Stockholm 2024 Community Kick-Off Panel
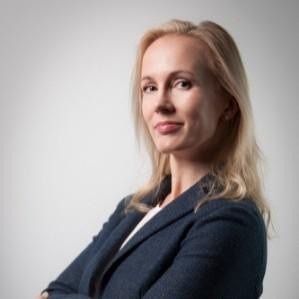
With over nine years of experience in solution engineering and architecture, Anna Maria today is working as a Senior Solution Architect at Elastic, the company behind Elasticsearch, Kibana, Beats, and Logstash. Anna Maria helps customers and partners design, implement, and optimize solutions that leverage Elastic's products and services for search, observability, and security use cases.
Anna Maria is passionate about learning new technologies, sharing best practices, and collaborating with diverse and talented teams. A consultant at heart, an engineer by training, and a Solutions Architect by trade.
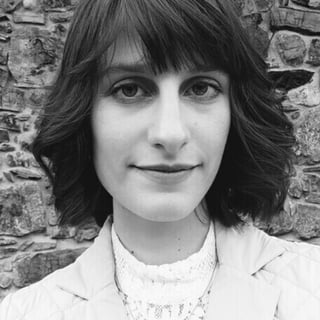
Francesca has a background in pure mathematics and has held the position of data scientist and AI/ML engineer over the course of her career, most recently at King and Peltarion. Francesca collaborated with various companies across diverse industries, contributing to multiple use cases with a focus on language models and computer vision. Currently, Francesca develops tools for ML engineers and data scientists as part of King’s ML Platform.
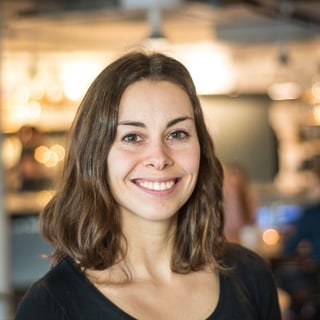
Rebecka has 8 years of experience building data products and teams and is passionate about enabling fast iterations on ML models. She is now co-founder of Twirl, a data platform for analytics and machine learning that runs within your cloud account. Before Twirl, Rebecka was Head of data at Tink and ML lead at iZettle, where she led teams building both internal and customer-facing data products. She has also co-founded Women in Data Science AI & ML Sweden and works hard to get more women into the field.
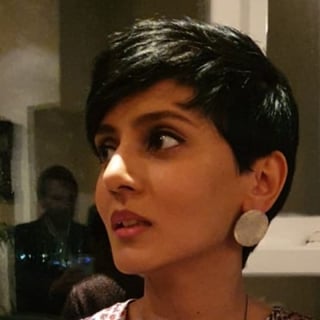
Data/ML scientist with a PhD in Quantum Optics. Saroosh specializes in solving complex industrial problems in multi-disciplinary environments, having worked closely with design, engineering, and business teams over the years. In Saroosh's 5+ years of experience, she worked across the entire product maturity spectrum, from exploratory PoCs to production-level code that is currently used in real-world services.
Currently learning about SciML and Physics informed neural networks and also exploring Quantum machine learning frameworks.
The panel of guests Anna Maria Modée, Francesca Carminati, and Rebecka Storm, guided by host Saroosh Shabbir, dive into an insightful discussion about the balance of simplicity and complexity within ML systems. They emphasize the need for providing straightforward solutions for common tasks, whilst allowing customization as necessary and prioritizing business impact over mere scalability. The panelists address diverse topics, such as avoiding over-engineering, operational efficiency, code ownership, and managing technical debt. They also discuss the societal implications of AI, data sensitivities, and the necessity for robust safeguards. The lively debate also covers the scalability of ML systems, method validation, co-ownership of projects, and the importance of good documentation practices. The panel sums up pointing out the need for the value of data work to align with company goals, and for technical professionals to bridge the gap between technical solutions and business needs. Finally, they respond to audience questions about model complexity and debt accumulation throughout production processes, sparking thoughts on tools and governance in development.
Join us at our first in-person conference on June 25 all about AI Quality: https://www.aiqualityconference.com/
Saroosh Shabbir [00:00:02]: Hi, everyone. Thank you so much for staying. We're gonna have a panel discussion, and the theme was supposed to go up on the slide. So it's basically operational efficiency and scalability of ML systems, particularly the purview of Genaid. And you've heard a lot about our speakers. I'll try to mention a few things that you may not heard in the introduction. So, first up we have Rebecca, and as you heard, she started her career at Izato and is now developing world. What you didn't hear is that she's also the co founder of Women in Data Science, which is an organization that focuses on increasing the representation of women in MLAi and data science related fields, and organizes events and training to that end.
Saroosh Shabbir [00:01:05]: But it's open for everyone, so you're welcome to join and listen in. And the presentations and events tend to be very high quality, so I would recommend them very much. And then we have MIa, which we heard is working at elastic for the last three years, and with that, basically working mostly with enterprise and public sector in Sweden and Finland on highly available architectures, focusing on search, observability and security use cases. And finally, we have Francesca, who has background in pure mathematics, has been working in the industry as data and AI ML engineer, most recently as Potarian and kings, as you heard. And the projects that she's been working with has been on natural language, language models and computer vision. And now she is developing tools and for the ML platform at things. So welcome again, and thank you very much for being here. So I want to start with the fact that we, as we delve into mlops, particularly in the area era of Genai, we are presented with unique challenges and opportunities.
