Balancing Speed and Safety
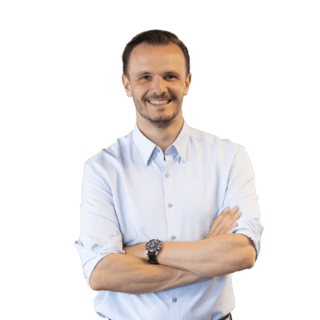
Remy Thellier is a recognized expert in AI and ML, currently serving as the Head of Growth and Strategic Partnerships at Vectice. He leads the largest community of AI top leaders in the USA and is a sought-after speaker at major AI and ML events. His topics of predilection focus on leadership, managerial, technological, and regulatory challenges, specifically in the AI/ML space and its ever-evolving ecosystem of vendors.
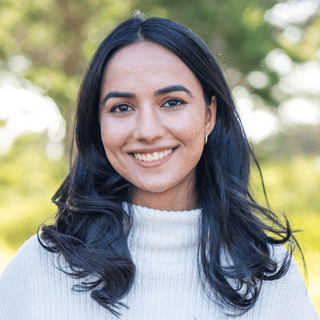
Shreya Rajpal is the creator and maintainer of Guardrails AI, an open-source platform developed to ensure increased safety, reliability, and robustness of large language models in real-world applications. Her expertise spans a decade in the field of machine learning and AI. Most recently, she was the founding engineer at Predibase, where she led the ML infrastructure team. In earlier roles, she was part of the cross-functional ML team within Apple's Special Projects Group and developed computer vision models for autonomous driving perception systems at Drive.ai.
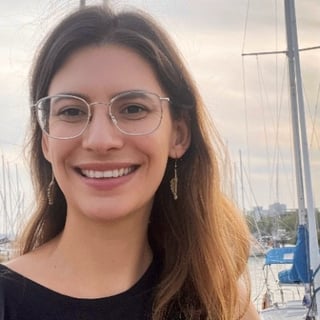
Erica Greene is a director of engineering at Yahoo where she works on the ML models that power Yahoo News. Erica has worked in the tech industry for 15 years at companies including Etsy and The New York Times. She is based out of New York City where she can be found playing cards, shopping at vintage stores and cooking. She writes a weekly-ish newsletter about AI and the media called Machines on Paper.
The need for moving to production quickly is paramount in staying out of perpetual POC territory. AI is moving fast. Shipping features fast to stay ahead of the competition is commonplace. Quick iterations are viewed as strength in the startup ecosystem, especially when taking on a deeply entrenched competitor. Each week a new method to improve your AI system becomes popular or a SOTA foundation model is released. How do we balance the need for speed vs the responsibility of safety? Having the confidence to ship a cutting-edge model or AI architecture and knowing it will perform as tasked. What are the risks and safety metrics that others are using when they deploy their AI systems. How can you correctly identify when risks are too large?
Remy Thellier [00:00:09]: Today we're going to so we're very lucky to have gardeners AI CEO here on stage and Yahoo's director. We're going to talk about AI safety and how to really balance this with speed. But before we get there, I just wanted you to introduce yourself. Um, go ahead, Shreya.
Shreya Rajpal [00:00:30]: Yeah. Hey everyone, my name is Shreya. As Rami mentioned, I'm the CEO of guardrails AI. Guardrails AI is primarily a company focused on building platforms to add AI reliability and controllability to any generative AI applications that you're building. We're primarily an open source company with an enterprise offering as well. Before starting guardrails, I have worked in machine learning for ten years across research and computer vision, classical AI and planning, et cetera. Self driving cars for a number of years before that. And then I guess like everybody else here, Tor and mlops as well.
Shreya Rajpal [00:01:05]: Awesome.
Erica Greene [00:01:07]: Hi everyone. My name's Erica. I'm an engineering director at Yahoo. I manage a team that owns recommendation systems and other AI ML applications at Yahoo. Specifically focused on news. So content recommendations. I've worked in this industry almost 15 years back when we trained machine learning models ourselves. I've worked across media and e commerce mostly on consumer products and.
Erica Greene [00:01:31]: Excited to be here and chat about, yeah, speed and safety.
