much the revenue profitability of the company changes per percentage wise. If you find those variables of very, very high elasticity, meaning a small change here changes kind of a lot, your top line and bottom line. Then you found an area where you want to dig deeper. And often what I do when I join a new company, I do an analysis of their business model. I try to find those areas of high elasticity, looking really at the composing the business model, kind of like a graph of variables. The second one is that, and this is more like a leadership principle, you don't need to come up as a leader with all the answers all the time.
Luca Fiaschi [00:44:40]: You can rely on your team. And often if you think about your portfolio of teams as a head of data, you have data platform teams, your analytics teams, you have machine learning teams, and so on and so forth. Your analytics teams, it's the gold nugget finder team. So they work closely with stakeholders. They often uncover needs, insights, opportunities that your data platform teams and your data scientists, the machine learning engineers, wouldn't see otherwise. And you can use them really to evangelize the approach that your team has and to uncover new interesting problems. And so often, or actually a trick that I learned that I use in all my teams is that data teams should have business calls. And a business call that I often give and KPI often give to my analytics teams is by the end of the quarter you need to find 10, 20 million dollars of new opportunities.
Luca Fiaschi [00:45:42]: And so I give the problem back to them and I ask them to solve the problem in this way. And it's a core KPI that they have, they got to eat it and it really doesn't matter whether it's 10 million or 20 millions. And if they achieve X percent of it only, but it puts them on the offensive to going to their stakeholders and instead of just getting the problem from them, helping them think through their problems and finding new opportunities. This.
Demetrios [00:46:13]: So this makes me think almost in, in a different way that I normally will look at it. But if you are looking almost at a pipeline of use cases or potential use cases, you have the analysts that are out there like Sherlock Holmes trying to uncover new use cases and then figure out how much that is going to make for the business or save the business. And then once that is properly scoped and there's an idea there, then they can go and hand it off to the respective teams or they can go and champion for it and then it's up to the leader to make the decision if it's worth pursuing or not. And then you go and you say okay, well this is actually going to be implemented by the machine learning team or it's going to be something that the data platform or machine learning platform team has to go and implement. Because we see that if we can increase the velocity of getting a machine learning model to production by 2% or 10%, then that's going to save us or make us X amount of money or if we can bring down the fraud by 0.3%, then that's going to save us X amount of money, whatever it may be. That is a really cool way of thinking about it as like the analysts are out there searching through the business just like lifting up rugs and trying.
Luca Fiaschi [00:47:45]: To find dirt or they get it from the stakeholders. For example. It's completely new problems we haven't thought about. For example, I mean in Hindsight now at HelloFresh, you always had this problem of getting amazing pictures of food and creatives of food and you know there is entire photo op team that's dedicated to that well I mean like the analysts could have noticed that specific angles, specific like type of pictures got tons more engagement and don't go figure with your MML team. How do you actually scale that process up with generative AI. So here it's new business problems that was uncovered by an analyst amid the and a good observation. It's not the team as a head of data that I work with that often the team of like photo op for like food. So that insight is brought to me back to from my analyst.
Luca Fiaschi [00:48:42]: I say wait a minute, there is something to dig further here. Let me go make the connections with my data science teams and let's try to automate that process and get value out of it it so that's the way it actually works. The value of the analytics teams is to be decentralized in your data portfolio and to really get this inside gold nugget that comes across the business from everyone in a way.
Demetrios [00:49:10]: Yeah. And then figuring out I imagine there's gotta be some tough calls that are made that potentially like you were saying, the technology isn't there yet. I know that there was someone who I was talking to a few weeks ago that is embedded in a finance team and there's over 42 people on this finance team and the majority of the stuff that takes this finance team ages to do and what they are constantly being flooded with are PDFs from banks and they've tried so hard to figure out how to ingest those PDFs so that LLMs can do the bulk of the work. But it has been a tedious process and they haven't been able to get there with technology to ingest the PDFs and allow the LLM to fill in the PDFs for them. And so there it's almost like the is it worth it to spend x amount more time? I. I guess if it's a gigantic business problem like you were saying back to the first part of your answer on how important is this? If we spend a year on it and we get 1% savings, if it's a million dollar company that's probably not good. But if it's a billion dollar company that's going to be well worth it.