Saroosh Shabbir [00:02:31]: One of them is the scalability of ML solutions. We know that good mlops tends to be lean and simple, which means that it's amenable to scalability. It makes it easy to scale to various use cases, and it has to be versatile enough for prototyping and testing also. So in your experience, particularly as you've been building your platform, Rebecca, how easy have you found these two spectrum side of the spectrum to manage, and what strategies have you been able to come.
Rebecka Storm [00:03:13]: Up with to try to tackle the solutions? Yeah, just to make sure I understand the question. Right, you're talking about the trade off between sort of simplicity and easy to use and sort of being complex enough to be useful, right? Yes. So I think one sort of important philosophy that has gone into how we both world is we want to. We want to hide a lot of complexity from users until they need it. So this is kind of one of our core design principles, and this is basically because of this exact repeat, because we think it really helps with this trade off. So, so our intention is to always build it such that if you want to do something that's like simple and straightforward and don't have, maybe you don't have a very technical background, or you just want to do something that's very like, vanilla and common, you shouldn't need to like deal with a lot of complex, like, settings and making a lot of decisions along the way. That should be easy. But then once you need to do something complex, it should be there.
Rebecka Storm [00:04:06]: And we'll try to make this a little more specific. So I think I mentioned in my talk that like, I think a common, like, best practice that most companies use, whether they use twirl or not, when you run python jobs, you typically want to run them in isolated containers so they don't end up in kind of dependency madness. And some users of twirl, they have no idea what a container is. So we want to make sure that their Python code still runs in an isolated container, but we don't want to force them to write the docker file. And we actually even go as far as to not even let them see a docker file, so we hide it. So all of your python jobs in twelve will always run in isolated docker containers, but you don't see a docker file. And we kind of by default give you a user docker image. And then the day that you kind of launch that level of control, so you want to add like a custom requirement, or you want to have like an entirely custom docker image, you just add like a Docker file.
Rebecka Storm [00:04:56]: So, like, I think to come back to like your question, I don't think it's necessarily a trade off. I think you can have systems that are easy to use and kind of complex enough to cover your needs. But I do think an important, like, design principle there, and this goes like when you go to the platform too, is to try to hide as much like strip away boilerplate code or docker files in this case, and kind of keep things as simple as possible, because that makes this whole existence much less intimidating to use. And of course.
Saroosh Shabbir [00:05:30]: What about your perspective, Francesca?
Francesca Carminati [00:05:34]: Yes. So I can say that MS system can actually scale like in different direction, right? You might have a vertical scaling, maybe you are using like more complex models. You might need more computational power, or you might need to serve more prediction daily, so you might need horizontal scaling. But what can also happen is that you instead basically start using an ensemble of models. So now you have more artifact to manage in production because now you start maybe with one model. So you have one model, one set, one data set, one set of hyper parameters, but then maybe you add another model and now basically the artifacts need to manage like double. But I think that for sure, as Rebecca said, you would like to start with a simple solution and then iterate and make it complex iteratively. However, in some cases I think we tend to focus too much on scaling and maybe we dedicate too much resources or too much thought into scaling before even having validated the solution actually as an answer to the business.
Francesca Carminati [00:06:48]: Because especially if you rely on cloud native solutions, you can actually scale your computational power or the amount of prediction need to serve per day pretty easily. But in some cases, actually what you really need to verify first is if your how you're approaching the solution does even having a positive impact on your business. Because for example, if you are, let's say you want to have MS system that basically helps you detecting defects like in manufacturing. And of course when you evaluate this model offline, when you're training it, you're going to use maybe some metrics that are just like a proxy from your real problem. Because the real problem you are trying to solve is for example, maybe detecting the speed for which you're detecting these defects, or how basically the money saves. So that's actually the real business metric that you want to optimize. But maybe your problem is framed in a wrong way from the start. So you end up maybe dedicating like an entire team ready to scale your solution for like million of predictions per day.
Francesca Carminati [00:08:12]: But then like while you go to production or realize you don't even have frame your problem correctly. So that's really important to, I mean to really think how if you really need the scaling to start with, or if you need to instead focus your like basically put your effort in something else first. If you need to validate something else occurs.
Saroosh Shabbir [00:08:36]: Do you think that happens more in the context of large enterprises perhaps? Or is that a problem that startups tend to run into at the same rate?
Anna Maria Modée [00:08:47]: Engineers in general, we think it's so fun that we tend to over engineer everything from the start. I think it's in our nature. It really is. I see it day to day. And that's kind of like we have to tell ourselves, does this really need to be as complex and custom as we think it should be? And to that end, like it's really you're just echoing what I see every day. And Rebecca, your design principles, they echo ours. So it really is about making it simple to start hide complexity until the user needs it. Because that is the key to getting speed to volume.