Remy Thellier [00:01:36]: Awesome. Thank you so much. I'll give a quick introduction about myself too. My name is Remy. I'm leading growth at Vectus. We're a documentation platform for machine learning and AI. And I also lead a community of AI leaders of over 1300 director plus executives all across enterprises in the US, specifically in AI. So today I'm super excited about the fact that we get both on the field experience about the topic and also a vendor here which at the same time has this broad view of all the companies they've been working with.
Remy Thellier [00:02:17]: So I'm very, very excited about hearing about this complementarity of the two profile here. I want to start by defining AI safety. I think starting off with definitions is always a great way to start. So yeah, Erica, if you want to start with that, what does that mean for you?
Erica Greene [00:02:34]: Sure. And I would love your answer as well. So I actually, I asked for us to start this question with a definition because I think this term means different things to different people. And I guess I wanted to start out with the, the call out that this is not AI safety conversation. Like the robots are going to take over the world. Unless you want to talk about that. But I don't really want to talk about that. I don't think it's really relevant to us living on earth right now.
Erica Greene [00:03:05]: But I do think that there are real risks to these products. There's always risk to any sort of company. There's certainly risk to tech companies. And as we add more and more complex technology quickly, there's sort of more and more risks, and we have to be thoughtful about it. So I would say broadly risks. And the other thing I wanted to say is that it's contextual to the industry that you're working in. And certainly if you're working in self driving cars, there are very large risks, as we heard this morning from the CEO of cruise. But there's risks in other areas as well.
Erica Greene [00:03:35]: I mean, I work in media right now, and there's a real risk if you're auto generating summaries, auto generating content or headlines that you might give people misinformation about the election or something like that. Right? Tell people that the polls are closing at a different time than they are actually closing, and you send that out to a bunch of people in Wisconsin or Philadelphia that has real risk. So I think safety and risks are not just confined to industries where you could cause, like, physical harm. Yeah.
Shreya Rajpal [00:04:04]: Yeah. I really, really want to underscore that safety, AI safety especially, is very, very context dependent. I think, like a widely, are a very commonly used definition of that is something that the Senate bill in California, SB 1047, talks about, which is essentially, can you. It's very specific to models and it's very specific to very concrete harms in terms of, is this model going to make it very easy for an average person to look up how to create weapons of mass destruction or bio warfare or increased cybersecurity risks, etcetera. So that's a very specific, narrow definition of safety. But, you know, often how you're using these models, right, is like, within the context of an application. And so safety becomes like, okay, I have, like a chatbot or I have a new summarizer, right? Is this news that I, that somebody, you know, who maybe doesn't understand the nuances of, like, a specific AI system? If somebody like that comes onto my platform, are they just going to verbatim trust everything I produce? And then as the generator of that news, then, you know, what's the responsibility in me? So factuality, you know, not adding any types of bias into your predictions, et cetera, is kind of what that definition evolves to if you look at it outside of the model and within the context of an application. And then that really varies upon what your application is, what your domain is, et cetera, amazing.
Remy Thellier [00:05:26]: Thank you. So now I want to ask you a question, Erika. In your experience, you've been working at many enterprises and you've been going from traditional machine learning to LLMs, and I'd like to know what's changed in terms of approach to safety.
Erica Greene [00:05:47]: Yes. So I think that the thing that's changed predominantly is that executives want to launch things quickly. I felt like in my early part of my career, it was a lot about convincing people who had never heard of this to use it and that it could work. And I was constantly pitching people on projects and they were like, meh, I don't know, is this really important? I don't think it's going to work. And now there's all this top down pressure. Right. So we used to be able to actually be much more measured, and we had to make a case for it working, and now we have to make cases for not launching it because it doesn't work. And that has been a real shift in the approach and the landscape.
Erica Greene [00:06:31]: And so I actually just, today I wasn't working. I wasn't working because I was here, but I killed a project successfully that I thought was a really, really bad idea. So normally I was on the other side of that argument, and now I'm on this side, and that's been the biggest change for me. Yeah.
Remy Thellier [00:06:48]: And as you were building those projects now, we touched on the communication, the drivers behind those initiatives. What on the field, the way you develop models, the way you design the systems. What has changed?
Erica Greene [00:07:04]: Yeah, I mean, to state the obvious, we used to have to train the models and get the data and have expertise in machine learning. I mean, that's what my background is in, in statistics and convex optimization. And you don't need that anymore. You just need to call an API. And so it's completely, as you say, democratized. Open up. Who can build AI applications? There's good things about that. There's bad things about that.