Luca Fiaschi [00:50:36]: That's exactly right. There is also like or there. There are principles that you can also apply here depending on the phases your company is in. So for example, if you are in a growth stage company it's rarely the right thing to focus your team on cost saving opportunities and automation opportunities. Why? Because your business is growing really really fast and Typically when it grows really really fast, the opportunity cost of solving a growth problem like how do I get the next $10 million of revenue versus solving a relatively small scale automation problem. How do I get four people to spend 10 hours less on this specific problem? It's rarely worth the money. However, if you are already a business at scale where growth is really hard, then actually focusing on cost saving opportunities is important. For example, if you are in Amex and you have thousands and thousands of agents doing phone calls and that's big chunk of your costs, then of course working on automations of client success processes is extremely important.
Luca Fiaschi [00:51:55]: So you need to think where your company is at, where the strategy of the company is at, and of course these gold nuggets that your team identify needs to be contextualized within the broader diction.
Demetrios [00:52:09]: Now, you mentioned at the first part of that answer a while back how you will get very intimate with the business model. What are some things that you do there? Is it just you're reading their S1s or 10Ks if it's a public company and if not, are you going to the CFO and saying hey, how does, how does this thing work? Or do you have other strategies of making sure that again you're able to uncover rocks that seem like pebbles, but really they're boulders?
Luca Fiaschi [00:52:42]: Wow, that's such a. I don't know exactly if I have a good recipe for doing this. I definitely talking to people is extremely important. I mean if you're sitting in the company as the head of data at the VP or chief executive level, you of course need to work very closely with your peers to understand the business model, with the CEO to understand the business model and how the company works. In a way, as a head of data, you also have the superpower of being able to look at the data very closely. And what I typically do, I look at concept like ltv, which is, you know, a very historically it's a very concept that's been built and studied throughout. But understanding where the value is created in your business model and how these accrues to revenue, it's the first step if you really understand that, that you can model it even with a Bayesian model, for example, then you have understood your business model. And then I look at consumer research, I look for example NPS surveys that we did to our users and what problems they surface within our product to identify other areas of opportunities.
Luca Fiaschi [00:54:04]: Often talking to your CPO is extremely insightful. CPOs are obsessed with using the products themselves and they often uncover like very interesting Insights and problems. So this is the tricks that I use to really get familiar and acquainted with the business model. Most lately I also use deep research from OpenAI a lot. I'm a power user. I think of them often. I have just the prompt. If I'm working with a company I never thought or I never worked with before, I asked Deep Research to give me like an overview of the industry, the trends and why this company differentiate from the competitions is what's their strategy.
Luca Fiaschi [00:54:47]: And that's actually is a good way from an external point of view to familiarize with the new business model and with the new companies. I Talked even to VCs that are using Deep Research to think about the investment area and opportunities. So.
Demetrios [00:55:05]: Actually now that you say that, it is so cool to think about how you can use Deep Research just to get an understanding of what the competition is doing also and what their most valuable products are or services that they may be selling. And then it can inspire you in different ways potentially. Or it can show you where, oh, maybe there's a product line or an offering that we can incorporate into our company also. So I've just been using Deep Research and I use the Gemini version, but I've just been using it to like research stuff that I want to buy. I didn't think about researching that kind of thing. You are much further ahead of me and I appreciate that little tip. So now whenever I talk to a company, I'm going to use Deep Research and get a full report before I talk to them.
Luca Fiaschi [00:56:01]: That's right. Very, very, very important.
Demetrios [00:56:04]: And it's like, yeah, if you're ever comparing what shoes you want to buy or what, you know, like I went from watching a ton of YouTube videos to now asking it, hey, I want to buy a new car. What's the best hybrid or what has the highest score? What cars do people like the most? You know, that kind of thing on those bigger ones, just so I can know like, where's the signal, where's the noise? And it does a pretty good job of it. Or yeah, shoe. I, I think I've done it for shoes, cars and my buddy told me about it. He told me he was doing it for his watches for like exercise, you know, like Garmins. Because there's anything where it's like there's a million different models and each one has its own little things. However, I have tried it for GPUs and it did not work. I was like, I want to know all.