Anna Maria Modée [00:09:31]: It really is. But not having hidden so much that it's a black box that you can't actually customize when you need it. So there's a. There is a balance. But I think the key is just to not over engineer it.
Saroosh Shabbir [00:09:48]: What principles or strategies do you tend to keep in mind or introduce new ways of working for you to enable that not over engineer and make things more complex than it to be.
Anna Maria Modée [00:10:02]: I generally tend to recommend the best practice of is there a model out there that does the job use it or even better if it's being maintained by someone else so I don't have to do the job of that. Have a lifting of keeping it up to date. Is it supported by some of our licensed products? Even better, someone else is responsible and who I can hold accountable for it ownership of it. It really is. Do I need to build this and then utilizing the tools that we have in house. So do I need to bring in another tool to our already probably complex ecosystem or can I utilize the ones that we have? Just trying to keep it as simple as possible as a design principle, no matter if it's machine learning or. But I tend to see that machine learning engineers are breed of their own when it comes to creating custom complex processes.
Saroosh Shabbir [00:11:10]: Do I understand correctly you're saying that off the shelf is if it's good.
Anna Maria Modée [00:11:15]: Enough, if it does the job.
Saroosh Shabbir [00:11:17]: So.
Anna Maria Modée [00:11:18]: And there's the evaluation part that you need to do so. There's absolutely cases where you need to bring your own and create your own, but you should be mindful of evaluating what you already have and if that is good enough.
Rebecka Storm [00:11:33]: I'm a big fan of to avoid over engineering systems. I'm a big fan of like trying to build something that's end to end quickly as like humanly possible. And I think very often like the perception will be like, we can't like, you know, it is going to be like so much work to build this thing and but if you like really, really question yourself when you're asked like how quickly can we build something, I do think it's usually possible to do it quite early. What I think tends to be a little off putting about that is, and so just to clarify, like I think building something end to end early allows you to catch the kind of errors that Francesca was pointing out earlier. Like, you know, you realize your optimized for the wrong metric or something like that. That's the point of building ends end and very early. I think the reason that that's a little sort of off putting for a lot of people is it means the machine learning part isn't very exciting. Like if you're building something end to end and the idea is to keep it as simple as possible, that probably means no machinery like that probably means like implementing some super basic rule and testing that like the idea of like data all the way to action and that you can measure the results that you have that entirely.
Rebecka Storm [00:12:35]: That's probably kind of the first 30. And so as a machine learning engineer, and I say that this happens work as a machine learning engineer, that's like not so exciting from like a pure mo perspective. It's exciting from like a business value perspective. So for me that's both. And like, I mean you don't get to spend time on maintaining your model, which I think a lot of people tend to be excited about. And I think that's, that's sometimes will go back to like truly building it first and then optimizing and building an ML model and optimizing that and sometimes.
Anna Maria Modée [00:13:08]: Proving the value of having a model could be the reason to actually go the step to customizing and building your own.
Saroosh Shabbir [00:13:15]: I guess in your platform you tried to, since you tried to reduce the distance between analytics and ML, I guess you prioritize simple solutions as much as possible and that reduces the, what you would call as collaborative headwind between teams. But in terms of tech debt, how do you think that affects the organization? Because the solution you build on the analytics side might not be very robust on the ML side. How do you balance these things? Yeah.
Rebecka Storm [00:13:55]: I think what you're on to is like part of one reason companies don't always build like combined analytics and machine learning platforms, because they have very different types of requirements in terms of like reliability and like processing seed and things like that. I obviously, I'm a little biased here, but I obviously like firmly believe that it's possible to build a system that can do both. And tomorrow is kind of my answer to help do that. So. But it's not easy and it requires a lot of things that I mentioned in my talk. And I think specifically when it comes to technical depth, I do think the idea of ownership is super important. I think that's like a really powerful way of avoiding building up tons of like dead tables in your data warehouse that you're like, I have no idea who does this and like, you know, I have five on it, but no one knows of what this code does. Like, I think code owners is like, or.
Rebecka Storm [00:14:48]: Yeah, it doesn't depend matter if you're specifically using like a code owners feature in the table I suggested in my talks. But like, having clear ownership of all the components, I think that's a secret that you dedicate.
Saroosh Shabbir [00:15:01]: And then, Francesca, you highlighted that there's various kind of tech debt. One of the things that the paper that you mentioned also mentions is that all tech debt isn't necessarily bad, like, just like fiscal debt. I think we can have opinions about that. But what, in your opinion, is.
Rebecka Storm [00:15:25]: The.
Saroosh Shabbir [00:15:25]: Least amount of bad debt that you would accrue and which, which kind of debt? Like you mentioned, there's duplication, configuration. And on the consumer level, undeclared consumer, as Rebecca is also mentioning, where would you. Yeah, where would you risk having that, picking these aspects?