Erica Greene [00:07:29]: But yeah, anyone can do it. And in many ways, people who have no machine learning background do it much more quickly because they don't even think, they don't even know that you can tune certain parameters. They just plug it in and launch it. So that's the primary thing that's changing. You don't need to own the models, but it's not that everything has moved that way. I mean, many things. I own a big recommendation systems for content. Those are still trained on our own data that you don't want to, on every single request, go and call OpenAI or something like that.
Erica Greene [00:08:03]: But yeah, it's opened up a whole new area of applications that work and you can do fairly cheaply. It's cool.
Shreya Rajpal [00:08:11]: Yeah, I guess just to interject there, I think from a safety perspective, what's novel for this current era of machine learning, which is very lm gen AI driven, is that the surface area of what your task means is just so much broader than what it would be in the past for recommendations. It would be this very, very well defined, well scoped out task, which is, here's a list of things and then rank them or something. I'm oversimplifying, obviously, but I think now you have, I'm sure a lot of people here are familiar with, like Devin, which is this AI junior software engineer, and it, can you give it an abstract task and it'll go inside your GitHub repo and write up a pr for you, right? That like the surface area of everything that that task touches is so broad and that means that like what is, what correctness means or what it means for this AI system to do this task well is just very ill defined. It's a very complex function to define that well. And that means that now to figure out is this autonomous system that I'm trusting, is it doing the right thing is much, much harder to solve compared to the previous generation of machine learning, where tasks would be much smaller now.
Remy Thellier [00:09:24]: That the ecosystem changed and there's a whole new domain that opened. How do you keep track of all the new things that come up? What do you try? What do you not try? How do you manage the FOMO? So I'd love to get your input, Erica, first and then Shreya. Also, from a vendor perspective, how do you help companies navigate this new space?
Erica Greene [00:09:51]: Yeah, I guess the FOMO is real. Things are moving so quickly. Personally, I go through phases of digging in into the details and then building, and it's really hard to build things while also digging in. So I can't personally focus that much on both things at the same time. I'm in the very much applied space. A lot of the activity right now is going on in foundational models, scaling these models, the hardware, super interesting, totally irrelevant almost to my day to day job. And so the truth is that you don't need to follow all of the, the twists and turns. And if you kind of don't pay attention for a few months, a company could be started, founded, funded, and fail before you even know that you had to pay attention to them.
Erica Greene [00:10:37]: So like, I think there's just a lot of noise. There's a lot of noise. I have. I asked a friend of mine who's very balanced. He's a machine learning engineer, very competent, but very balanced, not into the hype. And I asked him last week how he deals with the FOMO and how he pays attention to the industry. And he says, there's one newsletter. Every Friday I open it.
Erica Greene [00:10:57]: I post two things that they post into our company, Slack. And my manager loves me. So I do think that that is probably sufficient. Maybe that's bad advice, but.
Shreya Rajpal [00:11:11]: I hope the newsletter is the MLops newsletter. Yeah. So I guess I do work in this area where you have this fire hose of new developments all the time. And my work is very, like, LLM focused, and it helps to have a short attention span, you know, and, like, be able to kind of, like, split attention across, like, a bunch of different things. I think it's like, there's no really good answer. I think there's, like, a few newsletters that I really like. There's a few, you know, LinkedIn accounts, Twitter accounts that there's a few, like, company blogs that I watched like a hawk, some subreddits that I think are really high value, shout out to r local llama, which, you know, I kind of watch like a hawk, which is fantastic. I think there's a lot of, like, just being keyed into the right communities.
Shreya Rajpal [00:11:58]: And honestly, mlops is a great place to do that. Yeah, that's kind of my answer. There's no secret sauce. You just watch some people that are really high signal and then focus on those.
Remy Thellier [00:12:14]: Amazing. So I want to touch now a bit on how do we take care of safety? First, very quickly upstream of the model with you, Erica, and then we can dive much deeper into downstream of the model. So, Erica, what do you put in place? What do you pay especially attention when it comes to the data? We talked earlier in this conference about RAC system, et cetera, the data that is fed or that the model has been trained on, et cetera. How do you manage the risk associated to this?
Erica Greene [00:12:49]: Right, so, right, so I'm for LLMs. I'm not training LLMs, to be clear, we're training traditional machine learning models and neural networks. We're using LLMs. I have no idea what these things are trained on. Lots of copyright data, likely. So I think that when you're thinking about, like, risk or safety, you need to define the harms. Right. You need to think about what could possibly go wrong and essentially do a pre mortem on what the application is and think outside the box.