Demetrios [00:56:58]: Yeah, like GPU reserve. Like basically if I want to pay for some GPUs. I want to know how much, what's the pricing models, what are the different value props that each GPU provider has? And there's like managed GPU services and then there's non managed. And so what are the ones that do this or that can't really get a good handle on it. And the whole reason I wanted to do that was because we're creating this like GPU buyer's guide. And so we're trying to put everything that folks would want to know when they are trying to buy GPUs or just like rent GPUs even. What do you want to know? You're on the Market for some GPUs, what do you want to know? And so we're putting that all together. I tried to do it with Gemini and I couldn't get a good because.
Luca Fiaschi [00:57:54]: I what is the reason do you think? And they didn't get to the right answer.
Demetrios [00:57:59]: I think it's super confusing. And so I don't know, maybe the, maybe the LLM, I shouldn't underestimate the LLM like that. But one thing is to be able to find first of all where I think deep research fell down was that it wasn't able to find all of the different providers which you would think like with Google it would be able to, but I don't. There's a million GPU providers out there and I know of a lot of them and I know even more now after doing this research and the report that it put together did not have half of them. It also had a bunch that weren't really what I was looking for. So it was, I, I don't want to say it was like scam pages, but it wasn't high quality pages. And then the value props and the pricing, they had no idea like they really didn't get well. And so I don't know if it was hallucinated or if it was just especially on the pricings, you know, and, and I want to go as deep as when somebody's on the market for a gpu, they're probably on the market or they could be convinced to use a TPU or Amazon AWS's like Inferencia or Trainium.
Demetrios [00:59:18]: And so I also wanted to incorporate those in. But as when you say GPU for deep research, it's only looking at GPUs it's not looking at now like oh TPUs and inferencia and all of that. I, I, of course I could prompt it better by just Saying now also look at Inferencia, but it might be my prompt. It might just be that, you know, like. But we're really. I can invite you to the notion space where we're trying to do all of it in case you have any feedback on. Have you ever been on the market for GPUs? I feel like you have.
Luca Fiaschi [00:59:51]: No, I haven't. I haven't.
Demetrios [00:59:53]: No. All right then maybe not the best.
Luca Fiaschi [00:59:56]: Yeah, cloud. Cloud resources off the shelf as well. We haven't shopped ourselves or GPUs, but it's so fascinating. I actually never thought about using it for shopping, which is an obvious application. In retrospect, I'm lucky. And second, is that on such a specialized product that cannot really find a good summary, which is interesting.
Demetrios [01:00:20]: Yeah, it's weird because of maybe there's just too many providers and so the context or I don't know what they're doing behind the scenes with the deep research agents that they've got. But it's not really. At least when I did it a month ago it wasn't working. So maybe it has changed since then. And I also again was using like Gemini's Deep Research. So Maybe like the OpenAI's deep research.
Luca Fiaschi [01:00:49]: Significantly better than the Gemini ones.
Demetrios [01:00:51]: Is it?
Luca Fiaschi [01:00:52]: Yeah.
Demetrios [01:00:52]: Oh really?
Luca Fiaschi [01:00:53]: Way better. So like while Gemini is good for a quick summary like the one from OpenAI looks like there is some thought into it. There is a good thinking structure process that comes out of it. It's like the difference between a senior analyst that just compiles sources and maybe like a consulting project manager or partner that actually think through the storyline. That's what would think a report.
Demetrios [01:01:26]: Also after the fact you can ask.
Luca Fiaschi [01:01:30]: For different formats to give you like a full report. You can tell him even hey, I want a podcast out of it style presentation actually format. You want to digest the information.
Demetrios [01:01:46]: That's. That's interesting because this is. Well, if you want to ask Deep research on the OpenAI side and send me the report it gives you, I would be happy because I, I don't pay the 200 bucks a month in the prompt.
Luca Fiaschi [01:01:59]: I'll. I'll. You can send me the prompt that you use for Gemini. We'll try it on open. You can send back the link to the result so you can see the link the.