Francesca Carminati [00:15:48]: I mean, you can, you can get that at like every different phase of a workflow. I think one of the most affected, it's probably the experimental phase where you're actually like, because you're, when you're experimenting with a new model in your architecture, you can very, actually no limit of how many things you can try. And so if you need, and there is also no really a clear goal, you really don't. You want to maximize maybe a specific metric for you, for your model, but there is really not a clear goal or where you should end. But like, I would say, and I mean, technical debt can happen at any stage. It can happen in the data collection, it can be in your data pipelines, it can be everywhere, because, like, it can affect every single module in your system. I think that a really hard, like a pivotal point where there's like a lot of problems arising is when you try to go from experiment to production, because typically there are also different professionals with different kind of expertise that basically works on these two different areas. And so making sure that there is like a clear contract and all the artifacts and that are needed from the experimental phase, from experiments are managed appropriately in production, that's actually really hard and especially for how many of these artifacts are produced in different experiments.
Francesca Carminati [00:17:20]: So, yes, I would say this is probably where the most problem arise, but that's really, actually really important because you really want to ensure traceability. So you really want to know if you have prediction, the prediction that you have generated on a given moment. You want to trace them back to the experiments and the data of.
Rebecka Storm [00:17:43]: A.
Francesca Carminati [00:17:43]: Particular, like a particular experience, a particular version of your data and a particular set of hyper parameters, a particular version of your code. So that's really hard to do.
Saroosh Shabbir [00:17:55]: And Rebecca mentioned one of the features that you can use in GitHub, which is declaring who is the owner? Do you have any suggestions for any other kind of tooling or platforms that help in making this possible?
Francesca Carminati [00:18:12]: I mean, I would say having co owner for sure helps and having declared responsibility between the teams and making this contract expandable. But what I think it's very hard to generalize because it depends on what tooling different company uses. But usually it's really hard to balance as well flexibility with standardization, especially if you build tooling, it's really hard to provide at the same time like a golden path and provide a standard and say this is how we go from, from a trained model to a deployed one, but at the same time allow flexibility to basically allow data scientists to try whatever, even like state of the art architecture. So yeah, that's like an ongoing process, really. I don't have like a fixed answer because it depends, but tooling can help a lot if you identify what are the main areas where problems arise and what are basically our, the problem when in this massage, basically when you try to promote something, production and standardization was.
Anna Maria Modée [00:19:27]: Really something that hit me when you're speaking, because that is something that comes up again and again. And it's not just about standardization of processes, but just naming data types that really enables reusability. So if you want to get that scalability and reusing your model across different teams and really getting it working on an enterprise scalable level, I think just setting something as simple as having a schema for your company, it may sound simple, but that really is the secret sauce in a lot of different companies to making it work. So standardization is so boring for a lot of people, but so important. Yeah.
Francesca Carminati [00:20:13]: Or you might have like templates that generate code for standard project or templates for infrastructure as well, to avoid development and production mismatch. But there is a lot of things you can do, but they are very dependent on your context.
Saroosh Shabbir [00:20:30]: We also heard that, as you mentioned, that ML systems tend to be different than other software in terms of doing that. Going a bit further, do you think Mia, like Gen AI, is there even more different than ML in accruing tech debts or making things more complex in that situation?
Anna Maria Modée [00:20:53]: I think the tech depth is going to be, there's going to be a lot of projects out there based on Genai, but very unclear business values of why they are there. It's a fun gimmick but there's going to be a question eventually. Why is this here? So if that's not clearly defined early on, why we're doing something and someone is just saying we need Genai, that's a question that's going to come as a technician. Well, it is a technical depth because it's technically implemented, but it's going to be at business value. At the end of the day, I think that's something that Genai is going to generate a lot of us, but so what this.
Saroosh Shabbir [00:21:38]: Okay, so changing gears a little bit here. So we know that AI advancements push mlops to develop extensively and rapidly. It needs to adapt very quickly. But on the other side, have you seen mlops pushing the boundaries of AI or becoming catalyst for more AI development? Do you ever see the flow in that direction? I'm thinking, particularly for Jenning, are there mlops frameworks that are pushing AI to develop in a particular way?
Anna Maria Modée [00:22:19]: So when it comes to mlops, and.
Saroosh Shabbir [00:22:24]: I mean, there is this catalyst, right.
Anna Maria Modée [00:22:26]: Of just, and I can't really say, I mean, prediction of the future, what frameworks there might be. We are elastic, we're very agnostic to the frameworks being used. We have suggestions, we see trends. We never really say this is the framework. We suggest having a set framework, but standard one. I don't know if you have anything else to, to the.
Rebecka Storm [00:22:54]: No, not really. One thing that I thought is like striking, but I think maybe it's changing is at least how early on.
Anna Maria Modée [00:23:08]: It.
Rebecka Storm [00:23:09]: Seemed like the standard way of developing an AI application was to kind of do what works and then deploy, which kind of like convinced all of MLL traditional machine learning best practices, having training data and validation data and testing data, folding out the nigger samples, actually using metrics. I think in both better, but I think that's been interesting to see how AI has been partially treated as something that's very different from machine learning, when in my mind there's very similar.
Anna Maria Modée [00:23:46]: It's just a subset in my mind. Yeah, I think that you really came to an important thing. We don't have validation data for Genai, really. So a lot of the issues that I mentioned with hallucinations, that's going to prevail and that's going to be a blocker for people really trusting genaid, even though it's very cool and creates a lot of fast things, unless we can validate whatever is produced, it needs to have that extra step and will not be as production ready.