Erica Greene [00:13:24]: If you are a reporter reporting on this company and this feature, and you want to try to poke a hole in it and write a tell all investigative piece, what are you going to start looking for? What are the edge cases? People who work at newspapers, who write all these stories or who work at the markdown or something, they're not about how all these systems fail. They don't have some special sauce. They just think and try it and think about the worst case scenario. And so there's no reason you can't do that. And so it's shocking to me how many companies get caught, like left footed on these applications because there's no secret sauce. You can also sit down and think about how these applications could go sideways. I just, anyway, how Google does not get people to do this correctly, it just blows my mind. And yeah, that's what you have to do.
Erica Greene [00:14:13]: You have to be really, really thoughtful. And I think you need people in the room that have different backgrounds than you. You need open brainstorming sessions. You need an environment where people feel comfortable to raise concerns at any point in the project. There's no right or wrong answer about the data that goes into it. It's about how you structure the system and how you integrate the AI ML component. And I'll just give one quick example, again, contextualize safety. This is, again, not killing people.
Erica Greene [00:14:43]: But I used to work at the New York Times and I worked on comment moderation. And the New York Times used to moderate all their comments by hand. So there's a team of people, they moderate the comments. If you write a comment into the New York Times, someone would read that comment and would have to approve it before it got posted to the website. So like pre moderation. And we wanted to, they had, we had a great data set to train these models on and we wanted, we trained a model and we implemented like, AI augmented system. And we were thinking about how to design it. And the biggest concern, the biggest risk to us was that some voices of our readers would be automatically declined, automatically rejected, and we would not know about it, that the system would maybe learn that comments about race or comments about homosexuality or something like that had a higher likelihood of being toxic and would just automatically reject them, even if they weren't inappropriate, and that those voices would be silenced.
Erica Greene [00:15:40]: So we built the system such that it would never auto reject, it, would only auto accept. And if something got through that was not good, it would be flagged by people and then it could be taken down. But that was the mechan, it was about the mechanism of creating the system and not about the data specifically it was trained on. We did see in the end that some of that data was biased and we were able to correct for that, but we thought about it ahead of time and that was the key thing. Yeah.
Remy Thellier [00:16:05]: So, Shreya, if we look at downstream of the model, like, how would you categorize the main risk that people experience?
Shreya Rajpal [00:16:14]: Yeah, I think it's actually weirdly parallel. Right. Which is that how do you even think about, like. So the risks are very different, like, depending on the application, depending on the domain. If you're a financial institution doing, like, customer support with AI chat bots, your risk profile is going to be really, really different than a consumer tech company that's building like an internal, you know, employee knowledge QA bot thing. Right. So depending on that, the risks are really different. But I think the key challenge if you're thinking about risk is still the same, which is that somebody has to really understand the system, understand your downstream stakeholders, and use cases and domains, and then come up with some opinionated manner of, these are the things we care about.
Shreya Rajpal [00:17:01]: Hallucinations is almost like a buzzword. People talk about hallucinations so much as the failure mode of large language models. But for a lot of use cases, hallucinations might not be bad. Or an organization has to decide if hallucinations are bad for them, and, you know, what can they really do? So just kind of like sitting down ahead of time and thinking about how bad is it for us if our system or chatbot or something hallucinates versus how bad is it for us if, you know, we accidentally leak some information that we're not supposed to, somebody has to come up with that determination. There's a bunch of different frameworks that help you do this. I think the nisten AI RMf is like one of the ones that comes to mind. ML commons, the same guys that do ML perf, like the ML perf benchmark, are also coming up with an AI safety, I forget, like AI safety benchmark or taxonomy or something. So that is a good full set of everything that can go wrong and that you have to kind of like, think about we, you know, at guardrails, we released this thing called a guardrails hub, which is basically for all of the different types of AI risks.
Shreya Rajpal [00:18:08]: These are the guardrails that you care about. And we thought, okay, it's the actual, like, guardrail or asset, you know, the code asset that verifies your risk. That'll be interesting for people. But actually it's just the enumeration of, you know, all of these types of risks that a product developer or AI developer should think about that, you know, people end up finding really interesting. So, yeah, I think it's like, mimics a lot of what you see pre, like upstream of the model.