Saroosh Shabbir [00:24:26]: Can I say that? Yeah, I was actually getting to that. You mentioned that there are ways to fix the problem of grandma is hallucinating. But how do you find out grandma's hallucinating? Like how, what architecture do you use to figure out valuation and verification in the et cetera?
Anna Maria Modée [00:24:51]: Yeah, and that is one of the reasons why we've at least at elastic, have approached rag as context driven. So you're really telling the, the LLM the type of information you want to have in your answer. You just don't know exactly in the exact format generated. You provided a template and tell it.
Saroosh Shabbir [00:25:16]: What data to use to generate that.
Anna Maria Modée [00:25:18]: Template and only using that data. That is the way we've solved it with rag and elastic and it's proven to be very, very good. But we still in our AI assistant because we can do, and that is really, really cool. We can do, can we change this query from this language to this language? You can do translations directly in the EI system. We also have that little please note that this is a Genai tool. It's tech preview. Please validate the response. So there is that extra aspect of validation that is still to be solved.
Saroosh Shabbir [00:26:04]: What techniques are being used? I suppose there are ways to have like step or chained verification that makes you more confident in what the model is saying. Are there any other techniques that seem to be evolving or coming into play?
Anna Maria Modée [00:26:22]: I think the standard, the ones for with the test data set is still with llms. It's the standard practices for benchmarking different llms and genius still, the big data sets that are used across them and.
Saroosh Shabbir [00:26:43]: See how good they hit.
Anna Maria Modée [00:26:47]: We have some blogs around it. I can send it to anyone who's interested. But yeah, it is a big thing and you need the context driven approach. I think. I talked to some people in the break about how we, before the really good search engines became a thing, we were taught as end users how to Google. How do we get the actual answer from Google that we want? We needed to be good at really naming keywords. We were providing the syntax we were providing, and now we are expecting more from the search engines than before. And in the same way that syntax and context have changed from going to being directly in the search bar to being in the context bar that you provide when you send the data on to the guy.
Anna Maria Modée [00:27:51]: So yeah, that is, it's still there. It's just in a different place in the search.
Saroosh Shabbir [00:27:58]: I think I touched a bit about your favorite frameworks and practices. If you would like to highlight a little bit more what has helped you in your various projects all throughout your career. Like one thing that you managed to sort of always remember in any project that you've done, like your go to thing.
Rebecka Storm [00:28:24]: Are we talking about technical tools now?
Saroosh Shabbir [00:28:27]: Both what philosophy or tool or framework.
Rebecka Storm [00:28:32]: Philosophy and doing data work is like, to always think about the value. Like, that's been a big realization for me throughout the roles I've had, is it's so easy to do work that produces little or no value because someone asks and you're like, oh, I want to, like, make this nothing. They ask for it. So at least two important. And like, very, very often when you, like, ask one more time, like, okay, if I pull this data for you, what are you going to use it for? You realize that, you know, they were just curious, or like, someone wants the machine learning model to, like, influence metric, and you dig a little bit further and you realize even if I build a perfect model, there's like very limited impact, small problem. So those kinds of things, like, that's been really big. And it's like, regardless of the kind of data work, it doesn't matter if you're building a machine learning model or like a dashboard for analytics or some, like, system. You're doing data engineering.
Rebecka Storm [00:29:28]: You're building a system for like, processing data. Like, that question is also always relevant, like, how, how valuable will this be if I build it? Is it like, really worth my time?
Saroosh Shabbir [00:29:37]: That I think is an important question. Is that limited to business value or do you interpret valuable?
Rebecka Storm [00:29:43]: Yeah, this is the hard part. How do you measure value? I don't have a great answer. I think. I think what's important is just thinking about value at all. And I think it's going to be super complex dependent at some companies. Like, it makes sense to always measure it in money. You know, that's like the one common denominator. If you're looking with like three departments, like, you might be able to load them for different metrics, but, you know, if you translate the value into money, you can compare between different types of projects.
Rebecka Storm [00:30:09]: Not saying that's like, always the right way. Again, it's, it's so complex dependent in terms of, like, what you're trying to achieve. But, but at least, like, whatever definition of value you choose should be aligned with kind of what is your company trying to achieve? Because otherwise, like, it's value is like having a great system. Like, that's probably not at all a good point.
Francesca Carminati [00:30:29]: Yes. I could say one thing that really helps. Having, like, a standard evaluation process that basically does not necessarily entail having. It's not necessarily technical, but like, at every phase of your. Of your project, there's. At the end of every phase, there needs to be some quality question regarding if at that stage, your project is, like, actually the best way that you could have, and it started at the very beginning, like, before even you start coding, before even the data scientists start experimenting. For example, there should be, like, a feasibility study, like, do we have, that encompass different areas? Right. For example, is there, like, a strong business reason for this project? Because if there is not, like, there is not going to be any effort from the organization in order to push it forward, or, like, is there a quality in the data? Is this solution? It's possible to frame this solution in a ML way from, like, a business problem to, like, an ML problem.
Francesca Carminati [00:31:32]: And then once you have validated that you actually, your project is, like, feasible in the first set, you. Then you continue, like, in the second set. In the second phase, which could be, for example, experimentation. Experimentation, you just need to set, like, specific metrics. For example, in that case, and you say, before we even proceed to thinking about production, we want to reach this threshold for the metrics that we think are the best proxy for the business metric. And then when you move to the next stage, which may be maybe, for example, maybe testing, you need to set before, like, okay, this is, like, the result that I. I need to reach this kind of value in my a b test to even think about scaling the project to, like, all to really, basically, organization and whole prediction, I actually need. So, and this standardized process of validation that in the experimentation phase, in the evaluation phase and deployment should actually be defined, like, possibly with, like, defined specific metrics that should be checked.
Francesca Carminati [00:32:46]: This should be repeated every time you do an iteration of the same project. So that's really help to basically understand if you are basically pushing forward something that actually might not have value in the end, you might still have this risk, you might still have, like, a project in the end, that is not really meaningful to the business. But at least you have, like, a set of rules that kind of mitigate this risk and put a lot of effort, or maybe many, many months of.
Anna Maria Modée [00:33:15]: Work.
Francesca Carminati [00:33:17]: Into something, then it's not really valuable.
Anna Maria Modée [00:33:20]: Yeah. And for me, it's been, and always has been solution oriented. So I like solving problems. And initially, as an engineer, it's been technical problems. How do I solve this riddle with an algorithm, with an implementation? How do I break it then? But going into my profession, it's been obvious that there's problems who are more business aligned, and then the job has been bridging that technical solution and almost translating it through KPI's to the business owners. So a lot of the time I see engineers doing amazing work, but they can't explain how important that work is or how much their team has improved over time. So measuring improvements and having clear expectations that can be understood by business. That is so interesting and very fun to work with the engineers.
Anna Maria Modée [00:34:29]: So I'm fortunate enough to work with and just helping them understand how to explain their success in a way that makes it understandable for a non technical person.
Saroosh Shabbir [00:34:46]: Do you think it's shorter distance for engineers to be better at communication for that kind of problem or for business leaders to born a big mobile?
Anna Maria Modée [00:34:57]: I don't think that necessarily they need to learn each other's and start overlapping in the understanding. I think there's more an understanding in we measure things differently, but having that standard, in that contract, in how we measure it and how we measure success, that is invaluable. And then keeping project evaluation going. So for the technical depth, it could be just starting to understand when an ongoing project that has existing for a long time, that has a lot of dependencies when there is an actual need for. Okay, we have incurred this technical debt enough, so we need to go in and put hours into optimizing it, because it is that important to the business and we can measure it. And that having that in place from the beginning really saves a lot of time and bottlenecks.
Saroosh Shabbir [00:35:55]: Now it's time to open questions from the audience.
Question 1 [00:36:00]: I liked the last talk because it made me feel a little bit bad conscience. And then I thought that the other two talks, you're basically the solutions to this bad conscience. So let's take a purely hypothetical example of a model being built by a company. Maybe Sarish built the first model, and then somebody else takes over it. And then I hand it over to Finnick, and we all add to the complexity and to the debt all the time. And then somebody else brings in a stadium to work for this model, and we realize that nobody can deal with it anymore. So how do we solve it? And can I blend the student with this?
Saroosh Shabbir [00:36:48]: Is it the germ model?
Anna Maria Modée [00:36:51]: That's when you get someone to do masters master thesis.
Question 1 [00:36:57]: How should we use tools? Or, I don't know, owning code? How do we do it so that we keep this in check all through the development process?
Francesca Carminati [00:37:11]: Well, first of all, it should be like a shared responsibility, so. But I think that there should be like an effort that might be a team effort, or if we are talking about collaboration between different team or organizations, should be like organization effort in order to set standards and basically set what are your, what tooling are you using, what are your practices and how you make changes to your system. And you should not deviate from those changes unless there is very good reason to do so. Another thing that's very useful, it could be if you identify that there is a problem with a recurring problem, you might have maybe templates in your code reviews with points to check and say so every time, like every time that I hope you open a VR and you can make explicit that these are points and needs to be checked before we move forward, basically. So that helps a lot. And then of course, it is an ongoing effort, because if you see, for example, that you can package your code in libraries, I think you need to make some time in your schedule in order to do so, because it seems like it's taking time away from development, but like long term it really helps. And then it needs to be also good documentation and updated as well, which also, it's a very boring topic and hurts, but it's needed. So.
Rebecka Storm [00:38:56]: But I would also, I would also consider a more drastic solution, which is killing this model. I think we should kill more energy learning models than deadwell. And I think we should celebrate when we're able to, like, I think if you get to that state of like, something that's like, clearly not maintained and like Nolan has insight into, then that's, I think, clear indication this might not be physics critical, it might not be providing that much value, because if it was, there would have been a clear hand over. So I would also, I would almost use like, the situation as a signal that like, maybe I should be questioning the existence.
Anna Maria Modée [00:39:28]: Yeah, we will all celebrate when Kobel is standing in the classroom.