Erica Greene [00:18:32]: Yeah. I'd also add that I think about risk as risk, the whole company. How much risk is the company entirely taking on, not just the AI systems? So if your company has a negative impact on somebody in a real material way, they do not care if there was an LLM in the code path that resulted in that. They don't care if there was AI in that code path. And I think that we can also use, and this is not generally part of the conversation, but we can also use AI to de risk. The company's in general. Right. Like, it doesn't always need to be adding risk.
Erica Greene [00:19:03]: It can also be taking risk away. And so one example of that is I used to work at Etsy. Etsy, you can people, it's UGC essentially. They can upload, anybody can upload items and sell it on Etsy. And they have a real problem of people uploading items that are against policy. One thing is that people will, there's this category that we refer to as baby bling. So people would like create toys and bottles and whatnot and bejewel them and then sell them on Etsy. And this is really bad.
Erica Greene [00:19:36]: Don't do this because it's a choking hazard for children. But, and so there was a team that would try to find the baby bling on Etsy. They would be much more aided if they had some image models to help them. And so you could actually use AI to de risk the entire company. So I do think we should think about it on both sides of.
Remy Thellier [00:19:58]: And Shreya, in your experience, what are the main use case that you've seen where it's been applied and where people really put metrics and frameworks in place? Could you have a couple of examples to share?
Shreya Rajpal [00:20:13]: Yeah, I think that's a great question. I think use cases, again, I guess I'm talking from the perspective of the current era of machine learning, which is very massively driven by LLMs and not about a lot of the work that I used to do in the past about self driving and other kind of different sets of ML problems. I think if you're specifically thinking about LLMs, most of the use cases that are successfully deployed end up being very constrained use cases. So use cases that are typically behind one layer of protection. So, for example, a lot of people have support chat bots, which are guarded by an actual human that gets the final say of yes or no. Is this okay or not to kind of, like, send forward? A lot of those use cases end up kind of like, making it to prod. I think some of the other ones are very internal question answering, like, asking questions about your HR policies, et cetera. So employee question answering, in terms of, like, the metric, like the evals and the metrics that people care about, I think you're kind of, like, circling, like, what the big unsolved question in LLM land.
Shreya Rajpal [00:21:23]: I haven't counted, but I don't know how many talks today were about LM evaluation. So I think it is an evolving conversation. I think people have some ideas of what works well or not. I think one thing we know for certain is what worked for the ML 2.0 landscape, which is very deep learning driven, and very static metrics, et cetera, is not as applicable in LLM land, because even the, like, even if you have ground truth and you can, like, even comparing ground truth with, like, prediction is really hard because the tasks are really, really hard. So some general principles for evaluation that work well is, you know, setting up your system so that you are accurately collecting human feedback and then, you know, looping that in and essentially, you know, using it to update, like, specific parts of your pipeline, like retrieval or generation or, you know, prompting, et cetera. But I think evals are. I think they are the kind of big problem of our times.
Remy Thellier [00:22:20]: Erika, earlier, during the conversation, you talked about killing projects which were not suitable. We talked about balancing speed and safety here. So when speed exists, the product has not been killed. How do you put the cursor, how do you select how much work to put, how much time to put into the safety compared to just shipping the product as soon as possible?
Erica Greene [00:22:50]: Yes, it's a good question. Good question. I think a lot of these applications, you can get something, a demo going really fast, so you might as well get a demo going really fast, and it's good to get as many eyes on it as possible to start to define a rubric for how to evaluate whether it's working or nothing, and having a rubric for what is really bad. Right. So I think one of the themes that I think is emerging from all this evaluation work is that it's good to have tiers of your test cases. Not every test case is the same. Right. So you really want it to work on the easy examples, and it better be really, really good on the easy examples.
Erica Greene [00:23:29]: And then there's the hard or the wishy washing. People don't agree on them. And then there's like the really bad examples, or it would be very bad. So you need to start, but you really need to get in to the data deeply and have a lot of people looking at it, not just the engineers. So bots that push the responses to slack channels send emails every night or something to get people looking at it. This stuff is so cool and looks so magical and it's so tempting, I think, for people like us who have worked in the trenches of this industry for so long when it didn't really work, to be excited to handpick the examples that are working and be like, oh, CTo, how cool is this? We can do this now. Make a demo with these handpicked examples. And then they're like, great, ship it.
Erica Greene [00:24:19]: But actually, that was one in ten. And the nine other examples don't really work, right. So I think we have to temper our enthusiasm a little bit and be really honest when we show people examples. So the project I killed, sorry I keep mentioning this, but this project I killed today, I was totally guilty of this. I found some really cool examples. They wanted to auto write some headline and auto generate some images and personalize it for everybody about news stories. And it works great on a few examples with a few hand tuned prompts, with a few hand chosen news stories. And then I was like, you know, I really should look on this, on like 100 examples, randomly chosen, look through it.
Erica Greene [00:24:58]: And there was a bunch of examples where the summarization misstated the headline and it said, like, so and so canceled the tour. Willie Nelson canceled his tour because he's sick or something. And then the summary was that he didn't cancel the tour and he's having like, a cancer tour or something, something really bad and really wrong. And I put that that's how I killed the project because, you know, there's no way to test that. And these models are really bad at news, breaking news misinformation because they don't know what's happening right now. So anyway, I think if the project is really not super risky and you feel comfortable with it, then just get your hands on the data and come up with a rubric for evaluation.
Remy Thellier [00:25:43]: Triya, how do you guide your partners customers on that balance in between safety and speed journey?
Shreya Rajpal [00:25:51]: Yeah, yeah, I think it's a great question. I think a lot of my view, and this might be, you know, the unpopular view, but unfortunately, a lot of this, a lot of this trade off, right, is like, it's almost like a product trade off, and there's no one right answer for what's safe for one person versus another. So in general, like, what I really recommend is like, as you're kind of like, you know, when you're, when you're doing the scoping of what this product should kind of like look like, right? It's like, okay, what are the inviolatable conditions for me as somebody who owns this feature or this application that wants their users to be successful with this application, what are the inviolatable constraints for me? What behavior do I want to guide my user towards? And then being able to ground that criteria in data? So not just picking, okay, I tried it five times and it works five times. But we're in this era where you really have this very, very, very long tail. And that is the key problem with machine learning, which is so many tasks are very hard to capture in, like, small datasets. So you really then, like, take that criteria, ground that in data, and then really think about, you know, this inviolatable condition that I care about a lot. How many times does it get violated with the understanding that it's never going to be zero. You know, you're always in like, non deterministic land, you're always going to have some sort of failures.
Shreya Rajpal [00:27:12]: But like, what's your susceptibility to that, which really varies for people, for everyone. Like yesterday I was talking to somebody who kept asking me, when you talk to your customers, what is the hallucination rate that they see and what is the hallucination rate that's acceptable to them? And that question, what's a hallucination rate that's acceptable to people? It's an impossible to answer question, even for me, versus for a financial institution that uses guardrails versus for somebody who is doing an indie hacking project, hallucination rate acceptability criteria is vastly different. So depending on, like, in terms of, you know, how you, given this, like, post metrics land that we're all in right now, like, how do you, how do you still execute really quickly while, you know, balancing safety is to, like, think upfront about, like, what does success look like for me? And then once you kind of like, build it out, like, try to ground that success in some data, you know, so is 5% hallucination bad? Tie that to some KPI or to some product metrics that's important, and then kind of build accordingly.
Erica Greene [00:28:20]: I'd also add to think about the reputational risk to your company. If your company is building something that's a fun, I don't know, fun, goofy consumer, social media, maybe you have, it's fine. But if your company's brand and trust and differentiator is having authority and being trustworthy and being reliable and being accurate, maybe this is not the technology for you. Skip it. I don't know. I think of the New York City government had a chatbot that gave advice about business permits and for, for small businesses, et cetera, and it started giving misinformation, incorrect information. Really bad, very bad. Like I would say, not at all worth building that because you want the government to be trustworthy.
Erica Greene [00:29:16]: You do not want the people living in your city or living in your country not to trust the government. I mean, people don't trust the government very much already. So I don't know, it's always a choice not to use it. And yeah, I don't know, you don't have to get on this bandwagon.
Remy Thellier [00:29:35]: So I see that everything is very particular to the use case. But what I'm wondering is, is there any complexity triggered by the technology, the audience, the industry? So maybe let's start with the technology. If we look at pure, like just prompt engineering or fine tuning or some rag applications, are there some very specific risk associated to those technologies that needs to be taken care of?