Rebecka Storm [00:39:35]: But I.
Anna Maria Modée [00:39:35]: Think both of you are on a good thing. So for you, Francesca, I always imagine, like, how would I like this?
Rebecka Storm [00:39:44]: If I have a shared thing, like.
Anna Maria Modée [00:39:45]: A shared space, like a kitchen, a room, how would I like to enter that room? Do I want, do I expect there to be a cleaner who goes up and takes away all the dishes and just a dishwasher? Is that part of our shared responsibility, that kitchen that is so deeply forgotten at some companies and no one wants to enter, but everyone wants a pot of coffee? I think that is really a good metaphor. So leave it the way you would like to have it received, ideally in a cleaner state than you would usually receive them, just to set a precedent. Because if someone starts lacking, if someone starts putting trash in the corner, everyone will start putting trash in the corner. So it really is a culture. And we should definitely kill more models.
Francesca Carminati [00:40:38]: But it should be hard for the wrong things. So enforce it. You can use precommit works, for example. Well, and your checks, like, should be hard to do the wrong thing so.
Rebecka Storm [00:40:49]: People don't do it because it's not only. Yeah, I like that. You're just great. I enjoy that.
Saroosh Shabbir [00:41:00]: I think.
Rebecka Storm [00:41:03]: I think parts of what you say I really agree with. Like, I think it's super important that other people than the code owners can go in and suggest changes to the code. Like, I absolutely think, like hiding it, not even getting like read access. I think that's the terrible. So I'm all for that. I think it's important that other teams can come in and kind of suggest changes and so on. But I think, I think what's important is the ownership part, so that you have one theme or one individual that's like responsible for making the change, for making sure that it won't break anything and stuff like that. So only the not having to do all the work is what I mean when I talk about, and I think like the situation you described where it's sort of that the angle person just blocks any objects.
Rebecka Storm [00:41:48]: That sounds terrible, but that sounds like a cultural problem to me, rather than a technical. I don't think that kind of problems can be solved with technical issues.
Saroosh Shabbir [00:42:07]: Yeah, yeah.
Rebecka Storm [00:42:08]: Of course, I'm all for like good testing, and yes, I think that can mitigate sort of some kinds of breakage that you might see. But I also think it's hard to think of everything. Like, no matter what kind of test you set up, no matter how good your test publishes, I think it's very hard to test for everything. And so I think the people, I.
Anna Maria Modée [00:42:28]: Think the code artists should typically be.
Rebecka Storm [00:42:29]: The people develop the code, but there might be exceptions to those of it, but the assembly should be someone else. They should be people who are experts and think about what it's value. I think they will typically have. It's not like knowledge. They'll at least have like intuition around what might belong. So I think there's value in how to get people involved.
Question 2 [00:42:48]: In addition, I will ask a question. I've got two questions. Basically, the first one is a generic question. First of all, very good talks on AI and machine learning. I work for elastic, by the way, so I work with Mia closely, actually. It's just interesting. And I'm just thinking of taking advantage of this time that we put experts in the room to get this your perspective. We talk in AI we talk machine learning.
Question 2 [00:43:17]: I work for elastic between so much at the moment in the generalized space. Right. My question first of all, and I'm just interested to get your opinions, is from a society point of view of the friends and family that ask me, like this AI noise that's happening, right. Of what relevance is it to society? First of all, where I'm coming from with that is I'm looking at the talks and most of them focusing on business use cases, enterprise use cases, and it totally makes sense, I get it. Right. But from a layman point of view, I just want to get your opinion. Why does this matter from a societal point of view? And also in addition to that, I think there was an example about Google as well. When Google came into the market, it changed how we looked at data, it changed how easy it was to find data.
Question 2 [00:44:08]: But then even today, an individual can go on Google, do a search and get relevant context. Are we seeing the same applications of AI and machine learning impacting society at that personal level as well? First of all. And then the second question also is, what's your viewpoint on AI and machine learning from the perspective of. Sounds to me, and I'm also an engineer, but I kind of feel like we're working so much on finding solutions to who, like where problems are not clearly defined. Right. Is that a fair statement or are there enough problems and we're trying to find solutions to those problems. So is it more working on a solution than looking for problems to that solution? Or are we more focusing on these problems and we're trying to use machine learning and AI to solve those problems? Thank you.
Francesca Carminati [00:45:07]: I'm really not the best person to answer this question. I don't think my perspective is as broad as it needs to be, should be, in order to answer this question appropriately. So I can just say that. I mean, of course, like on an individual level, even if we. I mean, on an individual level, of course we're going to see an impact of AI on the lives of everyone. Personally, what I try to do with the people around me is just to make them understand a bit better what AI it is and what AI is not. But this is just on the personal, personal level and like on. Yes, on my family around me that really ask me questions that's.
Francesca Carminati [00:45:50]: But again, I'm not the best person to give, like such, like such a, let's say a perspective on a topic that is important and like this.
Rebecka Storm [00:46:01]: So basically, I'll chime in, but also, like you asked, sort of how you explain the relevance of AI I think when I have conversations with like friends and family that are sort of not so technical or in the data space, I don't get asked like, why is this important? Why does it matter? I get asked like, will it kill us? It's like everyone seems so convinced already that, you know, Al changed the world and they're worried about like impacts and I mean, they're like serious questions about, you know, what form of income. And so I tend to do exactly what you do, like focus on like, you know, trying to kind of bring down the hype a little bit because people talk about AI as if it's like a person keep that as like secret agenda. And I like to talk about, you know, here's how mission learning works and like, you know, talk about AI. Here's actually what we're talking about. Here's what these models, like, try to make it much more tangible. And I think that helps. I think this is like perception that, yeah, AI is like this terrible beast, something that will take over all. But I think it helps to just like share my knowledge of what it actually is.
Rebecka Storm [00:47:04]: Like what is the deep low level? That's usually my approach.
Anna Maria Modée [00:47:09]: I think probably a few movie franchises have helped in that aspect. But yeah, AI will not kill anyone. And I think that's probably the most prevalent misunderstanding about among layman's. But maybe when it comes to defense, there's a lot of philosophical questions about, for example, use of drones and automated systems in, yeah, in weapons, for example. Maybe those are more applicable to philosophical discussions on how it's going to change society as a whole. From a search perspective, I think what's driving technology is actually the expectations from the end users. So when we, when I said earlier how we were taught how to, how to Google, but that's now put into the engineer to put a context in, for example, RaC to give the most relevant user and then letting the LLM generate that answer. We're still, when it comes to semantic search, that is now the expectation from the end user.
Anna Maria Modée [00:48:22]: They expect to have custom made recommendations. If they have a user profile at an e commerce retailer tailor, for example, they want to get tailored content recommended for them rather than generic, or maybe they don't in some aspects and opt out. So I think it's more those type of aspects that we are seeing more day to day tailored recommendations, primarily commerce for the most common people. But yeah, that is just my observation almost. Second question, Morgan. I think Rebecca actually answered it pretty well. There's a lot of people who are curious but don't actually have an application for it. It's like, oh, what's this data? What should I do with it? I think that's pretty common, honestly.
Anna Maria Modée [00:49:22]: So we're mostly just happy engineers and sometimes we hit the mark and find something that, I mean you need to be curious, but then you need to be able to kill your baby if it's not actually bringing value. That goes for any code project you do not specifically machine learning, I think.
Francesca Carminati [00:49:44]: Yeah.
Anna Maria Modée [00:49:44]: So that is multiple sense.
Question 3 [00:49:47]: So my question is very simple. So do you think that JNi based applications are ready to be placed by public in case of sensitive data? So there are two notes that I can think of. One is that, I mean, there are different security techniques that people are using. People can use to speak out information that they're not supposed to have access to. Maybe that is of a different person or something like that. So how do we say part of what are the security measures that are possible? So we previously used that type of things. Now we can have copy from the engineering attacks. That is at first thing with NAI system and the second being that that is, although we have lag based concept, but there's still input.
Question 3 [00:50:37]: There is no guarantee that the same input will generate same output. There is always probabilistic model, then there is always a risk, but it will stick out to you. What are your views in that case that our genius models are ready to keep up, although we have packed of.
Anna Maria Modée [00:50:57]: So you will get a very elastic answer. It depends. So if your data that's being queried is already publicly available, there's no reason why not. It just makes it easier for to consume, easier to find, easier to understand. When it comes to secret data, you still have to use safeguards and on different levels. Authorization authentication you can use role level or in elastic case document level, field level security. Make sure that the role permissions are correct. Very boring stuff.
Anna Maria Modée [00:51:39]: And then you can of course before if you want to avoid things being leaked. If you do query it and use it in production, but using private data, sending it to a public LLM and then getting it back, you can anonymize the fields. So you can just tell the LLM what type of field it is, but you don't. You make sure not to send the actual data and then de anonymize it for the end user who then receives the oh, fill in this field in space X for example. But that is something that needs to be if you're creating your own rag that is very important to keep track on and just be mindful of your data if it's not supposed to be publicly facing, rather know your security level. I work a lot with the different defense ministries across Europe and there applications, they would never send anything classified because they are in a completely offline environment. So there are levels of. So it comes down to knowing your data.
Anna Maria Modée [00:52:55]: And surprisingly often large enterprises don't know the data. They don't know what's in it. They have a big data lake which composes is composed of sometimes or quite often private information that shouldn't be there, but they don't know it's there, so they don't know to protect it. That is.
Francesca Carminati [00:53:16]: Yeah.
Anna Maria Modée [00:53:16]: So it's absolutely production ready, but you need to know the data you have and if you need to safeguard it.
Question 4 [00:53:25]: All right, in that case, this is a wrap. I need to put my four year old kid bed. So this is a wrap for everybody. So thank you so very much, Rebecca, Francesca, Mia, Saraje for this great content. Thank you all for attending. You guys are like the hardcore group. Those that stay on the longest are also the most valuable people to get responses for. So please provide feedback.
Question 4 [00:53:48]: Just Poppix if there's anything you would like to hear more about, less of, or you know, how can we change this community around, please let us know. Right co creative thing. So with that said, thank you everybody for joining.