Shreya Rajpal [00:30:06]: Yeah, I think not owning the model and the models kind of being this black box that is hidden behind an API for this completely different organization, I think that introduces a lot of risk. So, essentially, I think a lot of people here might use LLM as a judge style of evals, where you essentially throw stuff at an LLM and ask, does this look right or not? Yes or no? I think if you run that experiment multiple times without changing any input, et cetera, you'll see that you just get different results each time. If you were to aggregate it, that's not reliable. Essentially, if you look at it with how it used to be before, which is, what's the delta with LMS? Earlier, even though models were black boxes and you had these small models that were neural networks and weren't interpretable, you would still have a lot of consistency. Like you own the training data, you could set the seed, you could set the inference stack, etcetera. And that meant that even if it wasn't this magical answer anything model, you still kind of like, knew where you stood with that model. Now that a company you don't even own model updates, your model provider, maybe under the scenes, ships a new model for the same GPT 3.5 version or something. That means that it makes it really hard to understand, like, what you're really signing up for when you build an application.
Shreya Rajpal [00:31:30]: And so the fix for that, right, is to like, this is what I kind of suggest. If any of you are interested in shipping this, which is when you're in that land, and if you think that you can benefit from using LLMs for any stack, the key thing to focus on is like, what is the inviolatable criteria? That is important to me, you know, as an application builder and thinking about whatever you build from that criteria perspective, rather than just like from a, this looks good on like a handful of examples or in demo or something, and so it's kind of good to go.
Erica Greene [00:32:02]: Yeah. I also think that there's a middle ground that you can take, and I'm curious if any of the people that you work with do this, of using LLMs to annotate data and then, and then train more traditional machine learning models on that data. Neural networks are powerful models, and in many of the applications that I see people using could be solved very, with very high accuracy with just a plain neural network. And so if you have the capability of training your own models, that's a way to get consistency, much more sort of observability into what's going on. You can then go edit the data up, sample the data, et cetera.
Shreya Rajpal [00:32:38]: Yeah, I think. Sorry, just. Yeah, I guess so. We do see that a bunch, and we've done a bunch of work on it ourselves as well, for like guardrails, for example, under the hood RML model. So we do this for a bunch of things. I think it's consistent and you can shape your data and you end up getting the results that you want, but it's not as robust as on an outer distribution example as an LLM would be. So let's say you train some data, the data looks a certain way. Now, in production, you end up getting a data point that looks completely different.
Shreya Rajpal [00:33:10]: It's not reflected in the data set that you have. You are. If I had to, if I were a betting person, if I had to make a bet, I would bet on an LLM with like, you know, nicely outlined instructions to handle that outer distribution example better than your small, consistent, you know, fine tuned model would. And that's, you know, that's where that balance kind of has to come in.
Remy Thellier [00:33:33]: So we're getting quite tight on, on time. So I just want to touch on one last topic, which is the people perspective on risk. Who do you think, who are you involving at the moment when it comes to risk? Which departments, which role are involved in those discussions? And also, Shreya, who do you recommend and who do you see in other companies?
Shreya Rajpal [00:33:56]: Yeah, I think it's the product owner if you're a large enterprise. Also, the risk and compliance teams typically end up getting involved in the later stages of development. And then finally it's the data science or dev teams that implement or evaluate how much that risk is actually present in your data. So those three stakeholders, product owner, risk and compliance teams and then the actual developers that enforce whatever risk mitigation strategies.
Erica Greene [00:34:26]: Yeah, I agree with that. Legal, I mean, depending on what industry you're in, you have to know what regulatory framework you lie under. And even though tech broadly is not regulated in the United States, we do have lots of regulations of other industries and there's technology companies that work in those industries. And so that is a hard constraint if you're rolling these out. So you should know that and you should talk to your friendly neighborhood lawyers about that. I work maybe not that applicable to a lot of you, but I work in media. We actually have an editorial arm. And I find that people, journalists are really good at poking holes in questions, defining good questions and poking holes in things.
Erica Greene [00:35:05]: So I actually always involve my editorial partners in these types of questions about defining. We actually have like contracts, we write our constitutions of how the product should work with very specific language. And it's very helpful actually to be, to have people with that kind of background involved in the process.
Remy Thellier [00:35:24]: Well, Eric Ashria, thank you so much for joining us today. And thank you so much to the audience. See everything filling as we talk. And that was wonderful. Thanks so much.
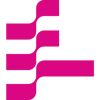